1. Domain-general and domain-specific accounts of human cognition
“Animal behavior is driven by instincts, whereas human beings behave rationally.” Views like these are still commonly expressed and deeply anchored in the Western worldview (e.g., Pinker Reference Pinker2010). A modern version of this dichotomy construes animals as having domain-specific, modular cognitive adaptations, whereas humans have domain-general intelligence. However, we now know that in human cognition, domain-specific components are ubiquitous too (Cosmides & Tooby Reference Cosmides and Tooby2013), perhaps even in complex cognitive tasks such as logical inference (Cosmides et al. Reference Cosmides, Barrett and Tooby2010) or solving Bayesian probability problems (Lesage et al. Reference Lesage, Navarrete and De Neys2013). At the same time, much evidence has accumulated that nonhuman minds are not exclusively made up of domain-specific specializations, but that domain-general cognitive processes may also be widespread. These empirical findings have implications for contemporary theories of the evolution of general intelligence, highlighted in section 3, provided it is established that general intelligence in animals is both real and refers to the same construct as in humans.
The evolution of general intelligence poses a major puzzle. Because modular systems may readily evolve (Pavlicev & Wagner Reference Pavlicev and Wagner2012; Schlosser & Wagner Reference Schlosser and Wagner2004; Shettleworth Reference Shettleworth2012b; but see Anderson & Finlay Reference Anderson and Finlay2014; Lefebvre Reference Lefebvre2014), the evolution of the mind as a set of domain-specific adaptations or modules can easily be imagined. Indeed, a small set of dedicated modules, without any domain-general cognitive abilities, to which additional modules can be added as needed, may be the ancestral state of vertebrate cognition. This perspective is so convincing that it has led to accounts of massive modularity, not only for animal cognition, but also for human cognition as well (reviews: Barrett Reference Barrett, Zeigler-Hill, Welling and Schackelford2015; Frankenhuis & Ploeger Reference Frankenhuis and Ploeger2007; Hufendiek & Wild Reference Hufendiek, Wild and Perler2015).
Evolutionary pathways leading to the emergence of domain-general cognitive processes, on the other hand, may appear less straightforward, because such open-content processes translate far less reliably into fitness-enhancing behavior, and because they may also require disproportionate amounts of energetically costly brain tissue compared to domain-specific specializations (van Schaik et al. Reference van Schaik, Isler and Burkart2012). Consequently, compared to the evolution of additional cheap and reliable, domain-specific, specialized cognitive solutions to specific problems, the evolution of general cognitive processes might pose greater obstacles to natural selection. Nonetheless, humans possess general intelligence, and if general intelligence can also be found in nonhuman animals, we can attempt to identify the evolutionary processes that can lead to its emergence, including the specific case of humans.
The aims of this review are (1) to critically evaluate the evidence for general intelligence in nonhuman animals, and (2) to explore the implications of its presence in nonhumans for current theories of cognitive evolution. To achieve these aims, we will review the theoretical background and evidence from a variety of research traditions, such as animal behavior and psychology, psychometrics and developmental psychology, and evolutionary psychology. Whereas all of these fields share an interest in understanding how the mind works, they are not well integrated, and attempts at integration have not yet produced consensus (e.g., Eraña Reference Eraña2012; Evans Reference Evans2011; Reference Evans2013; Toates Reference Toates2005). In this target article, we will therefore selectively focus on those aspects that are necessary to integrate the findings from animal studies on general intelligence with what is known about humans. As non-experts in several of these fields, we are aware that we may not fully represent all of the relevant aspects of the respective theories, let alone solve current controversies in individual fields. Nevertheless, we hope that this article serves as a first step in achieving the much-needed integration across these disciplines at a more fine-grained level, which will eventually enable the development of a more unified theory of cognitive evolution.
This article is structured as follows. We first briefly review conceptualizations of both domain-generality and domain specificity of human cognition, and use this as background to situate current evidence for general intelligence in nonhuman animals, which is increasingly reported in various species based on factor-analytical approaches. We examine alternative explanations for these findings and develop a set of empirical criteria to investigate to what extent a statistically derived psychometric factor does indeed correspond to general intelligence as broadly defined. Such criteria are increasingly met in rodent studies but are strikingly underexplored in primates or birds.
Next, we discuss different evolutionary theories that may explain why and how general intelligence can be widespread in nonhuman animals even though it is not immediately obvious how it can reliably produce fitness-enhancing behavior. We argue that the broad version of the cultural intelligence approach (Tomasello Reference Tomasello1999; van Schaik & Burkart Reference van Schaik and Burkart2011; van Schaik et al. Reference van Schaik, Isler and Burkart2012) can best account for the current body of evidence. We end by proposing a model that construes the mind of both humans and nonhuman vertebrates as a mix of truly modular skills and seemingly modular skills that are ontogenetically constructed using general intelligence abilities. We refer to them as primary and secondary modules, respectively. Species differences are likely with regard to the importance of these components, and we formulate tests to quantify their strength.
1.1. The positive manifold and general intelligence
Intelligence in humans has been intensely studied for more than a century (e.g., reviewed in Deary et al. Reference Deary, Penke and Johnson2010; Nisbett et al. Reference Nisbett, Aronson, Blair, Dickens, Flynn, Halpern and Turkheimer2012). It is broadly defined as involving “the ability to reason, plan, solve problems, think abstractly, comprehend complex ideas, learn quickly and learn from experience. It is thus not merely book learning, a narrow academic skill, or test-taking smarts. Rather it reflects a broader and deeper capability for comprehending our surroundings – ‘catching on,’ ‘making sense’ of things, or ‘figuring out what to do’” (Gottfredson Reference Gottfredson1997, p. 13). This definition has received broad acceptance (Nisbett et al. Reference Nisbett, Aronson, Blair, Dickens, Flynn, Halpern and Turkheimer2012). In animals, intelligence is thought to involve an individual's ability to acquire new knowledge from interactions with the physical or social environment, use this knowledge to organize effective behavior in both familiar and novel contexts, and engage with and solve novel problems (Byrne Reference Byrne, Slater and Halliday1994; Rumbaugh & Washburn Reference Rumbaugh and Washburn2003; Yoerg Reference Yoerg2001). Thus, general intelligence, as defined in either humans or nonhuman animals, stresses reasoning ability and behavioral flexibility.
The concept of human general intelligence is built on one of the most replicated findings in differential psychology. In humans, performance across tasks of different cognitive domains is positively correlated: the positive manifold. Factor-analytical procedures applied to large data sets of individual performance across tasks consistently reveal a single factor that loads positively overall and can explain a significant amount of variation, often termed g for (psychometric) general intelligence. Within this psychometric, factor-analytical approach, an individual's loading on this factor thus estimates its intelligence. Performance in specific cognitive tasks (e.g., Raven's Progressive Matrices) or test batteries (e.g., Wechsler Adult Intelligence Scale [WAIS]) is highly correlated with g, and is in fact often used as a proxy measure for it, for instance in studies aimed at localizing g in the brain (Burgess et al. Reference Burgess, Gray, Conway and Braver2011; Colom et al. Reference Colom, Jung and Haier2006; Gläscher et al. Reference Gläscher, Rudrauf, Colom, Paul, Tranel, Damasio and Adolphs2010). In this article, we will speak of general intelligence when referring to the broad definition of Gottfredson (Reference Gottfredson1997) that stresses reasoning ability and behavioral flexibility, and of psychometric intelligence when referring to the entity estimated by the psychometric variable g. For humans, it is generally assumed that g estimates general intelligence, based on the strong empirical correlations between the two, as reviewed below.
Psychometric intelligence, estimated through g, typically explains around 40% of variance in test performance, whereas the rest is explained by group factors and variance unique to specific tasks (Plomin Reference Plomin2001). It has been found that g in humans has a clear genetic foundation (Davies et al. Reference Davies, Tenesa, Payton, Yang, Harris, Liewald, Liewald D, Le Hellard, Christoforou, Luciano, McGhee, Lopez, Gow, Corley, Redmond, Fox, Haggarty, Whalley, McNeill, Goddard, Espeseth, Lundervold, Reinvang, Pickles, Steen, Ollier, Porteous, Horan, Starr, Pendleton, Visscher and Deary2011), and in the absence of adverse environments that compromise the complete deployment of an individual's capacity, heritability can explain remarkably high proportions of variance (Joshi et al. Reference Joshi, Esko, Mattsson, Eklund, Gandin, Nutile, Jackson, Schurmann, Smith, Zhang, Okada, Stančáková, Faul, Zhao, Bartz, Concas, Franceschini, Enroth, Vitart and Trompet2015; Nisbett et al. Reference Nisbett, Aronson, Blair, Dickens, Flynn, Halpern and Turkheimer2012). Furthermore, g has robust correlates in brain structure and function, such as brain size, gray matter substance, cortical thickness, or processing efficiency (Deary et al. Reference Deary, Penke and Johnson2010; Jung & Haier Reference Jung and Haier2007). However, rather than being localized in specific brain parts, it seems to be a system-level property of the brain (Pietschnig et al. Reference Pietschnig, Penke, Wicherts, Zeiler and Voracek2015). Finally, g is also a good predictor for various measures of life outcome, including school achievement, the probability of being in professional careers, occupational attainment, job performance, social mobility, and even health and survival. In particular, it is better at predicting such variables than specific cognitive abilities on their own (reviewed in Deary et al. Reference Deary, Penke and Johnson2010; Reeve Reference Reeve2004).
1.1.1. The structure of cognition
The structure of human cognition continues to be debated (e.g., Ortiz Reference Ortiz, Goldstein, Princiotta and Naglieri2015). Nonetheless, the presence of g is now widely accepted due to the pervasive evidence from Carroll's (Reference Carroll1993) seminal meta-analysis of over 460 carefully selected data sets on human cognitive ability. An influential account is Horn and Cattell's fluid-crystallized gf-gc model (see also Major et al. Reference Major, Johnson and Deary2012). Fluid intelligence gf refers to the capacity to think logically and solve problems in novel situations independently of previously acquired knowledge, and to identify patterns and relationships, whereas crystallized intelligence gc refers to the ability to use skills, knowledge, and experience and crucially relies on accessing information from long-term memory. An explicit causal link from gf to gc is provided by investment theory (Cattell Reference Cattell1987), which is the developmental version of the gf-gc model and finds considerable empirical support (Thorsen et al. Reference Thorsen, Gustafsson and Cliffordson2014).
An integrated version, the so-called CHC (Cattell-Horn-Carroll) theory, has been supported by several studies and is a widely accepted consensus model (McGrew Reference McGrew2009). The CHC model is hierarchical, placing a general factor g at the top, which affects both gf and gc. Most current models involve some hierarchical structure involving a general factor, g, and fluid intelligence, gf (but see, for instance, Bartholomew et al. Reference Bartholomew, Deary and Lawn2009; Major et al. Reference Major, Johnson and Deary2012; van der Maas et al. Reference van der Maas, Dolan, Grasman, Wicherts, Huizenga and Raijmakers2006). In fact, some have argued that gf and g represent the same entity (Kan et al. Reference Kan, Kievit, Dolan and van der Maas2011), and the previously mentioned definition of intelligence in a broad sense in fact emphasizes elements of both constructs.
Some models of general intelligence that do not involve g are also still being considered. Van der Maas et al. (2006), for instance, have presented a dynamic model of general intelligence that assumes independent cognitive processes early in ontogeny. Over the course of development, the positive manifold emerges because of mutually beneficial interactions between these initially independent processes. To the extent that one agrees to equate general intelligence with the positive manifold, the mutualism model may be viewed as a model of general intelligence for human and nonhuman animals in which variation between species would reflect the extent to which mutually beneficial interactions between cognitive processes arise during development. Because, across species, bigger brains require more time to mature than smaller brains (Schuppli et al. Reference Schuppli, Isler and van Schaik2012), and thus have more opportunities to develop such mutually beneficial interactions, such a scenario is compatible with an evolutionary perspective.
1.1.2. Executive functions and intelligence
Closely related to general intelligence are executive functions, or EFs (Barbey et al. Reference Barbey, Colom, Solomon, Krueger, Forbes and Grafman2012; Blair Reference Blair2006). EFs refer to “general-purpose control mechanisms that modulate the operation of various cognitive subprocesses and thereby regulate the dynamics of human cognition” (Miyake et al. Reference Miyake, Friedman, Emerson, Witzki, Howerter and Wager2000, p. 50). In other words, they are “a family of top-down mental processes needed when you have to concentrate and pay attention, when going on automatic or relying on instinct or intuition would be ill-advised, insufficient, or impossible” (Diamond Reference Diamond2013, p. 136). Three core EFs can be distinguished, namely inhibitory control (behavioral inhibition, cognitive inhibition, and selective attention), working memory (Baddeley Reference Baddeley2010), and cognitive flexibility.
Various measures of EFs have shown strong correlations with g/gf. Whereas the average correlation between working memory and g is 0.72, in some studies using latent variable analysis, it even reached identity (Colom et al. Reference Colom, Abad, Rebollo and Shih2005; Nisbett et al. Reference Nisbett, Aronson, Blair, Dickens, Flynn, Halpern and Turkheimer2012), leading some authors to suggest that the two cannot be distinguished from each other (Royall & Palmer Reference Royall and Palmer2014). That g and EF are closely related is consistent with two further lines of evidence. First, working memory can be trained, and these training gains can translate into gains in general intelligence even though not all procedures are effective, and it is not always clear whether the training affects working memory per se or instead improves learning strategies (reviewed in Klingberg Reference Klingberg2010; Morrison & Chein Reference Morrison and Chein2011; Nisbett et al. Reference Nisbett, Aronson, Blair, Dickens, Flynn, Halpern and Turkheimer2012; Shipstead et al. Reference Shipstead, Redick and Engle2012). Second, growing up bilingually, which makes high demands on a variety of EFs on a routine basis, is associated with stronger EFs in non-linguistic contexts, and thus with g (Abutalebi & Clahsen Reference Abutalebi and Clahsen2015; Bialystok et al. Reference Bialystok, Craik and Luk2012; Rabipour & Raz Reference Rabipour and Raz2012). Nonetheless, because EFs do not provide the logical problem-solving functions and learning that are the hallmark of general intelligence (Embretson Reference Embretson1995), some aspects of general intelligence are independent of EFs.
In sum, evidence for domain-general intelligence in humans, estimated by the first factor derived in psychometric, factor-analytical approaches, is pervasive, and is backed up by neurobiological evidence and various correlates of life-outcome measures. The psychometrically derived g factor is thus consistent with the broad notion of general intelligence, which stresses reasoning ability and behavioral flexibility and invokes cognitive processes such as learning and remembering, planning, and executive functions. This conclusion raises the question of the evolutionary origin of general intelligence in humans, which we will address by reviewing recent developments in the nonhuman literature. To do so, we will review evidence for g in animals, and whether it is warranted to assume that g in animals is also consistent with a broader notion of general intelligence.
Intelligent behavior needs to be distinguished from behavior that may appear intelligent but lacks flexibility (Shettleworth Reference Shettleworth, Vonk and Shackelford2012a). Intelligent behavior in animals is often referred to as behavior that shows some degree of flexibility and emanates from some kind of mental representation rather than immediate perception only (Tomasello & Call Reference Tomasello and Call1997). For instance, when digging wasps are interrupted anywhere in the sequence of actions involved in measuring the size of a hole to place a larva together with a prey item into it, they must start again at the very beginning of the behavioral sequence (Wooldridge Reference Wooldridge1968). Thus, many behaviors that at first sight look like they are the product of reasoning or learning turn out to be inflexible adaptations or modules (Sherry Reference Sherry2006). A collection of such dedicated adaptations presumably represents the ancestral state (e.g., Shettleworth Reference Shettleworth, Vonk and Shackelford2012a; Reference Shettleworth2012b), and thus the null model against which the hypothesis of general intelligence has to be tested. Before turning to nonhuman animals, we will therefore provide an overview of domain-specific, modular conceptions of the mind that have been put forward particularly, but not exclusively, by evolutionary psychologists.
1.2. Cognitive adaptations and domain specificity
A domain-general factor of intelligence can be contrasted with domain-specific cognitive mechanisms or adaptive specializations (Cosmides & Tooby Reference Cosmides, Tooby, Sternberg and Kaufman2002). The basic idea is that whenever a fitness-relevant cognitive problem arises repeatedly and predictably over long periods of time in a given species, natural selection favors a genetically based, developmentally canalized (“hardwired”) solution to this problem. For instance, natural selection may provide a species with a particularly strong spatial memory to retrieve stored food, without endowing it with more-powerful cognitive capacities in other contexts (Sherry Reference Sherry2006). Importantly, domain-specific mechanisms cannot be used in domains other than the ones for which they evolved, whereas domain-general mechanisms can be used to solve problems across domains.
Thus, the mind of animals, including humans, can be conceived of as a collection of adaptive specializations, often construed as modules, each of which evolved to solve a specific adaptive problem (Duchaine et al. Reference Duchaine, Cosmides and Tooby2001). Notice that a mind uniquely made up of these kinds of specific adaptations is arguably incompatible with standard accounts of intelligence, because virtually no learning and flexibility are involved. Similarly, none of these specific cognitive adaptations require the presence of the domain-general processes underlying intelligence such as executive functions.
1.2.1. Modularity and general intelligence
A modular organization of mind is particularly appealing to evolutionary thinking because modular systems allow parts to be removed, added, or modified without affecting the function of the structure as a whole. Therefore, modular systems may be more evolvable or even the only evolvable systems (Clune et al. Reference Clune, Mouret and Lipson2013; Pavlicev & Wagner Reference Pavlicev and Wagner2012; Ploeger & Galis Reference Ploeger and Galis2011; Schlosser & Wagner Reference Schlosser and Wagner2004; Shettleworth Reference Shettleworth2012b). Thus, whenever conditions are sufficiently stable or at least predictable across generations, natural selection should favor solving recurrent fitness problems via modules rather than via general cognitive processes, because the former solve these problems on average quickly, effortlessly, and efficiently (Cosmides et al. Reference Cosmides, Barrett and Tooby2010) and can presumably evolve more readily. General intelligence, in contrast, is thus expected to evolve under conditions of social or environmental unpredictability. Solutions to these evolutionarily novel problems have to be acquired effortfully, via slow learning (e.g., Geary Reference Geary2005; Geary & Huffman Reference Geary and Huffman2002).
The advantages of a modular solution to recurrent fitness problems, however, are not necessarily as straightforward. First, the fundamental assumption that a modular solution is indeed more evolvable can be questioned on both empirical and conceptual grounds (e.g., Anderson & Finlay Reference Anderson and Finlay2014; Bolhuis et al. Reference Bolhuis, Brown, Richardson and Laland2011; d'Souza & Karmiloff-Smith Reference d'Souza and Karmiloff-Smith2011; Lefebvre Reference Lefebvre2014). Empirical evidence for a direct mapping of specialized adaptive behavioral functions to specific modular neural units is actually rare, even for neural systems as simple as those of invertebrates. Novel adaptive functions seem mostly to be achieved via massive re-use of neural tissue rather than via the addition of encapsulated neuronal pools. Conceptually, the evolvability argument seems largely incompatible with what is known about short-term neuromodulation, brain plasticity over the life span, response to damage, and ontogenetic principles of brain development. The a priori evolvability argument, therefore, does not lead to an unambiguous conclusion as to the superiority of domain-specific over domain-general organization.
Second, the other advantage of modularity – fast, effortless, and ultimately efficient solving of evolutionarily recurrent fitness problems – may hold only for particular notions of modularity, such as Fodorian modules (Fodor Reference Fodor1983). These are thought to be domain-specific functional units that process distinctive input stimuli using distinctive mechanisms. In particular, a module is thought to exclusively process information from a specific domain and to produce a correspondingly specific output in the form of representations and/or a behavioral response. Fodor listed criteria that must – at least to “some interesting extent” (Fodor Reference Fodor1983, p. 37) – be fulfilled by a functional unit to qualify as modular. These criteria include domain specificity, mandatory processing, high speed, production of shallow outputs (i.e., not requiring extensive processing), limited accessibility, a characteristic ontogeny (reliable emergence without explicit learning), a fixed neural architecture, and informational encapsulation (meaning it is not affected by other cognitive processes, a criterion thought to be particularly important). Paradigmatic examples of Fodorian modules are optical illusions. Accordingly, the presence of modules involving the processing of sensory information is widely accepted, and that their speed and efficiency are beneficial is obvious. However, a modular organization has also been proposed for more higher-level cognitive processes including ones related to folk psychology (e.g., processing of faces and facial expressions, theory of mind, cheater detection), folk biology (e.g., animate-inanimate distinction, flora-fauna), or folk physics (e.g., movement trajectories, gravity biases, representation of space, solidity, and causality; summarized in Geary Reference Geary2005). Indeed, massive modularity accounts hold that the mind is exclusively made up of modules (Barrett Reference Barrett, Zeigler-Hill, Welling and Schackelford2015; Carruthers Reference Carruthers and Stainton2005; Sperber Reference Sperber and Dupoux2001).
Massive modularity would appear to be irreconcilable with general intelligence (and therefore with the ability to solve evolutionarily novel problems), but much of the longstanding controversy about the massive modularity hypothesis of the human mind comes down to the use of different notions of modularity (see also Barrett & Kurzban Reference Barrett and Kurzban2006). Indeed, a variety of highly divergent notions have developed (Barrett Reference Barrett, Zeigler-Hill, Welling and Schackelford2015; Barrett & Kurzban Reference Barrett and Kurzban2012; Chiappe & Gardner Reference Chiappe and Gardner2012; Coltheart Reference Coltheart2011; Grossi Reference Grossi2014; Mahon & Cantlon Reference Mahon and Cantlon2011), and many of these are much broader than the Fodorian one (e.g., Sternberg Reference Sternberg2011). Because they also encompass the possibility of overarching, central control processes (Carruthers Reference Carruthers2011), they are entirely compatible with the coexistence of domain-general processes and general intelligence (Barrett Reference Barrett, Zeigler-Hill, Welling and Schackelford2015; Carruthers Reference Carruthers2011). In fact, Carruthers (Reference Carruthers2011) argued that most modules are specialized learning systems. Such broad notions of modularity, however, arguably no longer support the original idea of automatically providing fast and frugal solutions to recurrent fitness problems.
Unlike many proponents of massive modularity in humans, comparative behavioral biologists and comparative psychologists typically refer to notions of modularity that hew closely to the classical Fodorian modules, that is, dedicated, inflexible cognitive adaptations that have evolved in response to specific recurrent fitness-relevant problems (e.g., Fernandes et al. Reference Fernandes, Woodley and te Nijenhuis2014; Shettleworth Reference Shettleworth, Vonk and Shackelford2012a; Reference Shettleworth2012b). Functional specialization here is mostly used in the biological, ultimate sense – that is, referring to the specific adaptive pressures that gave rise to the evolution of specific dedicated modules. This perspective is grounded in research traditions such as neuroecology (Sherry Reference Sherry2006) that have provided empirical evidence for the occurrence among animals of dedicated cognitive adaptations, such as spatio-temporal memory abilities in food-caching species, birds in particular (Brodin Reference Brodin2010; Pravosudov & Roth Reference Pravosudov and Roth2013). These cognitive adaptations typically do not generalize to problems for which they did not evolve.
A mind composed of such dedicated adaptations represents a plausible null model, and indeed a plausible ancestral state of vertebrate cognition. Dedicated adaptations and general intelligence can obviously coexist (e.g., Cosmides et al. Reference Cosmides, Barrett and Tooby2010; Geary Reference Geary2005) – for instance, when the output of modules serve as inputs for intelligent reasoning, which may be responsible for the fact that in humans general intelligence predicts reasoning ability even in evolutionarily familiar contexts (Kaufman et al. Reference Kaufman, DeYoung, Reis and Gray2011). The key questions with respect to the evolution of general intelligence, therefore, are how central, domain-general processes could evolve on top of domain-specific adaptations, whether and to what extent they also exist in nonhuman animals, and what adaptive benefits drove their evolution.
1.2.2. Adaptive canalization beyond modularity
Strictly domain-general approaches that construe the mind as a general-purpose computer face several well-known problems (Cosmides & Tooby Reference Cosmides, Tooby, Hirschfeld and Gelman1994; Cosmides et al. Reference Cosmides, Barrett and Tooby2010; Frankenhuis & Ploeger Reference Frankenhuis and Ploeger2007; Heyes Reference Heyes2003; Kolodny et al. Reference Kolodny, Edelman and Lotem2015; see also Table 1). First, an agent has to efficiently identify relevant information and filter out irrelevant information in the process of problem solving, a challenge known as the frame problem. Second, once the relevant information has been identified, the agent has to decide what to do with it. To do so, she has to solve the problem of how to pick and combine correct, adaptive behavioral options or cognitive processes out of an exponentially growing number of possibilities (the problem of the combinatorial explosion) or to learn important associations and skills in a limited period of time despite dealing with relevant stimuli that occur at a low rate (the poverty of the stimulus problem). Third, correct responses have to be made quickly and efficiently (the urgency problem). And fourth, while doing so, the agent has to find general, rather than only locally successful, solutions (the functionality problem). It is thus beyond doubt that some canalization of cognitive processes is necessary.
Table 1. Overview of some specific problems that a domain-general cognitive apparatus has to overcome in order to produce ultimately adaptive behavior, as well as potential solutions – that is, adaptive canalization mechanisms. Note that these solutions may be very general themselves, such as a preference for social learning. See text for references.

1 That is, evolved cognitive domains that are fleshed out with experience; for example, Gelman (Reference Gelman1990).
Evolved Fodorian modules (referred to as “cognitive adaptations” by behavioral biologists and neuroecologists) are clearly one way of solving the problems highlighted previously, in particular when they define the entire sequence from the acquisition of information to the adaptive behavioral response. However, they are not necessarily the only possible way, and natural selection may also overcome these problems in a different way that would allow domain-general abilities to evolve. A straightforward solution to this problem would be that domain-general abilities coevolve together with adaptive canalizing mechanisms that guide how general abilities are applied. Canalizing mechanisms can have a phylogenetic origin, such as a genetically predetermined preference for a certain category of stimuli: for example, the preference for faces in human infants (Shah et al. Reference Shah, Happé, Sowden, Cook and Bird2015). Alternatively, they can have an ontogenetic origin, such as the propensity of chimpanzees from tool-using communities to automatically perceive a stick as a potential tool, compared to genetically indistinguishable chimpanzees from non-tool-using communities who do not recognize this affordance (e.g., Gruber et al. Reference Gruber, Muller, Reynolds, Wrangham and Zuberbühler2011).
Table 1 summarizes the phylogenetic and ontogenetic canalizing mechanisms that ensure that domain-general cognition produces adaptive behavior despite the problems highlighted previously. Unlike Fodorian modules, these mechanisms do not define the entire sequence from signal detection to behavioral output, but may be deployed at different stages during information processing. We will now examine the evidence for such domain-general canalization processes.
The first problem an individual faces is what to attend to in the continuous stream of stimuli coming in from different sensory modalities. This can be solved by innate dispositions or data acquisition mechanisms (also referred to as phylogenetic inflection: Heyes Reference Heyes2003). Importantly here, innateness is not equivalent to inflexibility because innate dispositions to pay attention to one stimulus over another can be conditional. For instance, an animal foraging for berries may have an attentional bias to perceive small red entities, but the same animal when exposed to a raptor will be biased to perceive only potential hideouts. Alternatively, animals can learn ontogenetically which targets are particularly worth attending to (ontogenetic inflection). Here, social guidance of attention may play a particularly important role. Ontogenetic inflection automatically arises whenever immatures follow the mother and later other conspecifics, and is even more powerful in species that follow gaze (Shepherd Reference Shepherd2010). In many species, including humans, immatures are particularly attracted to everything conspecifics are interacting with, and immatures of some species, such as aye-ayes (Krakauer Reference Krakauer2005), marmoset monkeys (Voelkl et al. Reference Voelkl, Schrauf and Huber2006), or orangutans (Forss et al. Reference Forss, Schuppli, Haiden, Zweifel and van Schaik2015) are highly neophobic toward stimuli they have not witnessed their mother or other familiar conspecifics interact with. Natural selection can, therefore, favor the disposition to preferentially use social information to decide which stimuli to attend to, and thus leave the specific target of attention largely unspecified.
In a second step, the individual has to “decide” what to do with the stimuli that have captured its attention, because input mechanisms filter incoming stimuli but do not produce behavior. Subsequent processes are therefore required to determine what to do with these stimuli without being stymied by the problems of poverty of stimulus and the combinatorial explosion. First, in the case of phylogenetic inflection, coevolution of input mechanisms and response tendencies is frequent (Lotem & Halpern Reference Lotem and Halpern2012), as when a moving stimulus in the sky automatically triggers a flight reaction, but also when individuals are more likely to associate a snake (but not a flower) with fear (Cook & Mineka Reference Cook and Mineka1989), or a taste (but not an auditory stimulus) with subsequent nausea (known as biologically prepared learning or the Garcia effect: Garcia & Koelling Reference Garcia and Koelling1966). Second, in the case of ontogenetic inflection, social learning can also affect how the individual processes a stimulus that has come to its attention. Third, the stimuli that have attracted an individual's attention may be integrated with innate bodies of knowledge, so-called core knowledge (Gelman Reference Gelman1990; Spelke & Kinzler Reference Spelke and Kinzler2007) or psychological primitives (Samuels Reference Samuels2004), and so give rise to more elaborate skills and conceptual systems (Carey Reference Carey2009).
A third problem for the individual is that decisions often have to be made under time pressure (the urgency problem). Evolved modules, heuristics, or direct and reflexive triggering of responses are particularly good at providing fast responses because they bypass central processes. But quick and efficient responses can also be achieved in evolutionarily novel contexts, such as solving algebraic equations or playing chess, if a learned heuristic approach becomes an automated subroutine and can be applied effortlessly (Bilalić et al. Reference Bilalić, Langner, Ulrich and Grodd2011; Chang Reference Chang2014). Such problem solving has similar surface properties to modular organization sensu Fodor. This fact has sometimes led to conceptual misunderstandings (see also section 1.2.3), and is relevant for approaches that try to identify domain-general processes in nonhuman animals (see also section 2.4.3).
A final potential problem is that developmentally acquired response tendencies may be successful in solving local problems, but nevertheless may not ultimately help an individual survive and reproduce (the functionality problem). Individuals, be they animals or humans, typically do not represent ultimate fitness goals in their everyday behavior. Rather, they pursue a set of innate psychological goals, which on average results in fitness-enhancing behaviors (Tinbergen Reference Tinbergen1963) but may become maladaptive in environments other than the one in which the goals evolved, as shown by our strong preferences for sweet, fatty, and salty foods. However, innate goals may be modified or supplemented by socially acquired end-state preferences. For immatures, who are most strongly affected by the canalization problems listed in Table 1, copying successful adult individuals is widespread and generally results in adaptive behavior because they are copying individuals who have survived until adulthood and managed to reproduce. Socially acquired end-state preferences and goals are particularly widespread in humans, who are highly susceptible to conformity and prestige biases (Dean et al. Reference Dean, Vale, Laland, Flynn and Kendal2014; Richerson et al. Reference Richerson, Baldini, Bell, Demps, Frost, Hillis, Mathew, Newton, Narr, Newson, Ross, Smaldino, Waring and Zefferman2016). Increasing evidence also suggests the existence of such biases in at least some nonhuman primates and birds (Aplin et al. Reference Aplin, Farine, Morand-Ferron, Cockburn, Thornton and Sheldon2015; Kendal et al. Reference Kendal, Hopper, Whiten, Brosnan, Lambeth, Schapiro and Hoppitt2015; Luncz & Boesch Reference Luncz and Boesch2014; van de Waal et al. Reference van de Waal, Borgeaud and Whiten2013).
Despite being incomplete, Table 1 serves to highlight that adaptive canalization of cognition not involving Fodorian modules is possible, indeed potentially quite frequent. It also highlights the prominent reliance on social inputs to overcome the canalization problems inherent to domain-general mechanisms. Social learning is broadly defined in the animal literature – that is, learning influenced by observation of, or interaction with, another animal or its products (Heyes Reference Heyes1994; see also Box Reference Box1984). It is widespread in the animal kingdom, both in vertebrates and invertebrates, and ranges from processes as simple as social facilitation and enhancement learning to observational forms of social learning such as true imitation (e.g., Hoppitt & Laland Reference Hoppitt and Laland2013). Interestingly, it is increasingly assumed that many of the cognitive mechanisms involved in social learning are of a general nature rather than specialized, and are thus not specific to social learning (Behrens et al. Reference Behrens, Hunt, Woolrich and Rushworth2008; Heyes Reference Heyes2012; Reference Heyes2016). Indeed, all forms of social learning also include a major element of individual learning. This is most evident in forms such as stimulus enhancement, where the attention of a naïve individual is drawn to stimuli other individuals are interacting with, which then releases individual exploration, play, and trial-and-error learning with this stimulus. Individual learning and practice, however, are also involved in the acquisition of skills through imitation learning, whereby it is typical that, after observation, a phase of individual practice is required (Galef Reference Galef2015; Jaeggi et al. Reference Jaeggi, Dunkel, Van Noordwijk, Wich, Sura and van Schaik2010; Schuppli et al. Reference Schuppli, Meulman, Forss, van Noordwijk and van Schaik2016). Thus, natural selection for social learning seems to automatically trigger selection on individual learning and general cognitive ability, suggesting that ontogenetic canalization through social learning may have contributed to enabling the evolution of domain-general cognition, an issue to which we return in section 3.3.
1.2.3. Primary and secondary modularization, and implications for general intelligence in nonhuman animals
Evolved Fodorian modules have specific surface properties: they work fast, effortlessly, and automatically, and they do not require significant amounts of executive control and working memory. Nevertheless, identifying modules in animals based on these properties is problematic because skills, capabilities, and solutions to problems that are acquired through effortful problem solving and learning based on general cognitive processes may become automatized over time, a process we refer to as secondary modularization. After such secondary modularization, or automatization, these skills have many of the surface properties in common with primary, evolved Fodorian modules. Note that this distinction in primary and secondary modularization is analogous to the distinction in primary and secondary cognitive abilities by Geary (Reference Geary1995), but whereas the latter has been developed specifically for humans, the former is thought to apply to a broad array of animal species.
Despite the similarities in surface properties, primary and secondary modules differ fundamentally with regard to their origin (see Table 2): Primary modules are evolved adaptations with canalized, buffered development, whereas secondary modules represent ontogenetically acquired skills that were automatized during ontogeny. In fact, secondary modularization is particularly common during the immature period (d'Souza & Karmiloff-Smith Reference d'Souza and Karmiloff-Smith2011). A consequence of the different etiology of primary and secondary modules is that the latter are more variable in their content and distribution across individuals or populations of the same species. Because little is known about the ontogeny of many of the specialized cognitive modules postulated for humans (Geary Reference Geary2005), we should also acknowledge the possibility that some or all of these are secondary rather than primary (Anderson & Finlay Reference Anderson and Finlay2014) or at least subject to experiential influences. For instance, even some prototypical modules such as those involved in face perception depend on experience (Dahl et al. Reference Dahl, Chen and Rasch2014).
Table 2. Primary and secondary modules differ with regard to their etiology and development, which has implications for their content and distribution within a species or population

1 Greenough et al. (Reference Greenough, Black and Wallace1987).
The implication for the question of general intelligence in nonhuman animals is that it is no longer possible to uniquely rely on surface properties such as speed, effort, efficiency, and reliability to infer the presence of evolved domain-specific modules, because secondary modules have similar properties. Instead, a better diagnostic tool for the presence of general cognitive abilities is the presence of variable skill profiles across individuals and genetically similar populations due to secondary modularization (see section 2.4.3).
We have shown that human cognition involves elements of domain-specific and domain-general processes, but that the same can potentially be true for animals as well. Hence, animal minds need not be bundles of specialized cognitive adaptations. Having thus leveled the playing field, we first, in section 2, review recent evidence for whether a positive manifold (g) is present in nonhuman animals at all, and if so, how such a g factor is best explained. In particular, we will focus on the question whether such psychometric intelligence shows any of the features usually referred to as general intelligence. Even if we can be confident that this is the case in humans, whether the same applies to animals must be an empirical question (Galsworthy et al. Reference Galsworthy, Arden, Chabris, Finkel and Reynolds2014), and we highlight different research strategies that may prove to be fruitful in the future. In section 3, we then use this pattern of results to examine the ultimate evolutionary question of why general intelligence evolved, and which selection pressures may have favored it.
2. General intelligence in nonhuman animals?
Unless general intelligence is inextricably linked to language, considerations of evolutionary continuity suggest that nonhuman animals, especially our closest extant relatives, the great apes, may well possess it too, at least to some extent. The presence of evidence for executive functions in animals (Chudasama Reference Chudasama2011) supports this contention, as does the overall flexibility of brains in animals, both during development and as response to experience, including the training of cognitive skills (Johansen-Berg Reference Johansen-Berg2007; Kolb & Gibb Reference Kolb and Gibb2015; Matsunaga et al. Reference Matsunaga, Nambu, Oka, Tanaka, Taoka and Iriki2015; Sale et al. Reference Sale, Berardi and Maffei2014). According to most neurobiologists, such developmental plasticity is incompatible with purely domain-specific descriptions of cognitive abilities (Anderson & Finlay Reference Anderson and Finlay2014; Prinz Reference Prinz and Stainton2006; Quartz Reference Quartz, Scher and Rauscher2003). Nonetheless, evolutionary plausibility does not amount to empirical evidence, to which we turn now.
The question of whether general intelligence is unique to humans has typically been addressed by asking whether we find a positive manifold or psychometric intelligence, by following two complementary approaches: First, within a given species, in analogy to human studies, psychometric test batteries have been applied to many individuals. Second, broad comparative analyses (both experimental and meta-analytical) have been conducted across species to investigate whether species differ from each other in general intelligence, rather than in specific cognitive adaptations. In addition, some studies have simultaneously analyzed intraspecific and interspecific variation in cognitive performance. In the following subsections, we first give an overview of these studies. We refer to general factors extracted from intraspecific studies as g, and to those extracted from interspecific studies as G. We then critically assess to what extent alternative explanations may account for the findings, and formulate criteria for future studies that should help pin down to what extent a statistically derived g/G factor reflects general intelligence as broadly defined.
2.1. Intraspecific studies of psychometric intelligence: g
Interest in the question of whether general intelligence may be found in nonhuman animals briefly spiked in the 1930s and 1940s (Locurto & Scanlon Reference Locurto and Scanlon1998), after Spearman's g factor (Spearman Reference Spearman1927) had become widely known. These studies reported positive correlations across various types of tasks, but predominantly concerned mazes and mostly in non-primate species such as mice, rats, and chicks (Locurto Reference Locurto, Tomic and Kigman1997). Because the model of a hierarchical structure of human cognition and the methodological tools to detect it became widely available only in the late 1940s, the design of these early studies was often not suitable to detect g or any factor structure.
For the next half century, the question of animal general intelligence was largely ignored, with interest resurging only after the late 1990s, mainly focusing on mice and primates. Table 3 provides an overview of these studies that have assessed and analyzed correlated performance across three or more cognitive tasks within subjects of the same species, for rodents, primates, and other species (see also Bouchard Reference Bouchard2014; Chabris Reference Chabris and Roberts2007; Galsworthy et al. Reference Galsworthy, Arden, Chabris, Finkel and Reynolds2014; Matzel et al. Reference Matzel, Sauce-Silva and Wass2013).
Table 3. Intraspecific studies that have assessed and analyzed correlated performance across at least three cognitive tasks within subjects of the same species, for rodents, primates, and other species
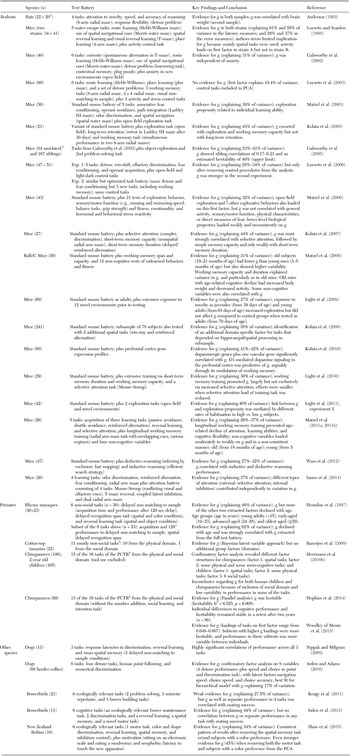
1 In the second sample, variation in brain size was induced by prenatal exposure to methyl-azoxymethanol, which induces microcephaly
2 Data for 40 subjects were taken from Galsworthy et al. (Reference Galsworthy, Paya-Cano, Monleo and Plomin2002)
3 Inhibition (occluded reach, A-not-B error, reversal learning), perceptual speed (targeted reach), exploration, numerical discrimination, acoustic discrimination, inspection time (objects and social), memory (hidden reward retrieval), food extraction puzzle
4 The PCTB (Primate Cognition Test Battery, Herrmann et al. Reference Herrmann, Call, Hernández-Lloreda, Hare and Tomasello2007) consists of 16 tasks from the physical domain (space: spatial memory, object permanence, rotation, transposition; quantities: relative numbers, addition numbers; causality: noise, shape, tool use, tool properties) and the social domain (social learning; communication: comprehension, pointing cups, attentional state; theory of mind: gaze following, intentions)
In rodents, robust evidence for g is available from a range of studies, mostly on mice, from test batteries including as many as eight different tasks and various regimes of principal component analysis (e.g., reviewed in Bouchard Reference Bouchard2014; Galsworthy et al. Reference Galsworthy, Arden, Chabris, Finkel and Reynolds2014; Matzel et al. Reference Matzel, Wass and Kolata2011b; but see Locurto et al. Reference Locurto, Fortin and Sullivan2003; Reference Locurto, Benoit, Crowley and Miele2006). In general, g explains between 30% and 40% of variation in cognitive performance, and in rats, it is positively correlated with brain size (Anderson Reference Anderson1993). Moreover, heritability estimates of up to 40% have been reported (Galsworthy et al. Reference Galsworthy, Paya-Cano, Liu, Monleón, Gregoryan, Fernandes, Schalkwyk and Plomin2005). Test batteries often include typical, rather basic learning tasks, such as associative fear conditioning, operant avoidance, path integration, odor discrimination, and spatial navigation. Nevertheless, as in humans, the derived g factors have been shown to covary with executive functions, such as selective attention (Kolata et al. Reference Kolata, Light, Grossman, Hale and Matzel2007; Matzel et al. Reference Matzel, Light, Wass, Colas-Zelin, Denman-Brice, Waddel and Kolata2011a) and working memory (particularly working memory capacity: Kolata et al. Reference Kolata, Light, Townsend, Hale, Grossman and Matzel2005; Matzel et al. Reference Matzel, Grossman, Light, Townsend and Kolata2008; Sauce et al. Reference Sauce, Wass, Smith, Kwan and Matzel2014) as well as performance in tests of reasoning. For instance, g derived from a standard mouse test battery predicted performance in inductive (finding efficient search strategies in a complex maze) and deductive reasoning (inferring the meaning of a novel item by exclusion, i.e., “fast mapping”: Wass et al. Reference Wass, Denman-Brice, Light, Kolata, Smith and Matzel2012). Working memory training did increase g (Light et al. Reference Light, Kolata, Wass, Denman-Brice, Zagalsky and Matzel2010; Matzel et al. Reference Matzel, Light, Wass, Colas-Zelin, Denman-Brice, Waddel and Kolata2011a), mainly through its positive effect on selective attention (Light et al. Reference Light, Kolata, Wass, Denman-Brice, Zagalsky and Matzel2010; see also Sauce et al. Reference Sauce, Wass, Smith, Kwan and Matzel2014). Importantly, g did not simply capture fear and stress reactivity (Matzel et al. Reference Matzel, Townsend, Grossman, Han, Hale, Zappulla, Light and Kolata2006), anxiety (Galsworthy et al. Reference Galsworthy, Paya-Cano, Monleo and Plomin2002), or other lower-level biological processes such as sensory or motor abilities (Matzel et al. Reference Matzel, Townsend, Grossman, Han, Hale, Zappulla, Light and Kolata2006). In sum, for rodents, the finding of a first component in cognitive test batteries that corresponds to g is robust, and several implications of its presence have been confirmed.
In nonhuman primates, only a handful of studies on the consistency of individual-level differences in cognitive tasks are available. Herndon et al. (Reference Herndon, Moss, Rosene and Killiany1997) were interested in classifying patterns of age-related cognitive decline in adult rhesus macaques, an Old World monkey species. They found a first PCA factor that explained 48% of the variance in cognitive performance and on which all six tasks loaded positively. This factor, based on 30 subjects, was highly correlated with a factor derived from a subset of only three of these tasks (all of which, again, loaded positively on it) in an overlapping sample of 53 subjects. Furthermore, this putative g declined linearly with increasing age of the monkeys.
Banerjee et al. (Reference Banerjee, Chabris, Johnson, Lee, Tsao and Hauser2009) found evidence for g in a New World monkey species, the cotton-top tamarin (Saguinus oedipus). They tested 22 subjects with a battery consisting of 11 tasks that assess a range of cognitive abilities such as inhibitory control, quantity discrimination, and memory. Owing to the relatively high number of missing individual test scores, they used Bayesian analysis and found a g factor but no group factors that would have corresponded to more specialized cognitive domains (although the a priori classification of domains is inevitably tenuous without extensive validation; see also section 2.4).
Among great apes, evidence for g is more mixed. Herrmann et al. (Reference Herrmann, Call, Hernández-Lloreda, Hare and Tomasello2007) developed the Primate Cognitive Test Battery (PCTB) consisting of 16 tasks from the physical and the social domain, a priori placed into six categories (i.e., space, quantities, causality, social learning, communication, and theory of mind) and applied it to 106 chimpanzees, 32 orangutans, and 105 two-year old human children. Chimpanzees and human children performed equally well (and better than orangutans) in tasks from the physical domain, but the children outperformed both ape species in the social domain. These results were not consistent with g in any of the species, including human children. To explicitly address the structure of individual differences, Herrmann et al. (Reference Herrmann, Hernandez-Lloreda, Call, Hare and Tomasello2010b) re-analyzed the data from the chimpanzees and children in 15 of the 16 PCTB tasks (tool use was not included) using a confirmatory PCA (see sect. 2.4.1 for further discussion). They found a different structure of cognitive abilities for chimpanzees (2 factors) and children (3 factors). In addition to a “Spatial” factor in both species, only one additional “Physical-Social” factor emerged in chimpanzees, whereas two additional factors, a “Physical” and a “Social” one, emerged in children. The authors thus did not find evidence for g in either chimpanzees or humans. However, human test batteries typically do not include subtests assessing social cognition. In fact, the relationship between general cognitive processes and socio-cognitive processes is currently poorly understood in humans (Korman et al. Reference Korman, Voiklis and Malle2015). This problem, however, does not explain the presence of two other factors rather than a single g in human children.
More recently, Hopkins et al. (Reference Hopkins, Russel and Schaeffer2014) tested 99 chimpanzees with a reduced and slightly modified version of the PCTB consisting of 13 of the 16 tasks (including tool use but excluding one of two quantity tasks, the social learning task, and one theory of mind task). They report a g factor derived from a non-rotated PCA and used quantitative genetic analyses to estimate its heritability (h2), which was found to be 53% and highly significant. Furthermore, the results remained stable when 86 of the 99 chimpanzees were retested with the same test battery after two years, and were confirmed with parallel analysis. Woodley of Menie et al. (Reference Woodley of Menie, Fernandes and Hopkins2015) further analyzed the data set and concluded that the more g-loaded a task is, the higher its heritability and phenotypical variability, as also found in humans. The more g-loaded tasks also had higher coefficients of additive genetic variance, suggesting that cognitive abilities with higher g loadings have been subject to stronger recent selection.
Taken together, then, the psychometric studies in specific rodent and primate species lend increasing support to the notion that the positive manifold is not unique to humans but also present in nonhuman animals. Studies on other lineages such as dogs (Arden & Adams Reference Arden and Adams2016; Nippak & Milgram Reference Nippak and Milgram2005) and birds (Keagy et al. Reference Keagy, Savard and Borgia2011; Isden et al. Reference Isden, Panayi, Dingle and Madden2013; Shaw et al. Reference Shaw, Boogert, Clayton and Burns2015) are also beginning to provide evidence. However, a serious limitation of psychometric studies in nonhuman animals is that they tend to lack power with respect to sample size, the diversity of cognitive tasks, or both. We discuss these limitations in section 2.4. Fortunately, there is a complementary approach, which examines interspecific variation and is particularly powerful to reveal evolutionary trends.
2.2. Interspecific studies of psychometric intelligence: G
In comparative approaches, the fundamental question is whether some species systematically outperform others across an array of distinct cognitive tasks, consistent with the notion of psychometric and perhaps general intelligence, or whether species differences are instead characterized by independent variation in performance across tasks and domains, consistent with higher domain specificity. Comparative studies thus investigate whether what evolves are specialized skills or rather general intelligence. This approach (Table 4) has predominantly been applied to primates but also to birds and involves both meta-analyses and targeted experimental comparisons.
Table 4. Interspecific, comparative studies that have assessed correlated cognitive performance across species
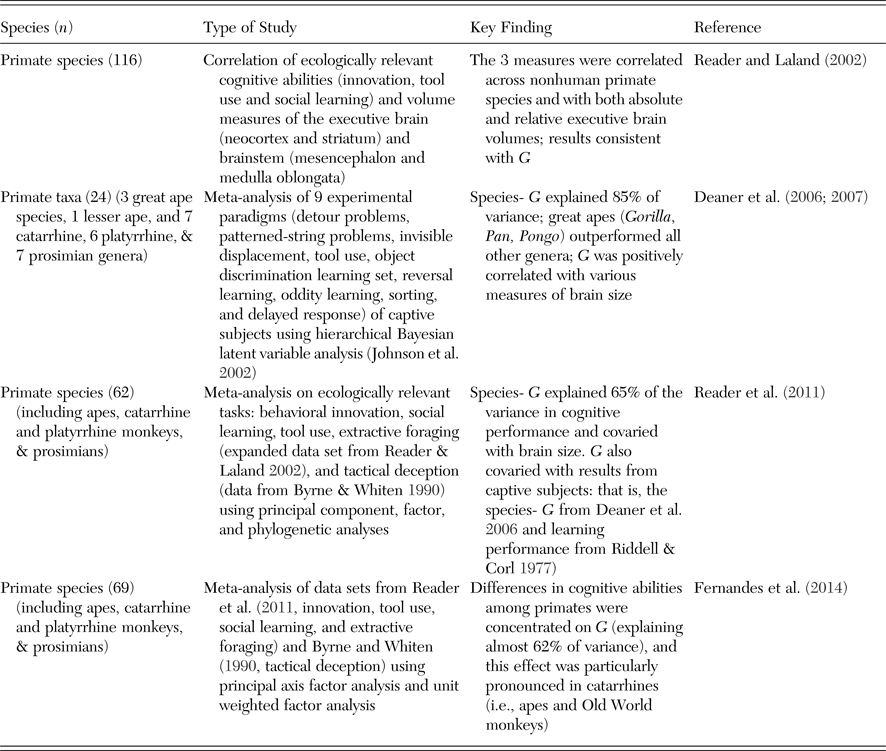
For primates, Deaner et al. (Reference Deaner, van Schaik and Johnson2006) conducted a meta-analytical study that compared the performance of 24 primate taxa tested with nine experimental physical-cognition paradigms using Bayesian hierarchical modeling (Johnson et al. Reference Johnson, Deaner and van Schaik2002). They found strong evidence for G, which correctly predicted 85% of the species rankings (but note that caution is needed when comparing the proportion of explained variance between standard PCA and Bayesian analyses). Moreover, in a follow-up study, G was strongly correlated with brain size (Deaner et al. Reference Deaner, Isler, Burkart and van Schaik2007).
In another set of studies, Reader and Laland (Reference Reader and Laland2002) collected data from the literature on the incidence of innovation, social learning, and tool use in 116 species of nonhuman primates, both in captivity and in the wild, and found that across species, all three measures were correlated with each other (r 2 values around 0.4), as well as with brain size. In a follow-up study involving 62 primate species (Reader et al. Reference Reader, Hager and Laland2011), they found evidence for general intelligence on the interspecific level (G) in principal component and factor analyses explaining 65% of the variance, based on measures of innovation, social learning, and tool use, as well as extractive foraging and tactical deception. As in Reader and Laland's (Reference Reader and Laland2002) earlier study, G was correlated with brain size, but also with a combined measure of performance across several learning tasks, with learning set performance (both taken from Riddell & Corl Reference Riddell and Corl1977), and the G measure of Deaner et al. (Reference Deaner, van Schaik and Johnson2006).
More recently, Fernandes et al. (Reference Fernandes, Woodley and te Nijenhuis2014) compiled published data from five cognitive domains (innovation, tool use, social learning, extractive foraging, and tactical deception) across 62 primate species (data sets from Reader & Laland Reference Reader and Laland2002; and Byrne & Whiten Reference Byrne and Whiten1990). Fernandes et al. found that a single factor G explained almost 62% of the total variance. Furthermore, they reported that cognitive abilities that load more strongly on G show bigger interspecific variation, weaker phylogenetic signals, and faster rates of evolution. These results are consistent with the idea that G has been subjected to selection pressure stronger than narrow, more domain-specific abilities and that G is thus the principal locus of selection in the evolution of primate intelligence (but see also section 2.5).
The only other taxon to which comparative approaches have been applied are birds. As in primates, significant positive correlations across species were found between innovation rates, tool use, and learning performance. These studies also found positive correlations between innovation rates and brain size as well as colonization success (Ducatez et al. Reference Ducatez, Clavel and Lefebvre2015; Lefebvre Reference Lefebvre2013; Lefebvre et al. Reference Lefebvre, Reader and Sol2004; Sol et al. Reference Sol, Duncan, Blackburn, Cassey and Lefebvre2005).
2.3. Mixed studies combining intraspecific and interspecific variation
Some studies have pursued a mixed approach by applying test batteries to multiple individuals from several species (see Table 5). For instance, Herrmann and Call (Reference Herrmann and Call2012) analyzed data of 23 individuals from all four nonhuman great ape species, which were studied in a range of tasks from the physical domain, and found no support for the existence of g. Nevertheless, some subjects performed particularly well (or poorly) across tasks, both in the sample of 23 great apes and in the 106 chimpanzees mentioned previously (Herrmann et al. Reference Herrmann, Hernandez-Lloreda, Call, Hare and Tomasello2010b), indicating that there was some consistency in individual performance.
Table 5. Mixed studies that have simultaneously analyzed correlated performance within and between species

In another mixed study, Amici et al. (Reference Amici, Barney, Johnson, Call and Aureli2012) found no evidence for G or g when re-analyzing data from seven primate species (all four great ape species, long-tailed macaques, spider monkeys, and capuchin monkeys, totaling 99 individuals) from 17 cognitive tasks. In the Bayesian approach used to analyze the data (see also Barney et al. Reference Barney, Amici, Aureli, Call and Johnson2015), the 17 tasks were a priori attributed to the domains of inhibition, memory, transposition, and support, similar to Herrmann et al. (Reference Herrmann, Hernandez-Lloreda, Call, Hare and Tomasello2010b).
In contrast to intraspecific and interspecific studies, mixed studies thus provide less support for psychometric intelligence. Here, we offer a tentative suggestion to explain this absence of evidence for psychometric intelligence in mixed studies that will need to be examined in more detail in future work. First, despite including a large number of individuals overall, the effective sample size to identify g remains the number of individuals within each species, and to identify G is the number of species. This may strongly influence the outcome because in mixed studies the detection of G is not based on average species-specific performance as is done in interspecific studies, but is instead based on individual values, which are more susceptible to noise. A recent memory task illustrates the superiority of species averages in estimating abilities at the species level. In this study, both marmoset and squirrel monkeys as a group provided results fitting the Ebbinghaus forgetting curve, but at the individual level, several individuals did not, indicating that the performance of these individuals was strongly affected by noise (Schubiger et al. Reference Schubiger, Kissling and Burkart2016). Such noise may overshadow G, especially in species that are very close in G.
A second issue is that in a sample of species with similar G (e.g., according to Deaner et al. Reference Deaner, van Schaik and Johnson2006), and thus both the great ape study by Herrmann and Call (Reference Herrmann and Call2012) and the study by Amici et al. (Reference Amici, Barney, Johnson, Call and Aureli2012), species-specific predispositions linked to domain-specific adaptations may mask a G effect. Thus, chimpanzees and orangutans are more extraction-oriented than bonobos or gorillas (van Schaik Reference van Schaik2016), as expressed, for instance, in species differences in tendencies to handle objects (Koops et al. Reference Koops, Furuichi and Hashimoto2015), or to solve social problems (Herrmann et al. Reference Herrmann, Hare, Call and Tomasello2010a). Such variation is bound to produce species differences in mean performance on some but not on other tasks, reducing the correlation across tasks in the overall data set. Intraspecific comparisons obviously are not affected by this problem, whereas the effect on interspecific comparisons is reduced the broader the comparison in terms of G are, because major interspecific differences in G lessen the effects of species differences in domain-specific predispositions.
More generally, we can ask, if in a given lineage, a robust G is found, whether this implies that all species in the comparison must have g, and vice versa. Several combinations of evidence for g and G are possible, in particular in mixed studies, as summarized in Table 6, and we discuss likely explanations for these combinations.
Table 6. Summary of the potential combinations of evidence for g and G, and under what conditions apparently conflicting findings can be reconciled

The interpretation is straightforward whenever evidence for g and G point in the same direction (see entries I and IV in Table 6), and where it is positive for both, can be externally validated separately at both the level of g and G (see also section 2.5). One potentially conflicting constellation is when positive evidence for g but no evidence for G is available (as for entry II in Table 6). Such a result can arise if g is present in only a few of the species involved in the comparison, which might occur when distantly related lineages are compared. The other conflicting constellation (entry III in Table 6) is that comparative studies provide evidence for G, but there is no evidence for g within the species involved in the comparison. This was the case in some primate studies. In principle, it is possible that we are dealing with cumulative modularity and that by chance the distribution of modules across the species included in the sample is hierarchically nested. In this scenario, no correlation between G and EFs or, arguably, brain size is expected, which is inconsistent with current findings. The most likely cause of constellation III, therefore, is lack of power of animal studies to reliably detect the absence of g, due to the small sample sizes and difficulties to construct a suitable test battery, which make animal psychometric g studies prone to Type II errors (see also section 2.4.1).
Taken together, there is increasing evidence for g in nonhuman animals, particularly in mice and primates, for which positive evidence is available for New World monkeys, Old World monkeys, and chimpanzees (but see Herrmann et al. Reference Herrmann, Hernandez-Lloreda, Call, Hare and Tomasello2010b). At the interspecific level based on comparative analyses across species, studies of primates and birds provide a robust pattern consistent with G. Finally, mixed studies in primates that simultaneously analyze within- and between-species variation yield a more ambiguous pattern.
2.4. Facts or artifacts?
A legitimate concern is whether a presumptive g/G factor can arise as an artifact, and a legitimate question is to what, exactly, it corresponds. We now review why statistical or methodological artifacts may produce false positives, whereas secondary modularization may lead to false negatives, and formulate criteria for future directions that may be used to evaluate whether g/G corresponds to general intelligence broadly defined.
2.4.1. Statistical issues
The use of PCAs or related procedures involves a suite of decisions, including whether exploratory or confirmatory analyses are applied, whether non-rotated or rotated factors are considered, and whether oblique or orthogonal rotations are used. A detailed discussion of factor-analytical procedures is far beyond the scope of this review, and we refer readers to the specialized literature (e.g., Barney et al. Reference Barney, Amici, Aureli, Call and Johnson2015; Garson Reference Garson2013; Stevens Reference Stevens2012). However, because these decisions may critically affect the conclusions of animal studies, we must highlight some issues that appear relevant to the empirical results summarized previously.
First, the use of confirmatory analyses requires an a priori decision of what a domain is, and which tasks are associated with the respective domains (this also applies to Bayesian approaches that likewise categorize tasks a priori to hypothesized domains: Amici et al. Reference Amici, Barney, Johnson, Call and Aureli2012; Barney et al. Reference Barney, Amici, Aureli, Call and Johnson2015). The identification of domains of animal cognition, however, is not straightforward. For instance, some classify spatial reversal learning tasks as spatial cognition (e.g., Locurto & Scanlon Reference Locurto and Scanlon1998) whereas others stress their inhibition component (Tapp et al. Reference Tapp, Siwak, Estrada, Head, Muggenburg, Cotman and Milgram2003). In reality, of course, subjects may recruit several specific abilities to solve a particular task, and in fact different subjects may even recruit a different mix. Accordingly, Hopkins et al. (Reference Hopkins, Russel and Schaeffer2014) found that their exploratory PCA findings were not entirely consistent with the a priori structure of the PCTB originally proposed by Herrmann et al. (Reference Herrmann, Call, Hernández-Lloreda, Hare and Tomasello2007; Reference Herrmann, Hernandez-Lloreda, Call, Hare and Tomasello2010b). An a priori allocation of tasks to domains is thus not straightforward; in fact, the structure of a species' cognition is an empirical question (see also sect. 1.1.1 for corresponding efforts in human intelligence research). Accordingly, the use of confirmatory techniques may lead to diverging results compared to analytical approaches that are a priori agnostic with regard to factor structure.
Second, studies vary with regard to whether they present rotated or non-rotated solutions. Because rotations are designed to make the pattern of factor loadings more pronounced, it is generally recommended to use non-rotated solutions in g studies (Galsworthy et al. Reference Galsworthy, Arden, Chabris, Finkel and Reynolds2014; Jensen & Weng Reference Jensen and Weng1994; Locurto et al. Reference Locurto, Fortin and Sullivan2003; Plomin Reference Plomin2001; Woodley of Menie et al. Reference Woodley of Menie, Fernandes and Hopkins2015). Rotated and non-rotated solutions from the same data set are presented in Hopkins et al. (Reference Hopkins, Russel and Schaeffer2014) and Woodley of Menie et al. (Reference Woodley of Menie, Fernandes and Hopkins2015). Whereas the varimax-rotated solution (Hopkins et al. Reference Hopkins, Russel and Schaeffer2014, Table 1) appeared to suggest that a general factor g was lacking, the results of non-rotated solutions, verified by parallel analysis, demonstrated it was in fact present.
Third, a common intuition in general intelligence studies on animals is to compare the amount of variance explained by a first factor, and to conclude that the higher the amount of explained variance, the stronger the evidence for g. In human studies, the first non-rotated factor typically accounts for about 40% of variance (Plomin Reference Plomin2001), which is in fact similar to what has been reported for mice (see Table 3). However, an exclusive focus on the amount of explained variance is problematic for empirical and conceptual reasons. Empirically, the proportion of explained variance not only depends on the statistical issues discussed previously, but also on the heterogeneity of the subjects in the sample: the more heterogeneous, the higher the proportion of variance explained. In interspecific investigations, for instance, this means that studies that involve species that vary widely in general intelligence and brain size (e.g., 20 species of primates ranging from great apes to prosimians) will find higher proportions of explained variance than studies with a similar sample size, but where the species are all relatively similar (e.g., 20 different species from the same genus or taxonomic family). Conceptually, to the extent that the mind is a combination of both specialized cognitive adaptations and domain-general processes (see also sect. 4.1), very small proportions of explained variance may still be indicative of a real g. Likewise, a first factor with high loadings of some tasks but not others may reflect the absence of general intelligence, but may also reflect the co-occurrence of a general factor and one or several additional, more specialized domains (e.g., for spatial orientation, see Herrmann et al. Reference Herrmann, Call, Hernández-Lloreda, Hare and Tomasello2007; see also first PCA factor in Hopkins et al. Reference Hopkins, Russel and Schaeffer2014).
Last but not least, the most severe statistical restriction of nonhuman psychometric studies is that they critically lack power due to their small sample sizes. Reaching a near-consensus about the structure of human intelligence required meta-analyses involving thousands of subjects (Carroll Reference Carroll1993). Obtaining sample sizes comparable to human studies is unrealistic for most nonhuman animal species, in particular for nonhuman primates (albeit less so for rodents). However, replicating studies is feasible, and if this reveals the same factorial solution in a different set of subjects, and if combining such data sets also increases the fit of the solution, we can be increasingly confident that we are not dealing with statistical artifacts. Unfortunately, although this approach minimizes Type I errors, it suffers from very limited power to avoid Type II errors. In other words, if successful, we can be confident that we have obtained a real result, but if it fails, this may reflect either the absence of a general factor or too low a number of subjects. This shortcoming highlights the need to use external validation for psychometric g/G studies, as discussed below in section 2.5.
2.4.2. Methodological issues
We now turn to the possibility that a g/G factor may arise as a methodological artifact, because the results reflect variation in underlying variables other than general intelligence (see also Macphail's [1982] contextual variables) or because the tasks mainly tap into problems of the same domain.
Some individuals, or some species, may systematically outperform others not because they are more intelligent, but because they are less fearful and better habituated to testing, are more motivated to participate in tasks, have sharper senses, or are simply more active than others (Macphail Reference Macphail1982). Ideally, such confounds are directly quantified, as for instance in Matzel et al. (Reference Matzel, Townsend, Grossman, Han, Hale, Zappulla, Light and Kolata2006). In a sample of 43 mice individuals, they examined to what extent the general learning ability g extracted via PCA from a test battery of six cognitive tasks was correlated with 21 measures of exploratory behavior, sensory/motor function (e.g., running and swimming speed, balance tasks), activity, or fear/stress sensitivity. They found that g was not explained by general activity, sensory/motor function, physical characteristics, or direct measures of fear, but was correlated with several exploratory behaviors. Follow-up studies suggested that this link is caused by variation in habituation rates when exposed to potentially stressful situations (Light et al. Reference Light, Grossman, Kolata, Wass and Matzel2011) rather than by fearfulness influencing both exploration and task performance: Treatment with anxiolytic drugs did increase exploratory behaviors but did not improve performance in individual tasks or g (Grossman et al. Reference Grossman, Hale, Light, Kolata, Townsend, Goldfarb, Kusnecov and Matzel2007). Likewise, temporary environmental enrichment resulting in increased exploration tendency did not improve performance on the cognitive test battery (Light et al. Reference Light, Kolata, Hale, Grossman and Matzel2008). Thus, exploration and g may covary because more exploratory individuals are more likely to encounter contingencies in the environment that promote learning and problem solving, which over time leads to greater experience. The correlation between exploration and g may thus reflect a long-term, cumulative effect of experience on g. This is in line with investment theory (Cattell Reference Cattell1987), and with findings in human infants, where the preference for novelty and habituation is positively correlated with later performance in IQ tests (Teubert et al. Reference Teubert, Vierhaus and Lohaus2011), but also with apes, where individuals more likely to approach novel objects and a human stranger performed better in physical-cognition tasks (Herrmann et al. Reference Herrmann, Call, Hernández-Lloreda, Hare and Tomasello2007). Thus, the rodent studies support the idea that g is not an artifact of confounding factors.
Another non-cognitive factor that may explain variation in cognitive performance is motivation to participate. Female callitrichid monkeys have been reported to outperform males in problem-solving tasks (Brown et al. Reference Brown, Kaplan, Rogers and Vallortigara2010; Yamamoto et al. Reference Yamamoto, Domeniconi and Box2004). However, female callitrichids are typically also more food-motivated, whereas males are more vigilant than females (Koenig Reference Koenig1998). Accordingly, males are less interested in participating in experimental tasks and more easily emotionally aroused during testing. But if male performance is controlled for the presence of attention to the test stimuli, their performance is no longer inferior to that of females (Schubiger et al. Reference Schubiger, Wüstholz, Wunder and Burkart2015). The sexes thus do not differ in cognitive ability, but in their motivation to participate in experimental tasks.
The problem that we may never be sure if species differences in cognitive performance are the result of differences in cognitive ability or differences in contextual variables (Macphail Reference Macphail1982) remains an ongoing challenge for any species comparison. Nevertheless, not all tasks are affected by this problem to the same extent. Reversal learning tasks, for instance, are arguably less affected, because individuals first have to reach a criterion of making an initial discrimination. Differences in sensory-motor abilities and so on may well influence how difficult it is for a species to learn a particular discrimination. However, the crucial test is applied only once a specific criterion has been reached, and at least in marmosets, the time needed to achieve this criterion does not predict performance in the reversal trials (Strasser & Burkart Reference Strasser and Burkart2012). Furthermore, it is reassuring that the strongest association between a specific task and G in Deaner et al. (Reference Deaner, van Schaik and Johnson2006) was the one between reversal learning and G.
A second fundamental methodological issue refers to the task selection and battery development. With respect to task design, it is increasingly recognized that small differences in methodological details can strongly influence task performance, which has to be taken into account when performing species comparisons. For instance, memory performance strongly depends on task format in both marmosets and squirrel monkeys. Tests of memory often rely on a two-option choice task (e.g., Banerjee et al. Reference Banerjee, Chabris, Johnson, Lee, Tsao and Hauser2009), but many individuals are then happy to follow a random choice, which yields a 50% reward rate. When the choice involves many more options, subjects will be more motivated to remember the location of the food items and provide more-accurate estimates of their ability to memorize the location of the food item (Schubiger et al. Reference Schubiger, Kissling and Burkart2016). Regarding battery design, if all tasks in the test battery are drawn from the same domain (i.e., a lower-order group factor), rather than from a variety of domains, the positive correlations will reflect a domain-specific ability rather than a more general underlying cognitive factor (g/G). For instance, a positive manifold across a number of maze tasks is consistent with a spatial factor, but not informative with regard to g. The issue of task selection is thus closely linked to the identification of domains in animal cognition, which in fact is part of the empirical question that needs to be addressed in intelligence research in animals in general, by using batteries as diverse as possible and statistical procedures that are a priori agnostic to the underlying factor structure.
2.4.3. False negatives as a result of secondary modularization
Task selection may also bias the result and potentially produce false negatives if tasks prone to secondary modularization are included. Secondary modularization refers to the process that during ontogeny, individuals may specialize on a specific set of problems in a particular domain (Table 2). Problem solving in this domain becomes automatized and thus acquires many features commonly associated with modules rather than domain-general reasoning, particularly fast and frugal information processing, which is independent of reasoning. Thus, despite the presence of g in a given species, performance among individuals across domains need not be correlated whenever heterogeneous developmental inputs prevail that lead different individuals to specialize in different tasks (see Fig. 1c). This applies in particular to the small samples typical for nonhuman primate studies.
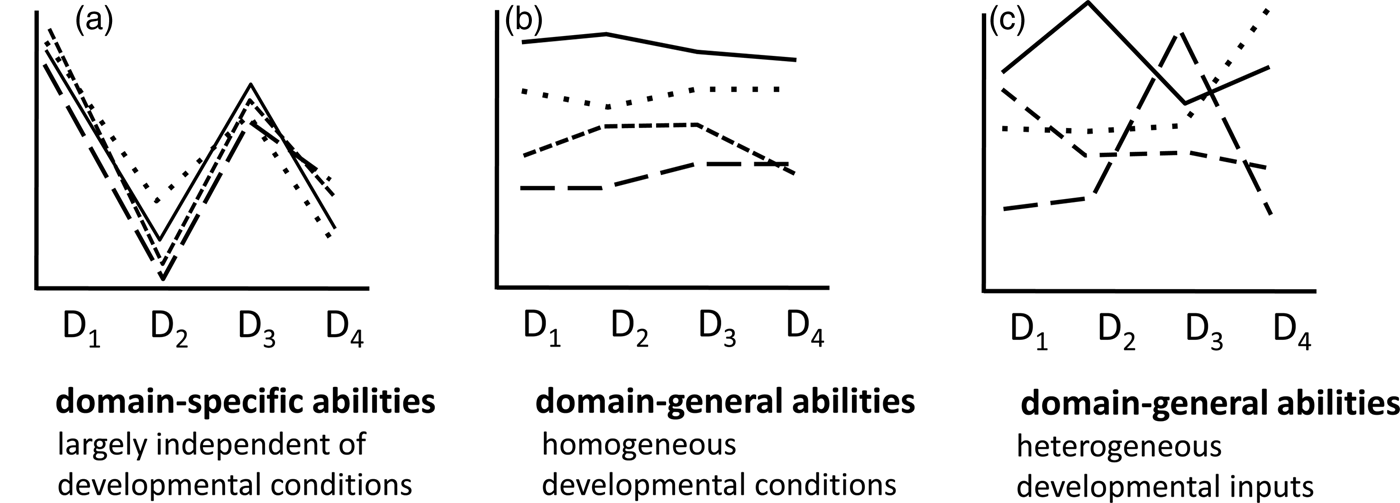
Figure 1. Performance across different cognitive domains (D1-D4). Each line represents the performance of an individual (or, in highly cultural species, of a population). (a) Performance is driven by domain-specific abilities; all individuals perform well in some domains but worse in others, but individual differences across domains are random; (b) performance is driven by domain-general abilities and individuals experience homogeneous developmental conditions, which leads to correlated performance between individuals across domains; (c) performance is driven by domain-general abilities but heterogeneous developmental conditions lead to specialization and secondary modularization of individuals in different domains. As a result, performance between individuals across domains is not correlated despite the presence of g.
Prima facie, this situation (Fig. 1c) may seem incompatible with the positive manifold, which is well documented in humans and perhaps other animals. It is important to keep in mind, however, that psychometric studies in humans are typically performed on subject pools with a rather uniform cultural background (the same is also true for the rodent studies performed on lab animals with virtually identical rearing conditions). If, in human studies, the cultural backgrounds of subjects were more diverse (e.g., ranging from Western-industrialized to a variety of hunter-gatherer societies), and only a small number of subjects tested, such an outcome (as in Fig. 1c) is quite likely (see also Reyes-García et al. Reference Reyes-García, Pyhälä, Díaz-Reviriego, Duda, Fernández-Llamazares, Gallois, Guèze and Napitupulu2016). The notorious difficulty of devising culture-free or at least culture-fair intelligence tests is a direct consequence of this problem (Saklofske et al. Reference Saklofske, van de Vijver, Oakland, Mpofu, Suzuki, Goldstein, Princiotta and Naglieri2014).
The prime example for secondary modularization in nonhuman primates is tool use, which is part of many test batteries typically used with nonhuman primates (e.g., Herrmann et al. Reference Herrmann, Call, Hernández-Lloreda, Hare and Tomasello2007; Reader et al. Reference Reader, Hager and Laland2011). Nonhuman primates vary considerably with regard to tool use, with great apes typically outperforming monkeys. But differences also occur within a species, both between wild and captive animals and among wild populations. Individuals of the same species show much higher propensities to use tools in captivity compared to their counterparts in the wild (Meulman et al. Reference Meulman, Sanz, Visalberghi and van Schaik2012; van Schaik et al. Reference van Schaik, Deaner and Merrill1999). Once proficient, individuals show tool use with high degrees of automatization and efficiency. Wild populations, too, vary significantly with regard to their propensity to use tools and solve tool-related problems (e.g., chimpanzees, Gruber et al. Reference Gruber, Muller, Reynolds, Wrangham and Zuberbühler2011; orangutans, van Schaik et al. Reference van Schaik, Ancrenaz, Borgen, Galdikas, Knott, Singleton, Suzuki, Suci and Merrill2003; or capuchin monkeys, Cardoso Reference Cardoso2013), arguably because they have ontogenetically acquired systematically different affordances of sticks or stones, which are perceived as potential tools in habitual tool users but not in non-tool users.
False negatives resulting from secondary modularization (see Fig. 1c) can be minimized if subjects with comparable rearing conditions are selected for the tests, but also if tasks prone to secondary modularization are excluded from test batteries. Thus, instead of naturalistic tasks that test for ontogenetically constructed skills that are likely to become automatized, such as tool use, or the ability to point and understand pointing, or even to use human language systems (Savage-Rumbaugh et al. Reference Savage-Rumbaugh, Fields, Segerdahl and Rumbaugh2005), it is preferable to include tasks testing for more elementary cognitive abilities, such as reversal learning, mental rotation, or quantity discrimination. Likewise, tests should avoid reliance on experience and knowledge of affordances that may differ among individuals depending on their biographies.
Although it is important to identify tasks and abilities prone to secondary modularization, it is not always easy to identify them. One way to do so is to examine the ontogeny of skills that are suspected to be the result of secondary modularization. Such skills should be acquired by developing immatures after a period of learning (perhaps following alternating series of instances of social learning and practice: Meulman et al. Reference Meulman, Seed and Mann2013; Schuppli et al. Reference Schuppli, Meulman, Forss, van Noordwijk and van Schaik2016), and could also potentially show high variation among adults. The increasing evidence for a major amount of skill learning by immature primates (e.g., Schuppli et al. Reference Schuppli, Meulman, Forss, van Noordwijk and van Schaik2016) and mammals and birds more generally (van Schaik et al. Reference van Schaik, Burkart, Damerius, Forss, Koops, van Noordwijk and Schuppli2016) suggests a greater prevalence of secondary modularization in nonhumans than revealed by the size of cultural repertoires (Whiten & van Schaik Reference Whiten and van Schaik2007). Because in wild populations, social and ecological problems tend to be very uniform for all individuals, variation of skill profiles (see Fig. 1) between populations (that live under similar, wild or captive, conditions), rather than among individuals of the same population, provides an additional heuristic tool to distinguish between genuine primary and secondary modularity. This criterion would work for primate tool use, for instance. Most powerful to disentangle primary from secondary modularity, finally, are cross-fostering experiments. When cross-fostered individuals exhibit species-typical behavior from the foster species rather than their own species, these behaviors clearly cannot result from primary modules. If the same procedure works within a species at the level of populations, it is similarly evidence for secondary, and thus learned, modules.
2.5. Psychometric or general intelligence? Future directions for animal studies
A crucial question that remains unanswered so far is to what extent a reliably identified g/G actually captures general intelligence in a broad sense: that is, reasoning ability and behavioral flexibility (Byrne Reference Byrne, Slater and Halliday1994; Gottfredson Reference Gottfredson1997; Rumbaugh & Washburn Reference Rumbaugh and Washburn2003; Yoerg Reference Yoerg2001; see also sect. 1.1). If it indeed does so, the processes underlying general intelligence (see also sects. 1.1.2 and 1.2.3) in animals should be broadly similar to those found in humans, with the obvious exception of language, and general intelligence should be correlated with independent measures of reasoning ability and behavioral flexibility (see also Bailey et al. Reference Bailey, McDaniel and Thomas2007). If it is not, the statistically derived psychometric factors may reflect cumulative modularity: that is, the coexistence of separate, but coevolved modules.
These two possibilities can be teased apart empirically: If g/G represents intelligence in a broad sense, it must be possible to independently assess its validity, for instance, by showing that g/G is correlated with the domain-general EFs, as has been shown for humans. In principle, an association at a higher hierarchical level (e.g., between-species G) may be absent within the subgroups comprising it (e.g., within-species g), a phenomenon known as Simpson's paradox (Kievit et al. Reference Kievit, Frankenhuis, Waldorp and Borsboom2013). In the present case, we may thus find a correlation between G and EFs but not between g and EFs, which would suggest that g and G were not aspects of the same phenomenon: that is, general intelligence. Thus, to ensure that g and G are related to the same phenomenon, one must validate both of them independently.
Intraspecific studies of primates have so far largely neglected the approach to validate g, but this approach has provided fruitful insights in rodent studies. In rodents, individual levels of g have been shown to correlate with executive functions such as working memory. Matzel et al. (Reference Matzel, Grossman, Light, Townsend and Kolata2008; Reference Matzel, Wass and Kolata2011b) have compared performance on standardized test batteries that reliably quantify g in mice with several measures of working memory, including short-term memory duration (how long can the mouse remember which arms of a maze it has already visited?), simple memory span (how many symbols associated with food can the mouse remember?), and selective attention (an adapted version of the STROOP task, in which the subject has to focus on one dimension of the task while suppressing a second dimension that provides conflicting information). As in human studies, they found that g was most strongly correlated with selective attention, followed by simple memory span and only weakly with short-term memory duration (Matzel et al. Reference Matzel, Grossman, Light, Townsend and Kolata2008; Reference Matzel, Wass and Kolata2011b; Kolata et al. Reference Kolata, Light, Grossman, Hale and Matzel2007). Moreover, they showed that training working memory capacity, but not simple working memory span, promotes selective attention and g (Light et al. Reference Light, Kolata, Wass, Denman-Brice, Zagalsky and Matzel2010). Future validation tests could also examine the correlation between g and conduction speed or the ability to ignore irrelevant, distracting information, which are known correlates of g in humans (Melnick et al. Reference Melnick, Harrison, Park, Bennetto and Tadin2013; Sheppard & Vernon Reference Sheppard and Vernon2008).
The corresponding validation of psychometrically derived g-scores in other species, particularly in nonhuman primates, would be highly desirable. Nonetheless, some evidence consistent with g representing domain-general cognitive mechanisms is already available from nonhuman primates. Within chimpanzees, heritability was strongest for overall cognitive performance g rather than for distinct aptitudes (Hopkins et al. Reference Hopkins, Russel and Schaeffer2014), as expected when the latter are due to secondary modularization rather than reflect specific adaptations. As a result, cognitive abilities that load higher on g in chimpanzees are more heritable, phenotypically variable, and presumably the result of recent natural selection (Woodley of Menie et al. Reference Woodley of Menie, Fernandes and Hopkins2015).
The independent evolution of large numbers of modules instead of general intelligence is particularly difficult to reconcile with interspecific findings of G. If we are dealing with independent modules, each species would be expected to possess a different repertoire of primary modules, according to the specific adaptive problems it faces. Importantly, across species, this should not result in a stable G factor. Studies providing evidence for G, however, suggest that particular species generally perform better or more poorly across all tested domains. This is also consistent with the empirical findings suggesting that differences in cognitive abilities among primates are concentrated on G (Fernandes et al. Reference Fernandes, Woodley and te Nijenhuis2014). Furthermore, because specific skills, even if complex, can be performed with a very modest amount of brain tissue (e.g., Chittka & Niven Reference Chittka and Niven2009; Holekamp et al. Reference Holekamp, Dantzer, Stricker, Yoshida and Benson-Amram2015; Patton & Braithwaite Reference Patton and Braithwaite2015), one would not necessarily expect that G as a reflection of a large number of dedicated modules would correlate with brain size. The well-documented positive correlations between G and brain size thus further suggests that G reflects general intelligence, as does the finding that across primate species G was the principal locus of selection in the macroevolution of intelligence (Fernandes et al. Reference Fernandes, Woodley and te Nijenhuis2014).
Empirical data also support a link between interspecific G and executive functions: Across primate species, brain size is not only correlated with G, but also with self (inhibitory) control (MacLean et al. Reference MacLean, Hare, Nunn, Addessi, Amici, Anderson, Aureli, Baker, Bania, Barnard, Boogert, Brannon, Bray, Bray, Brent, Burkart, Call, Cantlon, Cheke, Clayton, Delgado, DiVinventi, Fujita, Herrmann, Hiramatsu, Jacobs, Jordan, Laude, Leimgruber, Messer, Moura, Ostojic, Picard, Platt, Plotnik, Range, Reader, Reddy, Sandel, Santos, Schumann, Seed, Sewall, Shaw, Slocombe, Yanjie, Takimoto, Tan, Tao, van Schaik, Viranyi, Visalberghi, Wade, Watanabe, Widness, Young, Zentall and Zhao2014). This measure of self-control is directly correlated, in addition (Fig. 2), with G as established by Deaner et al. (Reference Deaner, van Schaik and Johnson2006), which has been derived from a completely independent data set.

Figure 2. Positive correlation between the composite self-control measure of MacLean et al. (2014) and G (Deaner et al. 2006). Adjusted r 2 = 0.66, F (1,9) = 20.75, p = 0.001 based on PGLS (phylogenetic generalized least squares) analyses. The same results are also found when the inhibitory control tasks included in the composite measure are analyzed separately (Cylinder task: adjusted r 2 = 0.58, F (1,11) = 20.75, p = 0.002; A-not-B error: adjusted r 2 = 0.41, F (1,12) = 10.03, p = 0.008).
A particularly powerful, but likewise underexplored, approach to construct validation consists in training individuals to solve a task in one domain and test to what extent they are able to apply their solution in a different domain. Intraspecific variation in this kind of cognitive flexibility (which is consistent with broad notions of general intelligence as applied by comparative scientists) should be correlated with psychometrically derived measures of individual g. Such a pattern would confirm that g is indeed a proxy of animal intelligence broadly defined.
Equally promising is to focus on unusually difficult problems relative to individual performance (i.e., problems that cannot be solved in a routine way). For instance, performance in difficult problems such as fast mapping or inductive reasoning was correlated with independently assessed g in mice (Wass et al. Reference Wass, Denman-Brice, Light, Kolata, Smith and Matzel2012). Particularly strong evidence would include the demonstration that individuals recruit the same basic cognitive processes for such difficult problems that are also strongly correlated with g, such as selective attention or working memory capacity (Matzel et al. Reference Matzel, Sauce-Silva and Wass2013; Geary Reference Geary, Platek and Shackelford2009).
Finally, particularly rigorous validation would be based on extra-domain assays. Just as human g correlates with academic success, workplace success, health, and even happiness (for references, see section 1), one could in principle ask whether g in animals is correlated with outcomes such as the size of cultural repertoires in nature, the ability to rise in social dominance, or to find food during periods of scarcity, and thus survival and even fitness. Unfortunately, this approach is difficult to achieve because it requires both reliably quantifying g and the various real-life outcomes in animals under natural conditions. More importantly, g may not necessarily predict basic fitness measures such as reproductive success, because of possible tradeoffs between investment into improving general intelligence and other vital activities, such as vigilance or social monitoring.
Table 7 summarizes the issues we have discussed in the form of criteria that may be fruitful to guide future studies.
Table 7. Criteria that may be useful in guiding future efforts to (a) reliably identify g/G in nonhuman animals and (b) evaluate whether a statistically identified g/G captures intelligence in a broad sense: that is, reasoning ability and behavioral flexibility. The last two columns indicate to what extent corresponding criteria have been applied in rodents and primates. See text for details (the relevant sections are indicated in italics).
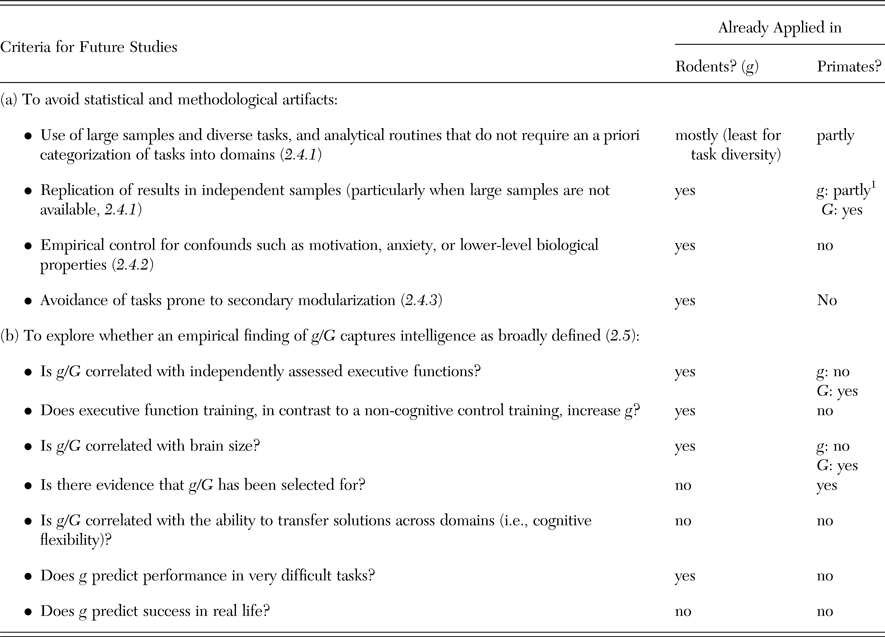
1 But not successfully: see Herrmann et al. (Reference Herrmann, Hernandez-Lloreda, Call, Hare and Tomasello2010b); Hopkins et al. (Reference Hopkins, Russel and Schaeffer2014); Woodley of Menie et al. (Reference Woodley of Menie, Fernandes and Hopkins2015)
3. Implications for the evolution of general intelligence
Taken together, although more validation remains to be done, especially in primates, the body of evidence is currently more consistent with the presence of domain-general cognitive abilities in primates and mice, reflected in g and G, than with the exclusive presence of independent, domain-specific cognitive adaptations. If general intelligence is not limited to humans, this inevitably leads to the question of the conditions favoring the evolution of general intelligence, to which we now turn. Whereas the evolution of Fodorian, specific, dedicated cognitive adaptations in response to recurrent fitness-relevant problems is seemingly straightforward (but see sect. 1.2.1), the evolution of general intelligence poses a puzzle. Domain-specific cognitive adaptations can be instantiated with modest amounts of neural tissue (Chittka & Niven Reference Chittka and Niven2009; Holekamp et al. Reference Holekamp, Dantzer, Stricker, Yoshida and Benson-Amram2015; Patton & Braithwaite Reference Patton and Braithwaite2015) and directly bring about fitness-relevant benefits. Domain-general cognitive ability, however, seems to require substantial amounts of additional expensive brain tissue (Deaner et al. Reference Deaner, Isler, Burkart and van Schaik2007; Reader et al. Reference Reader, Hager and Laland2011), and is not automatically linked to fitness-relevant benefits because survival-relevant skills have to be ontogenetically constructed during a process of learning (van Schaik & Burkart Reference van Schaik and Burkart2011). This ontogenetic construction may be more successful in individuals with higher cognitive ability, as posited by investment theory (Cattell Reference Cattell1987), but additional factors also come into play, which renders the link between cognitive ability and fitness-relevant skills more fragile. For instance, whether a survival-relevant skill is acquired may also depend on pure chance (van Schaik & Burkart Reference van Schaik and Burkart2011; van Schaik et al. Reference van Schaik, Burkart, Damerius, Forss, Koops, van Noordwijk and Schuppli2016). Furthermore, in order to more reliably translate general cognitive ability into fitness-relevant skills, some mechanisms for adaptive canalizations (as highlighted in sect. 1.2.2, Table 1) remain necessary, which have to coevolve or, if already present, be linked to the evolving domain-general cognitive processes. We are, therefore, faced with the puzzle that domain-general cognitive ability apparently evolved in at least some lineages, or perhaps even in birds and mammals in general, even though its evolution has had to overcome more obstacles compared to the emergence of domain-specific cognitive adaptations. Hence, the goal of this section is to delineate the conditions favoring the evolution of general intelligence.
3.1. General intelligence as response to domain-specific selection pressures
The most common approach to explain variation in cognition across species, which has a long and venerable tradition, is to look for specific cognitive challenges in the social or ecological environment and investigate to what extent species facing these challenges have evolved bigger brains (Dunbar & Shultz Reference Dunbar and Shultz2007a; Reference Dunbar and Shultz2007b; Holekamp et al. Reference Holekamp, Dantzer, Stricker, Yoshida and Benson-Amram2015; Humphrey Reference Humphrey, Bateson and Hinde1976; Jolly Reference Jolly1966; Parker Reference Parker2015; Parker & Gibson Reference Parker and Gibson1977). Comparative analyses, particularly in primates, have shown that brain size is indeed correlated across species with various social and ecological variables, such as social complexity based on bonded relationships (Dunbar Reference Dunbar1992; Dunbar & Shultz Reference Dunbar and Shultz2007b) and tactical deception (Byrne & Corp Reference Byrne and Corp2004), or extractive foraging (Parker Reference Parker2015), manipulative complexity (Heldstab et al. Reference Heldstab, Kosonen, Koski, Burkart, van Schaik and Isler2016), and perceived seasonality (van Woerden et al. Reference van Woerden, van Schaik and Isler2014; Reference van Woerden, van Schaik and Isler2010; Reference van Woerden, Willems, van Schaik and Isler2012). Shultz and Dunbar (Reference Shultz and Dunbar2006) presented similar analyses for ungulates, with similar conclusions.
Nonetheless, much variation in brain size across species remains unexplained by domain-specific pressures (Holekamp Reference Holekamp2007; van Schaik et al. Reference van Schaik, Isler and Burkart2012). Furthermore, not all species that excel in socio-cognitive tasks, most likely because of their complex social environment, also excel in non-social tasks and evolve big brains. Socio-cognitive abilities in hyenas, for instance, are on a par with those of the larger anthropoid primates, but there is no evidence that as in primates, this would be correlated with particularly powerful cognitive abilities outside of this domain (Holekamp et al. Reference Holekamp, Dantzer, Stricker, Yoshida and Benson-Amram2015). Likewise, callitrichid monkeys outperform their sister lineage, capuchin and squirrel monkeys, in socio-cognitive abilities, but the latter have superior physical cognition (Burkart & van Schaik Reference Burkart and van Schaik2010; Reference Burkart and van Schaik2016a; Reference Burkart and van Schaik2016b). For additional examples of how specific sophisticated cognitive skills can be achieved with very small brains, see Chittka and Niven (Reference Chittka and Niven2009) for insects or Patton and Braithwaite (Reference Patton and Braithwaite2015) for fish.
The crucial question thus is: Under what conditions do specific cognitive challenges result in an increase in general intelligence (and thus brain size) rather than in domain-specific cognitive solutions that do not require large amounts of brain tissue and do not translate into benefits in other domains too?
3.2. Direct selection on general intelligence
Some have argued that general cognitive ability is not the result of a domain-specific challenge but that it is directly selected so as to help animals cope with novel or unpredictable environments and overcome unusual or complex ecological challenges. According to this cognitive buffer hypothesis, large brains facilitate the construction of novel behavioral patterns through domain-general cognitive processes such as innovation and learning (Lefebvre et al. Reference Lefebvre, Reader and Sol2013; Sol 2009a). In support of this hypothesis, more innovative species tend to indeed have bigger brains – in birds (Lefebvre et al. Reference Lefebvre, Whittle, Lascaris and Finkelstein1997) and primates (Reader & Laland Reference Reader and Laland2002) – and innovation rates in the wild are correlated with G across primate species (Reader et al. Reference Reader, Hager and Laland2011). Furthermore, innovation rates and brain size, and thus presumably G, predict colonization success in birds (Sol et al. Reference Sol, Duncan, Blackburn, Cassey and Lefebvre2005), mammals (Sol et al. Reference Sol, Bacher, Reader and Lefebvre2008), amphibians and reptiles (Amiel et al. Reference Amiel, Tingley and Shine2011), and in fishes (Shumway Reference Shumway2008; but see Drake Reference Drake2007). Furthermore, large-brained birds use more successful learned strategies to avoid collision with human vehicles on roads (Husby & Husby Reference Husby and Husby2014). Finally, anthropoid primates (but not lemurs, rodents, and omnivorous carnivores) cognitively buffer environmental seasonality (S. Graber et al. in prep.; van Woerden et al. Reference van Woerden, van Schaik and Isler2014). Taken together, this work convincingly demonstrates that big brains are associated with greater behavioral flexibility and higher innovation rates under naturalistic settings, and that these in turn can be beneficial for a range of species when they face novel and unpredictable environments.
What remains to be answered in light of these obvious benefits, then, is why not all species evolved bigger and more-powerful brains. It is self-evident that all extant species are clearly smart enough for their current niche, but it is equally evident that a slightly better understanding that traces of a predator actually mark its presence, a better memory for which food sources already have been visited, or better object permanence to better keep track of a disappearing prey would convey a fitness benefit relative to conspecifics. We suggest that it is unlikely that focusing exclusively on potential benefits resulting from gains in brain size will further advance our understanding of the conditions under which domain-specific pressures lead to increased general intelligence. Rather, answering this question requires a focus not only on the benefits, but also on the costs of evolving a bigger brain.
3.3. Who can afford to evolve general intelligence? Cultural intelligence
Some species have larger brains than others, which, at least in primates, is associated with higher G. Why did these species respond to domain-specific selection pressures with an increase in general intelligence (see also sect. 3.1), or cope with environmental unpredictability by increasing their brain and intelligence, rather than opting for alternative, domain-specific adaptations (see also sect. 3.2)?
To answer these questions, it is important to keep in mind that the conditions under which large brains can evolve are to a substantial degree restricted by their costs (Isler & van Schaik Reference Isler and van Schaik2014). Brains are energy-hungry organs that consume a large proportion of the energy available to an organism, particularly in growing immatures (Kuzawa et al. Reference Kuzawa, Chugani, Grossman, Lipovich, Muzik, Hof, Wildman, Sherwood, Leonard and Lange2014). Thus, natural selection more readily favors an increase in brain size when this leads to an increase in net energy intake, a reduction in its variance, or ideally both. Furthermore, a big brain slows down the organism's development, which means that a species' ability to slow down its life history is a fundamental precondition for its opportunity to evolve larger brain size. Accordingly, the life-history filter approach (van Schaik et al. Reference van Schaik, Isler and Burkart2012) shows that slowing down life history, and thus evolving a larger brain, is only possible for species that can increase adult survival and are not subject to unavoidable extrinsic mortality, such as high predation pressure. Isler and van Schaik (Reference Isler and van Schaik2014) have shown that such a cost perspective can explain a substantial amount of variation in brain size across primates, and that allomaternal care plays an important role in accommodating the costs associated with bigger brains (in particular, because food subsidies by allomothers help pay for the energetic costs of the growing immatures, and because of life-history consequences; see also Burkart Reference Burkart, Call, Burghardt, Pepperberg, Snowdon and Zentall2017).
Natural selection thus evaluates the net fitness benefit of a bigger brain, which also takes the costs into account. The balance of benefits and costs is critically influenced by how efficiently an individual can translate brain tissue (or general cognitive potential) into survival-increasing innovations – that is, knowledge and skills. The cultural intelligence approach stresses that species that rely more systematically on social learning are more efficient in ontogenetically constructing survival-relevant skills (Herrmann et al. Reference Herrmann, Call, Hernández-Lloreda, Hare and Tomasello2007; van Schaik & Burkart Reference van Schaik and Burkart2011; van Schaik et al. Reference van Schaik, Isler and Burkart2012; Whiten & van Schaik Reference Whiten and van Schaik2007) because social influences are very powerful domain-general canalization processes (as highlighted in Table 1). Whereas in the human literature, many approaches stress the importance of social inputs in the development of intelligence (e.g., Moll & Tomasello Reference Moll and Tomasello2007; Tomasello Reference Tomasello1999), the evolutionary version of this approach that suggests that social learning also plays a crucial role for the evolution of intelligence and brain size has received far less attention. Importantly, it builds on a broad notion of social learning (Heyes Reference Heyes1994, Box Reference Box1984; see also van Schaik et al. [Reference van Schaik, Graber, Schuppli and Burkart2017], for a classification of social learning particularly suitable to test the predictions of the evolutionary dimension of the cultural intelligence hypothesis).
Consistent with the cultural intelligence approach, empirical results show that innovation rates in birds and primates are not only correlated with brain size or G, but also with the efficiency of social learning (Reader Reference Reader2003; Reader et al. Reference Reader, Hager and Laland2011). According to the cultural intelligence hypothesis, this is the case because, for species engaging systematically in social learning, additional brain tissue translates more reliably in survival-relevant skills, which lowers the threshold for evolution to favor an increase in brain size and general cognitive ability compared with species that do not rely on social learning. The frequency of opportunities for social learning is thus part of the answer why some lineages did evolve bigger brains, whereas others did not, even though they would all benefit from being more intelligent (van Schaik & Burkart Reference van Schaik and Burkart2011). Put in other words, we can use the pattern of solutions to the canalization problem (outlined in Table 1) to better understand under what conditions a species responds to a domain-specific selection pressure with a domain-general adaptation rather than with a narrow, domain-specific modular adaptation. The core message (to be derived from Table 1) was that all identified canalization problems can readily be overcome by social learning, and, therefore, species able to rely more on social learning should be more likely to be able to evolve domain-general cognitive adaptations. In sum, the cultural intelligence approach seems to best accommodate the findings of general intelligence as reviewed previously. For a more detailed comparison and discussion of the different approaches, see van Schaik et al. (Reference van Schaik, Isler and Burkart2012) and Burkart (Reference Burkart, Call, Burghardt, Pepperberg, Snowdon and Zentall2017).
The cultural intelligence hypothesis was originally developed to explain why humans have evolved far bigger brains and far greater intelligence than other great apes. Tomasello (Reference Tomasello1999; see also Herrmann et al. Reference Herrmann, Call, Hernández-Lloreda, Hare and Tomasello2007) stressed that humans have evolved a set of species-specific socio-cognitive skills that facilitate social transmission, by allowing us to participate and exchange knowledge in cultural groups from an early age on. In other words, humans have become specialized in making use of social inputs to ontogenetically construct their skills, and rather than having evolved predominantly into a “cognitive niche” (Pinker Reference Pinker2010), they have evolved into a “cultural niche” (Boyd et al. Reference Boyd, Richerson and Henrich2011). Our extreme dependence on the socially guided ontogenetic construction of skills can also explain why the intraspecific link between g and brain size within humans is relatively weak (Muthukrishna & Henrich Reference Muthukrishna and Henrich2016; Pietschnig et al. Reference Pietschnig, Penke, Wicherts, Zeiler and Voracek2015).
Humans can thus be seen as a special case of cultural intelligence, due to the active involvement of caretakers and the improved imitative abilities of our species. This view is consistent with approaches to human cognitive evolution that stress the role of allomaternal care, which not only results in energy subsidies to growing immatures but also increases the scope of social learning through the availability of more, and more tolerant, role models, who eventually also engage in teaching (Burkart & van Schaik Reference Burkart and van Schaik2016a; 2016b; Burkart et al. Reference Burkart, Hrdy and van Schaik2009; Hrdy Reference Hrdy2009; Isler & van Schaik Reference Isler and van Schaik2014; Kline Reference Kline2015).
4. Discussion
4.1. Preliminary synthesis
The current body of evidence reviewed in this article is arguably most consistent with general intelligence not being unique to humans but also present in other species, even though much validation remains to be done, as outlined in sections 2.4 and 2.5. At present, the best-supported model for both animals and humans therefore views the ecological and social cognitive skills that can be measured in a species as the result of two pathways (indicated in Fig. 3).

Figure 3. The origin of cognitive skills. Green: Ecological and social cognitive skills that can be measured in animals and that are visible to natural selection because they can result in fitness benefits. Yellow: Empirical interrelations between brain size, general intelligence, and executive functions. The latter two entities are only visible to selection to the extent that they are translated into fitness-enhancing cognitive skills. Blue: Adaptive canalizations that either guide the ontogenetic construction of cognitive skills from general intelligence (see Table 1 for details) or represent fully fledged Fodorian, modular adaptations that are independent of the domain-general (yellow) nexus.
In the downward pathway, cognitive skills result from general intelligence, which shows strong empirical correlations with brain size and executive functions. These skills correspond to Cattell's (Reference Cattell1963) crystallized intelligence and Geary's (Reference Geary2005) secondary learning. In this case, the cognitive skills are ontogenetically constructed, facilitated by mechanisms of adaptive canalization beyond Fodorian modularity (summarized in Table 1) and eventually may become automatized (secondary modularization, which makes these skills particularly difficult to identify). As stressed by the cultural intelligence approach, social learning is a particularly efficient mechanism of ontogenetic canalization, particularly in large-brained animals. In the upward pathway, cognitive skills directly emerge as a result of dedicated, Fodorian cognitive modules that are independent of general intelligence, executive functions (Firestone & Scholl Reference Firestone and Scholl2015), or brain size.
These two pathways to cognitive skills can coexist, and in fact almost certainly do. This situation has major implications. First, closely related, big-brained species that rely to some significant extent on the downward pathway and thus general intelligence may nevertheless exhibit rather distinct social and ecological skill sets. Some of their species-specific ontogenetic canalization mechanisms can result in species differences in performance in specific domains, such as extractive foraging and tool use. Second, species may not primarily vary with regard to whether they have g, but with regard to the relative importance of these two pathways in building their skill sets, consistent with the increasing evidence for g in several nonhuman species. Approaches like cultural intelligence and the expensive brain framework delineate the conditions under which one or the other is more likely to evolve. This model is thus consistent with the broad pattern of results summarized in this review, including the results of mixed-species studies (sect. 2.3), and also with the idea of evolutionary continuity.
This preliminary synthesis suggests there is an alternative way of estimating the importance of general intelligence in a given species. Rather than exclusively relying on comparing the percentage of variance in performance explained by g (which in fact may be misleading, under the conditions outlined in sect. 2.4.1), one may attempt to estimate the importance of one pathway over the other in constructing an individual's skill set. To do so, it is crucial to be able to distinguish the origin of the skills in the green box (see Fig. 3), whether they result from the upward or the downward pathway. This is particularly difficult because eventually, skills constructed via the upper pathway may become automatized, and thus difficult to distinguish from primary modules (see also Table 2 in sect. 1.2.3). To identify them, one needs to show that they critically rely on EFs (see also Table 6b) and show signs of being effortfully learned (see also Meulman et al. Reference Meulman, Seed and Mann2013; Schuppli et al. Reference Schuppli, Meulman, Forss, van Noordwijk and van Schaik2016). This is most feasible when the learning is social, either by directly recording the socially induced patterns of attention and practice (e.g., Jaeggi et al. Reference Jaeggi, Dunkel, Van Noordwijk, Wich, Sura and van Schaik2010) or by interspecific cross-fostering (see section 2.4.3) where this is feasible. This alternative way of estimating the importance of general intelligence in a given species may turn out to be a promising complement to the alternatives pursued in nonhuman intelligence research so far.
4.2. Conclusions
Overall, the body of evidence from comparative studies lends increasing support to the notion that general intelligence is not unique to humans but also present in nonhuman animals and thus is not as tied up with language as some have suggested. Intraspecific evidence for g is particularly strong in rodents, whereas interspecific evidence (G) finds most support from primate and bird studies. Nevertheless, the rather young field of research into animal general intelligence still needs to mature just as work on human intelligence has taken decades to mature.
This enterprise can obviously profit from better integrating knowledge accumulated in the longstanding tradition of human psychometrics, not only with respect to the methodological aspects highlighted previously, but also to conceptual issues. For instance, obvious parallels exist between investment theory (Cattell Reference Cattell1987) and cultural intelligence approaches; pursuing them further may lead to novel insights. In other domains, however, superficial similarities are misleading. Modern massive modularity, for instance, based on very broad notions of modularity and inspired by evolutionary biology (Barrett Reference Barrett, Zeigler-Hill, Welling and Schackelford2015), hardly informs the debate about whether general intelligence exists in nonhuman animals. Among nonhuman animals, the ancestral state most likely corresponds to animal minds being made up entirely of dedicated modular adaptations (Shettleworth Reference Shettleworth, Vonk and Shackelford2012a; Reference Shettleworth2012b). Among extant species, the question is, which behaviors are (still) regulated this way?
It is worth emphasizing that fruitful inputs can flow in the other direction too. For instance, the availability of valid animal models of general intelligence increasingly allows studying the underlying neurobiological and genetic mechanisms in ways that would not be possible in human studies (reviewed in Galsworthy et al. Reference Galsworthy, Arden, Chabris, Finkel and Reynolds2014; Matzel et al. Reference Matzel, Sauce-Silva and Wass2013; Plomin Reference Plomin2001). Furthermore, via animal studies we can experimentally address the role of factors such as exploration tendency, known to be linked to g in mice (Grossman et al. Reference Grossman, Hale, Light, Kolata, Townsend, Goldfarb, Kusnecov and Matzel2007; Light et al. Reference Light, Kolata, Hale, Grossman and Matzel2008), most likely via mechanisms stressed by investment theory (Cattell Reference Cattell1987). Finally, comparative studies are indispensable in addressing the broader question of where, why, and how g evolved. Among the most promising evolutionary explanations for general intelligence is the cultural intelligence approach, which predicts the coevolution of social learning and general intelligence. This perspective is strongly supported by interspecific studies where social learning, but also other social abilities such as deception, are strongly correlated with G across species (e.g., Reader et al. Reference Reader, Hager and Laland2011) and where brain size is linked to opportunities for social learning during development (van Schaik et al. Reference van Schaik, Isler and Burkart2012).
A final issue concerns both animal and human studies. In most intraspecific studies, socio-cognitive tasks were not part of the test battery, but where they were, the results were inconclusive. Thus, whereas Hopkins et al. (Reference Hopkins, Russel and Schaeffer2014; Woodley of Menie et al. Reference Woodley of Menie, Fernandes and Hopkins2015) found socio-cognitive abilities loading on g in chimpanzees, Herrmann et al. (Reference Herrmann, Hernandez-Lloreda, Call, Hare and Tomasello2010b) did not, neither in chimpanzees nor children. This may be because the intraspecific measures of socio-cognitive abilities used so far are less suitable than interspecific ones, for instance, because they sometimes produce ceiling or floor effects. However, human test batteries typically also do not include social cognition, and the relationship between general intelligence and socio-cognitive abilities in humans therefore remains poorly understood (Korman et al. Reference Korman, Voiklis and Malle2015). Investigating the link between socio-cognitive abilities and general intelligence within humans thus is an important research priority.
ACKNOWLEDGMENTS
We would like to thank Sonja Koski and Kay Holekamp for discussion, Sandra Heldstab for performing the PGLS analyses for Figure 2, and Jonathan Evans, Barbara Finlay, David Geary, and Willem Frankenhuis, as well as the anonymous reviewers for their valuable and very constructive feedback. This work was supported by Swiss National Funds projects 130383 and 160363 to JB, and by the A. H. Schultz-Stiftung.
Target article
The evolution of general intelligence
Related commentaries (28)
G and g: Two markers of a general cognitive ability, or none?
G but not g: In search of the evolutionary continuity of intelligence
A pointer's hypothesis of general intelligence evolved from domain-specific demands
An all-positive correlation matrix is not evidence of domain-general intelligence
Coexistence of general intelligence and specialized modules
Contemporary evolutionary psychology and the evolution of intelligence
Disentangling learning from knowing: Does associative learning ability underlie performances on cognitive test batteries?
Domains of generality
Evolution, brain size, and variations in intelligence
General intelligence does not help us understand cognitive evolution
General intelligence is a source of individual differences between species: Solving an anomaly
General intelligence is an emerging property, not an evolutionary puzzle
Genomic data can illuminate the architecture and evolution of cognitive abilities
Habit formation generates secondary modules that emulate the efficiency of evolved behavior
Hierarchy, multidomain modules, and the evolution of intelligence
It's time to move beyond the “Great Chain of Being”
Negative results are needed to show the specific value of a cultural explanation for g
Of mice and men, nature and nurture, and a few red herrings
Taking a multiple intelligences (MI) perspective
The evolution of analytic thought?
The evolution of fluid intelligence meets formative g
The evolution of general intelligence in all animals and machines
The false dichotomy of domain-specific versus domain-general cognition
Theory of mind: A foundational component of human general intelligence
Understanding the relationship between general intelligence and socio-cognitive abilities in humans
When does cultural transmission favour or instead substitute for general intelligence?
Where is the evidence for general intelligence in nonhuman animals?
“Birdbrains” should not be ignored in studying the evolution of g
Author response
Future directions for studying the evolution of general intelligence