Introduction
Increased rates of cardiovascular diseases (CVDs) have become a major area of concern for patients with schizophrenia (SCZ) or bipolar disorder (BD) (Weiner et al., Reference Weiner, Warren and Fiedorowicz2011; Ringen et al., Reference Ringen, Engh, Birkenaes, Dieset and Andreassen2014). People with SCZ have a life expectancy of around 15–20 years shorter than the average population, while the life expectancy for bipolar patients is 10–15 years shorter (Laursen, Reference Laursen2011). Deaths from CVDs have been proposed as a major contributor to the increased mortality (Laursen, Reference Laursen2011; Ringen et al., Reference Ringen, Engh, Birkenaes, Dieset and Andreassen2014).
The metabolic syndrome (MetS) is a key risk factor for cardiovascular morbidity and mortality. It represents a cluster of metabolic abnormalities, including dyslipidemia, impaired glucose tolerance, insulin resistance, hypertension and central obesity (Kaur, Reference Kaur2014). A raised prevalence of MetS has been observed in both SCZ (McEvoy et al., Reference McEvoy, Meyer, Goff, Nasrallah, Davis, Sullivan, Meltzer, Hsiao, Scott Stroup and Lieberman2005) and BD (Vancampfort et al., Reference Vancampfort, Vansteelandt, Correll, Mitchell, De Herdt, Sienaert, Probst and De Hert2013) patients.
The underlying causes for increased risk of MetS are not completely understood. A variety of factors, including smoking, physical inactivity, inadequate health-care services, medications and underlying genetics may all contribute to the heightened risks (Ringen et al., Reference Ringen, Engh, Birkenaes, Dieset and Andreassen2014). In particular, anti-psychotics and mood stabilizers are known contributors to metabolic abnormalities (Newcomer, Reference Newcomer2006; Pramyothin and Khaodhiar, Reference Pramyothin and Khaodhiar2010). Nevertheless, metabolic abnormalities have also been observed in drug-naïve SCZ patients. For example, meta-analyses (Perry et al., Reference Perry, McIntosh, Weich, Singh and Rees2016; Greenhalgh et al., Reference Greenhalgh, Gonzalez-Blanco, Garcia-Rizo, Fernandez-Egea, Miller, Arroyo and Kirkpatrick2017; Pillinger et al., Reference Pillinger, Beck, Gobjila, Donocik, Jauhar and Howes2017a) revealed worse glucose profiles in drug-naïve SCZ subjects than in controls. Another meta-analysis reported elevated triglycerides, but lower low-density cholesterol (LDL) and total cholesterol (TC) in first-episode psychosis patients (Pillinger et al., Reference Pillinger, Beck, Stubbs and Howes2017b) [see (Chadda et al., Reference Chadda, Ramshankar, Deb and Sood2013) for review].
Very few studies have investigated metabolic traits in drug-naïve bipolar patients. A recent study showed an increased rate of insulin resistance in these patients, but there was no difference in the rate of MetS (Guha et al., Reference Guha, Bhowmick, Mazumder, Ghosal, Chakraborty and Burman2014). Nevertheless, SCZ and BD are known to have a partially shared genetic basis (Cardno and Owen, Reference Cardno and Owen2014), and they share certain clinical characteristics and both are responsive to antipsychotics (Murray et al., Reference Murray, Sham, Van Os, Zanelli, Cannon and McDonald2004). Given the relationship between SCZ and BD, it will be interesting to explore whether BD itself is linked to cardiometabolic (CM) abnormalities.
Regarding the limitations of prior work, previous individual studies are usually of small sample size (most N < 100), and often included only a subset of metabolic parameters. Although a few meta-analyses have been performed, the range of traits studied was limited (mainly glucose and lipid) and heterogeneity among studies was inevitable (see online Supplementary Text S1). Another limitation is that causal relationship is difficult to infer due to cross-sectional design and confounding factors. As (germline) genetic variations are not affected by drugs, exploration of shared genetic bases may be more useful in discerning whether the disorders (SCZ/BD) themselves contribute to CM abnormalities. Also, our study included large samples from GWAS, totaling over a million participants.
As both psychiatric and CM traits are highly heritable, it is reasonable to hypothesize that a shared genetic basis may contribute to the comorbidities. In a related work, Andreassen et al. (Reference Andreassen, Djurovic, Thompson, Schork, Kendler, O'Donovan, Rujescu, Werge, van de Bunt, Morris, McCarthy, Roddey, McEvoy, Desikan and Dale2013) proposed an approach to identify loci associated with both SCZ and CM traits. Compared to this work, we also covered BD, analyzed a wider range of CM traits, utilized a much larger SCZ sample, and investigated the directions of associations (see online Supplementary Text S1).
The overall analytic workflow is described in Fig. 1. Firstly, we made use of large-scale GWAS meta-analyses results for SCZ, BD and a comprehensive panel of 28 CM traits to test for associations of polygenic risk scores (PRS). Linkage disequilibrium score regression (LDSR) was conducted as well. We also examined PRS associations with cardiovascular risk factors in the Northern Finland Birth Cohort (NFBC) with individual-level data. We then performed Mendelian randomization (MR) analysis to assess the causal relationship between the two groups of disorders. Finally, we ‘zoomed in’ to discover the genetic variants shared between SCZ and BD with each metabolic trait and inferred the likely involved pathways.

Fig. 1. The overall analytic workflow. First, we made use of large-scale GWAS meta-analyses results for SCZ, BD and a comprehensive panel of 28 metabolic and cardiovascular traits to test for shared genetic bases by PRS and LDSR. We also examined PRS associations with cardiovascular risk factors in the NFBC with individual genotype and phenotype data. We then performed MR analysis to assess the causal relationship between the two groups of disorders. Finally, we ‘zoomed in’ to discover the genetic variants shared between SCZ and BD with each metabolic trait and inferred the likely involved pathways by gene-set analyses.
We adopted a variety of analytic methods as each approach has its own strengths and limitations. For example, PRS is a widely used technique, but it is relatively difficult to account for linkage disequilibrium (LD) and sample overlap in PRS analyses. Although LDSR takes into account these factors, it relies on other assumptions, for example that the causal variants are randomly distributed in the genome regardless of the LD structure, and (ideally) all single nucleotide polymorphisms (SNPs) contribute equal variances. While PRS analyses are often performed on individual-level data, in order to increase the sample size, we also employed a more complex analytical technique that requires only summary statistics. On the other hand, MR is used to assess the causal relationship between the traits.
To our knowledge, this is the first systemic and the most comprehensive study to date on the shared genetic bases of CM traits with both SCZ and BD, and the first to uncover shared genetic variants between BD and CM diseases with large-scale GWAS data. Except for a recent study, which employed MR to study the relationship between body mass index (BMI) and psychiatric disorders (Hartwig et al., Reference Hartwig, Bowden, Loret de Mola, Tovo-Rodrigues, Davey Smith and Horta2016), we are unaware of other works studying causal links of SCZ/BD with CM traits.
Materials and methods
Due to space limits, readers are encouraged to refer to online Supplementary Text S1 for details.
GWAS samples for summary statistics
Summary statistics for SCZ (Schizophrenia Working Group of the Psychiatric Genomics Consortium, 2014) was based on a large-scale meta-analysis (N = 82 315) available from https://www.med.unc.edu/pgc. Summary statistics for BD was based on a recent study (Hou et al., Reference Hou, Bergen, Akula, Song, Hultman, Landen, Adli, Alda, Ardau, Arias, Aubry, Backlund, Badner, Barrett, Bauer, Baune, Bellivier, Benabarre, Bengesser, Berrettini, Bhattacharjee, Biernacka, Birner, Bloss, Brichant-Petitjean, Bui, Byerley, Cervantes, Chillotti, Cichon, Colom, Coryell, Craig, Cruceanu, Czerski, Davis, Dayer, Degenhardt, Del Zompo, DePaulo, Edenberg, Etain, Falkai, Foroud, Forstner, Frisen, Frye, Fullerton, Gard, Garnham, Gershon, Goes, Greenwood, Grigoroiu-Serbanescu, Hauser, Heilbronner, Heilmann-Heimbach, Herms, Hipolito, Hitturlingappa, Hoffmann, Hofmann, Jamain, Jimenez, Kahn, Kassem, Kelsoe, Kittel-Schneider, Kliwicki, Koller, Konig, Lackner, Laje, Lang, Lavebratt, Lawson, Leboyer, Leckband, Liu, Maaser, Mahon, Maier, Maj, Manchia, Martinsson, McCarthy, McElroy, McInnis, McKinney, Mitchell, Mitjans, Mondimore, Monteleone, Muhleisen, Nievergelt, Nothen, Novak, Nurnberger, Nwulia, Osby, Pfennig, Potash, Propping, Reif, Reininghaus, Rice, Rietschel, Rouleau, Rybakowski, Schalling, Scheftner, Schofield, Schork, Schulze, Schumacher, Schweizer, Severino, Shekhtman, Shilling, Simhandl, Slaney, Smith, Squassina, Stamm, Stopkova, Streit, Strohmaier, Szelinger, Tighe, Tortorella, Turecki, Vieta, Volkert, Witt, Wright, Zandi, Zhang, Zollner and McMahon2016) (N = 40 255) available from https://www.ebi.ac.uk/gwas/downloads/summary-statistics. We also obtained GWAS summary statistics for a range of CM traits (references and sample size given in online Supplementary Text S1), including LDL, high-density lipoprotein (HDL), TC, triglycerides (TG), BMI, fasting glucose (FG), fasting insulin (INS), waist-to-hip ratio (WHR), type 2 diabetes, coronary artery disease (CAD), leptin, adiponectin, systolic blood pressure and diastolic blood pressure (SBP/DBP), body fat percentage, subcutaneous adipose tissue (SAT) volume, visceral adipose tissue volume, pericardial fat (PAT) volume, SAT attenuation (SATHU), visceral adipose tissue attenuation (VATHU) and VAT/SAT ratio. For FG, INS, WHR, leptin, VAT, VAT/SAT ratio, we obtained the summary statistics both with and without adjustment for BMI. For PAT, we included test statistics adjusted for height and weight. Summary statistics was downloaded via http://ldsc.broadinstitute.org and https://grasp.nhlbi.nih.gov/FullResults.aspx. The analyses are primarily based on samples of European ancestry.
Polygenic score analysis
PRS can be formulated as a weighted sum of allelic counts, with the weights determined by the effect sizes of individual genetic variants. PRS analysis was conducted using two different methods: individual-level genotype data and summary statistics.
Individual-level analysis: PRS testing in the NFBC 1966
Individual-level PRS testing was performed on GWAS data of the NFBC 1966. Data was accessed from dbGaP (phs000276.v2.p1) (Sabatti et al., Reference Sabatti, Service, Hartikainen, Pouta, Ripatti, Brodsky, Jones, Zaitlen, Varilo, Kaakinen, Sovio, Ruokonen, Laitinen, Jakkula, Coin, Hoggart, Collins, Turunen, Gabriel, Elliot, McCarthy, Daly, Jarvelin, Freimer and Peltonen2009). We included TG, HDL, LDL, BMI, WHR, FG, INS, SBP and DBP in the analysis. After quality control procedures, 4982 individuals and 334 458 SNPs were retained (see online Supplementary Text S1). Gender and the top ten principal components were included as covariates (Price et al., Reference Price, Patterson, Plenge, Weinblatt, Shadick and Reich2006). Analyses were repeated with and without BMI as a covariate.
PRS testing based on GWAS summary statistics
In this approach, only the summary statistics for each pair of traits are required. Association testing was carried out by a method first described by Johnson (Reference Johnson2012). Details of this methodology were also described in several other studies (Ehret et al., Reference Ehret, Munroe, Rice, Bochud, Johnson, Chasman, Smith, Tobin, Verwoert, Hwang, Pihur, Vollenweider, O'Reilly, Amin, Bragg-Gresham, Teumer, Glazer, Launer, Zhao, Aulchenko, Heath, Sober, Parsa, Luan, Arora, Dehghan, Zhang, Lucas, Hicks, Jackson, Peden, Tanaka, Wild, Rudan, Igl, Milaneschi, Parker, Fava, Chambers, Fox, Kumari, Go, van der Harst, Kao, Sjogren, Vinay, Alexander, Tabara, Shaw-Hawkins, Whincup, Liu, Shi, Kuusisto, Tayo, Seielstad, Sim, Nguyen, Lehtimaki, Matullo, Wu, Gaunt, Onland-Moret, Cooper, Platou, Org, Hardy, Dahgam, Palmen, Vitart, Braund, Kuznetsova, Uiterwaal, Adeyemo, Palmas, Campbell, Ludwig, Tomaszewski, Tzoulaki, Palmer, Aspelund, Garcia, Chang, O'Connell, Steinle, Grobbee, Arking, Kardia, Morrison, Hernandez, Najjar, McArdle, Hadley, Brown, Connell, Hingorani, Day, Lawlor, Beilby, Lawrence, Clarke, Hopewell, Ongen, Dreisbach, Li, Young, Bis, Kahonen, Viikari, Adair, Lee, Chen, Olden, Pattaro, Bolton, Kottgen, Bergmann, Mooser, Chaturvedi, Frayling, Islam, Jafar, Erdmann, Kulkarni, Bornstein, Grassler, Groop, Voight, Kettunen, Howard, Taylor, Guarrera, Ricceri, Emilsson, Plump, Barroso, Khaw, Weder, Hunt, Sun, Bergman, Collins, Bonnycastle, Scott, Stringham, Peltonen, Perola, Vartiainen, Brand, Staessen, Wang, Burton, Soler Artigas, Dong, Snieder, Wang, Zhu, Lohman, Rudock, Heckbert, Smith, Wiggins, Doumatey, Shriner, Veldre, Viigimaa, Kinra, Prabhakaran, Tripathy, Langefeld, Rosengren, Thelle, Corsi, Singleton, Forrester, Hilton, McKenzie, Salako, Iwai, Kita, Ogihara, Ohkubo, Okamura, Ueshima, Umemura, Eyheramendy, Meitinger, Wichmann, Cho, Kim, Lee, Scott, Sehmi, Zhang, Hedblad, Nilsson, Smith, Wong, Narisu, Stancakova, Raffel, Yao, Kathiresan, O'Donnell, Schwartz, Ikram, Longstreth, Mosley, Seshadri, Shrine, Wain, Morken, Swift, Laitinen, Prokopenko, Zitting, Cooper, Humphries, Danesh, Rasheed, Goel, Hamsten, Watkins, Bakker, van Gilst, Janipalli, Mani, Yajnik, Hofman, Mattace-Raso, Oostra, Demirkan, Isaacs, Rivadeneira, Lakatta, Orru, Scuteri, Ala-Korpela, Kangas, Lyytikainen, Soininen, Tukiainen, Wurtz, Ong, Dorr, Kroemer, Volker, Volzke, Galan, Hercberg, Lathrop, Zelenika, Deloukas, Mangino, Spector, Zhai, Meschia, Nalls, Sharma, Terzic, Kumar, Denniff, Zukowska-Szczechowska, Wagenknecht, Fowkes, Charchar, Schwarz, Hayward, Guo, Rotimi, Bots, Brand, Samani, Polasek, Talmud, Nyberg, Kuh, Laan, Hveem, Palmer, van der Schouw, Casas, Mohlke, Vineis, Raitakari, Ganesh, Wong, Tai, Cooper, Laakso, Rao, Harris, Morris, Dominiczak, Kivimaki, Marmot, Miki, Saleheen, Chandak, Coresh, Navis, Salomaa, Han, Zhu, Kooner, Melander, Ridker, Bandinelli, Gyllensten, Wright, Wilson, Ferrucci, Farrall, Tuomilehto, Pramstaller, Elosua, Soranzo, Sijbrands, Altshuler, Loos, Shuldiner, Gieger, Meneton, Uitterlinden, Wareham, Gudnason, Rotter, Rettig, Uda, Strachan, Witteman, Hartikainen, Beckmann, Boerwinkle, Vasan, Boehnke, Larson, Jarvelin, Psaty, Abecasis, Chakravarti, Elliott, van Duijn, Newton-Cheh, Levy, Caulfield and Johnson2011; Dastani et al., Reference Dastani, Hivert, Timpson, Perry, Yuan, Scott, Henneman, Heid, Kizer, Lyytikainen, Fuchsberger, Tanaka, Morris, Small, Isaacs, Beekman, Coassin, Lohman, Qi, Kanoni, Pankow, Uh, Wu, Bidulescu, Rasmussen-Torvik, Greenwood, Ladouceur, Grimsby, Manning, Liu, Kooner, Mooser, Vollenweider, Kapur, Chambers, Wareham, Langenberg, Frants, Willems-Vandijk, Oostra, Willems, Lamina, Winkler, Psaty, Tracy, Brody, Chen, Viikari, Kahonen, Pramstaller, Evans, St Pourcain, Sattar, Wood, Bandinelli, Carlson, Egan, Bohringer, van Heemst, Kedenko, Kristiansson, Nuotio, Loo, Harris, Garcia, Kanaya, Haun, Klopp, Wichmann, Deloukas, Katsareli, Couper, Duncan, Kloppenburg, Adair, Borja, Wilson, Musani, Guo, Johnson, Semple, Teslovich, Allison, Redline, Buxbaum, Mohlke, Meulenbelt, Ballantyne, Dedoussis, Hu, Liu, Paulweber, Spector, Slagboom, Ferrucci, Jula, Perola, Raitakari, Florez, Salomaa, Eriksson, Frayling, Hicks, Lehtimaki, Smith, Siscovick, Kronenberg, van Duijn, Loos, Waterworth, Meigs, Dupuis, Richards, Voight, Scott, Steinthorsdottir, Dina, Welch, Zeggini, Huth, Aulchenko, Thorleifsson, McCulloch, Ferreira, Grallert, Amin, Wu, Willer, Raychaudhuri, McCarroll, Hofmann, Segre, van Hoek, Navarro, Ardlie, Balkau, Benediktsson, Bennett, Blagieva, Boerwinkle, Bonnycastle, Bostrom, Bravenboer, Bumpstead, Burtt, Charpentier, Chines, Cornelis, Crawford, Doney, Elliott, Elliott, Erdos, Fox, Franklin, Ganser, Gieger, Grarup, Green, Griffin, Groves, Guiducci, Hadjadj, Hassanali, Herder, Isomaa, Jackson, Johnson, Jorgensen, Kao, Kong, Kraft, Kuusisto, Lauritzen, Li, Lieverse, Lindgren, Lyssenko, Marre, Meitinger, Midthjell, Morken, Narisu, Nilsson, Owen, Payne, Petersen, Platou, Proenca, Prokopenko, Rathmann, Rayner, Robertson, Rocheleau, Roden, Sampson, Saxena, Shields, Shrader, Sigurdsson, Sparso, Strassburger, Stringham, Sun, Swift, Thorand, Tichet, Tuomi, van Dam, van Haeften, van Herpt, van Vliet-Ostaptchouk, Walters, Weedon, Wijmenga, Witteman, Bergman, Cauchi, Collins, Gloyn, Gyllensten, Hansen, Hide, Hitman, Hofman, Hunter, Hveem, Laakso, Morris, Palmer, Rudan, Sijbrands, Stein, Tuomilehto, Uitterlinden, Walker, Watanabe, Abecasis, Boehm, Campbell, Daly, Hattersley, Pedersen, Barroso, Groop, Sladek, Thorsteinsdottir, Wilson, Illig, Froguel, van Duijn, Stefansson, Altshuler, Boehnke, McCarthy, Soranzo, Wheeler, Glazer, Bouatia-Naji, Magi, Randall, Elliott, Rybin, Dehghan, Hottenga, Song, Goel, Lajunen, Doney, Cavalcanti-Proenca, Kumari, Timpson, Zabena, Ingelsson, An, O'Connell, Luan, Elliott, Roccasecca, Pattou, Sethupathy, Ariyurek, Barter, Beilby, Ben-Shlomo, Bergmann, Bochud, Bonnefond, Borch-Johnsen, Bottcher, Brunner, Bumpstead, Chen, Chines, Clarke, Coin, Cooper, Crisponi, Day, de Geus, Delplanque, Fedson, Fischer-Rosinsky, Forouhi, Franzosi, Galan, Goodarzi, Graessler, Grundy, Gwilliam, Hallmans, Hammond, Han, Hartikainen, Hayward, Heath, Hercberg, Hillman, Hingorani, Hui, Hung, Kaakinen, Kaprio, Kesaniemi, Kivimaki, Knight, Koskinen, Kovacs, Kyvik, Lathrop, Lawlor, Le Bacquer, Lecoeur, Li, Mahley, Mangino, Martinez-Larrad, McAteer, McPherson, Meisinger, Melzer, Meyre, Mitchell, Mukherjee, Naitza, Neville, Orru, Pakyz, Paolisso, Pattaro, Pearson, Peden, Pedersen, Pfeiffer, Pichler, Polasek, Posthuma, Potter, Pouta, Province, Rice, Ripatti, Rivadeneira, Rolandsson, Sandbaek, Sandhu, Sanna, Sayer, Scheet, Seedorf, Sharp, Shields, Sigurethsson, Sijbrands, Silveira, Simpson, Singleton, Smith, Sovio, Swift, Syddall, Syvanen, Tonjes, Uitterlinden, van Dijk, Varma, Visvikis-Siest, Vitart, Vogelzangs, Waeber, Wagner, Walley, Ward, Watkins, Wild, Willemsen, Witteman, Yarnell, Zelenika, Zethelius, Zhai, Zhao, Zillikens, Borecki, Meneton, Magnusson, Nathan, Williams, Silander, Bornstein, Schwarz, Spranger, Karpe, Shuldiner, Cooper, Serrano-Rios, Lind, Palmer, Hu, Franks, Ebrahim, Marmot, Wright, Stumvoll, Hamsten, Buchanan, Valle, Rotter, Penninx, Boomsma, Cao, Scuteri, Schlessinger, Uda, Ruokonen, Jarvelin, Peltonen, Mooser, Musunuru, Smith, Edmondson, Stylianou, Koseki, Pirruccello, Chasman, Johansen, Fouchier, Peloso, Barbalic, Ricketts, Bis, Feitosa, Orho-Melander, Melander, Li, Cho, Go, Kim, Lee, Park, Kim, Sim, Ong, Croteau-Chonka, Lange, Smith, Ziegler, Zhang, Zee, Whitfield, Thompson, Surakka, Smit, Sinisalo, Scott, Saharinen, Sabatti, Rose, Roberts, Rieder, Parker, Pare, O'Donnell, Nieminen, Nickerson, Montgomery, McArdle, Masson, Martin, Marroni, Lucas, Luben, Lokki, Lettre, Launer, Lakatta, Laaksonen, Konig, Khaw, Kaplan, Johansson, Janssens, Igl, Hovingh, Hengstenberg, Havulinna, Hastie, Harris, Haritunians, Hall, Groop, Gonzalez, Freimer, Erdmann, Ejebe, Doring, Dominiczak, Demissie, de Faire, Caulfield, Boekholdt, Assimes, Quertermous, Seielstad, Wong, Tai, Feranil, Kuzawa, Taylor, Gabriel, Holm, Gudnason, Krauss, Ordovas, Munroe, Kooner, Tall, Hegele, Kastelein, Schadt, Strachan, Reilly, Samani, Schunkert, Cupples, Sandhu, Ridker, Rader and Kathiresan2012; Palla and Dudbridge, Reference Palla and Dudbridge2015) and see online Supplementary Text S1. The formula is equivalent to the ‘inverse variance weighted’ (IVW) approach in MR (discussed below). However, in a PRS analysis, we do not require the variants to be strongly associated with the disease and pleiotropic effects are allowed.
In order to investigate the bi-directional effects of the polygenic risk of SCZ and BD on metabolic traits, we performed two sets of analyses, one using PRS from SCZ/BD to regress on CM traits and the other using PRS from CM traits to regress on SCZ/BD.
Analysis in PRsice
For both types of analyses (summary statistics and individual genotype-based) listed above, PRS analyses were performed by ‘PRsice’ (Euesden et al., Reference Euesden, Lewis and O'Reilly2015). Details are given in online Supplementary Text S1.
Control for multiple testing by the false discovery rate (FDR) approach
Multiple testing was corrected by the FDR procedure, which controls the expected proportion of false positives (FP) among those declared to be significant. As an example, among all the hypothesis with FDR (or q-value) <0.05, we expect that on average the proportion of FP will be <5% (or the proportion of true positives is expected to be >95%). In this study, FDR <0.05 is regarded as significant while hypotheses with corresponding FDR between 0.05 and 0.1 are considered suggestive (see online Supplementary Text S1).
Cross-trait LDSR
Cross-trait LDSR was performed to assess genetic correlations (Bulik-Sullivan et al., Reference Bulik-Sullivan, Finucane, Anttila, Gusev, Day, Loh, ReproGen, Duncan, Perry, Patterson, Robinson, Daly, Price and Neale2015). We employed the LDSC program (https://github.com/bulik/ldsc, ver 1.0.0, accessed June 2017) for the analysis, following default parameter settings. This approach allows sample overlap and accounts for LD between markers. The program ‘popcorn’, which accounts for differing ethnic groups of samples, was used for LDSR involving the Japanese DM sample (Brown et al., Reference Brown, Ye, Price and Zaitlen2016). We also assessed the significance of the cross-trait LDSC intercept, which reflects the degree of sample overlap. LDSR was not conducted for SBP and DBP as these were exome-based studies and LDSC was not designed for such studies.
MR analysis
Next, we performed MR analysis to assess causal relationships between SCZ/BD and CM disorders. MR utilized genetic variants as ‘instruments’ to represent the risk factor and analyzed the relationship with an outcome. Intuitively, MR is analogous to a randomized controlled trial (RCT). For example, in MR subjects with genetically lower LDL are analog to receiving a lipid-lowering drug in RCT. Compared to conventional observational studies, MR is less susceptible to confounding and reverse causality (Smith et al., Reference Smith, Timpson and Ebrahim2008; Wehby et al., Reference Wehby, Ohsfeldt and Murray2008).
Details are in online Supplementary Text S1. Briefly, we performed two-sample MR with GWAS summary statistics using the package ‘TwoSampleMR’ (Hemani et al., Reference Hemani, Zheng, Elsworth, Wade, Haberland, Baird, Laurin, Burgess, Bowden, Langdon, Tan, Yarmolinsky, Shihab, Timpson, Evans, Relton, Martin, Davey Smith, Gaunt and Haycock2018). SNPs passing genome-wide significance (p < 5 × 10−8) were selected as instruments. We conducted MR with four approaches, namely inverse-variance weighted (IVW) (Ehret et al., Reference Ehret, Munroe, Rice, Bochud, Johnson, Chasman, Smith, Tobin, Verwoert, Hwang, Pihur, Vollenweider, O'Reilly, Amin, Bragg-Gresham, Teumer, Glazer, Launer, Zhao, Aulchenko, Heath, Sober, Parsa, Luan, Arora, Dehghan, Zhang, Lucas, Hicks, Jackson, Peden, Tanaka, Wild, Rudan, Igl, Milaneschi, Parker, Fava, Chambers, Fox, Kumari, Go, van der Harst, Kao, Sjogren, Vinay, Alexander, Tabara, Shaw-Hawkins, Whincup, Liu, Shi, Kuusisto, Tayo, Seielstad, Sim, Nguyen, Lehtimaki, Matullo, Wu, Gaunt, Onland-Moret, Cooper, Platou, Org, Hardy, Dahgam, Palmen, Vitart, Braund, Kuznetsova, Uiterwaal, Adeyemo, Palmas, Campbell, Ludwig, Tomaszewski, Tzoulaki, Palmer, Aspelund, Garcia, Chang, O'Connell, Steinle, Grobbee, Arking, Kardia, Morrison, Hernandez, Najjar, McArdle, Hadley, Brown, Connell, Hingorani, Day, Lawlor, Beilby, Lawrence, Clarke, Hopewell, Ongen, Dreisbach, Li, Young, Bis, Kahonen, Viikari, Adair, Lee, Chen, Olden, Pattaro, Bolton, Kottgen, Bergmann, Mooser, Chaturvedi, Frayling, Islam, Jafar, Erdmann, Kulkarni, Bornstein, Grassler, Groop, Voight, Kettunen, Howard, Taylor, Guarrera, Ricceri, Emilsson, Plump, Barroso, Khaw, Weder, Hunt, Sun, Bergman, Collins, Bonnycastle, Scott, Stringham, Peltonen, Perola, Vartiainen, Brand, Staessen, Wang, Burton, Soler Artigas, Dong, Snieder, Wang, Zhu, Lohman, Rudock, Heckbert, Smith, Wiggins, Doumatey, Shriner, Veldre, Viigimaa, Kinra, Prabhakaran, Tripathy, Langefeld, Rosengren, Thelle, Corsi, Singleton, Forrester, Hilton, McKenzie, Salako, Iwai, Kita, Ogihara, Ohkubo, Okamura, Ueshima, Umemura, Eyheramendy, Meitinger, Wichmann, Cho, Kim, Lee, Scott, Sehmi, Zhang, Hedblad, Nilsson, Smith, Wong, Narisu, Stancakova, Raffel, Yao, Kathiresan, O'Donnell, Schwartz, Ikram, Longstreth, Mosley, Seshadri, Shrine, Wain, Morken, Swift, Laitinen, Prokopenko, Zitting, Cooper, Humphries, Danesh, Rasheed, Goel, Hamsten, Watkins, Bakker, van Gilst, Janipalli, Mani, Yajnik, Hofman, Mattace-Raso, Oostra, Demirkan, Isaacs, Rivadeneira, Lakatta, Orru, Scuteri, Ala-Korpela, Kangas, Lyytikainen, Soininen, Tukiainen, Wurtz, Ong, Dorr, Kroemer, Volker, Volzke, Galan, Hercberg, Lathrop, Zelenika, Deloukas, Mangino, Spector, Zhai, Meschia, Nalls, Sharma, Terzic, Kumar, Denniff, Zukowska-Szczechowska, Wagenknecht, Fowkes, Charchar, Schwarz, Hayward, Guo, Rotimi, Bots, Brand, Samani, Polasek, Talmud, Nyberg, Kuh, Laan, Hveem, Palmer, van der Schouw, Casas, Mohlke, Vineis, Raitakari, Ganesh, Wong, Tai, Cooper, Laakso, Rao, Harris, Morris, Dominiczak, Kivimaki, Marmot, Miki, Saleheen, Chandak, Coresh, Navis, Salomaa, Han, Zhu, Kooner, Melander, Ridker, Bandinelli, Gyllensten, Wright, Wilson, Ferrucci, Farrall, Tuomilehto, Pramstaller, Elosua, Soranzo, Sijbrands, Altshuler, Loos, Shuldiner, Gieger, Meneton, Uitterlinden, Wareham, Gudnason, Rotter, Rettig, Uda, Strachan, Witteman, Hartikainen, Beckmann, Boerwinkle, Vasan, Boehnke, Larson, Jarvelin, Psaty, Abecasis, Chakravarti, Elliott, van Duijn, Newton-Cheh, Levy, Caulfield and Johnson2011; Burgess et al., Reference Burgess, Butterworth and Thompson2013), MR Egger (Bowden et al., Reference Bowden, Davey Smith and Burgess2015), weighted median and weighted mode methods (Bowden et al., Reference Bowden, Davey Smith, Haycock and Burgess2016; Hartwig et al., Reference Hartwig, Davey Smith and Bowden2017). The latter three methods were employed to examine whether the results are robust in the presence of horizontal pleiotropy (i.e. the instrument genetic variant(s) affect the outcome via pathways other than through the exposure).
In the main text, we primarily reported the IVW estimates, however, if Egger's method revealed significant imbalanced pleiotropy, MR Egger result was presented (this method can give unbiased estimates in the presence of imbalanced pleiotropy). Note that in PRS analysis horizontal pleiotropy is allowed, but it will affect the validity of causal inference in MR. PRS studies can elucidate shared genetic bases but are not designed for causal inference. For easy interpretation, units of exposures/outcomes are included in online Supplementary Table S15.
While there is overlap between the subjects used in GWAS of CM traits and the control subjects of SCZ/BD GWAS, the chance of false positive associations (in PRS or MR analysis) due to sample overlap is likely low according to Burgess et al. (Reference Burgess, Davies and Thompson2016), as detailed in online Supplementary Text S1.
Discovering shared SNPs and pathway
For each pair of diseases (psychiatric v. metabolic), we computed for every SNP the probability of being associated with both diseases (known as tdr 11). We also selected SNPs with tdr 11 ⩾ 0.5 and mapped them to genes, and inferred the pathways involved (see online Supplementary Text S1).
Results
SCZ and CM traits
Results from PRS and LDSR analysis
The associations of SCZ with CM traits using paired summary statistics are shown in Table 1 and online Supplementary Table S12. Intuitively, PRS from CM traits can be regarded as a proxy of the actual level of the trait (Evans et al., Reference Evans, Brion, Paternoster, Kemp, McMahon, Munafo, Whitfield, Medland, Montgomery, Consortium, Consortium, Consortium, Timpson, St Pourcain, Lawlor, Martin, Dehghan, Hirschhorn and Smith2013). For binary traits, the polygenic scores may be regarded as the underlying liability to the corresponding disorder.
Table 1. Polygenic association testing of SCZ with CM traits using summary statistics

SCZ was treated as the target phenotype (i.e. dependent variable) on the left block and treated as the base phenotype (i.e. as predictor variable) in the middle block. Best p, best p value threshold; coef, regression coefficient; rg, genetic correlation.
BMI, body mass index; CAD, coronary artery disease; DM, type 2 diabetes mellitus (sample mainly from Caucasians); DM-2nd set, type 2 diabetes (sample from Japanese); FG, fasting glucose; FG.adjBMI, fasting glucose adjusted for BMI; INS, fasting insulin; INS.adjBMI, fasting insulin adjusted for BMI; HDL, high-density lipoprotein; LDL, low-density lipoprotein; TG, triglycerides; TC, total cholesterol; WHR, waist-to-hip ratio; WHR.adjBMI, WHR adjusted for BMI; SBP and DBP, systolic blood pressure and diastolic blood pressure; SAT, subcutaneous adipose tissue volume; VAT, visceral adipose tissue volume; PAT, pericardial fat volume; SATHU, subcutaneous adipose tissue attenuation; VATHU, visceral adipose tissue attenuation; adjHtWt, adjusted for height and weight.
Results with FDR <0.05 are in bold while suggestive associations (0.05 ⩽ FDR ⩽ 0.1) are italics. PRS analysis was not conducted for CAD and DM due to significant sample overlap. LDSR was not performed for SBP and DBP as these are exome-based studies.
Here, we briefly describe findings with at least suggestive evidence (FDR<0.1). The strongest association was observed for BMI (lowest p = 1.67 × 10−11) in PRS analysis when SCZ was used either as the predictor or target phenotype. Interestingly, the coefficient was negative, signifying an inverse association. For lipid traits, we observed a positive polygenic association of SCZ with HDL, but no significant associations with LDL and TG. For adipokines, we observed positive associations with leptin (BMI-adjusted) and an inverse association with adipokine when SCZ PRS was treated as exposure. Both raised leptin and reduced adiponectin are associated with heightened CM risks (Han et al., Reference Han, Quon, Kim and Koh2007; Koh et al., Reference Koh, Park and Quon2008). As for measures of central obesity and fat deposition, positive association with WHR, BMI-adjusted WHR and BMI-adjusted visceral adiposity were observed. Finally, PRS of FG and fasting insulin were positively associated with SCZ; we also found a positive polygenic link of SCZ PRS with DM.
LDSR revealed a significant genetic correlation of lower BMI with SCZ, although there were no other significant results. The intercepts from cross-trait LDSR (reflecting sample overlap) were largely non-significant. While adiponectin showed p < 0.05, we expect (0.05*26)~one ‘false-positive’ at α = 0.05.
An earlier study (Bulik-Sullivan et al., Reference Bulik-Sullivan, Finucane, Anttila, Gusev, Day, Loh, ReproGen, Duncan, Perry, Patterson, Robinson, Daly, Price and Neale2015) conducted LDSR on SCZ with 15 of the 28 CM traits included here. While we have repeated the analyses, the results are generally similar and the conclusions are the same. The same trait (BMI) achieved significant (p < 0.05) correlation with SCZ. The results from Bulik-Sullivan et al. (Reference Bulik-Sullivan, Finucane, Anttila, Gusev, Day, Loh, ReproGen, Duncan, Perry, Patterson, Robinson, Daly, Price and Neale2015) are included in online Supplementary Table S13 for reference (some differences may be due to, e.g. different versions of the code).
For analyses of the NFBC, the most significant association was WHR adjusted for BMI (FDR = 0.055; online Supplementary Table S1). We were unable to replicate other significant results from analyses using summary statistics, probably owing to a much smaller sample size of NFBC compared with GWAS meta-analyses.
Results from MR
In the MR analysis (Table 2 and online Supplementary Table S14), when SCZ was considered as exposure, the most significant result was observed for TG. There was evidence of imbalanced horizontal pleiotropy, but no evidence of heterogeneity under MR Egger. The Egger's method suggested that SCZ may be causally related to an increase in TG, while the weighted median approach showed a trend towards significance (p = 0.077). We observed a nominally significant association with pericardial fat volume by IVW approach, which was stronger after adjusting for BMI (FDR = 0.288). We did not observe a significant causal relationship with other CM traits.
When SCZ was regarded as the outcome, there was no evidence of causal relationships for any CM trait, except that insulin was weakly significant (p = 0.042).
The full results of MR analysis are presented in online Supplementary Table S14. It includes results from IVW, weighted median, weighted mode and MR Egger. Other details, including F-statistic (reflecting instrument strength), I 2 for MR Egger, SIMEX-corrected Egger regression, SIMEX-corrected pleiotropy test and heterogeneity test results are also presented.
Table 2. MR analysis of SCZ with CM traits

b: coefficient estimate from MR; se, standard error; pval, p-value; FDR, false discovery rate.
a MR results for TG was taken from Egger's method as there was significant (unbalanced) horizontal pleiotropy (p = 0.001). Several traits do not have SNPs passing genome-wide significance, which also overlap with the SCZ GWAS SNPs, and hence are excluded here. Due to sample overlap between CAD and DM (Caucasian sample) with SCZ/BD, these traits are not included in the MR analysis here. Results with FDR <0.05 are in bold. Results with 0.05 <FDR <0.1 are in italics.
Results from shared SNP/pathway analysis
The full results of the shared SNPs analyses with tdr 11 > 0.5 are presented in online Supplementary Tables S5 and S7. The top three genes shared between SCZ/BD and each CM trait are shown in online Supplementary Table S2. Across all CM traits, we discovered 15 422 and 2890 shared genetic loci with tdr 11 > 0.5 and >0.8, respectively (clumping at R 2 = 0.1 with 250-kb windows; online Supplementary Table S11). Selected top pathways are presented in Table 3, taken from the top 25 most commonly top-ranked pathways across all traits (with reduced repetitions of similar pathways). Full results are in online Supplementary Table S9. If the direction of effect is not considered (Table 3), the most frequently listed pathway was ‘neuronal system’; other pathways included insulin secretion, VEGF signaling, aldosterone synthesis and secretion, statin pathway, epidermal growth factor receptor (EGFR) signaling, lipoprotein metabolism etc. We also presented pathways derived from SNPs having the same direction of associations with CM disorders, given the polygenic association of SCZ with worse CM risks with regard to several metabolic parameters. In this case, the most often top-ranked pathway was antigen processing and presentation (see online Supplementary Table S9).
Table 3. Selected top pathways derived from SNPs shared between SCZ/BD and CM traits
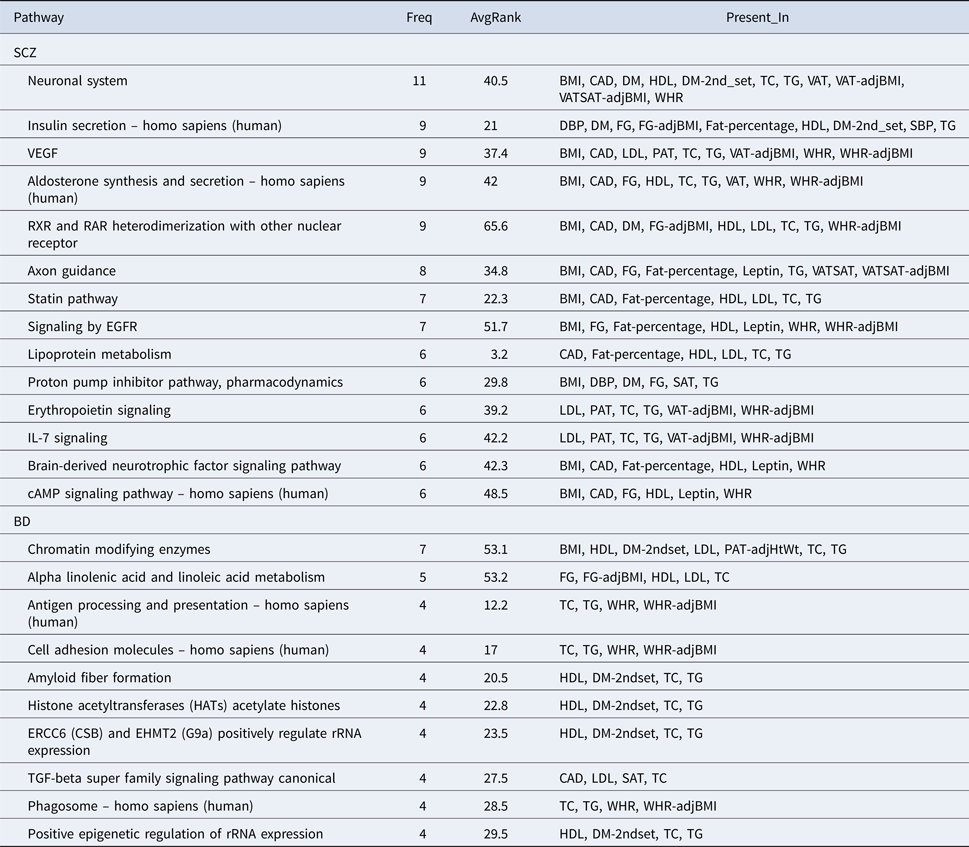
Freq, frequency of being top listed across all CM traits; AvgRank, average rank of pathway if top listed.
BD and CM traits
Results from polygenic score analysis and LDSR
Table 4 shows the polygenic associations of BD with CM traits using paired summary statistics. Similar to SCZ, we observed an inverse association with BMI when BD was treated as the exposure or outcome. We also observed a polygenic association of lower WHR with BD, but the result became non-significant after adjustment by BMI. For lipid traits, we observed polygenic associations of BD with higher HDL, as well as lower LDL, TC and TG. We also detected polygenic associations with lower leptin levels and SBP. All the above results have FDR <0.05.
Table 4. Polygenic association testing of BD with CM traits using summary statistics

BD was treated as the target phenotype (i.e. dependent variable) on the left block and treated as the base phenotype (i.e. as predictor variable) in the middle block. Best p, best p value threshold; coef, regression coefficient. Please refer to the legends of Table 1 for other abbreviations. PRS analysis was not conducted for CAD and DM due to significant sample overlap. LDSR was not performed for SBP and DBP as these are exome-based studies.
In LDSR, no genetic correlations achieved FDR<0.1, although several traits were nominally significant with FDR <0.2. These included BMI and WHR, which showed negative genetic correlations with BD, consistent with PRS analysis. FG and insulin showed nominally significant negative genetic correlations with BD. Interestingly, we observed a marginally significant positive correlation with DM. The overall direction of genetic correlations from LD score analysis leaned towards lower CM risks, broadly in line with PRS analyses. The LDSR intercepts were non-significant apart from CAD and DM.
Bulik-Sullivan et al. (Reference Bulik-Sullivan, Finucane, Anttila, Gusev, Day, Loh, ReproGen, Duncan, Perry, Patterson, Robinson, Daly, Price and Neale2015) have conducted LDSR on BD and 15 of the CM traits. However, our analysis is new. It is based on an expanded GWAS meta-analysis (N = 40 255) (Hou et al., Reference Hou, Bergen, Akula, Song, Hultman, Landen, Adli, Alda, Ardau, Arias, Aubry, Backlund, Badner, Barrett, Bauer, Baune, Bellivier, Benabarre, Bengesser, Berrettini, Bhattacharjee, Biernacka, Birner, Bloss, Brichant-Petitjean, Bui, Byerley, Cervantes, Chillotti, Cichon, Colom, Coryell, Craig, Cruceanu, Czerski, Davis, Dayer, Degenhardt, Del Zompo, DePaulo, Edenberg, Etain, Falkai, Foroud, Forstner, Frisen, Frye, Fullerton, Gard, Garnham, Gershon, Goes, Greenwood, Grigoroiu-Serbanescu, Hauser, Heilbronner, Heilmann-Heimbach, Herms, Hipolito, Hitturlingappa, Hoffmann, Hofmann, Jamain, Jimenez, Kahn, Kassem, Kelsoe, Kittel-Schneider, Kliwicki, Koller, Konig, Lackner, Laje, Lang, Lavebratt, Lawson, Leboyer, Leckband, Liu, Maaser, Mahon, Maier, Maj, Manchia, Martinsson, McCarthy, McElroy, McInnis, McKinney, Mitchell, Mitjans, Mondimore, Monteleone, Muhleisen, Nievergelt, Nothen, Novak, Nurnberger, Nwulia, Osby, Pfennig, Potash, Propping, Reif, Reininghaus, Rice, Rietschel, Rouleau, Rybakowski, Schalling, Scheftner, Schofield, Schork, Schulze, Schumacher, Schweizer, Severino, Shekhtman, Shilling, Simhandl, Slaney, Smith, Squassina, Stamm, Stopkova, Streit, Strohmaier, Szelinger, Tighe, Tortorella, Turecki, Vieta, Volkert, Witt, Wright, Zandi, Zhang, Zollner and McMahon2016) instead of the PGC study in 2011 (N = 16 731) (Psychiatric GWAS Consortium Bipolar Disorder Working Group, 2011). In the PGC-2011 analysis, no CM traits achieved nominal significance, while the present analysis revealed several traits with p < 0.05, possibly due to larger sample size.
As for the analyses of NFBC, we did not observe any results passing FDR correction (see online Supplementary Table S1). Nevertheless, for a few traits showing at least p < 0.05 at the optimal p value threshold (including INS, TG and SBP), the directions of associations were negative, largely in line with the PRS analysis and LDSR.
Results from MR
In an MR analysis (Table 5 and online Supplementary Table S14), when BD was treated as the exposure, there was no evidence of a causal relationship with CM traits. On the other hand, when CM traits were considered as exposures, we observed a few nominally significant results, but only BMI and FG (including BMI-adjusted FG) passed FDR threshold at 0.1; no results had FDR <0.05. Lower FG appeared to be causally related to BD (IVW: beta = −4.474, p = 0.0097, FDR = 0.067). Results for SBP and WHR (BMI-adjusted) were only nominally significant. We did not observe evidence of heterogeneity or imbalanced horizontal pleiotropy for the above results.
Table 5. MR analysis of BD with CM traits

Results with FDR <0.05 are in bold. Results with 0.05 < FDR < 0.1 are in italics.
a MR results taken from Egger's method as (unbalanced) horizontal pleiotropy was significant.
b Significant heterogeneity (p = 0.0217).
For BMI, there was evidence of both imbalanced pleiotropy and heterogeneity (under IVW and Egger). This may reflect the genetic instruments were measuring different quantities, casting doubt on the assumption of MR being valid for all genetic variants. MR Egger suggested a nominally significant causal effect, however, it was not supported by the weighted median or weighted mode approaches.
Results from shared SNP/pathway analysis
Full results of shared SNPs analyses are presented in online Supplementary Tables S6 and S8. Across all CM traits, we found totally 3115 and 297 shared genetic loci with tdr 11 > 0.5 and >0.8, respectively (see online Supplementary Table S11). Several top pathways (Table 3; see online Supplementary Table S10) appeared to be related to epigenetic regulation, such as ‘chromatin modifying enzymes’, ‘HATSs acetylate histones’ and ‘Positive epigenetic regulation of rRNA expression’. Some other pathways are related to immune system functioning, such as ‘Antigen processing and presentation’, ‘Phagosome’ and ‘TGF-beta super family signaling’ (Chen and Ten Dijke, Reference Chen and Ten Dijke2016).
Discussion
In this study, we have performed a variety of analyses to reveal the possible shared genetic basis of SCZ and BD with CM traits. Potential polygenic associations are discussed below.
SCZ and CM traits
Raised cardiovascular morbidity and mortality are well established in SCZ, yet it is difficult to disentangle the numerous possible underlying factors, including the side effects of antipsychotics. Despite increased cardiovascular risks in SCZ patients, surprisingly, we found evidence for an inverse relationship between PRS of BMI and SCZ. The association was consistent in both PRS and LDSR analyses. Interestingly, two large-scale studies reported subjects with lower BMI had an increased risk of SCZ (Sorensen et al., Reference Sorensen, Mortensen, Reinisch and Mednick2006; Zammit et al., Reference Zammit, Rasmussen, Farahmand, Gunnell, Lewis, Tynelius and Brobert2007). A few studies in drug-naïve patients also revealed a trend of lower BMI (Spelman et al., Reference Spelman, Walsh, Sharifi, Collins and Thakore2007; Padmavati et al., Reference Padmavati, McCreadie and Tirupati2010), although some did not (Ryan et al., Reference Ryan, Collins and Thakore2003; Venkatasubramanian et al., Reference Venkatasubramanian, Chittiprol, Neelakantachar, Naveen, Thirthall, Gangadhar and Shetty2007). The underlying mechanism is unknown, but one hypothesis is that poor nutritional status, however subtle, may adversely affect neural development (Zammit et al., Reference Zammit, Rasmussen, Farahmand, Gunnell, Lewis, Tynelius and Brobert2007) which leads to psychotic disorders. We noted that Bulik-Sullivan et al. (Reference Bulik-Sullivan, Finucane, Anttila, Gusev, Day, Loh, ReproGen, Duncan, Perry, Patterson, Robinson, Daly, Price and Neale2015) have reported a negative genetic correlation between BMI and SCZ, but here we provided further support with a different analytic approach (PRS), and suggested that the association is not causal but more likely attributable to shared genetic liability.
For other associations discussed below, we observed polygenic associations at FDR <0.05 (i.e. expected proportion of FP is ~5%), but did not find concordant evidence from LDSR. Also in view of other limitations of the current study (see discussions below), the findings may be considered more tentative, and further studies are required to confirm the results. Nevertheless, we believe our findings still provide a useful guide for future investigations, and they are broadly consistent with previous epidemiology studies.
In this study, we observed tentative polygenic associations of SCZ with glucose abnormalities, adverse adipokine profiles, central obesity and increased visceral adiposity. Notably, multiple studies have found impaired glucose tolerance or insulin resistance in drug-naïve SCZ patients and their relatives, e.g. (Pillinger et al., Reference Pillinger, Beck, Gobjila, Donocik, Jauhar and Howes2017a). Despite the negative genetic correlation between BMI and SCZ, an opposite trend for WHR was observed. Several clinical studies, e.g. (Ryan et al., Reference Ryan, Flanagan, Kinsella, Keeling and Thakore2004; Sengupta et al., Reference Sengupta, Parrilla-Escobar, Klink, Fathalli, Ying Kin, Stip, Baptista, Malla and Joober2008) also reported increased WHR in drug-free patients. WHR might predict CM risks independent of BMI (Yusuf et al., Reference Yusuf, Hawken, Ounpuu, Bautista, Franzosi, Commerford, Lang, Rumboldt, Onen, Lisheng, Tanomsup, Wangai, Razak, Sharma and Anand2005). Raised leptin and reduced adiponectin have also been reported in clinical studies of SCZ patients (Bartoli et al., Reference Bartoli, Lax, Crocamo, Clerici and Carra2015; Stubbs et al., Reference Stubbs, Wang, Vancampfort and Miller2016) (online Supplementary Text S1).
As for lipid traits, our results were generally consistent with a recent meta-analysis (Pillinger et al., Reference Pillinger, Beck, Stubbs and Howes2017b) on lipid profiles, which reported higher TG but favorable cholesterol profiles among first-episode psychosis patients, although they did not reveal changes in HDL observed in this study.
In general, we did not find evidence for a causal relationship between SCZ and CM traits, with the exception of TG. This suggests that SCZ per se may not directly cause changes in CM traits; the associations of SCZ with CM abnormalities are more likely due to shared genetic liability or environmental risk factors. In other words, similar risk factors may influence the risks of both SCZ and CM disorders together, but through different pathways.
BD and CM traits
For BD, we also found potential polygenic associations (at FDR <5%) with several CM traits. However, for many traits, we did not observe concordant results using LDSR, except for BMI and WHR. Also, as the relationships between BD and CM traits are less well-studied than SCZ, the supporting clinical evidence is generally weaker. On top of other limitations of this study, we caution that the results should be considered tentative rather than confirmatory, but we believe they are still valuable in adding knowledge to this under-researched area.
Similar to SCZ, we also observed an inverse genetic relationship between BMI and BD. One previous clinical study reported an increased prevalence of overweight in BD (Maina et al., Reference Maina, Salvi, Vitalucci, D'Ambrosio and Bogetto2008), but it has several limitations (see online Supplementary Text S1). We observed an inverse genetic relationship between BD and LDL, TC and TG. This result could be confounded by BMI, although studies have found a lower cholesterol level in bipolar patients (Gabriel, Reference Gabriel2007; Sagud et al., Reference Sagud, Mihaljevic-Peles, Pivac, Jakovljevic and Muck-Seler2007). There is also evidence of a correlation between low cholesterol levels and suicide risks (Lester, Reference Lester2002). Overall, we observed tentative polygenic associations of BD with favorable metabolic parameters, suggesting that other secondary risk factors (e.g. medications) may play a larger part than the disorder itself in affecting cardiovascular risks (see online Supplementary Text S1).
As for MR analysis, again the results were largely negative. When BD was considered as the outcome, lower FG appeared to be causally linked to BD. The underlying mechanisms remain elusive, but interestingly a recent study reported greater mood lability being associated with lower fasting glucose levels (Gupta et al., Reference Gupta, Anderson and Holt2016). However, yet another small-scale study revealed more glucose abnormalities in drug-naive bipolar patients (Guha et al., Reference Guha, Bhowmick, Mazumder, Ghosal, Chakraborty and Burman2014). Further studies are required to elucidate the exact relationship.
Clinical implications
We highlight the potential clinical relevance here, but we caution that the link between the disorders requires further validation in clinical studies.
Our findings suggest that increased awareness and better monitoring of CM risks in psychosis patients may be warranted, especially for SCZ. Taken together, we found tentative polygenic associations with adverse glucose and adipokine profiles, central obesity and increased visceral adiposity in SCZ. These findings are grossly consistent with epidemiological studies. If our findings are validated, this would suggest proper management of CM risk factors may be required from the disease onset, and even in patients taking drugs with less metabolic side effects. Also, the measurement of WHR (instead of BMI alone) may be useful in screening for metabolic risks, due to its close association with visceral adiposity. Moreover, adipokines such as leptin and adiponectin may warrant further investigations as biomarkers for CV risks in SCZ.
On the other hand, there was preliminary evidence of associations with favorable cholesterol profiles in SCZ and BD, and an overall favorable CM profile in BD patients. These results suggest that hypercholesterolemia in SCZ and many CM abnormalities in BD may be secondary (and hence more readily modifiable) instead of being related to the underlying pathophysiology of SCZ/BD.
Shared pathways of SCZ/BD with CM traits
We highlight a few pathways here (see Supplementary Text S1 for further discussions). For example, it is interesting to note that pathways associated with immune functioning (e.g. antigen processing, complement pathways) were ranked highly among a number of CM traits. Notably, immune system dysfunction has been implicated in SCZ and BD (Mondelli et al., Reference Mondelli, Dazzan and Pariante2015) as well as CM abnormalities (Fernandez-Ruiz, Reference Fernandez-Ruiz2016). Recent studies have suggested that chronic inflammation may be an important mediator linking metabolic abnormalities and severe mental illnesses (Henderson et al., Reference Henderson, Vincenzi, Andrea, Ulloa and Copeland2015). For example, elevations of pro-inflammatory cytokines such as tumor necrosis factor-alpha and interleukin-6 have been observed both in patients with psychosis and MetS. Chronic systemic inflammation may be coupled with microglia activation in the brain, which may disturb neuronal functions (Henderson et al., Reference Henderson, Vincenzi, Andrea, Ulloa and Copeland2015).
Lipid metabolism and the statin pathway were ranked among the top. Statins lower the risk of CAD (Taylor et al., Reference Taylor, Huffman, Macedo, Moore, Burke, Davey Smith, Ward and Ebrahim2013), yet it has also been investigated as an adjunctive therapy for SCZ due to its anti-inflammatory properties (Vincenzi et al., Reference Vincenzi, Stock, Borba, Cleary, Oppenheim, Petruzzi, Fan, Copeland, Freudenreich, Cather and Henderson2014). Another top-listed pathway was EGFR-1 signaling pathway, which was implicated not only in cardiovascular abnormalities (Makki et al., Reference Makki, Thiel and Miller2013), but also brain functioning and pathology of SCZ (Iwakura and Nawa, Reference Iwakura and Nawa2013).
Study limitations
There are a few limitations to our study. Most studies were performed in Caucasians, and it is worthwhile to extend to other ethnic groups. For analyses using paired summary results, we were unable to control for other clinical factors, for instance adjusting for WHR/BMI when studying lipid traits. Further clinical studies of metabolic abnormalities in SCZ and bipolar patients (ideally longitudinal ones), coupled with genetic testing, will be useful in providing more solid evidence of shared genetic susceptibilities. As alluded to earlier, LDSR and PRS each have its strengths and limitations (see online Supplementary Text S1). In addition, both methods, as well as MR, assume linearity of SNP effects. Also, SCZ and BD are likely to be heterogeneous disorders, and the association with CM abnormalities may differ within different patient subgroups.
While we have discussed a few shared pathways, further experimental studies are required to elucidate the exact mechanisms involved. Interestingly, we did not find uniformly increased polygenic risks of all metabolic abnormalities in SCZ and BD. Further investigations into the complex links between CM risk factors and SCZ and BD might shed light on new therapeutic measures for both types of disorders.
Supplementary material
The supplementary material for this article can be found at https://doi.org/10.1017/S0033291718001812 and at https://drive.google.com/open?id=1ISsvv90qXzCBFodekfGonTxuvU9h-9ZQ.
Acknowledgements
We would like to thank Prof. Stephen Tsui and the Hong Kong Bioinformatics Centre for bioinformatics support. Author contributions: Conceived and designed the study: HCS. Performed core data analyses: HCS and CKLC. Provided advice on computational/statistical analyses: PCS. Performed pathway analysis: F-K.A, C-H.M. Drafted the manuscript: HCS. Supervised the study: HCS.
Financial support
This study was partially supported by the Lo Kwee Seong Biomedical Research Fund and a CUHK Direct Grant (4054320) to H-.C.S.
Conflict of interest
The authors declare no conflicts of interest.