Introduction
There is a robust and well-documented relationship between birth weight (BW) and a higher risk of cardiometabolic diseases like type 2 diabetes (T2D) and hypertension in later life.Reference Barker1–Reference Hales and Barker3 The Barker hypothesis,Footnote 1 which posits that adverse intrauterine environments result in lower BW and increased future risk of cardiometabolic disease through developmental compensations, may explain this observed relationship.Reference Barker1 Evidence in favor of this theory has primarily come from experimental studies on animals,Reference Dickinson, Moss and Gatford4 which may not generalize to humans, and observational epidemiological studies,Reference Suzuki5 which are susceptible to confounding, bias, and reverse causalityReference Gage, Munafò and Davey Smith6. However, because randomized controlled trials (RCTs) cannot be performed easily in this context, definitive proof of the hypothesis in humans has been lacking.
Mendelian randomization (MR) is an epidemiological method that uses genetic variants robustly associated with a modifiable environmental exposure to estimate the causal relationship between the exposure and a medically relevant outcome of interest.Reference Davey Smith and Ebrahim7 Mendel’s Law of Segregation ensures that genetic variants segregate randomly and independently of environmental factors, while Mendel’s Law of Independent Assortment suggests that the genetic variants should also segregate independently of other traits provided certain conditions are metReference Davey Smith and Ebrahim7. This means that genetic variants are less susceptible to reverse causality and confounding than the “traditional” variables used in observational studies. In other words, genetic variants can be used to classify a study sample into subgroups, which differ systematically with respect to the exposure of interest, but not with respect to confounding factors (i.e. similar to an RCT). If groups defined by their genotypes also show differences in the outcome of interest, then, provided core assumptions are met, this provides evidence of a causal relationship (Fig. 1a).

Fig. 1. (a) Mendelian randomization (MR) studies share many similarities with randomized controlled trials (RCTs) and comparing the two study designs can be useful in understanding the MR method. MR uses genetic markers that are robustly associated with an exposure of interest. Mendel’s Laws of Segregation and Independent Assortment ensure that alleles are randomly transmitted from parents to their offspring independently of known and unknown confounders (with certain exceptions – see Davey Smith and Ebrahim 2003)Reference Davey Smith and Ebrahim7 – analogous to the physical randomization that occurs in RCTsReference Davey Smith and Ebrahim7. Provided that certain core assumptions are met (see below), any differences in outcome between groups defined by their genotypes should therefore reflect the causal effect of the exposure on the outcome, and this causal effect can be estimated using an appropriate statistical methodology (b) Directed acyclic graph illustrating the three core assumptions underlying MR analysis. The first assumption is that genetic variants used in the analysis should be robustly associated with the exposure of interest. The second assumption is that the genetic variants should not be associated with any confounders of the exposure–outcome relationship. The third assumption is that the genetic variants should only be related to the outcome through the exposure of interest (this is commonly known as the “no horizontal pleiotropy” assumption). (c) Diagrammatic representation of how MR principles can be used to investigate the Barker hypothesis. According to the Barker hypothesis, an adverse intrauterine environment subsequently results in decreased birth weight (BW) and increased risk of cardiometabolic disease in later life. Notably, there is no causal effect of BW on the risk of cardiometabolic disease outcomes. Maternal genotype at genetic markers (in this case, a single-nucleotide polymorphism (SNP)) can be used to proxy intrauterine environmental exposures that affect offspring BW. These maternal genetic markers can then be tested for association with offspring cardiometabolic outcomes, after conditioning on offspring genetic markers at the same genetic loci. By conditioning on offspring genotype (indicated by the box) at the same loci, a potential path to offspring BW and cardiometabolic outcomes through the offspring’s genome is blocked (dotted lines). Importantly, this same paradigm can be used to investigate hypotheses relating to the Developmental Origins of Health and Disease hypothesis more broadly by using maternal SNPs related to specific maternal environmental exposures during pregnancy and examining their association with offspring cardiometabolic disease conditional on offspring genotype.
Recently, several studies have attempted to use the technique of MR to investigate the relationship between BW and cardiometabolic disease and in some cases explicitly inform on the validity of the Barker hypothesis.Reference Barker1,Reference Zanetti, Tikkanen, Gustafsson, Priest, Burgess and Birthweight8–Reference Wang, Huang and Li10 For example, Zanetti et al. used two-sample MR to examine the relationship between BW and a variety of outcomes in the UK Biobank. The authors found evidence for an inverse correlation between BW-associated single-nucleotide polymorphisms (SNPs) and low-density lipoprotein cholesterol, 2 h glucose, coronary artery disease, and T2D, and a positive correlation between BW-associated SNPs and body mass index. The authors interpreted their findings as evidence that lower BW was causally associated with increased susceptibility to coronary artery disease and T2D.
While MR has a number of potential advantages over traditional observational epidemiological studies, we believe that previous studies that have used MR in an attempt to investigate the Barker hypothesisReference Zanetti, Tikkanen, Gustafsson, Priest, Burgess and Birthweight8–Reference Wang, Huang and Li10 contain several flaws that render them unsuitable for valid inference in this context. First, previous MR studies have used genetic variants in the fetal genome that are associated with their own BW as instrumental variables. We believe this framework is problematic because the Barker hypothesis postulates that an adverse intrauterine environment leads to low BW and increased risk of future cardiometabolic disease.Reference Godfrey and Barker11 This is different from postulating that BW itself has a direct causal effect on cardiometabolic disease (Fig. 1c), as did Huang et al. and Zanetti et al. Reference Zanetti, Tikkanen, Gustafsson, Priest, Burgess and Birthweight8,Reference Huang, Wang and Zheng9 We would argue that this underlying model is inappropriate because SNPs in the fetal genome are likely to be associated with BW through many different processes, and therefore do not necessarily proxy the intrauterine environment. In other words, these studies violate a core assumption of MR analyses, that the SNPs used in the analysis are associated with the environmental exposure of interest (in this case, the intrauterine environment – see the first assumption in Fig. 1b).
Second, due to the transmission of alleles from mother to offspring, offspring, and maternal genotypes are correlated (r ≈ 0.5). Consequently, any association between offspring genotype and offspring outcomes, when no adjustment has been made for maternal genotype, could actually reflect an effect of maternal genotype on offspring outcome, complicating interpretation of the analysis.Reference Warrington, Freathy, Neale and Evans12 In other words, these studies have violated another core assumption of MR analyses that the SNPs used in the analysis are not associated with potential confounders of the exposure–outcome relationship (see the second assumption in Fig. 1b). In this case, the maternal genotype may confound the analysis as it may be related to both offspring BW and also potentially offspring cardiometabolic risk (through the intrauterine environment).
Finally, many of the genetic variants robustly associated with BW are known to exert pleiotropic effects on cardiometabolic phenotypes.Reference Warrington, Freathy, Neale and Evans12,Reference Warrington, Beaumont and Horikoshi13 This means that the SNPs used in the analyses may violate the “no horizontal pleiotropy assumption” underlying MR – the assumption that SNPs that show a relationship with the outcome of interest (cardiometabolic disease), only do so through the exposure under study, and not via any other biological pathways (see the third assumption in Fig. 1b).Reference Didelez and Sheehan14 Additionally, variants most strongly related to BW are also likely to have the strongest pleiotropic associations with cardiometabolic phenotypes. This violates an assumption underlying MR-Egger regression, a special type of MR analysis that is thought to be more robust to horizontal pleiotropy than traditional MR methodsReference Bowden, Davey Smith and Burgess15. Violation of the assumption means that analyses using MR-Egger regression will also likely yield biased estimates of the causal effect.
Our aim was to use simulation and two contrived examples to show that MR using BW-associated SNPs in the offspring genome to examine the Barker hypothesis can provide spurious evidence of a causal effect of BW on future cardiometabolic risk, when no such relationship exists. We also examined if testing whether maternal genetic variants associated with decreased offspring BW were also associated with increased offspring cardiometabolic risk (after conditioning on offspring genotypes at the same loci) was a valid method for testing the validity of the Barker hypothesis (Fig. 1c).Reference Evans, Moen, Hwang, Lawlor and Warrington16
Methods
Simulations
We simulated data where the correlation between offspring BW and future cardiometabolic traits was generated by a combination of genetic pleiotropy (i.e. the genetic variants in the offspring had direct effects on offspring cardiometabolic outcomes not through BW) and maternal and offspring genetic effects on BW. We did not include a direct causal path between maternal genotypes and offspring cardiometabolic outcomes or a direct causal effect of BW on cardiometabolic risk (Fig. 2). We simulate two models, both of which represent plausible explanations for the empirical negative genetic correlation between BW and cardiometabolic phenotypes and have support from large-scale genetic studies.Reference Warrington, Beaumont and Horikoshi13,Reference Evans, Moen, Hwang, Lawlor and Warrington16–Reference Horikoshi, Beaumont and Day18
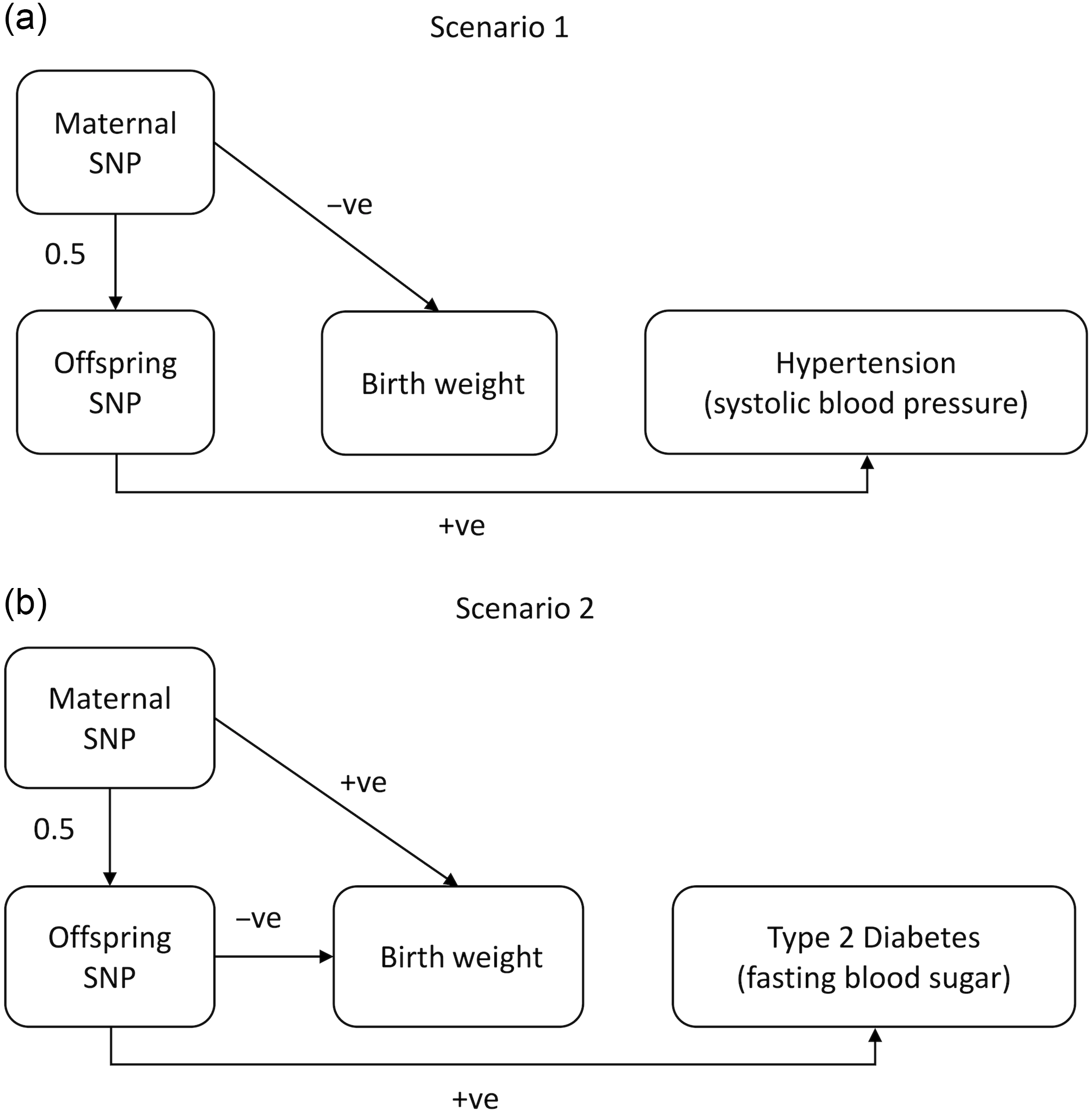
Fig. 2. Diagrams illustrating the two models underlying the relationship between BW and cardiometabolic phenotypes that were simulated in this manuscript. In Scenario 1, maternal SNPs negatively (−ve) affect offspring BW via intrauterine mechanisms. When alleles at these loci are transmitted from mothers to their children, they also exert positive (+ve) pleiotropic effects on hypertension as manifested by increased systolic blood pressure in later life. In Scenario 2, the SNPs used as instrumental variables are associated with increased offspring BW when present in the mother and also exert direct effects on lowering offspring BW through the fetal genome. These genotypes are associated with later life type 2 diabetes (T2D) (measured by fasting blood sugar levels). In both scenarios, the correlation between BW and cardiometabolic phenotypes is due solely to genetic pleiotropy (i.e. not developmental mechanisms).
Scenario 1 represents a possible model for blood pressure-related SNPs. We have previously shown evidence that SNPs that increase maternal systolic blood pressure causally lower offspring BW through intrauterine mechanisms, and those alleles are then transmitted from mother to offspring increasing offspring blood pressure in later life.Reference Warrington, Beaumont and Horikoshi13,Reference Tyrrell, Richmond and Palmer17 Scenario 2 is similar to the model espoused under the Fetal Insulin Hypothesis in which T2D-associated variants in mothers lead to increased maternal glucose levels during pregnancy (promoting increased fetal growth), but may also decrease insulin sensitivity (and fetal growth) when transmitted to offspring, and subsequently increase risk of offspring T2D in later life.Reference Hattersley and Tooke19
Following Fig. 2, we simulated maternal and offspring genotypes, the offspring’s BW (X) and cardiometabolic outcomes (Y), for each family i, using the following equations:


where
$${G_O}__{ij}$$
and
$${G_m}__{ij}$$
refers to the offspring and maternal genotype dosage (0, 1, 2), respectively, for family i at locus j. The offspring and maternal effects of SNP
j
on BW are quantified by
$${\beta _O}__j$$
and
$${\beta _m}__j$$
, respectively, γ
j
reflects the pleiotropic effect of the offspring SNP
j
on the cardiometabolic outcome Y, and ϵ
1
and ε
2
are residual terms affecting offspring BW and cardiometabolic phenotype, respectively, (with covariance ρ). Consistent with the absence of intrauterine mechanisms, we assume that there are no effects of maternal genotypes on the offspring cardiometabolic outcome Y (either directly or mediated by offspring BW), and no causal effect of offspring BW on offspring cardiometabolic outcome Y. Allele frequencies were drawn from a uniform distribution between 0.1 and 0.9, and for each replicate, we sampled maternal (G
m
) and paternal dosages at each locus (0, 1, or 2) assuming Hardy–Weinberg equilibrium. We simulated the transmission of genotypes to offspring assuming autosomal Mendelian inheritance.
For each scenario, we performed 10,000 replicates using 50,000 mother–offspring pairs, 20 SNPs, and a moderate covariance between the residuals (ρ = −0.3). In Scenario 1, all
$${\beta _O}__j$$
were set to zero (i.e. no effect of offspring SNPs on offspring BW), while negative
$${\beta _M}__j$$
were drawn from a uniform distribution (between −0.05 and −0.01). The pleiotropic effect γ
j
was induced to have a negative correlation with
$${\beta _M}__j$$
by multiplying
$${\beta _M}__j$$
by −1.1 and adding independently drawn error terms (drawn from a uniform distribution between −0.03 and 0.03). In Scenario 2, values for
$${\beta _O}__j$$
were drawn from a uniform distribution (between −0.05 and −0.01). Values for
$${\beta _M}__j$$
were drawn from a uniform distribution between 0.01 and 0.05, while values for γ
j
were calculated by multiplying
$${\beta _M}__j$$
by 1.1 and adding error terms (drawn from a uniform distribution between −0.03 and 0.03). These procedures induced positive or negative effects of the genotypes on the exposures and outcomes according to Fig. 2.
Mendelian randomization and conditional analyses
We investigated the performance of several types of MR analysis on the simulated data including MR using an allele score of offspring BW-associated SNPs weighted by the (offspring) effect size on BW (WAS-MR),Reference Palmer, Lawlor and Harbord20 inverse variance weighted MR (IVW-MR),Reference Burgess, Butterworth and Thompson21 weighted median MR (WM-MR),Reference Bowden, Davey Smith, Haycock and Burgess22 and MR-Egger regression.Reference Bowden, Davey Smith and Burgess15 These methods all use BW-associated SNPs in the offspring to perform MR analysis, similar to what has been done by previous authors investigating the Barker hypothesisReference Zanetti, Tikkanen, Gustafsson, Priest, Burgess and Birthweight8–Reference Wang, Huang and Li10. Our performance measures of interest are estimates of the causal effect of BW on cardiometabolic phenotypes (i.e. these estimates should be zero since no causal relationship is simulated) and type 1 error rates.
We compared these methods to the procedure that we recommend, which involves regressing offspring cardiometabolic outcome on an allele score of maternal SNPs that are associated with offspring BW, while conditioning on offspring genotypes at the same loci.Reference Evans, Moen, Hwang, Lawlor and Warrington16 We note that while our procedure uses MR principles to increase its robustness to confounding and reverse causality, it does not yield estimates of a causal effect. This is because we do not believe that BW causally influences future cardiometabolic phenotypes, but rather is only an imperfect marker of the rate of intrauterine growth, and so estimating causal effect sizes in this context is inappropriate.
First, we regressed the simulated offspring cardiometabolic outcome on an unweighted maternal allele score (i.e. a simple count of the number of BW increasing alleles in each individual) while conditioning on an unweighted offspring allele score of the same SNPs. Second, we regressed offspring outcome on a weighted maternal allele score of BW-associated SNPs, controlling for each of the 20 SNPs (as separate terms) in the offspring. Our performance measures of interest were the regression coefficient of offspring cardiometabolic phenotype on maternal allele score (which should be zero since no causal relationship is simulated) and type 1 error rate. The R code used for performing the simulations used the two-sample MR package for many of the analysesReference Walker, Davies and Hemani23 and is available in the Supplementary Material.
Results
The average causal effect estimate of BW on the outcome and type 1 error rate (α = 0.05) across 10,000 replicates for each of the traditional MR approaches is presented in Table 1. Under both scenarios, WAS-MR, IVW-MR, WM-MR, and MR-Egger regression produced nonzero estimates of the mean causal effect and inflated type 1 error rates. In contrast, the mean effects from the conditional analyses were centered on zero (i.e. no causal effect) and had appropriate type 1 error rates.
Table 1. Results of the simulation study. The average effect estimate (causal estimate in the case of the four Mendelian randomization (MR) methods, and regression coefficient of offspring cardiometabolic outcome on maternal allele score in the case of the two conditional analyses), 95% Monte Carlo confidence intervals (CIs), type 1 error rates (α = 0.05), and the Monte Carlo CIs of these type 1 error rates. The four traditional MR methods used were: weighted allele score MR (WAS-MR), inverse variance weighted MR (IVW-MR), weighted median MR (WM-MR), and MR-Egger regression. The conditional analyses estimated the effect of the maternal genetic scores (conditioned on offspring genotype) on cardiometabolic outcomes

Discussion
All “standard” MR methods, which didn’t take into account the relationship between maternal and offspring genotypes, produced inflated type 1 error rates and biased estimates of the causal effect of BW on the outcome under Scenario 1 and Scenario 2 (Table 1). Therefore, investigators naively using these methods would likely come to the incorrect conclusion that BW has a causal effect on the risk of cardiometabolic disease. In contrast, conditional association analyses using either an unweighted or weighted maternal allele score corrected for offspring genotypes yielded no evidence of association with the outcomes and produced correct type 1 error rates (Table 1; Mean = 0; type 1 error rate = 0.05 all scenarios).
The results of our simulations clearly show that traditional MR analyses, even those that are more robust to violations of core instrumental variable assumptions like MR-Egger regressionReference Bowden, Davey Smith and Burgess15 and weighted median approaches,Reference Bowden, Davey Smith, Haycock and Burgess22 that do not take into account the relationship between maternal and offspring genotypes, can produce spurious evidence in favor of a causal relationship between BW and cardiometabolic disease in later life, when in fact no such relationship exists. In contrast, we have demonstrated that when maternal allele scores are conditioned on offspring genotype, the results maintain correct type 1 error in the absence of maternal genetic effects on the offspring cardiometabolic phenotype. Ideally, evidence for the association should also be examined using conditional analysis of father offspring pairs as a negative control. If a similar, nonzero association is also observed using paternal SNPs (conditional on offspring genotype at the same loci), then this strongly implies that the association between parental genotype and offspring phenotype may be mediated through the postnatal environment, rather than the intrauterine environment.
If investigators are interested in testing the validity of the Barker hypothesis, we recommend a strategy of testing for association between maternal genotypes related to BW and offspring cardiometabolic phenotypes conditional on offspring genotypes at the same loci.Reference Warrington, Beaumont and Horikoshi13,Reference Evans, Moen, Hwang, Lawlor and Warrington16,Reference Moen, Brumpton and Willer24 Indeed if the focus of interest is on DOHaD more broadly, then we point out that a similar framework could also be used to test for causal relationships between specific environmental exposures during pregnancy (e.g. maternal blood pressure, maternal adiposity, etc.) and offspring cardiometabolic phenotypes conditional on offspring genotype. Using MR principles to investigate specific maternal environmental exposures during pregnancy in relation to future cardiometabolic risk may be a superior strategy to just using maternal SNPs related to offspring BW for a number of reasons including (a) the underlying mechanisms responsible for the association between many maternal SNPs and offspring BW is unclear, (b) offspring BW itself is an imperfect measure of many processes of interest including the rate of intrauterine growth, and (c) it is possible that maternal environmental exposures have long-term effects on offspring cardiometabolic health but no effect on offspring BW.
In our analyses, we have used a simple multivariable regression analysis to test our hypotheses. This is because using instrumental variables analysis to estimate the causal effect of the rate of intrauterine growth would not be appropriate in this situation, since we have not directly measured the exposure of interest, merely BW – an imperfect proxy of the rate of intrauterine growth.Reference Freathy25 That being said, it may still be possible to estimate the causal effect of the rate of intrauterine growth on later life phenotypes using, for example, latent variable methods (making certain assumptions). Indeed, creating statistical genetics models to do this is a current focus of our research group.
Finally, we note that our procedure requires estimates of the association between maternal SNPs, conditional on offspring genotypes at the same loci, and offspring cardiometabolic phenotype. While conditional estimates can be obtained using genotyped mother–offspring pairs, there is a paucity of cohorts around the world with such information available, particularly where the offspring are old enough to have developed cardiometabolic conditions. Therefore, such conditional analyses may be underpowered.Reference Moen, Hemani, Warrington and Evans26 This shortfall in numbers may be partially addressed by calculating conditional estimates of maternal and offspring genetic effects using separate genome-wide association studies of unrelated mothers and offspring via structural equation modellingReference Warrington, Freathy, Neale and Evans12,Reference Evans, Moen, Hwang, Lawlor and Warrington16 or similar statistical procedures.Reference Grotzinger, Rhemtulla and de Vlaming27,Reference Zhu, Zheng and Zhang28 However, the power to accurately estimate conditional effect estimates is far less compared to if mothers and children from the same families are used.Reference Moen, Hemani, Warrington and Evans26 We have developed methods that impute “virtual” parental genotypes from genetic studies of relative pairs that can be used to derive conditional maternal genetic effect estimates and further increase the power of these sorts of analyses.Reference Hwang, Tubbs and Luong29 We are hopeful that new statistical methods such as these, large-scale genetic studies with information on families,Reference Magnus, Birke and Vejrup30,Reference Krokstad, Langhammer and Hveem31 and collaborations such as the within families genetics consortium,Reference Brumpton, Sanderson and Heilbron32 can be combined productively to enable appropriate testing of hypotheses related to the Barker hypothesis and more broadly DOHaD using MR in the near future.
Supplementary material
To view supplementary material for this article, please visit https://doi.org/10.1017/S2040174420001105.
Acknowledgments
None.
Financial support
This research was carried out at the Translational Research Institute, Woolloongabba, QLD 4102, Australia. The Translational Research Institute is supported by a grant from the Australian Government. G.H.M is supported by the Norwegian Research Council (postdoctorial mobility research grant 287198), the Norwegian Diabetes Association, and Nils Normans minnegave. D.M.E. is supported by an NHMRC Senior Research Fellowship (GNT1137714) and this work was supported by project grants (GNT1125200, GNT1157714).
Conflicts of interest
None.