Book contents
- Frontmatter
- Contents
- Contributors
- Preface
- 1 The Modern Mathematics of Deep Learning
- 2 Generalization in Deep Learning
- 3 Expressivity of Deep Neural Networks
- 4 Optimization Landscape of Neural Networks
- 5 Explaining the Decisions of Convolutional and Recurrent Neural Networks
- 6 Stochastic Feedforward Neural Networks: Universal Approximation
- 7 Deep Learning as Sparsity-Enforcing Algorithms
- 8 The Scattering Transform
- 9 Deep Generative Models and Inverse Problems
- 10 Dynamical Systems andOptimal Control Approach to Deep Learning
- 11 Bridging Many-Body Quantum Physics and Deep Learning via Tensor Networks
Contents
Published online by Cambridge University Press: 29 November 2022
- Frontmatter
- Contents
- Contributors
- Preface
- 1 The Modern Mathematics of Deep Learning
- 2 Generalization in Deep Learning
- 3 Expressivity of Deep Neural Networks
- 4 Optimization Landscape of Neural Networks
- 5 Explaining the Decisions of Convolutional and Recurrent Neural Networks
- 6 Stochastic Feedforward Neural Networks: Universal Approximation
- 7 Deep Learning as Sparsity-Enforcing Algorithms
- 8 The Scattering Transform
- 9 Deep Generative Models and Inverse Problems
- 10 Dynamical Systems andOptimal Control Approach to Deep Learning
- 11 Bridging Many-Body Quantum Physics and Deep Learning via Tensor Networks
Summary
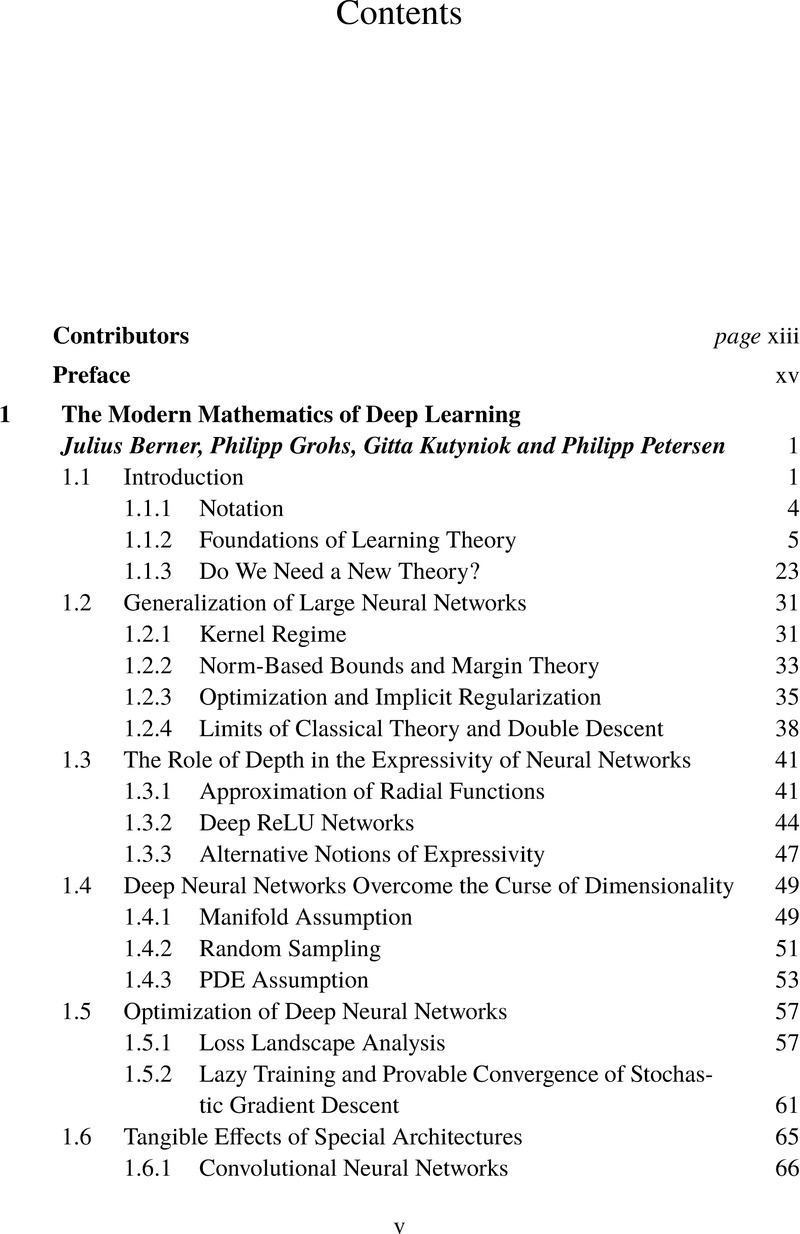
- Type
- Chapter
- Information
- Mathematical Aspects of Deep Learning , pp. v - xiiPublisher: Cambridge University PressPrint publication year: 2022