Introduction
Substantial co-morbidity among the mood and anxiety disorders (e.g. Kessler et al. Reference Kessler, Chiu, Demler and Walters2005) has led to questions about how these disorders should be organized and has resulted in a push for new conceptual models. In particular, a series of increasingly sophisticated dimensional models has generated much interest and support in recent years (e.g. Krueger & Finger, Reference Krueger and Finger2001; Krueger et al. Reference Krueger, Chentsova-Dutton, Markon, Goldberg and Ormel2003; Kupfer, Reference Kupfer2005; Watson, Reference Watson2005; Helzer et al. Reference Helzer, Kraemer and Krueger2006; Simms et al. Reference Simms, Grös, Watson and O'Hara2008), largely because they provide a compelling basis for describing the common and distinct components of anxious and depressive symptomatology in addition to the full breadth of features observed clinically in psychiatric and primary care settings. However, despite mounting evidence supporting dimensional conceptualizations, the fields of psychology and psychiatry have not yet reached a consensus on whether and how to implement such models in the next revision of the Diagnostic and Statistical Manual of Mental Disorders (i.e. DSM-5) and the International Classification of Diseases (i.e. ICD-11). Moreover, work remains on understanding the optimal dimensional model of emotional symptoms, including how and whether somatization symptoms relate to such dimensional models (e.g. Dimsdale et al. Reference Dimsdale, Xin, Kleinman, Patel, Narrow, Sirvatka and Regier2009; Goldberg et al. Reference Goldberg, Kendler, Sirovatka and Regier2010).
Dimensional models of internalizing symptoms
Clark & Watson's (Reference Clark and Watson1991) tripartite model proposed that (a) anxiety and depression share a non-specific component, negative affectivity (NA), that contributes to the co-morbidity between these disorder types, (b) depression is characterized specifically by anhedonia, and (c) anxiety and fear are marked by a specific component of anxious/somatic arousal. This model generated much support (e.g. Phillips et al. Reference Phillips, Lonigan, Driscoll and Hooe2002; Lambert et al. Reference Lambert, McCreary, Joiner, Schmidt and Ialongo2004; Watson et al. Reference Watson, Clark, Weber, Assenheimer, Strauss and McCormick1995a, Reference Watson, Weber, Assenheimer, Clark, Strauss and McCormickb) but later was deemed too limited to describe the full breadth of symptoms relevant to emotional disorders. As a result, more detailed models have been offered (e.g. Zinbarg & Barlow, Reference Zinbarg and Barlow1996; Brown et al. Reference Brown, Chorpita and Barlow1998; Mineka et al. Reference Mineka, Watson and Clark1998; Krueger & Finger, Reference Krueger and Finger2001; Simms et al. Reference Simms, Grös, Watson and O'Hara2008). For example, Simms et al. (Reference Simms, Grös, Watson and O'Hara2008) reported evidence for a symptom-based model that (a) replicated the general NA component and (b) supported a differentiated lower-order structure of mood and anxiety symptoms.
Disorder-based models (i.e. models of disorder covariances rather than symptom covariances) have also supported a dimensional reconceptualization of the mood and anxiety disorders. For example, based on latent trait analyses on a sample of treatment-seeking individuals, Krueger & Finger (Reference Krueger and Finger2001) proposed an internalizing spectrum model of anxiety and depressive disorders in which disorders were modeled as reflecting varying levels of severity along the same internalizing dimension. McGlinchey & Zimmerman (Reference McGlinchey and Zimmerman2007) replicated these results in a larger sample of out-patients and showed that internalizing is associated with several indicators of dysfunction and social burden. Other disorder-based studies have not only replicated the presence of an overarching internalizing factor but also identified NA as the core of the internalizing spectrum (e.g. Hettema et al. Reference Hettema, Neale, Myers, Prescott and Kendler2006; Griffith et al. Reference Griffith, Zinbarg, Craske, Mineka, Rose, Waters and Sutton2010). Unfortunately, disorder-based structural analyses can be problematic because of the low base rates associated with some disorders, heterogeneity within disorders, and changes in diagnostic criteria across different DSM versions (Watson, Reference Watson2005). Symptom-based analyses ameliorate many of these problems because they do not rely on rationally derived criterion sets or polythetic scoring rules.
Although not identical, both symptom- and disorder-based dimensional models share several important features. First, most include non-specific factors (e.g. NA) that have been shown to play a significant role in the mood and anxiety disorders. Second, most models include specific components that are offered to more finely differentiate symptom phenotypes (e.g. post-traumatic intrusions, panic, depression) that share NA as a common factor. Third, the models generally include a higher-order or hierarchical structure characterized by a general factor that subsumes multiple specific symptom factors. Higher-order factor models imply that the variance shared by specific factors (e.g. anxiety and depression) can be accounted for parsimoniously by an overarching dimension of severity (e.g. Krueger & Finger, Reference Krueger and Finger2001; Krueger et al. Reference Krueger, Chentsova-Dutton, Markon, Goldberg and Ormel2003). Hierarchical models describe symptoms as loading simultaneously on a general factor along with one or more specific factors (e.g. Simms et al. Reference Simms, Grös, Watson and O'Hara2008).
Based on these symptom- and disorder-based findings, some have argued for an empirically based classification system that accounts for the known patterns of disorder/symptom covariation (e.g. Watson, Reference Watson2005; Goldberg et al. Reference Goldberg, Krueger, Andrews and Hobbs2009). Goldberg et al. (Reference Goldberg, Krueger, Andrews and Hobbs2009) argued that anxiety and mood disorders defined primarily by NA [such as major depressive disorder (MDD), generalized anxiety disorder (GAD), phobias, post-traumatic stress disorder (PTSD), obsessive–compulsive disorder (OCD) and panic disorder] should be reclassified as falling into a single ‘emotional disorders’ cluster in DSM-5 and ICD-11. Similarly, Watson (Reference Watson2005) argued for an overarching category of emotional disorders and also three subclasses: (a) distress disorders, (b) fear disorders, and (c) bipolar mood disorders.
Symptomatology in primary care settings
The majority of individuals with mental disorders are seen by general practitioners, family physicians, and physicians in general hospitals (Regier et al. Reference Regier, Goldberg and Taube1978, Reference Regier, Narrow, Rae, Manderscheid, Locke and Goodwin1993; Goldberg & Goodyer, Reference Goldberg and Goodyer2005). In such settings, patients often present with combinations of anxious, depressive and somatic symptoms, in addition to physical health problems, and as a result they frequently satisfy the criteria for multiple diagnoses (Üstün & Sartorius, Reference Üstün and Sartorius1995). Somatic symptoms, both physical and psychological in origin, are commonly the reason for presentation to physicians in general medical settings (e.g. Kroenke, Reference Kroenke2003). Because of this, Mayou et al. (Reference Mayou, Kirmayer, Simon and Kroenke Sharpe2005) suggested that future versions of the diagnostic nosology adopt etiological neutrality about those somatic symptoms that are not clearly associated with a general medical condition, and argued for the abolition of the somatoform disorders category, with reassignment of specific somatoform symptoms to other parts of the classification system, such that somatic symptoms with depression are classified with depression and those associated with anxiety are classified with anxiety. However, relatively little is known about how somatic symptoms relate to existing dimensional models of the mood and anxiety disorders. Thus, before implementing such a major change, more work is needed to better understand how somatic symptoms behave psychometrically in the context of the emotional disorders.
Current study
Thus, although consensus is emerging on the basic tenets of the dimensional structure of common emotional disorders, less consensus exists on the exact form of the higher-order/hierarchical structure of such disorders. Moreover, much less is known about how somatic symptoms fit within a comprehensive dimensional structure of emotional disorders. Can variance in somatic symptoms be completely accounted for by the same overarching general factor common to the mood and anxiety disorders? Or are somatic symptoms statistically independent of mood and anxiety symptoms? To answer these questions and extend the structural literature, we examined several viable lower- and higher-order structural models of mood, anxiety and somatic symptoms in a large, multi-national sample of primary care patients. Krueger et al. (Reference Krueger, Chentsova-Dutton, Markon, Goldberg and Ormel2003) conducted disorder-based analyses using the same data set and found a structure similar to that reported in previous epidemiological studies (i.e. internalizing spectra). The present study extends previous work by Krueger and colleagues and others by (a) modeling symptom data directly, (b) studying the location of somatic symptoms in relation to other emotional symptoms, (c) examining these structural questions in primary care patients from around the world, and (d) including a range of viable lower- and higher-order models.
Method
Sample and measures
The present study used data from the World Health Organization's Collaborative Study of Psychological Problems in General Health Care (WHO/PPGHC; Üstün & Sartorius, Reference Üstün and Sartorius1995), consisting of 5438 patients interviewed with the Primary Care Version of the Composite International Diagnostic Interview (CIDI-PC; Sartorius et al. Reference Sartorius, Üstün, Costa e Silva, Goldberg, Lecrubier, Ormel, Von Korff and Wittchen1993) from 15 general health care clinics in 14 countries.Footnote 1Footnote † Compared to the usual version of the CIDI, the CIDI-PC used in the present study coded whether symptoms were currently present and was adapted to minimize skip-outs (i.e. most symptoms were asked of all participants), which is ideal for structural analyses and uncommon in standard interviews. Other ratings included the main reason for contact, chronic diseases and alcohol use; psychotic symptoms were excluded. Participants were selected from 25 916 consecutive patients by a stratified sampling procedure in each center using the General Health Questionnaire (GHQ-12; Goldberg & Williams, Reference Goldberg and Williams1988), in which all respondents in the top 20% of scores for that center, 35% of those in the next 20%, and 10% of the remaining scores were selected for clinical interview with the CIDI-PC. Thus, the sample was weighted toward patients presenting with current mental health concerns and related dysfunction. This particular within-center stratification scheme was adopted to account for variations in how readily patients admit to psychological symptoms across centers. The 14 centers included Ankara, Turkey (n=400); Athens, Greece (n=196); Bangalore, India (n=398); Berlin and Mainz, Germany (n=800); Groningen, The Netherlands (n=340); Ibadan, Nigeria (n=269); Paris, France (n=405); Manchester, UK (n=428); Nagasaki, Japan (n=336); Rio de Janeiro, Brazil (n=393); Santiago, Chile (n=274); Shanghai, China (n=576); Seattle, USA (n=373); and Verona, Italy (n=250).
Item-level psychiatric symptom data were used for the present study's latent structure analyses. All CIDI-PC symptoms related to depression, anxiety or somatic problems were included, except for two items with extremely low base rates (‘amnesia’ and ‘suicide attempt’). In total, 38 somatization, 25 anxiety and 28 depression symptom items were included. Symptoms that were clearly due to medical illness, or that were not currently present, were not counted as present in our analyses.Footnote 2 DSM-III-R (APA, 1987) diagnostic variables generated using the CIDI diagnostic algorithms were used as outcome variables in subsequent regression analyses; diagnoses representing current (as opposed to lifetime) psychopathology were used wherever possible. Disability was assessed using the Social Disability Schedule (SDS; Wiersma et al. Reference Wiersma, DeJong and Ormel1988) and the Brief Disability Questionnaire (BDQ; VonKorff et al. Reference VonKorff, Üstün, Ormel, Kaplan and Simon1996). Scores from the SDS (i.e. interviewer-rated disability in the occupational role) and the BDQ (i.e. total score and number of disability days in the past month) were used as outcome variables in regression analyses. Evidence supporting the reliability and validity of these measures has been presented in reports of the WHO/PPGHC (Ormel et al. Reference Ormel, VonKorff, Üstün, Pini, Korten and Oldehinkel1994).
Structural modeling and statistical analyses
We adopted a two-stage procedure to evaluate several competing latent variable models of the latent structure of psychiatric symptoms. First, we evaluated the fit of three lower-order factor models: model 1, a one-factor model in which all mood, anxiety and somatic symptoms were subsumed under a single internalizing factor; model 2, a two-factor model composed of correlated somatic and anxiety–depression factors; and model 3, a three-factor model in which somatic, anxious and depressive symptoms are loaded on three correlated factors. These lower-order models provided a basis for understanding how many factors underlie the symptoms, but they did not provide an opportunity to study the higher-order or hierarchical structure of the domain. Thus, second, we studied two variations on the best-fitting lower-order model: model 3h, a higher-order model in which the three factors from model 3 were subsumed under a general internalizing factor; and model 3b, a hierarchical (bifactor) model in which all symptoms were modeled as loading on a general internalizing factor along with one of three specific (i.e. residual) factors corresponding to depression, anxiety and somatic symptoms respectively (e.g. Gibbons et al. Reference Gibbons, Bock, Hedeker, Weiss, Segawa, Bhaumik, Kupfer, Frank, Grochocinski and Stover2007; Reise et al. Reference Reise, Morizot and Hays2007; Simms et al. Reference Simms, Grös, Watson and O'Hara2008). Graphical representations of all estimated models are presented in Fig. 1.
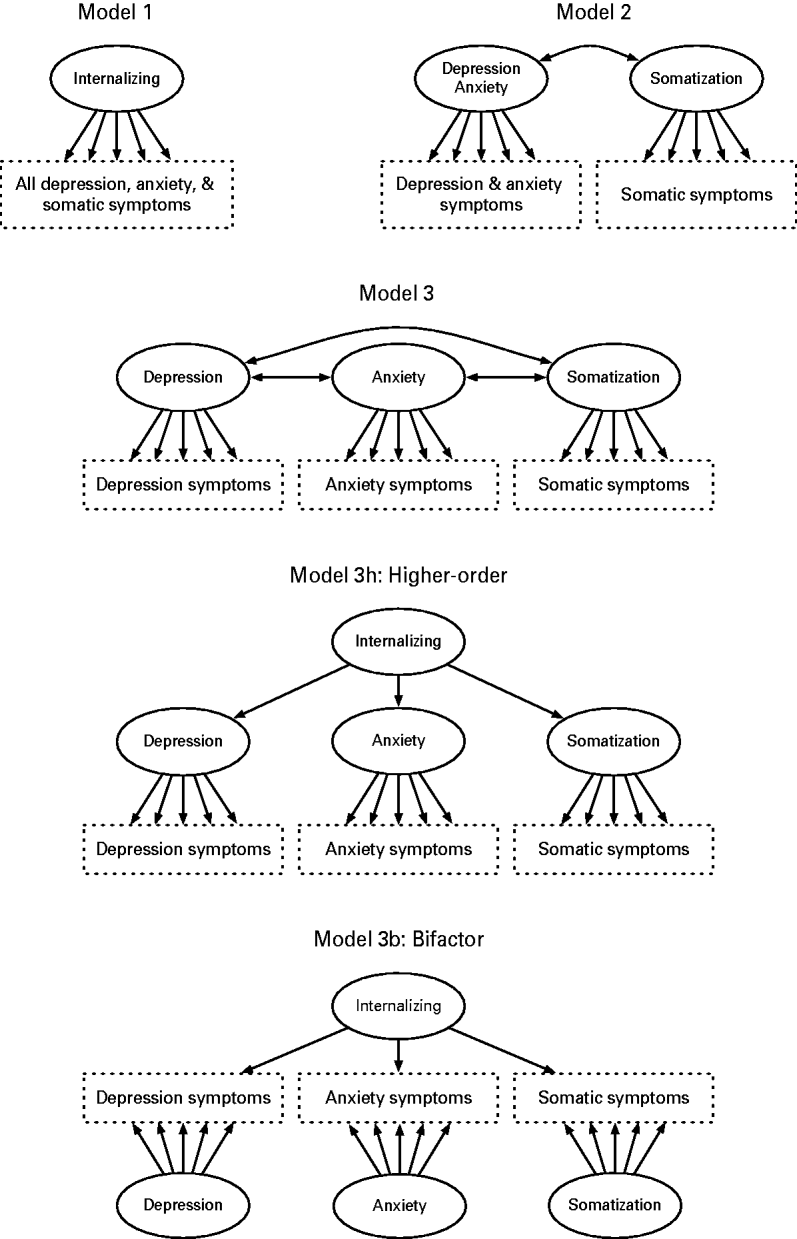
Fig. 1. Summary of a priori structural models. Dotted rectangles represent collections of symptoms. Error terms on symptoms and intermediate factors are omitted for clarity.
CIDI items were assigned to symptom factors based on the diagnostic category from which they were drawn (i.e. anxiety CIDI items were assigned to the anxiety factor, etc.). Notably, this strategy resulted in several similar items loading on multiple factors (e.g. similarly worded items reflecting a ‘lump in throat’ are included in the CIDI for both anxiety and somatization disorder). Piccinelli et al. (Reference Piccinelli, Rucci, Üstün and Simon1999), who used the same data set for a different grade-of-membership analysis of the symptoms, dealt with this potential structural confound by removing all similar items from their analyses. However, doing so necessarily changed the underlying constructs and their inter-relationships by artificially removing the overlap that is embodied in the DSM. Thus, in the present study we used all items within each diagnostic category to maintain maximum fidelity with the diagnostic constructs we were trying to represent.
Latent variable models were estimated using Mplus version 5.21 (Muthén & Muthén, Reference Muthén and Muthén2009). Sample weights and stratification variables were applied to obtain unbiased parameter estimates and standard errors. Parameters were estimated in a logistic modeling framework using maximum likelihood estimation with robust standard errors (MLR; Muthén & Muthén, Reference Muthén and Muthén2009), appropriate for data featuring categorical dependent variables, missing data, complex sampling designs, and violations of assumptions of normality and independence of observations. Model fit was evaluated using Akaike's Information Criterion (AIC) and Bayesian Information Criterion (BIC), which prefer models with a balance of fit and parsimony. In both cases, lower values indicate better model fit. Descriptively, a BIC difference of 0–2 equals ‘weak’ evidence, 2–6 equals ‘positive’ evidence, 6–10 equals ‘strong evidence,’ and >10 equals ‘very strong’ evidence in favor of the model with the lower BIC value (Raftery, Reference Raftery1995).Footnote 3
Following the empirical comparison of the estimated factor models, item response theory (IRT) parameters and factor scores were computed for each factor from the model with the lowest BIC value. IRT parameters were used to plot test information curves for each symptom type (i.e. anxiety, depression and somatic symptoms) along both the general and specific factors. IRT discrimination and difficulty/severity parameters were calculated from Mplus unstandardized factor loadings and thresholds respectively, as described in Muthén & Muthén (Reference Muthén and Muthén2006). Discrimination parameters were obtained by dividing unstandardized factor loadings by 1.7, and difficulty parameters were calculated by dividing unstandardized item thresholds by unstandardized factor loadings. Test information was calculated using the following formula:

where I ik(θ) is the test information as a function of underlying trait θ, a i is discrimination of item i, b i is difficulty of item i, and k is the number of items within a given symptom type.
Factor scores were used as predictors in a variety of regression models designed to investigate the relationship between the selected factor model and clinically relevant outcomes. As noted earlier, outcomes included psychiatric diagnoses (i.e. MDD, dysthymic disorder, GAD, panic disorder, agoraphobia, somatization disorder, hypochondriasis, ICD neurasthenia, and alcohol dependence) and disability (i.e. interviewer-rated SDS disability in the occupational role, BDQ total score, and number of disability days in the past month). Zero-order correlations among predictors and outcomes were also examined. Regression analyses were conducted in Mplus (Muthén & Muthén, Reference Muthén and Muthén2009). As with the above-mentioned latent structure analyses, the study's complex sampling design was explicitly modeled, parameters were estimated using MLR, and BIC was used to evaluate the relative parsimony-adjusted model fit among competing regression models (e.g. a model with a general factor predicting a given outcome versus a model with both general and specific factors predicting the outcome). Linear regression models were estimated for continuous outcomes (i.e. BDQ total score) and logistic regression models were estimated for categorical outcomes (i.e. all diagnostic variables and the SDS disability score). A Poisson regression model was estimated for the number of disability days in the past month, and zero inflation was tested by estimating a zero-inflated Poisson model and comparing BIC values between the zero-inflated and non-zero-inflated Poisson models. All reported β values were fully standardized, except for those associated with the Poisson model (Muthén & Muthén, Reference Muthén and Muthén2009).
Results
Structural modeling
Of the lower-order models, model 3, which included three correlated factors for anxiety, depression and somatic symptoms, yielded the lowest AIC and BIC values (Table 1). The differences in information criteria between model 3 and the next best-fitting model were 1822 and 1809 for AIC and BIC respectively, which provides very strong evidence of the superiority of model 3 over the other lower-order models according to Raftery's (Reference Raftery1995) criteria. We then fit higher-order and bifactor variations of model 3. The higher-order version, model 3h, yielded fit values that were identical to model 3. However, the bifactor model, model 3b, produced AIC and BIC differences over model 3 of 1417 and 836 respectively, which provides strong evidence of the superiority of model 3b over all other models.Footnote 4, Footnote 5
Table 1. Model fitting results

AIC, Akaike's Information Criterion; BIC, Bayesian Information Criterion.
a Lowest information criterion values.
Model statistics, including standardized factor loadings on both the general internalizing and specific factors, latent trait modeling parameters and symptom base rates, are presented in Table 2. All symptoms loaded significantly on the internalizing factor (median loading=0.64, range 0.25–0.84), which suggests that all of the sampled anxiety, depression and somatic symptoms share substantial variance that can be accounted for by a single general factor. The findings for the specific factors were more variable. Whereas the depression and somatic specific factors included moderate and consistently signed loadings of their respective symptoms (median loadings=0.35 and 0.39 respectively), the anxiety specific factor loadings averaged 0.10 and included both positively and negatively signed loadings. These results suggest that the depression and somatic specific factors reflect meaningful residual variance not accounted for by the internalizing factor, whereas the anxiety variance seems to be tapped primarily by the internalizing factor. The anxiety specific factor, which was marked by 10 low positive loadings reflecting psychological anxiety, eight low negative loadings reflecting somatic symptoms and seven non-significant loadings reflecting a mix of specific somatic and situational anxiety symptoms, defies a clear substantive interpretation and probably reflects relatively trivial residual variance not explained by the general internalizing factor.Footnote 6
Table 2. Base rates, factor loadings and latent trait parameters for each modeled symptom

CIDI-PC, Composite International Diagnostic Interview – Primary Care Version; a, discrimination; b, difficulty/severity.
Symptoms are presented in descending order of internalizing factor loadings, separately by symptom type. All loadings are standardized.
* Loading is significant, p<0.01.
IRT test information curves (TICs) were calculated to demonstrate graphically how the anxiety, depression and somatic symptoms related to the internalizing and specific factors. Using item discrimination and difficulty/severity parameters calculated from the standard Mplus output, we calculated and plotted TICs for each symptom type along the general and specific factors. The internalizing factor TICs (see Fig. 2 a) were calculated by summing item information separately for the anxiety, depression and somatic symptoms, and also across all 91 symptoms, using the internalizing factor IRT parameters in Table 2. The peaks of these curves show that anxiety and depression symptoms (which have higher peaks) share more variance with the internalizing factor than do the somatic items. However, the horizontal position of the curves reveals that somatic symptoms reflect the highest severity along the internalizing factor, anxiety reflects the lowest severity, and depression reflects intermediate severity. Similarly, specific factor TICs (see Fig. 2 b) were calculated by summing item information separately for the anxiety, depression and somatic symptoms using the specific factor IRT parameters in Table 2. Mirroring the internalizing factor results, the specific factor TICs showed that depression and somatic symptoms included substantial variance not shared with the internalizing factor, especially at higher severity levels, whereas anxiety symptoms yielded little information value above that shared with the internalizing factor.

Fig. 2. Bifactor model test information curves along the (a) internalizing and (b) specific factors.
Model correlates
To aid interpretation of the modeled factors and study their construct validity, we next conducted a series of correlational and regression analyses. Zero-order correlations between bifactor model factor scores and diagnostic variables (biserial correlations) and dysfunction variables (Pearson correlations) are presented in Table 3. Notably, bifactor model scores and CIDI-PC diagnoses were computed using the same symptoms. As such, these correlations, which demonstrate how the modeled factors map onto the psychiatric syndromes measured by the CIDI-PC, revealed that the internalizing factor correlated significantly with all diagnoses. The strongest internalizing factor correlates were MDD, GAD, neurasthenia, agoraphobia and dysthymic disorder, which together show that the internalizing factor reflects a broad range of distress-related internalizing symptomatology similar to that found in previous structural studies. By contrast, the specific factor results revealed relatively few substantial correlations: (a) the depression factor correlated specifically with MDD (r=0.49), (b) the somatic factor correlated specifically with somatization disorder (r=0.38), hypochondriasis (r=0.31) and neurasthenia (r=0.22), and (c) the anxiety factor correlated minimally with all diagnostic variables, except for hypochondriasis. The relatively low correlations with alcohol dependence provide evidence of discriminant validity for both the internalizing and specific factors.
Table 3. Correlations among bifactor model factor scores and diagnostic/dysfunction scores

INT, General internalizing factor; SOM, somatic specific factor; ANX, anxiety specific factor; DEP, depression specific factor; SDS, Social Disability Schedule; BDQ, Brief Disability Questionnaire.
n values range from 5356 to 5433. All r's ⩾0.04 are significant, p⩽0.01. Correlations ⩾0.20 are presented in boldface. Correlations involving diagnoses are biserial correlations.
Correlations with the dysfunction variables revealed a similar pattern. Psychosocial dysfunction, as measured by the BDQ, SDS and the number of disability days in the past month, correlated most strongly with the internalizing factor. The depression and somatic specific factors also correlated significantly, but not as strongly, with all three dysfunction variables, whereas the anxiety specific factor failed to relate consistently with the dysfunction variables. Taken together, these results support the interpretation of the general internalizing factor as a broad internalizing dimension with significant connections to dysfunction.
Follow-up hierarchical regression analyses (see Table 4) were conducted to study whether and how the specific factors improved the prediction of CIDI-PC diagnoses and dysfunction above the internalizing factor (i.e. incremental validity). These results confirmed that the internalizing factor is a proxy for MDD and GAD and, to a lesser extent, somatization disorder and neurasthenia (R 2 values=74.9, 72.4, 54.7 and 56.3% respectively), suggesting that much of the variance in these syndromes can be parsimoniously modeled as a single internalizing dimension. Moreover, the internalizing factor accounted for significant variance in all measures of dysfunction. Although the internalizing factor accounted for the most variance for all diagnostic and dysfunction variables, the specific factors significantly incremented these predictions in some cases. For example, the specific factors accounted for an additional 14.6% and 5.4% of the variance in MDD and dysthymia respectively (driven primarily by the depression specific factor), and an additional 13.1, 5.3 and 5.5% of the variance in somatization disorder, hypochondriasis and neurasthenia respectively (driven primarily by the somatic specific factor). Similarly, the specific factors significantly incremented the prediction of the dysfunction variables.
Table 4. Regressions predicting disorders and dysfunction from bifactor model factor scores

BIC, Bayesian Information Criterion; INT, general internalizing factor; SOM, somatic specific factor; ANX, anxiety specific factor; DEP, depression specific factor; MDD, major depressive disorder; GAD, generalized anxiety disorder; SDS, Social Disability Schedule; BDQ, Brief Disability Questionnaire; n.a., not applicable.
* Significant β, p<0.05.
a Note that, for regressions predicting BDQ disability days, which is a count variable for which R 2 statistics are not available, the zero-inflated Poisson model (BIC=29066) fits substantially better than the non-zero-inflated Poisson model (BIC=49733); thus results are reported for the zero-inflated model.
Discussion
We examined a range of viable symptom-based structural models of anxiety, depression and somatic symptomatology in a large, multi-national data set of psychiatric symptoms in primary care settings. Our results identified a hierarchical, bifactor model, with symptoms loading simultaneously on a general internalizing factor and on one of three specific anxiety, depression and somatic factors, as the best-fitting model. The construct validity analyses suggest that the general factor is a proxy for negative affectivity, distress and internalizing psychopathology more generally (e.g. Hettema et al. Reference Hettema, Neale, Myers, Prescott and Kendler2006; Simms et al. Reference Simms, Grös, Watson and O'Hara2008; Goldberg et al. Reference Goldberg, Krueger, Andrews and Hobbs2009; Griffith et al. Reference Griffith, Zinbarg, Craske, Mineka, Rose, Waters and Sutton2010), and that this factor has clear connections with psychosocial dysfunction. However, the results also revealed significant residual variance related to depression and somatic symptomatology that (a) was independent of the internalizing factor, (b) was specific to diagnoses of major depression and somatoform disorders respectively, and (c) incrementally predicted psychosocial dysfunction beyond the internalizing factor.
These findings are novel in several ways. First, although numerous studies have examined the structure of anxiety and depression symptomatology in the context of a broad general NA or internalizing factor, few studies have considered how somatic symptoms relate to such models. At the disorder level, Krueger et al. (Reference Krueger, Chentsova-Dutton, Markon, Goldberg and Ormel2003) found evidence that somatoform disorders shared variance with other mood and anxiety disorders through a broad internalizing factor. In the present study, we extended this finding to symptom-based analyses. Second, we used a large multi-national sample of primary care patients as the basis for structural analyses, which should improve the generalizability of our findings beyond what is typically possible in structural investigations of this variety. Third, we extended previous work by examining not only lower-order models (as in work by Krueger et al. Reference Krueger, Chentsova-Dutton, Markon, Goldberg and Ormel2003) but also higher-order and hierarchical variants of the best-fitting lower-order model. Examinations of the hierarchical structure of a domain are important, as they provide insights into how related psychiatric phenotypes (e.g. anxiety, depression and somatic symptoms) should be modeled in research or organized in the official psychiatric nosology. Similar to Simms et al. (Reference Simms, Grös, Watson and O'Hara2008), the present findings support a hierarchical, bifactor arrangement of internalizing symptomatology and extend it to include somatic symptoms. At a practical level, the findings suggest that somatic symptoms without clear physical causes may be best understood, at least partially, as manifestations of internalizing psychopathology rather than as an independent class of disorder.
The depression and somatic specific factors are interesting in the context of existing integrative hierarchical models of the mood and anxiety disorders (Brown et al. Reference Brown, Chorpita and Barlow1998; Mineka et al. Reference Mineka, Watson and Clark1998). The present results confirm that much phenotypic variance is shared across presumably different symptom types, but they also suggest that such symptoms/syndromes have unique components that cannot be ignored in any comprehensive psychiatric nosology. The present data do not directly assess the meaning of the specific components. However, an examination of the top-loading symptoms on the specific factors provides some clues regarding their meaning. The top-loading symptoms on the specific depression factor (e.g. ‘felt sinful’, ‘felt inferior to others’, ‘felt worthless’, ‘felt guilty’, ‘little self-confidence’, ‘difficulty enjoying good things’ and ‘thought about committing suicide’) reflect a range of cognitive, affective and behavioral symptoms that seem to be relatively specific to depression. Less is certain about the meaning of the specific somatic factor, which included the following top-loading symptoms: ‘nausea without vomiting’, ‘abdominal pain’, ‘temporary blurred vision’, ‘loose bowels or diarrhea’, ‘bad taste in mouth’, ‘trouble with back pain’ and ‘trouble with frequent urination’. Although somatic complaints with a clear physical origin were excluded during the interview, it is possible that this factor represents the variance due to physical disorders that had not been diagnosed by the doctor at the time of the complaint. Alternatively, the breadth of the somatic complaints suggests that this specific factor may represent variance related to somatization disorder that is not captured by the general internalizing factor.
A notable finding was that, in contrast to depression and somatic symptoms, little meaningful anxiety variance remained after accounting for the internalizing factor. Dimensional models have suggested that anxiety disorders should also include specific variance not shared with NA, so our failure to identify a meaningful specific anxiety factor was a surprise. The CIDI-PC included a range of both cognitive and somatic anxiety symptoms; thus, the lack of specific anxiety variance is probably not due to inadequacies in the pool of anxiety symptoms. One likely hypothesis is that the internalizing factor is broader than the NA factor proposed in many dimensional models of mood and anxiety disorders (e.g. Clark & Watson, Reference Clark and Watson1991; Mineka et al. Reference Mineka, Watson and Clark1998). Rather, our internalizing factor seems to include variance related to distress, anxious arousal and other somatic symptoms, the breadth of which is consistent with the category of internalizing or general distress disorders found in previous structural studies. Alternatively, the results may suggest that patients' responses to anxiety symptoms were strongly saturated with NA variance, perhaps due to differences in the expression of anxiety in primary care and/or multi-national settings. Additional studies are needed to replicate these structural results and to further examine the meaning and construct validity of the general and specific factors.
The present findings suggest that much variance associated with somatic symptomatology is shared with the mood and anxiety disorders under a broad umbrella of internalizing psychopathology. In the context of previous studies showing strong connections between somatic/hypochondriacal symptoms and NA (e.g. Longley et al. Reference Longley, Watson and Noyes2005; Noyes et al. Reference Noyes, Watson, Letuchy, Longley, Black, Carney and Doebbeling2005), our findings have implications for the ongoing preparations for the next revisions of the psychiatric nosology, DSM-5 and ICD-11. Our results confirm the presence of substantial overlap among somatic, mood and anxiety symptoms (Mayou et al. Reference Mayou, Kirmayer, Simon and Kroenke Sharpe2005; Löwe et al. Reference Löwe, Spitzer, Williams, Mussell, Schellberg and Kroenke2008). Given this overlap, somatic symptoms with non-physical etiologies may warrant relocation in the nosology as one of a broad group of emotional disorders (e.g. Watson, Reference Watson2005; Goldberg et al. Reference Goldberg, Krueger, Andrews and Hobbs2009) rather than as an independent category of somatoform disorders. However, although such an approach might be helpful in primary care settings, where combinations of anxious, depressive and somatic symptoms are commonly the presenting problems (Üstün & Sartorius, Reference Üstün and Sartorius1995; Kroenke, Reference Kroenke2003), nosological conclusions based on the present data must be considered tentative until confirming studies appear in the literature.
Finally, the test information analyses revealed an interesting pattern of internalizing factor severity associated with anxiety, depression and somatic symptomatology. Our data suggest that anxiety symptoms reflect the least severe manifestations of the general internalizing factor, followed by depressive symptoms that reflect somewhat higher severity and somatic symptoms that reflect the highest severity. Examining severity in this way may be useful in understanding the nature of different symptom types and their relationship to an underlying vulnerability to internalizing psychopathology. The present results suggest a possible progression of symptom severity in which the particular phenotype exhibited reflects, in part, the degree of underlying internalizing diatheses. Thus, mood, anxiety and somatic symptoms may differ, to some extent, in severity rather than in kind, which has interesting implications for clinical work. For example, the results suggest that the presence of somatic symptoms in a given patient is important because they suggest probabilistically that the patient may also be experiencing a range of other internalizing symptoms. Of course, these severity findings are novel and require replication before firm conclusions are possible.
Limitations and conclusions
Our study is not without limitations. First, although the multi-national nature of our sample was a strength compared to previous studies of this variety, we were unable to examine nation-, region- or language-specific structural moderation effects because of the computational demands associated with the large number of symptoms examined. Although the identified bifactor structure fit well across the aggregated sample, it is possible that sample-specific effects could emerge if such analyses were possible with the full symptom set. To that end, future research could develop short forms of each symptom type that would permit studies of how or whether the symptom structure varies as a function of national origin, region or language. The etic nature of the CIDI-PC symptom translation procedures, which assumed that the forms and symptoms of psychopathology are consistent across cultures, is a second weakness of our study. In particular, our results are limited to Western accounts of major psychopathology to the extent that cultural differences result in different manifestations of psychopathology around the world. Finally, strong nosological conclusions require replication in novel samples and in relation to a broader range of disorder classes (e.g. externalizing and personality disorders).
However, our results meaningfully extend previous structural studies, especially in highlighting the phenotypic overlap among anxiety, depression and somatic symptoms and providing an empirically based structure for organizing such symptom types in research settings and future nosological systems. The bifactor model provides a compelling way to model symptoms that have both shared and unique components, which is often the case in psychiatric settings in which NA drives much of the diagnostic overlap observed clinically. The present results confirmed the presence of a strong general internalizing factor along which anxiety, depression and somatic symptoms can be modeled, as well as specific factors for depression and somatic symptoms that presumably reflect aspects of those symptoms that are independent of the internalizing factor. Taken together, our results are consistent with efforts to build a psychiatric nosology that better accounts for the common and unique features of psychiatric disorders. In addition, diagnostic methods are needed (e.g. computerized scoring and/or administration of measures) that permit efficient parsing of such common and unique elements in clinical settings.
Acknowledgements
This study was supported by a grant from the American Psychiatric Institute for Research and Education (APIRE). The original study on which the present analyses were based was supported by a grant from the World Health Organization (WHO). Dr Simms is supported by National Institutes of Health (NIH) grant R01MH080086 and Dr Krueger is supported by NIH grant U01AA018111.
Declaration of Interest
None.