Introduction
Despite over half a century of research, many issues about the clinical and etiological heterogeneity of major depression (MD) are still unresolved (MacFadyen, Reference MacFadyen1975; Kendell, Reference Kendell1976; Cole et al. Reference Cole, McGuffin and Farmer2008). Is depression a homogeneous clinical syndrome? If not, is it possible to identify clinically meaningful and scientifically valid subtypes?
In recent decades, latent class analysis (LCA) has become the most popular of the numerous statistical techniques that have been used to address these questions. Most clinical diagnoses begin by experienced clinicians seeing a wide range of patients and looking for homogeneity in crucial signs and symptoms. LCA mimics this process in a more rigorous statistical manner. On the basis of selected symptoms (typically scored as present or absent) that serve as program input, LCA sorts patients into mutually exclusive categories.
We were able to locate 16 previously published LCA studies of MD (Table 1). These studies varied in their method of patient ascertainment (community versus clinical samples), sample size (from n = 80 to n = 12 180), and nature and source of the depressive symptoms used in the analysis (DSM criteria assessed at interview versus self-report depressive symptom scales). The results were also variable in both the number and characteristics of the identified classes. All of these studies were conducted in Western populations.
Table 1. Main features of prior published latent class analyses (LCAs) of depression
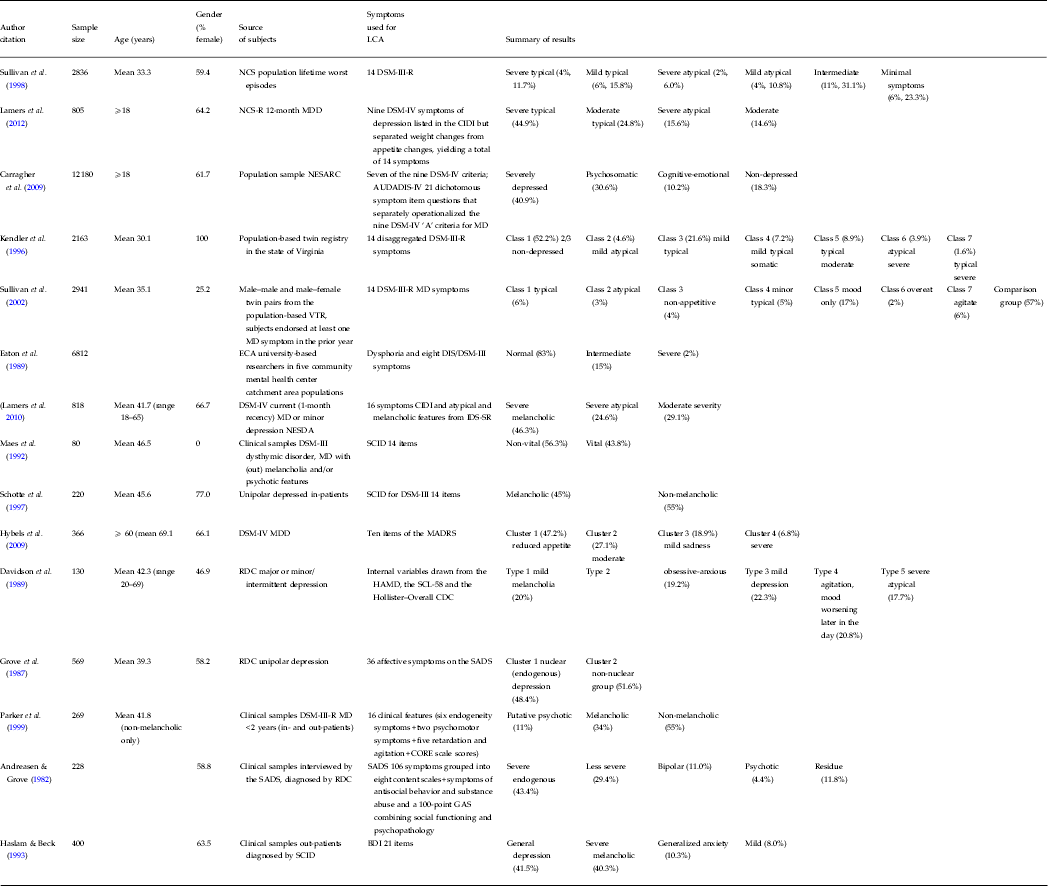
NCS, National Comorbidity Survey; NCS-R, National Comorbidity Survey Replication; MDD, major depressive disorder; NESARC, National Epidemiologic Survey on Alcohol and Related Conditions; AUDADIS-IV, Alcohol Use Disorder and Associated Disabilities Interview Schedule-IV; MADRS, Montgomery–Asberg Depression Rating Scale; MD, major depression; VTR, Virginia Twin Registry; ECA, Epidemiologic Catchment Area; DIS, Diagnostic Interview Schedule; IDS-SR, Inventory of Depressive Symptomatology, Self-Report; RDC, Research Diagnostic Criteria; HAMD, Hamilton Depression Rating Scale; SCL-58, Symptom Checklist-58; CDC, Composite Diagnostic Checklist; SADS, Schedule for Affective Disorders and Schizophrenia; GAS; Global Assessment Scale; BDI, Beck Depression Inventory.
The current study aimed to identify subtypes of depression with LCA in a homogeneous sample of female Han Chinese with recurrent depression, recruited through clinical settings. The analysis was performed on three sets of items: (i) the nine DSM-IV criteria for MD; (ii) 14 items representing the disaggregated DSM-IV criteria (i.e. increased and decreased appetite and weight, insomnia or hypersomnia, and psychomotor agitation or retardation); and (iii) 27 items consisting of detailed assessments of DSM-IV criteria and symptoms of melancholia, anxiety and Beck's cognitive trio (Beck & Alford, Reference Beck and Alford2008; Wang et al. Reference Wang, Liu, Shi, Gao, Liu, Li, Zhang, Wang, Zhang, Tao, Gao, Li, Wang, Lv, Jiang, Wang, Jia, Zhang, Lu, Li, Li, Hu, Ning, Li, Sun, Liu, Zhang, Ha, Tian, Meng, Hu, Chen, Deng, Huang, Wu, Li, Fang, Pan, Hong, Gao, Li, Yang, Chen, Liu, Cai, Dong, Mei, Shen, Pan, Liu, Wang, Tan, Flint and Kendler2013). We then attempted to validate the identified classes by examining other depression-related clinical features, co-morbidities and environmental risk factors.
Method
Data for the present study were drawn from the China, Oxford and VCU Experimental Research on Genetic Epidemiology (CONVERGE) study of major depressive disorder (MD). The analysis was based on a total of 6008 cases recruited from 57 mental health centers and psychiatric departments of general medical hospitals in 45 cities in 23 provinces. The case subjects were initially screened based on recruitment criteria obtained from their medical records or by asking patients directly. During the interview after the initial screening, patients’ symptom responses were used to validate the diagnoses. Patients who did not fulfill the DSM-IV MD inclusion criteria and where there was no evidence that these criteria were met from the medical records were excluded from the study.
The project uses samples collected for a molecular genetic study of MD. Given evidence that the genetic effects on MD are different between men and women (Kendler et al. Reference Kendler, Gardner, Neale and Prescott2001), to control for this known genetic heterogeneity we collected data on female participants only, with four Han Chinese grandparents. Cases were excluded if they had a pre-existing history of bipolar disorder, psychosis or mental retardation. Cases were aged between 30 and 60 years, had two or more episodes of MD with the first episode occurring between aged 14 and 50 years, and had not abused drugs or alcohol before their first episode.
The subjects were interviewed for an average of 2 h using a computerized assessment system. The interviewers, who were postgraduate medical students, junior psychiatrists or senior nurses, were trained by the CONVERGE team for a minimum of 1 week. The interview includes assessment of psychopathology, demographic and personal characteristics, and psychosocial functioning. Interviews were tape-recorded and a proportion of them were listened to by the trained editors, who provided feedback on their quality. The study protocol was approved centrally by the Ethical Review Board of Oxford University and the ethics committees in participating hospitals in China.
Measures
The diagnoses of depressive disorders (dysthymia and MD) were established with the CIDI (WHO lifetime version 2.1; Chinese version), which classifies diagnoses according to DSM-IV criteria. The CIDI was supplemented by items from the SCID-P (Spitzer et al. Reference Spitzer, Williams and Gibbon1987) and by items used in the Virginia Adult Twin Studies of Psychiatric and Substance Use Disorders (VATSPSUD) interview (Kendler & Prescott, Reference Kendler and Prescott2006). The interview was originally translated into Mandarin by a team of psychiatrists in Shanghai Mental Health Center, with the translation reviewed and modified by members of the CONVERGE team.
All interview sections were fully computerized into a bilingual system of Mandarin and English developed in-house in Oxford, called SysQ. Skip patterns were built into SysQ. Interviews were administered by trained interviewers and entered offline in real time onto SysQ, which was installed on laptops. Once an interview was completed, a backup file containing all the previously entered interview data could be generated with a database compatible format. The backup file together with audio record of the entire interview was uploaded to a designated server, currently maintained in Beijing by a service provider. All the uploaded files in the Beijing server were then transferred to an Oxford server quarterly. The diagnoses of MD were based on information collected in the medical records and DSM-IV symptoms during the worst episode.
Statistical methods
LCA was performed to examine the topologies of MD symptoms in three datasets. The first set of items comprised the nine DSM-IV MD A criteria. Each item was scored simply as present or absent. The second set of data included 14 items that represented the disaggregated DSM-IV criteria that expanded on three bidirectional criteria of the nine DSM-IV MD A criteria. The final dataset included all 27 unconditional items in the MD interview section that were used to determine the nine DSM-IV MD A criteria, along with several items that assessed criteria for melancholia and the two symptoms of Beck's cognitive trio (helplessness and hopelessness) not included in the DSM-IV criteria.
Mplus (Muthén & Muthén, Reference Muthén and Muthén1998–2010) was used for running the LCA (McCutcheon, Reference McCutcheon1987). Several criteria were used to guide the decision on the number of classes in mixture modeling, including the Akaike Information Criterion (AIC; Akaike, Reference Akaike1987), the Bayesian Information Criterion (BIC; Schwarz, Reference Schwarz1978), the sample size-adjusted BIC, the Vuong–Lo–Mendell–Rubin (VLMR) test (requested using TECH 11 in Mplus) and the bootstrapped parametric likelihood ratio test (LRT; requested using TECH 14 in Mplus). Both tests compared the model of the currently chosen number of classes (K) to a model of K – 1 classes. The analysis was performed by fitting a one-class model and gradually increasing the number of classes one at a time for model comparison, setting a random starting value arbitrarily from 500 to 100. We used a range of random start values to confirm that we had reached the true minimum.
Results
Analysis of the nine DSM-IV MD criteria
For an LCA on the nine DSM-IV criteria for MD, both the BIC and the sample size-adjusted BIC reached a low peak for the three-class solution (see Fig. 1). Additionally, the VLMR test and the bootstrapped parametric LRT both support a three-class solution. Table 2 depicts the resulting class membership and the item endorsement frequencies for this three-class solution. Class 1 was the largest class, constituting 85.6% of the cases, and was characterized by high endorsement on all criteria. We called this the ‘severe’ class. Class 2 was labeled ‘non-suicidal’ (8.0%), as it had zero endorsement on suicidal ideation (SI) and low endorsement of the worthlessness/guilt criterion. Class 3 was labeled ‘moderate’ (6.4%) because of a symptom pattern reflecting lower endorsement frequencies for all nine criteria. We examined the informativeness of the individual criteria for the LCA by examining their Cramér's V statistic (Table 2). Anhedonia, depressed mood and sleep changes were especially uninformative, probably because of their very high overall endorsement in our sample. The three most influential criteria for this LCA were psychomotor changes, SI and worthlessness/guilt.

Fig. 1. Plots of the Akaike Information Criterion (AIC), the Bayesian Information Criterion (BIC) and the sample size-adjusted BIC for the 9-, 14- and 27-item latent class analyses (LCAs).
Table 2. Item endorsement frequencies and performance on validators for the three-class LCA solution for nine DSM-IV MD A criteria a

LCA, Latent class analysis; MD, major depression.
a For binary external validators, frequencies (and standard errors) are reported for each class, Cramér's V as the effect size and χ 2 and p values; for quantitative external validators, means (and standard errors) are reported for each class, along with η 2 as the effect size, and F and p values for the ANOVA.
* p < 0.005, ** p < 0.0005, otherwise p ⩾ 0.01.
Analysis of the 14 disaggregated DSM-IV MD criteria
For the LCA on the 14 items reflecting the disaggregated DSM-IV MD criteria (which expanded on the four bidirectional symptoms of sleep, appetite, weight and psychomotor changes), the information criteria plot did not show a clear ‘elbow’ (Fig. 1). The VLMR test and the bootstrapped parametric LRTs both suggested a four-class solution, whose class membership and the item endorsement frequencies are depicted in Table 3. By far the most common class (class 1), called ‘severe typical’ (70.4%), was characterized by the highest endorsements on all typical symptoms of depression. Class 2 was called ‘moderate typical’ (13.6%) and had lower endorsement rates for all typical depression symptoms, especially loss of appetite and weight and also psychomotor changes. Class 3 was characterized by low endorsement of symptoms of death or SI along with feelings of worthlessness/guilt, and was labeled ‘non-suicidal’ (8.7%). Class 4 was labeled ‘atypical’ (7.4%) because of its high endorsements on atypical neurovegetative symptoms of increased appetite and weight gain in addition to hypersomnia.
Table 3. Item endorsement frequencies for the four-class LCA solution for the 14-item disaggregated DSM-IV MD A criteria a

LCA, Latent class analysis; MD, major depression.
a For binary external validators, frequencies (and standard errors) are reported for each class, Cramér's V as the effect size and χ 2 and p values; for quantitative external validators, means (and standard errors) are reported for each class, along with η 2 as the effect size, and F and p values for the ANOVA.
* p < 0.01, ** p < 0.005, *** p < 0.001, **** p < 0.0005, otherwise p ⩾ 0.01.
The most informative items for these analyses were those describing typical and atypical weight and appetite changes, and feelings of worthlessness/guilt. Although also disaggregated, the psychomotor and sleep items were considerably less influential on class assignment.
Figure 2 shows the proportion classified into the 14-item four-class solutions coming from the individual classes from the nine-item three-class solution. All of the 14-item severe typical class came from the nine-item severe class, as did 92% of the 14-item atypical class. The two non-suicidal classes were moderately closely related, with 52% of the 14-item class coming from the nine-item class. The two moderate classes were even less closely inter-related as 52% of the 14-item moderate class came from the nine-item severe class and only 27% from the moderate class.

Fig. 2. Flow chart of subjects from the 9-, 14- and 27-item latent class analyses (LCAs). For example, of the subjects in the moderate typical class from the 14-item analysis, 52% came from the severe class, 21% from the non-suicidal class and 27% from the moderate class in the nine-item LCAs.
Analysis of all 27 assessed symptoms
For the LCA on the complete set of 27 items in our interview assessed about the worst lifetime episode of MD, the information criteria plot also did not show a clear ‘elbow’ (Fig. 1). The VLMR test and the bootstrapped parametric LRTs both suggested a six-class solution, whose class membership and the item endorsement frequencies are depicted in Table 4. Again, the most common class (class 1) was best described as ‘severe typical’ and was characterized by very high endorsement rates for typical depressive symptoms. Contrary to prior solutions, however, this class now represented only 39.6% of subjects. Class 2 represented ‘moderate typical’ depression (19.5%). Classes 3 and 4 were both characterized by low rates of SI and thoughts of death. However, compared to class 4 (10.9%), class 3 (17.0%) had relatively high rates of guilt, worthlessness and hopelessness. Therefore, we labeled group 3 as ‘low suicidal/high guilt’ and group 4 as ‘non-suicidal/low guilt’. Group 5 is clearly an ‘atypical’ group (7.3%), and group 6 has consistently lower endorsement rates across most symptoms than any other of the groups and is therefore labeled a ‘mild’ group.
Table 4. Item endorsement frequencies for the six-class LCA solution for all 27 symptoms independently assessed for the lifetime worst depressive episode a

LCA, Latent class analysis.
a For binary external validators, frequencies (and standard errors) are reported for each class, Cramér's V as the effect size and χ 2 and p values; for quantitative external validators, means (and standard errors) are reported for each class, along with η 2 as the effect size, and F and p values for the ANOVA.
* p < 0.005, ** p < 0.0005, otherwise p ⩾ 0.01.
The most informative items for this LCA reflected SI, classic depressive cognitive symptoms of worthlessness and hopelessness, atypical vegetative symptoms, especially increased appetite and weight gain, and trouble thinking and concentrating. Somewhat surprisingly, the melancholic symptoms of distinct mood quality and mood worse in the morning were, along with the main items of sad mood and loss of interest/pleasure, the least informative for our class assignments.
Figure 2 presents the relationship between the individuals making up the 14-item four-class and the 27-item six-class solutions. Of the many results presented there, the most notable are: (i) nearly all subjects (97%) in the 27-item severe typical class were also in the 14-item severe-typical class; (ii) most subjects (84%) in the 27-item atypical class were also so classified in the 14-item LCA; (iii) the moderate typical class was again moderately stable, with a much higher proportion in the 27-item solution (69%) deriving from the 14-item severe typical than moderate typical class (24%); (iv) a large proportion of the low-SI/high-guilt 27-item class (83%) also came from the 14-item severe class; (v) the non-suicidal/low-guilt class was moderately stable over analyses, with 53% of those classified in the 27-item solution also so classified in the 14-item solution; and (vi) a majority of the new mild class in the 27-item solution derived from the moderate typical class in the 14-item solution.
External validation
Several external validators not used in the LCA were chosen to examine the classes identified by each of the three LCAs, including demographics, co-morbidities, clinical characteristics and environmental risk factors. For the three classes identified in the nine-item solution, the frequencies (for binary variables) and the means and standard deviations (for continuous variables) of several validators differed significantly across the three classes, mostly driven by the ‘severe’ class. This was characterized by samples of older age with higher numbers of episodes and higher neuroticism scores, in addition to higher frequencies of co-morbid anxiety disorders including panic, phobia and generalized anxiety disorder (GAD). Members of the severe class more frequently had a positive family history of depression. Notably, age at onset, body mass index (BMI) and a history of childhood sexual abuse (CSA) did not discriminate classes.
The pattern of validators differed substantially in the four-class solution with 14 items. The severe typical class had the highest rates of co-morbidity with panic disorder, but the atypical class stood out more with the highest rates of GAD, dysthymia and phobia, the highest rates of CSA, family history and stressful life events, the highest levels of neuroticism, the earliest age at onset, most and longest episodes, and the highest BMI. The non-suicidal class was characterized by low levels of anxiety disorder co-morbidity, relatively low neuroticism and the least and shortest episodes.
In general, the pattern of validation in the 27-class LCA bore a substantial resemblance to that seen with the 14-item solution. The atypical class was most unique with the highest levels of dysthymia, phobia, family history and CSA, the most frequent and longest duration of episodes, the youngest age and highest BMI and neuroticism levels. The severe typical class had the highest panic co-morbidity and was generally ‘second’ behind the atypical class on other severity markers. The mild and non-suicidal low-guilt classes were also the lowest on anxiety disorder co-morbidity, neuroticism, CSA, family history, and number and duration of depressive episodes.
Discussion
This study sought to determine empirically whether meaningful subtypes of depression could be identified in Han Chinese women with recurrent DSM-IV MD recruited from clinical settings throughout China. We performed an LCA on the (i) nine DSM-IV A criteria for MD, (ii) 14 items representing the disaggregated DSM-IV A criteria, and (iii) all 27 symptoms independently assessed in our interview for the worst lifetime depressive episode. The classes identified in each of the three sets of items were externally validated by depression-related clinical features, co-morbidities and environmental risk factors.
With respect to the identified subtypes, three major positive findings are noteworthy. First, in all three analyses, severity, as reflected by overall high item endorsements, played an important role in class assignment. In particular, we identified, in each LCA analysis, one severe group. This group consistently had other indices of clinical severity including high levels of co-morbidity, substantial environmental risk factors, and frequent and prolonged episodes. Second, each of our three analyses contained a class identified by low endorsement of items reflecting thoughts of death, suicide and guilt/worthlessness. In our validator analyses, this group was consistently more mildly ill and had relatively low rates of sexual abuse and stressful life events. Third, in our final two analyses that contained the relevant symptoms, we identified one class characterized by atypical vegetative symptoms, especially increased appetite and weight, and less prominently hypersomnia. This class stood out as particularly distinct in our validation analyses.
Two other findings are worthy of comment. First, we found no evidence for a specifically melancholic subtype of MD characterized by much higher endorsements of the key assessed symptoms (unreactive and distinct quality of mood, and mood worse in the morning) compared to the other classes. This was largely because of their overall high endorsement. For example, the key melancholic item ‘distinct quality to depressive mood’ was endorsed by more than 85% of subjects in all classes. Second, the utility of the individual symptoms in our LCA varied widely. The stem items for DSM-IV MD (sad mood and loss of interest/pleasure) had such high endorsements that they played a limited role in the LCAs. As noted, the melancholic items were of limited classificatory value. By contrast, atypical depressive symptoms and symptoms reflecting Beck's cognitive triad and SI were especially informative.
In relating our findings to the previous literature on LCA of depression (Table 1), we focused initially on the studies that used DSM criteria. One prior study used the nine main DSM MD criteria (Eaton et al. Reference Eaton, Dryman, Sorenson and McCutcheon1989) in an epidemiological sample. Broadly congruent to our findings, they identified two potential clinical classes, differing only on severity. They did not find a non-suicidal class. We identified five studies that examined disaggregated DSM criteria and were broadly comparable to our 14-item analysis (Kendler et al. Reference Kendler, Eaves, Walters, Neale, Heath and Kessler1996; Sullivan et al. Reference Sullivan, Kessler and Kendler1998, Reference Sullivan, Prescott and Kendler2002; Lamers et al. Reference Lamers, de Jonge, Nolen, Smit, Zitman, Beekman and Penninx2010, Reference Lamers, Burstein, He, Avenevoli, Angst and Merikangas2012). All of these studies identified at least one atypical subgroup. However, the frequency of atypical cases was greater in the most comparable Western clinical samples [15.6% in the National Comorbidity Survey Replication (NCS-R; Lamers et al. Reference Lamers, Burstein, He, Avenevoli, Angst and Merikangas2012) and the 24.6% in the the Netherlands Study of Depression and Anxiety (NESDA; Lamers et al. Reference Lamers, de Jonge, Nolen, Smit, Zitman, Beekman and Penninx2010)] than in our Han Chinese sample (~7.4%).
Several clinical features of the atypical class in our sample were similar to prior findings. This class had the highest BMI of any class, which was consistent with findings in other samples (Kendler et al. Reference Kendler, Eaves, Walters, Neale, Heath and Kessler1996; Sullivan et al. Reference Sullivan, Prescott and Kendler2002; Lamers et al. Reference Lamers, de Jonge, Nolen, Smit, Zitman, Beekman and Penninx2010, Reference Lamers, Burstein, He, Avenevoli, Angst and Merikangas2012). Congruent with findings from the NCS (Sullivan et al. Reference Sullivan, Kessler and Kendler1998), across all classes, atypical cases in our sample were the youngest with the earliest age of onset, the highest number of episodes and the longest episode duration. Patients in the atypical class had, in our sample, especially high rates of co-morbidity with GAD, any phobia and dysthymia. GAD, social phobia and simple phobia also had the highest prevalence rates in the severe atypical class in the NCS sample (Sullivan et al. Reference Sullivan, Kessler and Kendler1998). Finally, consistent with our findings, the atypical subtype of depression was associated with a higher rate of CSA than the non-atypical depression in the NCS sample (Matza et al. Reference Matza, Revicki, Davidson and Stewart2003). Of note, the fact that atypicality was associated with a higher rate of family history of MD and CSA suggested that it had both high genetic and high environmental predispositions.
A severe typical depressive class emerged when performing LCA on all three analyses. A similar class was identified in 12 of the 16 prior LCA studies of MD summarized in Table 1. In our 27-item analyses, our severe class had fairly high endorsements for melancholic items (distinct quality of mood 91%, lack of mood reactivity 88% and mood worse in the morning 68%) so could be considered a ‘severe, melancholic depression’ class. However, as noted earlier, these levels of melancholic symptoms were only modestly higher than those seen in nearly all of the remaining classes. Additionally, co-morbid panic disorder had the highest prevalence in the severe typical class in our study, which was consistent with findings in the NCS (Sullivan et al. Reference Sullivan, Kessler and Kendler1998; Lamers et al. Reference Lamers, Burstein, He, Avenevoli, Angst and Merikangas2012) but not with the NESDA sample, where panic had the highest prevalence in the atypical class (Lamers et al. Reference Lamers, de Jonge, Nolen, Smit, Zitman, Beekman and Penninx2010).
A class with low endorsements on symptoms of SI and guilt was seen in all three of our analyses. We were unable to locate a prior study of MD identifying such a non-suicidal class. Several characteristics of our sample might explain this unique finding, including ethnicity (Han Chinese), recurrence and single sex. Additionally, our sample size was much larger than any of the previously reported LCA studies; hence, we had more statistical power to identify classes compared with other studies.
Focusing on the 14-item analyses, the non-suicidal class had several noteworthy features including by far the lowest rates of co-morbidity with GAD, and the lowest rates of CSA and stressful life events. Of interest, when we added more cognitive depressive symptoms to the LCA, we were able to separate out the two low SI classes differing on their levels of worthlessness, guilt and hopelessness. The smaller of the two classes (non-suicidal/low guilt) had fairly low levels of symptoms indicative of Beck's cognitive model of depression and continued to have the lowest rates of any class of environmental traumas (Beck, Reference Beck1963, Reference Beck1967).
The epidemiology of suicide in Chinese women is unusual but we can only speculate about its possible relationship with our findings of a non-suicidal class of MD. In particular, China is one of the few countries where the suicide rate is higher in women than in men (Ji et al. Reference Ji, Kleinman and Becker2001; WHO, 2013) and is especially high in rural areas (Qin & Mortensen, Reference Qin and Mortensen2001; Phillips et al. Reference Phillips, Li and Zhang2002). Law & Liu (Reference Law and Liu2008) write thoughtfully of the place of suicide in Chinese culture and how this may be interacting with the changing role of women in modern China. They emphasize the role of powerlessness and shame in suicidal acts and especially in females as ‘a domestic strategy in a highly rigid patriarchal family setting’ (Law & Liu, Reference Law and Liu2008, p. 83). We note the strong association in our analyses between SI, worthlessness and CSA that suggests the possibility of two ‘paths’ to MD in Chinese women that are possibly shaped by personal experiences and cultural processes. Our work can no more than suggest possible directions for future study here.
In a prior article, we examined exploratory and confirmatory factor analysis on depressive symptoms in random split halves of this same sample (Li et al. Reference Li, Aggen, Shi, Gao, Li, Tao, Zhang, Wang, Gao, Yang, Liu, Li, Shi, Wang, Liu, Zhang, Du, Jiang, Shen, Zhang, Liang, Sun, Hu, Liu, Wang, Miao, Meng, Li, Huang, Li, Ha, Deng, Mei, Zhong, Gao, Sang, Zhang, Fang, Yu, Yang, Liu, Chen, Hong, Wu, Chen, Cai, Song, Pan, Dong, Pan, Zhang, Shen, Liu, Gu, Wang, Liu, Zhang, Flint and Kendler2013). It is useful to compare a variable-centered and a person-centered analysis of the same data. For example, LCA results with our nine items roughly paralleled our factor analysis. Factor analysis showed that the level of suicidal thoughts and guilt defined a symptom dimension whereas LCA showed that the non-suicidal patients form a distinct class. When the bidirectional neurovegetative symptoms were added in our 14-item analyses, in addition to a general depressive symptom factor, factor analysis identified two factors dominated by vegetative symptoms, one dominated by weight and appetite, and the other by sleep. In somewhat different ways, both LCA and factor analysis reflected the central role played by typical versus atypical neurovegetative symptoms in the typology of MD. When all the depressive symptoms were included, factor analysis identified five factors, which further supported the general depressive dimension, atypical dimension, suicide and cognitive impairment dimension, in addition to the bidirectional somatic and the newly emerged agitated depressive dimensions. There were some parallels but also some discrepancies between the methods, illustrated by the absence of any identified agitated depressive class. Overall, latent class and factor analysis are complementary approaches to understanding variation in clinical presentation at a person and symptom level.
Strengths and limitations
These results should be interpreted in the context of several potential methodological strengths and limitations. Four strengths are noteworthy. First, our phenotypic assessment was standardized and detailed with careful interview training and quality control. Second, our sample was very large. Third, in our design we sought to minimize heterogeneity so this sample was of a single sex with uniform ethnicity. Drug and alcohol abuse were vanishingly rare in our sample, reducing a further confound, and only 5% of the participants ever smoked. Fourth, the sample had a minimum age of 30 years and a mean age of 44.4 years (s.d. = 8.9), minimizing the proportion that might eventually develop bipolar illness.
Three major methodological limitations deserve comment. First, we only assessed symptoms for the self-appointed worst lifetime episode. In nearly all individuals, therefore, we relied on retrospective long-term recall, which might be biased by poor memory of past symptoms in a particular episode or conflated symptoms across multiple episodes to construct the symptoms of the index episode. Second, the results of the LCA are crucially dependent on the items analyzed. We used a standardized interview for the DSM-IV criteria in both aggregated and disaggregated form for our nine- and 14-item analyses but the other items added to form our final 27-item analysis reflected our own research interests. Furthermore, we could not include all the DSM-IV melancholic symptoms because some of them just assess the same symptoms as one of the A criteria at a more stringent level (e.g. melancholic criterion B.4 is just a severe version of criterion A.5). Including both items would cause estimation problems. Furthermore, we did not assess psychotic symptoms experienced during the depressive episode. Our results might have differed had other symptoms been included in the analysis.
Third, it could be argued that our study was overpowered and that the differences we detected in the subgroups were modest. This is true in some but not all instances. For example, rate of panic disorder differ threefold among the three classes from the nine criteria analysis, and GAD nearly twofold.
Conclusions
We were able to replicate in a large sample of relatively severely ill Han Chinese women two of the key findings of prior LCAs of MD in Western samples (Table 1): that severity and neurovegetative atypicality were important differentiators for subtypes of depression. Furthermore, we identified what might be a new subtype of serious depression with minimal symptoms of SI and guilt/hopelessness. These subtypes were meaningfully different on a range of validators. However, their ultimate utility will be judged by future research including molecular genetic analyses that we have planned for this patient cohort.
Acknowledgments
This work was funded by the Wellcome Trust and by National Institute of Mental Health (NIMH) grant MH100549. The authors are part of the CONVERGE consortium and gratefully acknowledge the support of all partners in hospitals across China.
Declaration of Interest
None.