1. Introduction
Writing proficiency in a foreign language constitutes an indispensable instrument for both student mobility and access to the job market, particularly among STEM (science, technology, engineering, and mathematics) students in France (Taillefer, Reference Taillefer2004). At the same time, while the share of French students who specialize in disciplines other than languages and who have to take language classes as part of their initial training represents about 90% of all students countrywide, French universities tend to face various issues such as coping with student influx or heterogeneity. To deal with these challenges, innovative online and/or blended English courses are often implemented. It is precisely why the online guided autonomy language course under study was designed, informed by research findings from the fields of second language acquisition (SLA) and technology-mediated language learning.
This paper sets out to explore the potential of this online course to foster English as a foreign language (EFL) learning – and, more precisely, writing accuracy development in English – among French threshold-level (B1) learners.
In a previous study (Sarré, Grosbois & Brudermann, Reference Sarré, Grosbois and Brudermann2019), we examined the potential of a blended EFL course – meant for students majoring in the humanities – to address the same issue. More specifically, we explored the effects of corrective feedback (CF) strategies on interlanguage (IL) development for the online component of a blended EFL course we had designed and implemented. We analysed and compared the effect of different types of online human-tutor CF on the learners’ writing accuracy. Our results showed that the use of online human-tutor CF seems to have acted as a lever to foster qualitative progression in most of the study participants’ written productions. We also came to the conclusion that unfocused indirect CF (that is to say, feedback on all error types through the provision of metalinguistic comments on the nature of errors made) combined with extra computer-mediated micro-tasks was the most efficient CF type to promote accuracy development in our context.
Based on these results, we set out to further explore the effects of this specific CF type on learners’ writing accuracy in an online EFL course that we created for freshmen STEM students by focusing on the impact of this specific CF strategy on error types. Moreover, in the online EFL course under study, this type of CF is computer-assisted: online CF provision by a human tutor is based on specific canned CF messages, since addressing a large sample of EFL learners and meeting their needs also implied relying at least partly on automated tools, to which teachers could delegate some of their prerogatives so as to devote a reasonable amount of time reviewing the students’ written productions. The medium of feedback provision thus slightly differs between the two studies: free online CF by a human tutor in the first case and online CF by a human tutor based on predetermined responses to common second language (L2) errors in the current study. We thus intend to further investigate the benefits of CF provision in computer-assisted language learning (CALL) settings, given that more empirical research is needed regarding “the role that technology plays in the provision and effectiveness of feedback” (Nassaji & Kartchava, Reference Nassaji and Kartchava2017: 198), and that, because of the differences between CALL and non-CALL environments, a key element of L2 acquisition such as CF may reveal different results in CALL contexts (Amiryousefi & Geld, Reference Amiryousefi and Geld2021).
In this paper, the literature will first be reviewed and background information on the online EFL course provided. The methods of the study will then be examined. Finally, the results will be analysed and discussed.
2. Literature review
2.1 The interlanguage hypothesis and corrective feedback
From a cognitive point of view, the process of L2 acquisition is a hypothetical activity whose aim is to build and develop an unstable system – the learner’s interlanguage – with continual restructurings that derive from the efforts put forward by the learner to get closer and closer to the model of the target language. L2 errors are therefore fundamental in so far as they are not only inevitable but also a necessary part of the language learning process (Corder, Reference Corder1967). One problem that arises within the IL hypothesis framework when dealing with foreign language (FL) accuracy development relates to the way large groups of heterogeneous student populations with unique idiosyncratic dialects should/could be provided with pedagogical guidance in order to lead them to further improve their FL writing.
This difficulty goes hand in hand with the type of CF to consider to foster FL writing accuracy development among students, because if the usefulness of FL CF is now beyond a doubt, research still has not reached a consensus as to the most effective type of CF to consider to promote accuracy development (Ellis, Sheen, Murakami & Takashima, Reference Ellis, Sheen, Murakami and Takashima2008).
This is why, following our previous study in which we highlighted that unfocused indirect CF (through the provision of metalinguistic comments on the nature of errors) combined with extra computer-mediated micro-tasks was able to spark L2 accuracy development in a blended learning course in the French context, our intention was to go further by testing out this CF type with a larger sample of French learners and to look into the evolution of error rates on an individual basis in order to (a) check whether we were able to confirm our previous results in a different context and among a larger sample of study participants and (b) analyse how IL develops over time on an individual basis.
2.2 Technology-mediated corrective feedback
The idea that automated tools could support L2 pedagogical practices has been paid much attention (see, for instance, Shermis & Burstein, Reference Shermis and Burstein2013). When it comes to CF on writing tasks, technology-mediated environments also offer new opportunities that range from CF provided by a human tutor via technologies – some researchers explore e-feedback given by teachers, not machines, in ESL/EFL courses (Chong, Reference Chong2019: 1090) – to CF provided by technology. In the latter case, automated writing evaluation (AWE) systems are, for instance, used to assess word-processed essays. AWE systems make the most of recent error detection technology and scoring engines and are able to provide machine-generated direct (explicit) and indirect (implicit) CF.
Research in AWE, and automated written corrective feedback (AWCF) (Ranalli, Reference Ranalli2018), has shown that the accuracy of machine-generated CF varies with error types (Feng, Saricaoglu & Chukharev-Hudilainen, Reference Feng, Saricaoglu and Chukharev-Hudilainen2016) and that there is no clear consensus about the correlation level between computer-generated scores and human scores (Heift & Hegelheimer, Reference Heift, Hegelheimer, Nassaji and Kartchava2017), although it should be noted that some of these systems are now also able to provide CF beyond grammatical correctness (Chukharev-Hudilainen & Saricaoglu, Reference Chukharev-Hudilainen and Saricaoglu2016). Mixed results have also been obtained when investigating the impact of AWCF on learner performance, and there is very little evidence to date that it impacts learner writing positively in CALL settings (Stevenson & Phakiti, Reference Stevenson and Phakiti2014) and, more generally, in non-CALL settings (Warschauer & Grimes, Reference Warschauer and Grimes2008). The specific characteristics of AWCF compared with human-tutor-provided CF have been highlighted and seem to point to a less efficient option (Ranalli, Reference Ranalli2018).
Although the use of AWE systems may seem appealing to address issues related to individualized instruction and the expansion of educational opportunities in EFL at the university level, such tools also pose a number of challenges, as shown by Saricaoglu (Reference Saricaoglu2019), and may limit student creativity and cultivate mechanical writing styles (Rich, Reference Rich2012). Another objection to automated evaluation systems is that they lack human sensitivity (Hearst, Reference Hearst2000).
Research also suggests that situational factors such as task design and implementation (Ziegler, Reference Ziegler2016a) and feedback delivery style (Amiryousefi, Reference Amiryousefi2016; Amiryousefi & Geld, Reference Amiryousefi and Geld2021) can impact students’ performance in non-CALL as well as in CALL environments, possibly in different ways. In this respect, if some researchers have highlighted the efficacy of CF in computer-mediated environments and the absence of significant differences with face-to-face CF efficacy (Li, Reference Li2010), others claim a small advantage for computer-mediated communication interaction, including feedback provision, on written production accuracy (Ziegler, Reference Ziegler2016b). Further investigating the link between feedback and its influence on students’ performance in CALL settings is thus crucial.
In our EFL course, online human-tutor CF provision is combined with online automated CF and coupled with computer-mediated micro-tasks that offer learners immediate computer-generated feedback. We hypothesize that this semi-automated CF could circumvent the challenges inherent to automated systems, alleviate teachers’ tasks, and lead students to improve their FL writing accuracy.
2.3 Corrective feedback: From theory to practice
The underlying theoretical principles of the CF strategies used in this study stem from a number of previously published studies. As “[l]earners are more likely to notice a correction if the strategy is explicit in nature” (Ellis, Reference Ellis, Nassaji and Kartchava2017: 10), CF explicitness impacts both learner noticing (which turns input into intake) and consequently CF efficiency: “The more explicit the written CF is, the more improved the accuracy is likely to be” (Guo, Reference Guo2015: 103). It should be noted that CF explicitness varies greatly on a continuum ranging from the underlining of errors (the least explicit form of CF) to the provision of the correct form (the most explicit form of CF). In our study, an explicit type of feedback (in the form of metalinguistic comments on the nature of errors, combined with extra micro-tasks to complete) was provided to draw students’ attention to linguistic elements in order to encourage attention to input and theoretically promote FL writing accuracy development. In addition, attention – that is, detection of linguistic input – “is crucial for learning” (Mackey, Reference Mackey and DeKeyser2007: 96). In a task-based language learning and teaching curriculum, the post-task phase should therefore encourage learners to (a) notice the gap between what they are able to produce on their own and the forms expected in the target language and (b) complete form-focused micro-tasks in order to draw their attention to their own difficulties (Robinson, Reference Robinson2002).
As such, the CF strategy that was implemented in this study was provided as follows: in the post-task phase, the students’ errors were highlighted (to foster attention) by tutors who also provided students with semi-automated individualized revision sheets containing highlighting of the errors made and metalinguistic comments on their nature as well as links to corresponding micro-tasks to further enable them to reflect upon their errors. Learners were then invited to self-revise their written productions.
3. From epistemological considerations to practical educational solutions
The course was designed along the following principles: task-based scenarios dealing with various scientific and societal topics were designed. Each scenario offered written and audio/video documents, self-assessment comprehension exercises, and selected additional readings, to have students focus on forms/grammatical structures, and notice given lexical items and handle ideas, which could prove useful for the subsequent oral and written production tasks they also had to submit.
The course spanned one semester only, due to institutional constraints and budget limitations. During the course, each student had to submit two online written tasks (cf. Appendix for an example), along with a number of other assignments. Each year, between 250 and 300 learners were enrolled in the course; the objective set by the institution was to have them reach B1 level in English by the end of the course.
As one of the objectives of this online course was to promote accuracy development in L2 written tasks, online linguistic CF was provided to guide the students and, to do so, the course was delivered using the following tools:
-
1. An online resource centre and an online platform where students could retrieve the tasks to be completed were set up (see Figure 1, frame #1 and left-hand side of frame #2);
Figure 1. Schematization of the computer-assisted unfocused indirect CF system
-
2. A web application was also developed to allow learners to write, edit, and eventually submit their essays (see Figure 1, right-hand side of frame #2).
The guided autonomy environment therefore possibly promoted the study participants’ “self-regulation” (Wenden, Reference Wenden1998: 519) – that is, their ability to take control of their learning process and procedures – since, as mentioned by Dickinson (Reference Dickinson1995), getting involved in one’s learning process favours both autonomy and motivation.
Tutors were equipped with personal working spaces, where the students’ written tasks could be retrieved and revised (see Figure 1, frame #3). Within this application, whenever tutors opened a submitted production, they could pinpoint the students’ errors directly in their productions by editing the students’ texts in the computer system. This system allows tutors to both highlight errors in students’ written productions and provide CF. What is specific about this explicit CF system is that every time a tutor highlights an error, a master file pops up automatically and the tutor can click on the generic category of the encountered error (see section 5.2 for more details regarding the way the master file and its underlying typology of errors works). As a result, the encoded comments – which are associated with the specific error – are automatically pasted in the students’ revision sheets. These comments systematically comprise explanations related to the student’s errors and a list of links to the online resource centre (see Figure 1, frame #1), where the student can find additional self-correcting micro-tasks. Besides, tutors can edit the revision sheets to adjust the comments, if needed. Students were then invited to revise their productions by taking into account their tutors’ comments displayed in their revision sheets. Unlike natural language processing systems or other methods such as statistical modelling, machine learning, or artificial intelligence, this system relies mainly on a human component. It is therefore likely to tone down the lack of human sensitivity argument. However, in this study, to ensure (as far as possible) data consistency and robustness of the results, the tutors were asked not to edit the canned messages. Moreover, in line with the underlying principle of autonomy sustaining the course – which was supposed to have the study participants take responsibility for their own learning – learner repair following feedback was not monitored, contrary to a previous study that was implemented in the very same course and that yielded conclusive results for L2 accuracy development (Brudermann, Reference Brudermann2013).
This CF assumes that a pedagogical system liable to empower students and encourage their active participation in their learning processes should foster L2 accuracy (see Figure 1, frame #4).
4. Research objective and research questions
The computer-assisted unfocused indirect CF described above was given to French learners enrolled in an online EFL course specifically designed for them from 2014 to 2019. Our research objective is to look into its potential to foster EFL writing accuracy on a large sample of French learners, thus following up on our previous study (Sarré et al., Reference Sarré, Grosbois and Brudermann2019). In this study, we had concluded that our experiment would benefit from being carried out on a larger cohort, as 93 students were then split into seven groups who were given different types of CF in the original study. More precisely, we now also aim to explore the evolution of error rates per error type when this CF type is provided, as we had also previously come to the conclusion that looking into the evolution of error rates on an individual basis could certainly provide valuable information about L2 learners’ IL development.
This study therefore seeks to explore the following two research questions:
-
1. What error types are the most frequent among the large cohorts of students who took part in this study?
-
2. Can an evolution of error rate per error type be detected when this CF type is applied? In other words, is the impact of this CF type more noticeable on certain error types?
5. Method
5.1 Participants
During five academic years (i.e. 2014–2015, 2015–2016, 2016–2017, 2017–2018, and 2018–2019), the online EFL course was experimented with different cohorts of freshmen STEM learners. The students were not the same over the five academic years, as the course was designed to target freshmen students only (institutional demand) with a level equivalent to A2 in English. However, the general context and the public were very similar in the five years of the study:
-
Both the English course and the written tasks remained unchanged during the five years of the study. All study participants also went through the same assignments. The tasks under study were handed in at the beginning (Task 1) and at the end (Task 2) of the semester.
-
Over the five academic years, the same tutors provided the online CF. They had also all been trained to do so for reliability’s sake, and because the whole project was a team effort with institutional support.
Table 1 provides background information regarding the study participants and the general organization of the course and indicates that 1,150 students took part in the study between 2014 and 2019 and that 1,986 written tasks were completed during the same period. In this study, the general completion rate is rather high as 1,986 out of 2,300 expected tasks were actually collected (i.e. 86.34%). These tasks (150 words long, level B1) were compiled into a corpus and further analysed.
Table 1. Background information regarding the general organization of the course

5.2 Corpus building: Data collection
The web application of the course (see Figure 1) comes with a tracking device that can store, compute, and extract predefined information in a database. Using these tools, errors could be monitored, quantified, and followed up. To do so, the master file we previously referred to was relied upon (cf. Figure 2).
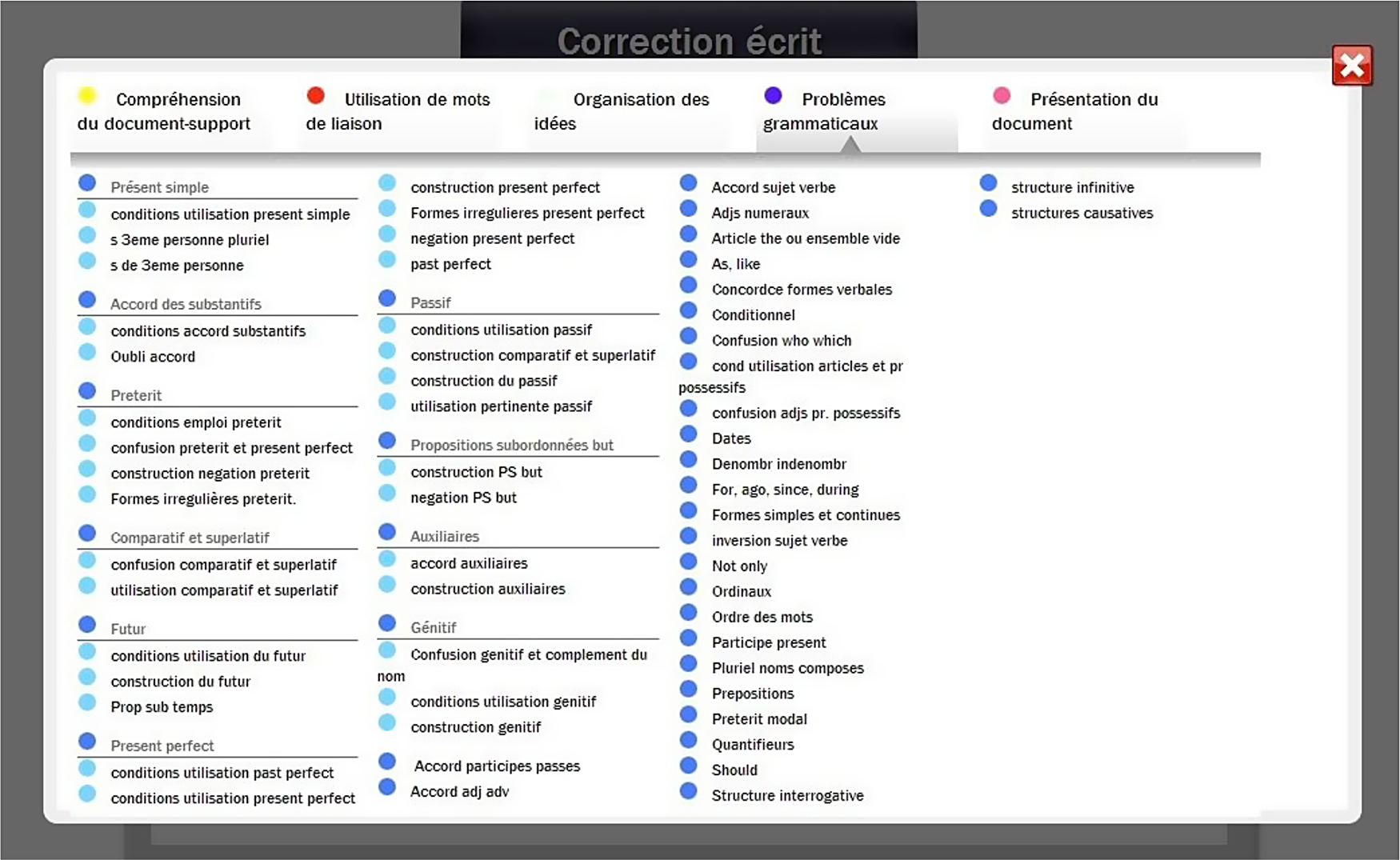
Figure 2. Screenshot of the master file and tagging process
The master file is a built-in tool that was incorporated in the submission/revision application we previously presented. To devise the master file – that is, a collection of ready-made pedagogical comments and resources (micro-tasks) associated with a certain number of recurring errors – we relied upon the Louvain typology of errors designed to carry out computer-aided error analysis (Dagneaux, Denness & Granger, Reference Dagneaux, Denness and Granger1998) in learner corpora. The Louvain typology of errors was relevant in our study as it focuses on learners of English from several mother-tongue backgrounds, including French. The error types highlighted in the Louvain typology of errors fall into an error taxonomy comprising 55 error tags (Dagneaux et al., Reference Dagneaux, Denness, Granger, Meunier, Neff and Thewissen2008) and were used in this study to tally and classify learners’ individual errors in their essays, assess the frequencies of occurrence of their L2 errors, and to identify the grey areas for which specific pedagogical resources would further have to be devised to address them.
However, while the 55 tags were used in this study for research purposes, it was not realistic to use them for pedagogical purposes (i.e. to provide students with CF), as their headings, for instance, “GVT” (Grammar, Verb, Tense) or “GVAUX” (Grammar, Verb, Auxiliaries), were bound to be unclear and difficult to interpret for students and not convenient to use for tutors. To circumvent this difficulty, we devised a correlation table (Table 2) between the tags from the Louvain typology and a list of categories translating their meaning in pedagogical terms, which was easier to comprehend by both tutors and learners.
Table 2. Excerpt from the correlation table (Research tagging – Louvain categories/Pedagogical tagging)

This list of categories was included in the master file (Figure 2), making the reorganization of the visual tagging more convenient for pedagogical purposes. As such, tutors could click on the translated headings in the master file to revise the students’ productions. Students could benefit from feedback messages comprising comments and micro-tasks Footnote 1 associated with the highlighted errors. However, whenever a tutor clicked on an item in the master file, even though the computer programme displayed the aforementioned comments and associated micro-tasks, the computer system stored the associated tag taken from the Louvain typology in its database to track and document the errors that appeared and determine whether some of them were recurring. The algorithm of the master file was thus designed to be sustainable for both research and teaching purposes.
5.3 Study rationale
To begin with, in order to find out what the most common error types were among the participants (N = 1,150) throughout the period under study (research question #1), the computer programme was relied upon. As previously mentioned, with this system, whenever a tutor highlighted an error, the Louvain corpus tag associated with the error message sent to the student was automatically stored in the database, allowing the system to perform calculations both on an individual and collective basis. At the individual level, the computer system thus tracked the study participants’ errors on a per task basis; this system was necessary for the tutors to offer individualized CF (i.e. to provide personal revision sheets and annotated productions). At the collective level, every year, all the highlighted errors were automatically added up by the computer system and sorted by error type (tags from the Louvain typology), in descending order. We then manually computed the sum total of the data collected from the five cohorts of study participants during the period considered and classified it by error type in descending order (Table 3). Table 3 thus presents data computed over a five-year period and provides final results.
Table 3. Breakdown of the error tags brought to the students’ attention (N = 1,150) over the 2014–2015 to 2018–2019 period (all written tasks included)

Then, in order to assess the study participants’ IL development – and answer research question #2: Can an evolution of error rate per error type be detected when this CF type is applied? – the data collected by the computer system also allowed the tracking of errors made in both the first and second writing tasks, at individual and collective levels. As the tracking device functioned on a per task basis, whenever the system monitored similar error tags in two successive tasks submitted by the same student, this data was stored in the database of the system. This is what we call recurring errors; that is, errors made in both the first and second writing tasks by the same student. For each recurring error highlighted, a recurrence rate was automatically computed by the programme, using the following formula:

In other words, the recurrence rate is the number of errors of a specific type found in a student’s task#2 minus the number of errors of the same specific type found in the same student’s task#1, divided by the number of errors of the same specific type found in the same student’s task#1, then multiplied by 100.
At the collective level, the total number of recurring errors made for each specific error type in both Tasks 1 and 2 was calculated, and an overall recurrence rate per error type was then calculated (using the above formula). At the individual level, for each recurring error type, the individual recurrence rates were automatically added up by the computer system and divided by the number of students concerned by the recurring error in question.
6. Results
Regarding the most common error types among the study participants (research question #1), Table 3 shows that 18,269 errors were highlighted in a corpus comprising 1,986 written tasks. This corresponds to an average of 9.19 errors per task (18,269/1,986).
It shows that, out of a typology that comprises 55 error types, 31 error types were identified in this study. Among these, it appears that, in quantitative terms, lexical issues come first with the top three error types (FS Form Spelling, LS Lexical Single, LP Lexical Phrase), which amount to more than 38% of all the errors highlighted over the period considered. Overall, lexical errors in our corpus amount to just over 46% of errors, and grammar errors (comprising lexico-grammatical errors) amount to just over 26%.
The collected data also reveal that the 10 most quantitatively important error types listed in Table 3 – that is, under a third of all the 31 error types that were identified – represent more than 77% of all the errors highlighted in the corpus. This suggests that a fairly limited number of error types were truly problematic for the participants in this study.
Regarding the study participants’ recurring errors (research question #2), Table 4 displays, for each error type: the number of students (out of 1,150) whose written productions contained recurring errors, the number of recurring errors made in Task 1 and in Task 2, the total number of recurring errors made (column 6; i.e. column 4 + column 5), and the average overall recurrence rate (column 7; i.e. at the collective level, using the above formula and data from columns 4 and 5).
Table 4. Breakdown of the number of recurring errors and average overall recurrence rate (at the collective level)

Regarding the study participants’ recurring errors, Table 4 shows that 7,483 such errors were highlighted, corresponding to 24 different error tags. This therefore suggests that, in this study, most errors – that is, 10,756 errors (18,269 – 7,483) representing 58.87% of the total number of errors tagged – were isolated errors, meaning errors that did not reappear in the students’ subsequent written productions after the CF they had received. This tends to show that the CF provided might have had a positive impact on the accuracy of the students’ written productions for a wide majority of error types.
Table 4 also highlights that the 12 most commonly recurring error categories (all data taken together) represent 95% ((7,129 x 100)/7,483) of all the recurring error types highlighted in the corpus. If we compare this with data from Table 3, we can see that 11 of the top 12 recurring errors from Table 4 are also part of the top 12 most frequent errors in Table 3. This suggests that these particular 11 error types constitute the ones for which further adjustments could/should be developed to help those having difficulties with them improve their writing accuracy. In addition, the average overall recurrence rate at the collective level seems encouraging, as exactly half of the recurring error types under study (i.e. 12) have a recurrence rate that is less than or equal to zero. This means that the number of errors made in Task 2 was smaller or identical to the number of errors made in Task 1, thus suggesting accuracy development. If we focus on the top 12 recurring errors, the proportion is similar, with five recurrence rates (out of 12) that are less than or equal to zero (that is to say, 42% of the top 12 recurring error types). However, it should be noted that our data were processed quantitatively (by comparing the total number of occurrences of a given error type in both Tasks 1 and 2) and that we did not explore how the erroneous forms from Task 1 were then used correctly in Task 2.
All in all, the data collected seem to yield encouraging results in terms of writing accuracy development, since, out of a typology that comprises 55 error types, 24 (i.e. 43.6%) were not relevant in this study and 12 error types in particular eventually reappeared in some of the observed students’ tasks with the CF system that was implemented. For the 19 remaining error types, the online CF system seems to have had a positive impact after one revision phase only.
Table 5 displays for each error type the number of students whose written productions contained recurring errors (column 3) and the average individual recurrence rate (column 4; i.e. at the individual level). This time, the recurrence rates were calculated by first (a) calculating individual recurrence rates for all students who made recurring errors in the two writing tasks and then by (b) dividing the total recurrence rate obtained per error type by the number of students who made the specific error.
Table 5. Average individual recurrence rate (at the individual level)

As shown in Table 5, all average individual recurrence rates per error type are either positive or equal to zero, which means that students systematically made more errors on average in the second writing task than in the first writing task. This of course mitigates the good results and positive impact of the CF provided on half of the recurring errors shown at the collective level in Table 4.
7. Discussion
In answer to research question #1 (What error types are the most frequent among the large cohorts of students who took part in this study?), the analysis of our corpus revealed that – contrary to what students usually ask for (cf. Brudermann, Reference Brudermann2018) – grammar issues do not come first, as lexical issues are the most recurring error types listed in Table 3 (followed by grammar issues). These results seem to go against some previously published research on EFL writing accuracy, which tends to show that grammar errors are usually the most frequent ones (Chuang & Nesi, Reference Chuang and Nesi2006; Dagneaux et al., Reference Dagneaux, Denness and Granger1998). At the same time, our results are in line with some other research (Granger, Reference Granger1998; Hamel & Milićević, Reference Hamel and Milićević2007; James, Reference James2013), as no clear consensus seems to have been reached on the most common error types in L2, probably due to the many factors that impact linguistic accuracy. It should be noted that in our task-based scenarios, the preparatory phase – that is, the self-correcting activities that the study participants were expected to carry out autonomously prior to the production tasks – was meant to allow for lexical noticing, analysis, and parsing. The fact that lexical errors are the most common error types in the production tasks therefore seems to suggest that the activities to be completed autonomously were actually not always (or not thoroughly) completed. In this respect, monitoring the completion rate of the comprehension tasks or making them compulsory could help refine the current results and better fathom the implications arising from what appears to be a situated element in our context.
However, since both categories account for over 77% of all errors made in our five-year study, they should certainly be given special attention in any L2 writing class. In particular, as the top 10 problem categories highlighted in the preliminary study (Sarré et al., Reference Sarré, Grosbois and Brudermann2019) are again almost all to be found in the top 12 problem categories of Tables 3 and 4 taken together, it appears that a very similar and limited type of error is truly problematic for our study participants (see the 12 error types in Table 4 amounting to 95% of all recurring errors found in the corpus) and that a high level of consistency between the different phases of the project can be noticed. It therefore seems important in the ongoing experiment to deal with these particular L2 issues by, for example, designing dedicated pedagogical resources targeting these specific error types, creating specific remedial materials, and readjusting the master file to allow tutors to offer more precise and thorough revision sheets regarding these specific errors. This effort could in turn inform both teacher education and curriculum development.
By tracking the amount of errors reappearing in two successive tasks (cf. Table 4) we were also able to answer research question #2 (Is the impact of this CF type more noticeable on certain error types?). It enabled us to assess the participating students’ IL development and, therefore, the potential of the online unfocused indirect CF system to foster EFL accuracy development. The collected data seem to indicate that – in line with the approach that was followed in our previous study (Sarré et al., Reference Sarré, Grosbois and Brudermann2019) – the IL orientation that was taken was also relevant in this project as encouraging results were obtained after the completion of two online tasks. It should nonetheless be noted that the scope of this study is limited, if we consider that only two written tasks per student were to be completed as part of the one-semester online course. In this respect, the organizational framework of the course represented a major limitation, since it didn’t make it possible to examine errors in two non-consecutive tasks.
In addition, this study has shown that, as students completed tasks, some errors – which we referred to as isolated errors – quickly disappeared from the students’ productions between the first and the second written task, after one revision only. Indeed, it should be noted that only 7,483 errors are recurring errors (out of a total of 18,269 errors), which means that almost 60% of errors are isolated. This tends to show that the CF provided probably had a positive impact on the participating students’ writing accuracy. This result is in line with previously published research that supports the positive impact of error-specific feedback provision through reactive focus on form (Choi, Reference Choi2016; Lado, Bowden, Stafford & Sanz, Reference Lado, Bowden, Stafford and Sanz.2014), as well as with studies showing that technology-mediated feedback provision can help sustain the revision process in L2 writing (Chong, Reference Chong2019; Heift, Reference Heift2001). In addition, the fact that lexical errors were the most common ones could also account for the overall positive impact of the CF provided, as previous research has shown that feedback on lexical errors is often more noticeable than that on other error types (Mackey & Goo, Reference Mackey, Goo and Mackey2007; Yang & Lyster, Reference Yang and Lyster2010).
Even though the limited time frame of the study did not allow us to identify whether these seemingly disappearing errors would come back after the completion of the second task, the current results suggest that, by leveraging students’ existing L2 knowledge, the CF system provided here pushed participants into drafting more accurate written productions in English.
8. Conclusion
The scope of this project was to investigate the evolution of the error rate per error type in L2 writing on an individual basis when learners benefit from unfocused indirect online computer-assisted CF. In this respect, this study helped gain a better understanding of the role that CF plays in the development of writing accuracy.
Concerning the impact of the CF system, the first results seemed to corroborate Van Beuningen’s (Reference Van Beuningen2010: 21) conclusion that “by offering learners opportunities to notice the gaps in their developing L2 systems, … and engage in metalinguistic reflection, written CF has the ability to foster SLA and to lead to accuracy development”. However, as the study that was conducted to observe the evolution of the students’ accuracy development in writing was set in a limited time frame (one-semester courses, 24-hour modules), it would also be interesting to implement the online unfocused indirect CF system we used in an annual course, as offering more regularity and more practice could possibly complement the current results. Such a new organizational framework would indeed lead students to further practice and test their FL hypotheses (Swain, Reference Swain and Lantolf2000) and allow us to gain further insight into the impact of time on the writing accuracy development process. This would also facilitate the tracking of learner repairs following the feedback given on more than two tasks.
Moreover, writing in an FL poses linguistic, cultural, and cognitive problems that vary with each individual, as stated by Hyland (Reference Hyland2003). In this respect, this study has other limitations: the general learning context as well as individual differences might have played a role, which was not evaluated, in the development of learners’ writing accuracy. Besides, the CF was provided on “surface-level” aspects (Paulson, Alexander & Armstrong, Reference Paulson, Alexander and Armstrong2007: 314), that is to say, targeting linguistic problems. Despite what some authors advocate (Storch, Reference Storch2018), social and personal factors (individual goals and beliefs, changing needs …) were not investigated in this study as it follows a more quantitative approach. Feedback on such aspects as argument structure, register, or textual complexity was not addressed either as the online CF focused exclusively on typological, lexical, and morphosyntactic aspects of the FL writing process.
This study has a number of implications in terms of pedagogical practice and language teacher education programmes. First, it suggests that lexical errors should not be overlooked as they can be the most common error types made by learners, but since the most common errors seem to cover a fairly limited number of categories (a dozen), these should probably be the ones teachers should focus on as a priority. In this respect, the potential of (online, physical, or hybrid) learning centres could, for instance, be harnessed to offer students activities particularly targeting the most recurring errors identified in this study. In addition, the CF type provided (unfocused indirect online CF by a human tutor using canned messages combined with additional computer-mediated micro-tasks) seems to be instrumental in the development of L2 learners’ writing accuracy. At the same time, our study results can inform language teacher education programmes regarding the use of digital tools by online human tutors for the development of FL accuracy and the issues related to online CF provision, since the use of the semi-automated CF (based on canned messages) seems to have fostered qualitative progression in some of the study participants’ written productions and, in so doing, to have held all its promises to meet the objectives it sought to pursue. However, another avenue for further research would be to explore the effect of the provision of canned versus adapted canned messages so as to investigate the impact of feedback delivery style on L2 accuracy development. Self-confrontation interviews could then also be carried out in order to better understand learners’ preferences and to adapt the provision of feedback, teacher training programmes, and practice in online settings accordingly.
Acknowledgements
The authors would like to thank the Centre for English Corpus Linguistics, Université Catholique de Louvain, for allowing them to use the Louvain error tagging editor. They are also grateful to the reviewers for their insightful comments.
Ethical statement
All authors listed in this article have made a significant contribution to the work reported, and all contributors to the research have been included. The authors certify that all data are accurate and representative of the research reported on in the paper. They declare that they are unaware of any conflict of interest.
Appendix
Example of a written production task
Write a 150-word email to the Editor in reaction to this reader’s opinion. Your email should be posted by December 10th.
➟ In line with the task-based orientation of the scenario, the editor, in this context, refers to the pedagogical team and the link actually points to the submission platform of the course; the second link points to a real online article, to be found at the following URL: https://cutt.ly/Hlu8thp
About the authors
Cédric Brudermann is a senior lecturer in English for specific purposes and instructional technology in the Faculty of Science and Engineering at Sorbonne Université, Paris, France. He is a member of the CeLiSo (Centre de Linguistique en Sorbonne) – EA 7332 research unit. His research interests include computer-assisted language learning, SLA, curriculum design, instructional technology, TBLT, and ESP.
Muriel Grosbois is a professor of English and applied linguistics at Conservatoire National des Arts et Métiers, Paris, France. She is a member of the FoAP (Formation et Apprentissages Professionnels, EA 7529) research unit and director of the Language Department. Her research focuses on language learning in a technology-enhanced context.
Cédric Sarré is a senior lecturer in the School of Education (INSPE de Paris) at Sorbonne Université. He is a member of the CeLiSo research unit. His research focuses on language learning technologies (computer-assisted language learning and computer-mediated communication). His research interests also include ESP didactics, peer interaction in L2 learning, and teacher education.
Author ORCIDs
Cédric Brudermann, https://orcid.org/0000-0003-2645-007X
Muriel Grosbois, https://orcid.org/0000-0003-2258-8733
Cédric Sarré, https://orcid.org/0000-0002-8548-2824