Borderline personality disorder (BPD) is a chronic, highly debilitating and lethal psychiatric condition, with an approximate prevalence of 1–2% of the population (Leichsenring, Leibing, Kruse, New, & Leweke, Reference Leichsenring, Leibing, Kruse, New and Leweke2011). BPD is heterogeneous, with 256 ways of meeting the DSM-defined diagnosis, such that patients could be mostly defined by more impulsive, affective, or interpersonal/identity-related symptoms. Patients can be distinguished by many clinical features, such as degree of suicidality and self-harm, comorbid personality disorder traits, attachment, or psychosocial dysfunction (Diamond et al., Reference Diamond, Levy, Clarkin, Fischer-Kern, Cain, Doering and Buchheim2014; Yun, Stern, Lenzenweger, & Tiersky, Reference Yun, Stern, Lenzenweger and Tiersky2013). Despite differences in presentation among patients with the diagnosis, treatments for BPD and their supporting clinical trials are generally not explicitly targeted to specific presentations.
Most head-to-head comparison of specialized treatments for BPD has found cognitive-behavioral and psychodynamic therapies to have similar outcomes (Cristea et al., Reference Cristea, Gentili, Cotet, Palomba, Barbui and Cuijpers2017; Keefe et al., Reference Keefe, McMain, McCarthy, Zilcha-Mano, Dinger, Sahin and Barber2019). While some therapies have demonstrated potentially unique benefits, such as transference-focused psychotherapy (TFP) improving attachment and mentalization (Keefe & DeRubeis, Reference Keefe and DeRubeis2019; Levy et al., Reference Levy, Meehan, Kelly, Reynoso, Weber, Clarkin and Kernberg2006) and dialectical behavior therapy (DBT; Linehan, Reference Linehan1993) improving coping behaviors that help prevent suicide attempts (Neacsiu, Rizvi, & Linehan, Reference Neacsiu, Rizvi and Linehan2010), generally there are few data recommending any particular approach over another.
Even assuming that there is no clearly superior BPD therapy, it is possible that particular treatments are a better fit to specific patients in terms of the mechanisms of the therapy or the patient's ability to use treatment interventions. Different therapies for BPD, while sharing some important characteristics (e.g., structure and attention to safety), posit different core deficits in BPD that relate to the interventions they provide. For example, the core role of emotional dysregulation in DBT's clinical theory (Crowell, Beauchaine, & Linehan, Reference Crowell, Beauchaine and Linehan2009) leads to a strong emotion focus in therapy including the prioritization of teaching concrete emotional regulation and distress tolerance skills and helping patients slow down and structure thinking about interpersonal situations. Alternatively, general psychiatric management (GPM; Gunderson, Reference Gunderson2016; Links, Bergmans, Novick, & LeGris, Reference Links, Bergmans, Novick and LeGris2009) views BPD as a disorder of dysregulated attachment (Levy, Reference Levy2005) and intolerance of being alone (Gunderson, Reference Gunderson2001), with difficulties in relationships and emotion regulation seen as downstream consequences. Interventions take place within a psychodynamic frame, focusing on validation and empathy, encouraging curiosity in feelings and their connections to (often attachment-related) situations and behaviors, clarifying maladaptive responses to feelings, and using both supportive and here-and-now focused interpretive interventions to help patients process feelings differently. Each treatment entails a distinguishable therapeutic milieu and tasks and goals of treatment that patients may find easier or harder to engage with or that may be better targeted to their particular causes for their psychopathology (Fisher, Reference Fisher2015; Hofmann, Curtiss, & McNally, Reference Hofmann, Curtiss and McNally2016).
Moderator research employing data from randomized clinical trials can help clinicians understand how specific, measurable differences between patients may inform which treatments they are probable to succeed in (Kraemer, Reference Kraemer2016). Moreover, combining information from multiple moderator variables may provide stronger and more robust predictions of which BPD treatment is most likely to promote positive outcomes for a given patient (Cohen & DeRubeis, Reference Cohen and DeRubeis2018; Delgadillo & Gonzalez Salas Duhne, Reference Delgadillo and Gonzalez Salas Duhne2020; Wallace, Frank, & Kraemer, Reference Wallace, Frank and Kraemer2013).
We examined the potential benefits of moderator-based treatment selection for enhancing BPD care using data from a randomized clinical trial (McMain, Guimond, Streiner, Cardish, & Links, Reference McMain, Guimond, Streiner, Cardish and Links2012; McMain et al., Reference McMain, Links, Gnam, Guimond, Cardish, Korman and Streiner2009) comparing 1 year's treatment with DBT or GPM for BPD patients. The original goal of the trial was to compare the effects of DBT (a gold standard, intensive BPD treatment) to robust expert care provided in the community, as represented by GPM, which reflected contemporaneous American Psychiatric Association recommendations for the treatment of BPD by general psychiatrists (American Psychiatric Association Practice Guidelines, 2001). While the trial hypothesized that DBT would have superior outcomes in suicidality and self-harm, at both treatment termination (McMain et al., Reference McMain, Links, Gnam, Guimond, Cardish, Korman and Streiner2009) and 2-year follow-up (McMain et al., Reference McMain, Guimond, Streiner, Cardish and Links2012) both treatments had statistically indistinguishable outcomes in all domains and patients were generally stabilized on high-risk behaviors. Our hypothesis was that baseline patient characteristics would moderate the degree to which patients evidence symptom relief within each specific treatment over follow-up. We then aimed to utilize information from several moderators in a combined moderator model, to estimate in a cross-validated manner how well a combined model might inform what treatment a patient would be recommended to receive to maximize their chance of long-term symptom relief.
Methods
Clinical sample
The parent trial for this study was a single-blind randomized clinical trial comparing two different 1-year manualized treatments for BPD. Treatment was offered at the Centre for Addiction and Mental Health and at St. Michael's Hospital, both University of Toronto teaching hospitals. The protocol was approved by each center's research ethics board (IRB codes 026-2014-23 and 2014s0263) and patients provided written informed consent prior to enrollment. Under the Canadian public health care system, participants did not pay for treatment.
For inclusion, patients between the ages of 18 and 60 years had to meet DSM-IV criteria for BPD based on the International Personality Disorder Examination (intraclass correlations for BPD symptoms ranged from 0.83 to 0.92). Patients also have had to have at least two episodes of suicidal or non-suicidal self-injurious episodes in the past 5 years, at least one of which had to occur within the 3 months preceding enrollment, and patients had to be fluent in English. Exclusion criteria included: DSM-IV diagnosis of psychotic disorder, bipolar I disorder, delirium, dementia, or mental retardation, or substance dependence in the preceding 30 days; having a medical condition that precluded taking psychiatric medication; and having a serious medical condition likely to cause hospitalization within the year. Gender was not an exclusion criterion although most patients were women (86.1%).
DBT and GPM were manualized therapies delivered by psychotherapists and psychiatrists with specific training and allegiance to each treatment. DBT was full-model, performed as manualized by Linehan (Reference Linehan1993), including all four modes of treatment (individual therapy, skills group, phone coaching, and therapist consultation group). DBT blends strategies from cognitive behavioral therapy, including psychoeducation, commitment, problem-solving, behavioral analysis, contingency management, and skills training techniques, with an emphasis on acceptance and validation techniques.
GPM, manualized for this trial (Links et al., Reference Links, Bergmans, Novick and LeGris2009), was originally conceptualized by John Gunderson and colleagues as a psychodynamically informed psychotherapy based on a model of BPD focusing on disturbed attachment relationships leading to emotional and relational dysregulation. Interventions include case management, psychoeducation about BPD, supportive psychodynamic interventions facilitating attachment, interpretive interventions encouraging making connections between thoughts/feelings/wishes and behaviors, and psychopharmacology targeted to specific symptoms. GPM is distinguished from TFP (Yeomans, Clarkin, & Kernberg, Reference Yeomans, Clarkin and Kernberg2015) in large part by its lack of focus on identifying and interpreting moment-to-moment relational dyads and associated affects emerging in the transference (the hallmark of TFP) and linking them to experiences outside of session, v. higher emphasis in GPM on case management, supportive interventions, and working in the positive transference (Gunderson, Reference Gunderson2016).
In DBT, patients met once a week each for group and individual sessions for a combined total of 3-h (plus 1-h of optional phone coaching), while in GPM patients met once a week for 1-hand therapists met in 1-h weekly consultation team. Ratings of taped sessions confirmed both adherence to each method and differentiation between the therapies (McMain et al., Reference McMain, Links, Gnam, Guimond, Cardish, Korman and Streiner2009). Overall use of psychopharmacology was indistinguishable between treatments (M = 1.8 medications DBT; 2.1 GPM). Additional information on the clinical sample, screening, randomization, treatments, and therapists can be found in the original trial publication (McMain et al., Reference McMain, Links, Gnam, Guimond, Cardish, Korman and Streiner2009).
Outcome and moderator variables
Outcome measure
Our primary outcome measure was the Global Severity Index (GSI) of the Symptom Checklist-90 (Derogatis & Unger, Reference Derogatis, Unger, Weiner and Craighead2010). We selected the GSI among study assessments because it reflects a broad assessment of psychopathology and dysfunction, and it evidenced a high variability in patient outcomes to be potentially explained by treatment moderators. Our focus was on the patient's long-term profile of response to treatment, consisting of outcomes assessed at treatment termination, 6-month, 12-month, 18-month, and 24-month follow-up. Utilizing all available measurements per patient, we calculated an area under the curve (AUC) for each patient. A lower AUC score for the GSI reflected that patients spent a relatively longer time with relatively less severe symptoms across the follow-up period of 2 years post-treatment. To be included in these analyses, a patient had to have completed at least two post-termination assessments. Out of 180 patients randomized to treatment, 156 (86.7%) provided enough outcome data to be included in the present analyses. Patients were followed regardless of whether they responded to therapy. There was no significant difference between the treatments in terms of the number of follow-up assessments attended after termination (DBT: M = 2.53, s.d. = 1.62; GPM: M = 2.88, s.d. = 1.44).
Moderator measures
Prior to engaging in any analyses, the authors selected 20 potential moderator variables. These variables include: (1) demographics/history (age, employment, education; childhood sexual and emotional abuse using the Childhood Trauma Questionnaire; Bernstein et al., Reference Bernstein, Stein, Newcomb, Walker, Pogge, Ahluvalia and Zule2003); (2) count of suicide and self-harm incidents (Linehan & Comtois, Reference Linehan and Comtois1997); (3) BPD-specific symptoms as measured by the Zanarini Rating Scale for BPD [interpersonal; cognitive-affective (merged due to high collinearity); impulsive; Zanarini et al., Reference Zanarini, Vujanovic, Parachini, Boulanger, Frankenburg and Hennen2003]; (4) general psychiatric symptoms (SCL-90 GSI; Derogatis, Reference Derogatis1992; Beck Depression Inventory; Beck, Steer, Ball, & Ranieri, Reference Beck, Steer, Ball and Ranieri1996; SCID-I diagnosed anxiety disorder; First, Gibbon, Spitzer, & Williams, Reference First, Gibbon, Spitzer and Williams2002); (5) personality traits (International Personality Disorders Examination paranoid, antisocial, dependent, narcissistic traits; Loranger, Reference Loranger1995; State-Trait Anger Inventory-Anger Expression; Spielberger, Krasner, & Solomon, Reference Spielberger, Krasner, Solomon and Janisse1988); and (6) psychosocial dysfunction (Social Adjustment Scale: Weissman & Bothwell, Reference Weissman and Bothwell1976; Inventory of Interpersonal Problems: Horowitz, Rosenberg, Baer, Ureno, & Villasenor, Reference Horowitz, Rosenberg, Baer, Ureno and Villasenor1988). Moderator variables were winsorized to the 95th percentile, retaining extreme observations but bringing them closer into the observed distribution. Variables with significant skew were log-transformed to improve normality (which included both CTQ abuse measures and suicide/self-harm counts). Missing baseline variables, assumed to be at worst missing at random, were imputed using a single-dataset random forest imputation (Stekhoven & Bühlmann, Reference Stekhoven and Bühlmann2011) with the R package missForest version 1.4.
Analyses
Our protocol was pre-registered at the Open Science Framework, ID 7JCP5.Footnote †Footnote 1 All analyses were conducted within the R statistical computing environment, version 3.6.1 in Windows 10.
Variable selection
We engaged in a two-step variable selection procedure. First, we employed random forest model-based recursive partitioning (MoB; Garge, Bobashev, & Eggleston, Reference Garge, Bobashev and Eggleston2013) which takes a basic parametric model, and detects variables along which splits into two subgroups lead to significantly different model behaviors on either side of the split. It has been applied previously in moderator investigations in psychiatry (Driessen et al., Reference Driessen, Smits, Dekker, Peen, Don, Kool and Van2016; Keefe et al., Reference Keefe, Wiltsey Stirman, Cohen, DeRubeis, Smith and Resick2018; Zilcha-Mano et al., Reference Zilcha-Mano, Keefe, Chui, Rubin, Barrett and Barber2016). The extension of this model employs MoB within bootstrapped re-samplings of the dataset. For each bootstrap, the resulting tree is tested on the out-of-bag sample, which is held out of a given tree construction. A variable's ability to predict out-of-bag is compared to the ability of randomly permuted data to make the same prediction. Variables with a prediction statistic higher than that of the absolute value of the moderator with the most negative predictive value (i.e. in which the permuted data are superior to the real data) are retained (Strobl, Boulesteix, Kneib, Augustin, & Zeileis, Reference Strobl, Boulesteix, Kneib, Augustin and Zeileis2008). We set the criterion such that at least 15 subjects must be present on each side of a tree split, with an α of 0.05 for the split. One-third of possible variables were randomly allowed to be selected at any given node. The R package mobForest version 1.3.1 was employed (Garge, Eggleston, & Bobashev, Reference Garge, Eggleston and Bobashev2013).
Variables retained in the first step were subjected to a bootstrapped Akaike information criterion-based backward selection model (Austin & Tu, Reference Austin and Tu2004). One major goal of this step is to consider the robustness of the predictive value of all selected variables considered in tandem in the same model, rather than separately as in the random forest partitioning variable selection. To make it to the final model, a variable had to be retained in the model in at least 60% of bootstrapped replicates (Austin & Tu, Reference Austin and Tu2004). The R package bootStepAIC version 1.2 was employed (Rizopoulos, Reference Rizopoulos2009).
Combined, the two-step bootstrap filtering partially protects against overfitting by ensuring that any given variable is predictive across bootstrapped replications of the data structure, can predict to groups of patients not used to select the variable (in mobForest), and shows predictive utility in a permutation test (Chekroud et al., Reference Chekroud, Zotti, Shehzad, Gueorguieva, Johnson, Trivedi and Corlett2016; Davidson & Hinkley, Reference Davidson and Hinkley1997; Koutsouleris et al., Reference Koutsouleris, Kahn, Chekroud, Leucht, Falkai, Wobrock and Hasan2016). Any variable that survived the two-step variable selection process was included in the final model.
Treatment selection
To estimate the degree to which assigning patients to a specific treatment based on the outputs of the combined moderator model would yield superior treatment outcomes, the personalized advantage index (PAI) approach was implemented. In this approach, multiple moderators are combined in a statistical model that is then used to predict a patient's response to each treatment. An index that reflects the magnitude of the predicted advantage in one treatment over the other is also given by the method (DeRubeis, et al., Reference DeRubeis, Cohen, Forand, Fournier, Gelfand and Lorenzo-Luaces2014).
A 10-fold cross-validation procedure was used to estimate the predictive value of the final model indicated by the variable selection process. Cross-validation is a common and effective method to approximate an out-of-sample generalization (Berk, Reference Berk2008). Model coefficient weights for moderators were set on 10 subsets of 90% of the sample and were used to predict response to each treatment in each subset of 10% of patients not used to generate a given model's weights. The difference between the two model-predicted probabilities of symptom improvement in each treatment was calculated, resulting in a signed (positive or negative) score, indicating which of the treatments was determined to be ‘optimal’ for that patient (i.e. the treatment in which they were predicted to have the most symptom improvement). The entire 10-fold cross-validation procedure was bootstrapped and repeated 1000 times with different 90%/10% subsets to ensure stability of the results. Outcomes were then compared among patients happening to be randomized to their optimal treatment v. those who were not. By convention, we also examined outcomes among patients given relatively stronger (40th percentile of PAI distribution and above) compared to weaker predictions of having an optimal treatment, as patients with weaker predictions may have estimates of advantage around the indifference point (i.e. 0). The R package caret version 6.0-84 was used for cross-validation (Kuhn, Reference Kuhn2008), and BSDA version 1.2 was used to test the cross-validated results.
In our primary analysis, the selection procedure applied cross-validation after model building to evaluate the generalizability of our specified model. However, this increases the susceptibility of our model's results to be overfit since our variable selection method evaluated the entire dataset (Poldrack, Huckins, & Varoquaux, Reference Poldrack, Huckins and Varoquaux2019), even with bootstrapping and some internal cross-validation during variable selection (in mobForest). We added an additional methodological check against potential effect size inflation that could occur due to selecting variables in the same data in which model weights are set. We did this by estimating the effect size of treatment selection when performing both variable selection and model weight setting in a 10-fold cross-validated manner, which means that a given hold-out sample is completely uninvolved in either choosing moderating or estimating effects. This serves as a conservative ‘test’ of the effect size of this specific process of selecting for variables to be included in a combined moderator variable, but it is less interpretable at an individual moderator level as 10 different potential lists are generated. By contrast, the original analyses are a better window into specific, potential moderator variables as they employ all of the data.
Results
Descriptive data
One-hundred fifty-six patients (78 GPM) were eligible for inclusion in our study. There was only one significant difference between treatments in terms of baseline characteristics (childhood sexual abuse; see Table 1). As in the analyses conducted in the parent clinical trial, there were no overall differences on the SCL-90 GSI in symptom levels across the course of 2-year follow-up (i.e. the AUC scores), controlling for baseline GSI scores [d = 0.03, t(153) = 0.186, p = 0.853]. AUC scores for the SCL-90 GSI correlated highly with those for the Zanarini BPD scale (r = 0.81), the Inventory of Interpersonal Problems (r = 0.83), and the Social Adjustment Scale (r = 0.84) over this same interval, suggesting that SCL-90 scores tapped into treatment response across these domains.
Table 1. Baseline data for DBT and GPM on possible moderator demographic and clinical variables for patients included in analyses (n = 156)
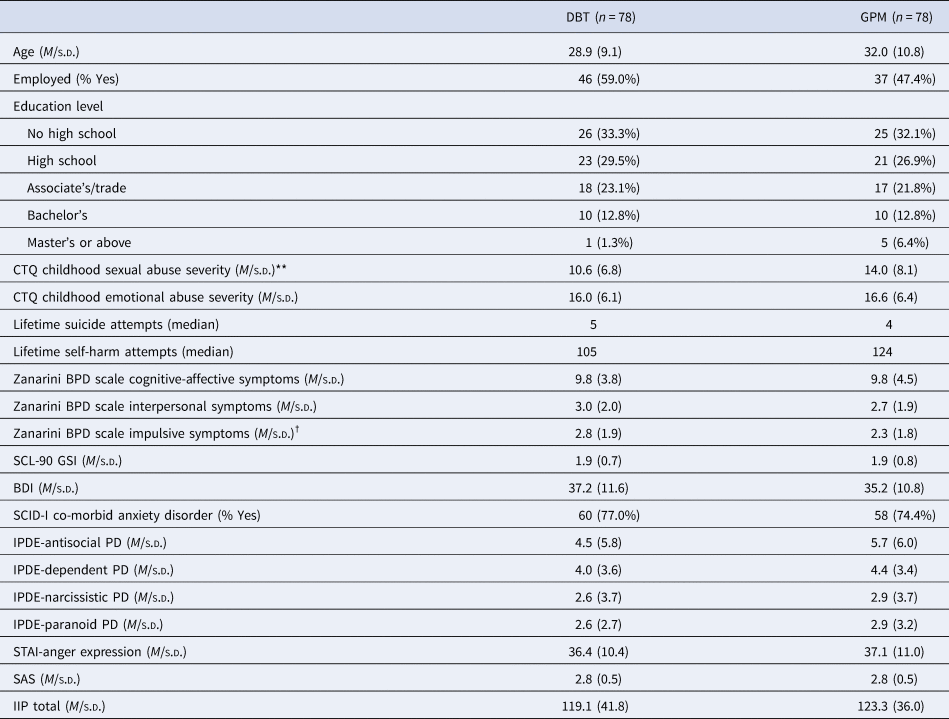
BDI, Beck Depression Inventory; CTQ, Childhood Trauma Questionnaire; IIP, Inventory of Interpersonal Problems; IPDE, International Personality Disorders Examination; SAS, Social Adjustment Scale; SCID-I, Structured Clinical Interview for the Diagnosis of Axis I Disorders; SCL-90 GSI, Symptom Checklist 90 Global Severity Index; STAI, State-Trait Anger Inventory.
† p < 0.10; **p < 0.01.
Selected moderators
Six variables interacting with treatment condition survived variable selection through both variable selection steps: baseline GSI symptom severity, childhood emotional abuse (CTQ), dependent personality traits (IPDE), impulsivity BPD symptoms (Zanarini scale), social adjustment (SAS), and depression (BDI). When considered in the same omnibus model, all variables maintained their statistical significance as moderators, with the exception of baseline BDI score. See Table 2 for a description of the effects of the individual moderators in this omnibus model. We also examined how well these moderators generalized to the three alternative outcomes (Zanarini BPD scale, IIP, SAS; and the average standardized AUC across these and the GSI), finding that baseline Zanarini Impulsivity score and SAS were relatively less generalizable to non-GSI outcomes (see online Supplementary Table).
Table 2. Descriptions of attained moderator variables (combined n = 156)

Note: β represents a standardized beta for comparison. Lower AUC values indicate better long-term outcomes on the GSI.
Treatment selection model
We then employed these moderators in the same model to generate a combined moderator profile, using the PAI method and 10-fold cross-validation employed across 1000 bootstrapped replicates. On average, 51.2% of patients were recommended on the basis of this moderator profile to receive DBT as compared to GPM.
Following this, we examined the degree to which patients whose random assignment happened to be to their model-predicted optimal treatment had superior outcomes relative to patients who did not. Utilizing our bootstrapped cross-validation procedure, we estimated that patients receiving their model-assigned optimal treatment had superior outcomes with a small-to-medium effect size [d = 0.36, t(154) = 2.22, p = 0.028; see Fig. 1]. The advantage for when a patient happened to receive their optimal treatment was similar when patients were recommended DBT as they were when recommended GPM (d difference < 0.05).

Fig. 1. The estimated advantage of randomization to a patient's model indicated optimal treatment over non-optimal treatment based on a combined moderator profile generated through cross-validated predictions. Bars reflect 95% bootstrapped confidence intervals, with non-overlap with the 0 point indicating significant cross-validated predictive value on GSI outcomes of the combined moderator. The average patient assigned to their optimal treatment was estimated to have significantly better long-term outcomes (p = 0.028). Among patients with a relatively stronger prediction (top 60% percentile), there was furthermore a significant advantage (p = 0.004) that was retained when engaging in a conservative statistical check (p = 0.043). However, patients with weaker predictions (bottom 40% percentile) were generally estimated to have no significant benefit to being assigned a predicted optimal treatment (p = 0.929).
On the basis of their characteristics, some patients may be estimated as more strongly needing a particular therapy. The effect magnitude of being randomized to receive one's model-predicted optimal treatment increased to a medium-to-large effect when only considering patients with relatively stronger predictions of optimal treatment [top 60% magnitude; d = 0.61, t(92) = 2.92, p = 0.004; see Fig. 1] compared to patients with weaker or equivocal predictions [bottom 40% magnitude; d = −0.02, t(60) = −0.09, p = 0.929] who showed no benefit.
Statistical checks
To better estimate the non-inflated effect size of the combined moderator and to check how much of the original model's results could be due to overfitting, we then repeated this process performing a 10-fold cross-validation not only at the point of model fitting, but also variable/moderator selection. This does not produce a uniformly interpretable model as moderators making it through the two-step selection process may differ depending on the included folds and fewer subjects are available to power variable selection per fold. However, by holding out sets of patients completely from both the variable selection and model fitting steps, it provides a conservative estimate of how well this process creates a model that would generalize to a similar sample.
Using this method, the magnitude of the effect size for receiving one's optimal treatment in the total sample was deflated approximately 40% and was no longer statistically significant [d = 0.22, t(154) = 1.38, p = 0.170]. On the other hand, among patients with a stronger magnitude of predicted advantage (top 60%), the advantage of happening to receive one's model-predicted optimal treatment remained significant, although the size was also deflated by approximately 25% [d = 0.46, t(79) = 2.06, p = 0.043].
Discussion
Patient characteristics can help indicate which of two BPD therapies (DBT v. GPM) is most likely to provide long-term symptom relief over the course of 2 years. When randomized to their optimal treatment as predicted by a combined moderator profile generated in a cross-validated manner, patients had significantly better symptom relief on the GSI across the follow-up period. This was furthermore the case even when using a conservative statistical check performing both moderator selection and model generating in a cross-validated manner. It is notable that no single moderator was a large-effect predictor of differential treatment response; this is consistent with a perspective that, to best match a patient with a treatment, considering multiple sources of information may be more clinically useful than examining single moderators in isolation.
A major strength of this investigation, relative to other efforts at identifying and reporting the effects of moderators, was the use of long-term follow-up data for up to 2 years, with excellent availability for this follow-up data. Inasmuch as the attained variables and their combination truly predict outcomes for patients with BPD, these outcomes can be considered relatively long-lasting and possibly more clinically meaningful than short-term results.
For patients with a combination of characteristics that led to them receiving a relatively stronger prediction of their optimal treatment, effects were of a medium-to-large size and robust to more conservative model-generating methods. Conversely, for patients with less strong predictions, there was no reliable benefit to receiving one's model-predicted optimal treatment. Equivocal predictions of optimal treatment may reflect that we did not include variables relevant to distinguishing response for these patients, that some patients have a balance of factors that both recommend them for and against a treatment, that some of these patients would do well in any bona fide treatment, or that some patients are very difficult to help with either treatment (DeRubeis, Gelfand, German, Fournier, & Forand, Reference DeRubeis, Gelfand, German, Fournier and Forand2014).
DBT performed comparatively well among patients who were more dependent, more psychosocially impaired, and burdened by significant childhood emotional trauma. Our finding on psychosocial impairment conceptually replicates a report from a randomized trial of DBT v. object-relations psychodynamic therapy v. treatment as usual for BPD – in this trial, patients who were less impaired at baseline had better outcomes in psychodynamic therapy relative to DBT or treatment as usual (Sahin et al., Reference Sahin, Vinnars, Gorman, Wilczek, Åsberg and Barber2018). Patients with especially low social adjustment may benefit from learning structured skills to improve their poor functioning, perhaps particularly in the context of pragmatically applying them in therapy group. More dependent patients may also be more likely to form stronger therapeutic alliances with high-contact DBT in which the therapist is more available (Hirsh, Quilty, Bagby, & McMain, Reference Hirsh, Quilty, Bagby and McMain2012).
By contrast, GPM relatively excelled at treating more intensely or complexly symptomatic patients with more impulsivity problems, potentially because GPM has more direct provision of targeted psychopharmacology (i.e. by a provider who sees the patient weekly) that can adapt to different symptoms. More impulsive or intensely symptomatic patients may also find it harder to practice and apply structured DBT skills even if they would in theory be helpful. Patients with more trauma history and interpersonal sensitivity (i.e. as indexed by dependent traits and psychosocial difficulties) may also find it more difficult to use the GPM relationship as a corrective experience. Patients diagnosed with current comorbid post-traumatic stress disorder (PTSD) on the SCID-I (41.6%) reported more intense childhood emotional abuse (d = 0.75), raising the possibility that some subset of these patients would further qualify for the recently ICD-defined ‘complex’ PTSD (Karatzias et al., Reference Karatzias, Cloitre, Maercker, Kazlauskas, Shevlin, Hyland and Brewin2017).
Limitations and future directions
While this trial had an atypically high assessment retention over follow-up (86.7%), we nevertheless cannot conclude how generalizable our findings would be to the approximately 13% of patients who did not provide assessments during long-term follow-up. Our sample also consisted of a relatively more impaired group of BPD patients who typically had significant recent problems with suicidality (including several attempts) and self-harm; these findings may be less generalizable to groups of comparably less high-risk BPD patients.
We also examined the GSI as a broad assay of symptoms and dysfunctional experiences common among BPD patients with high psychiatric comorbidity, and our results may not apply to other outcomes. On the other hand, AUCs for the SCL-90 GSI correlated very strongly with those for borderline symptoms, interpersonal problems, and psychosocial functioning (rs > 0.80). We also examined how well the moderators applied to these alternative outcomes, finding the best generalizability for baseline GSI, childhood emotional abuse, depression, and dependent personality traits and trend-level for Zanarini Impulsivity symptoms and SAS score. This suggests a fairly unitary response profile across outcomes. Nevertheless, further moderator efforts in BPD could focus on alternative outcomes such as psychosocial functioning, which in naturalistic samples tends to remain low even after remission of DSM-defined BPD criteria per se (Gunderson et al., Reference Gunderson, Stout, McGlashan, Shea, Morey, Grilo and Skodol2011).
For our primary model, the estimated effect sizes for receiving one's optimal treatment may be inflated even with the use of cross-validation and bootstrapped variable and model selection, as variables were selected in the same general sample in which model fitting occurred. However, we also performed a secondary analysis employing cross-validation through every step of variable selection and model-fitting. This analysis attained similar results among patients with stronger predictions of an optimal treatment. Ultimately, a prospective study of employing combined moderator models for treatment selection in BPD would be the definitive test for its utility, although to our knowledge no such trial has ever been performed in psychiatry for any disorder.
Eventually, replicated moderator findings could inform treatment recommendation decisions to direct patients to the treatment(s) most likely to help them, in conjunction with other factors such as patient preference (Windle et al., Reference Windle, Tee, Sabitova, Jovanovic, Priebe and Carr2019). Of relevance is that these two treatments had different time burdens, with approximately 3 h a week of therapeutic contact for DBT and 1 h for GPM. Full model DBT may also be more complicated to train in and implement than GPM which is intended to be used by general psychiatric practitioners (Gunderson, Reference Gunderson2016; Landes et al., Reference Landes, Rodriguez, Smith, Matthieu, Trent, Kemp and Thompson2017), although there is emerging evidence that focus on DBT skills alone (Linehan et al., Reference Linehan, Korslund, Harned, Gallop, Lungu, Neacsiu and Murray-Gregory2015; McMain, Guimond, Barnhart, Habinski, & Streiner, Reference McMain, Guimond, Barnhart, Habinski and Streiner2017) can be effective as well (though perhaps not as much as full-model DBT). An understanding of which BPD patients may do just as well or better in a lower-intensity but bona fide treatment (GPM) may lead to not only better outcomes but more efficiency for the patient and healthcare systems. A major limitation is that differential treatment recommendation requires more than one treatment for BPD be available to patients, which in some health systems (such as much of the United States) is uncommon.
Finally, our findings do not indicate the mechanisms by which these moderator variables interact with treatment to predict differential outcomes. Further understanding of the mechanisms of BPD treatment – such as personality change and acquisition of compensatory skills – may help inform how patient characteristics influence the impact of particular psychological changes encouraged in therapy, or how these characteristics may influence the degree to which these helpful changes occur in a given therapy.
Conclusion
Overall, our results suggest that a ‘one-size fits all’ or uniform approach to the treatment of BPD may leave some patients with an inadequate recovery from symptoms, as their baseline characteristics imply a need for or enhanced response to one treatment over another. Clinical trials comparing two active treatments for BPD are uncommon (Cristea et al., Reference Cristea, Gentili, Cotet, Palomba, Barbui and Cuijpers2017; Keefe et al., Reference Keefe, McMain, McCarthy, Zilcha-Mano, Dinger, Sahin and Barber2019), but may be useful to conduct to provide clinicians with more precise information as to optimal therapies for their patients. Research distinguishing and capitalizing on heterogeneity among patients meeting for BPD may lead to better targeted, patient-personalized treatments.
Supplementary material
The supplementary material for this article can be found at https://doi.org/10.1017/S0033291720000550
Acknowledgements
We would like to thank Dr Eliora Porter for her helpful comments on a version of this manuscript.
Conflict of interest
Dr Paul Links has received an unrestricted educational grant from Eli Lilly Canada Inc. He is also a co-author for the GPM text “Handbook of Good Psychiatric Managemment for Borderline Personality Disorder,” published by American Psychiatric Publishing. All other authors report no competing interests.