Introduction
Deciding between categorical and dimensional models of latent variables is a fundamental and enduring issue in psychiatry and psychology, which taxometric analyses were designed to resolve. Developed by Paul Meehl and his colleagues, these procedures allow researchers to determine whether observed variation is underpinned by a non-arbitrary latent class, or ‘taxon’, such as a discrete psychopathology or personality type. Discovering taxa and distinguishing them from latent dimensions has broad implications for how personality and psychopathology should be conceptualized, assessed and explained.
If a latent variable is taxonic, for example, it must be conceptualized as an entity with real category boundaries that exist independent of social convention or descriptive convenience. If it is not taxonic then no boundary exists unless a manifest distinction such as a diagnostic threshold is imposed on arbitrary or pragmatic grounds. The appropriate way to assess a taxonic variable involves assigning cases to categories at the taxon boundary, but assessing non-taxonic variables involves quantifying variation along the entirety of an underlying continuum. Taxa are likely to spring from mechanisms that Meehl (Reference Meehl1977) referred to as ‘specific etiologies’, such as single discrete causal factors, whereas non-taxonic variables generally result from the additive effects of multiple small causal influences. Determining whether or not a latent variable is best thought of as taxonic is a crucial scientific question and not merely a matter of theoretical taste or statistical botanizing.
The taxometric method makes this determination in a distinctive way. Unlike some more familiar statistical approaches to latent variable analysis, it does not impose a particular kind of structure, as cluster analysis presumes a categorical structure or factor analysis a set of underlying dimensions, but instead tests between these alternatives. Unlike most comparable forms of data analysis, it does not follow a null hypothesis testing approach to inference or yield a single definitive statistic. Where most other analyses use a single statistical procedure, the taxometric method seeks consistency among the findings of multiple mathematically independent procedures. Other analyses provide chiefly numerical output, whereas the output of taxometric analyses is largely graphical, based on the interpretation of curves. Despite these unusual features, the taxometric method has proven to be popular and versatile (Ruscio et al. Reference Ruscio, Haslam and Ruscio2006).
Taxometric analyses were first used to test Meehl's theory of schizophrenia, which proposed a taxonic genetic liability that manifests as schizotypal personality. The initial study (Golden & Meehl, Reference Golden and Meehl1979) supported the existence of the proposed taxon, and in the 1980s a series of articles reported taxonic findings in the domains of normal personality (self-monitoring: Gangestad & Snyder, Reference Gangestad and Snyder1985; Type A: Strube, Reference Strube1989), abnormal personality (schizotypy: Erlenmeyer-Kimling et al. Reference Erlenmeyer-Kimling, Golden and Cornblatt1989) and other psychiatric phenomena (dementia: Golden, Reference Golden1982; tardive dyskinesia: Golden et al. Reference Golden, Campbell and Perry1987; nuclear depression: Grove et al. Reference Grove, Andreasen, Young, Endicott, Keller, Hirschfeld and Reich1987). The trickle of studies in the 1980s became a stream in the 1990s and a torrent in the new millennium.
The fundamental questions that motivated the taxometric method are still pressing. Within clinical psychology and psychiatry, the categorical/dimensional issue remains contentious as many theorists, clinicians and researchers question the merits of categorical diagnosis and classification. These doubts have reached a crescendo, playing a role in the changes underway in DSM-V (Widiger & Samuel, Reference Widiger and Samuel2005; Helzer et al. Reference Helzer, Kraemer and Krueger2008), which embeds dimensional judgments in psychiatric diagnosis like never before, recognizing degrees of severity and impairment in many conditions and diagnosing personality disorders (PDs) along a set of continua.
The structural question that drives taxometric research also has continuing relevance to the psychology of normal personality. Dimensional views of traits predominate (Meehl, Reference Meehl1992), but there is enduring interest in the possible existence of personality types (e.g. Asendorpf, Reference Asendorpf2002) or configural prototypes (Eaton et al. Reference Eaton, Krueger, South, Simms and Clark2011). Personality psychology's status quo is thus the mirror image of psychiatry's: evidence for personality types challenges the default dimensional assumptions of trait psychologists, just as evidence that psychopathology is a matter of degree challenges categorical assumptions about psychiatric diagnosis. Taxometric research can provide a firmer empirical foundation for scientific taxonomy in both fields by testing these assumptions.
Taxometrics is not the only form of data analysis that can test between categorical and dimensional models of latent variables, and sophisticated alternatives exist (McLachlan & Peel, Reference McLachlan and Peel2000; De Boeck et al. Reference De Boeck, Wilson and Acton2005; Markon & Krueger, Reference Markon and Krueger2006). Nevertheless, taxometric research is a particularly rich source of knowledge on latent structure within psychology and psychiatry because its longevity means that it has built up a relatively large body of empirical findings on a wide assortment of latent variables. Reviewing those findings could help to answer basic questions about the latent structure of psychological variation. First, does existing research support the existence of any taxa in personality and psychopathology? Second, if solid evidence of taxa has been obtained, in what domains are they found? Taxa may be rarer in normal personality than in psychopathology, and rarer in PDs than in other psychiatric domains (Trull & Durrett, Reference Trull and Durrett2005). Whether taxa differ in prevalence across these domains and whether there is robust and replicated evidence for particular taxa are open questions.
In addition to clarifying substantive questions regarding the latent structure of personality and psychopathology, a systematic review of taxometric research might also shed light on methodological issues. First, are certain sample types (e.g. ‘abnormal’ clinical or forensic samples) more likely to yield taxonic findings than others (e.g. ‘normal’ community members)? Similarly, are taxa less frequent among children and adolescents than among adults, as might be expected if taxa differentiate developmentally? Sample size may also have implications for taxonic findings, as research using samples that fail to meet Meehl's (Reference Meehl1995) recommended minimum n of 300 may have a taxonic bias because they generate less stable curves. Second, are certain kinds of data more or less likely to produce taxonic findings? Some writers have argued that self-report data can generate spurious taxonic findings (Beauchaine & Waters, Reference Beauchaine and Waters2003).
A third set of methodological questions relates to aspects of measurement in taxometric research, which relies on multiple ‘indicators’ of the proposed latent variable. Researchers have argued that dichotomous indicators may induce a taxonic bias (Ruscio, Reference Ruscio2000), and that inadequate indicator validity may also impair taxometric inference (Meehl & Yonce, Reference Meehl and Yonce1994, Reference Meehl and Yonce1996). Research that uses indicators of limited reliability (e.g. based on single questionnaire items) or fails to report evidence of validity may yield taxonic findings at different rates than research using more valid indicators.
A final set of methodological questions involves statistical methods. Five taxometric procedures are in widespread use [latent mode (L-Mode), mean above minus below a cut (MAMBAC), maximum covariance (MAXCOV), maximum eigenvalue (MAXEIG), maximum slope (MAXSLOPE)] and some may be more likely to draw taxonic conclusions. Finding consistency among multiple procedures is a hallmark of the taxometric method, and the use of relatively few procedures can be considered one element of low methodological quality, along with small sample sizes, and dichotomous, single-item and unvalidated indicators, that might have a bearing on taxometric research findings.
One statistical method that deserves special mention is the use of simulated comparison data. This adjunct to taxometric practice (Ruscio et al. Reference Ruscio, Ruscio and Meron2007) involves the parallel analysis of simulated taxonic and dimensional data sets that match the distributional and correlational properties of the observed research data. If the graphical output generated from the research data more closely resembles the output of one set of simulations than the other, then the simulation procedure supports the corresponding latent structure. The comparative fit of the research data output to the two simulations can be quantified by an index, the comparison curve fit index (CCFI), where values equal to 0.5 represent equally good fit, values less than 0.5 support a dimensional finding and values greater than 0.5 support taxonicity. The CCFI offers an objective decision rule to supplement visual inspection of curves, the traditional basis for taxometric inference.
Although the simulated comparison data procedure has been controversial among some researchers (Beach et al. Reference Beach, Amir and Bau2005a; cf. Ruscio & Marcus, Reference Ruscio and Marcus2007), Monte Carlo studies provide very strong evidence for its validity and robustness under unfavourable data conditions. In an analysis of 25 000 simulated data sets constructed to present challenges for taxometric inference (e.g. indicator skew and coarseness, modest indicator validity, ‘nuisance covariance’ among indicators, unequal variance of latent distributions), Ruscio & Kaczetow (Reference Ruscio and Kaczetow2009) found that the CCFI achieved 93% accuracy in identifying taxonic and dimensional latent structures, rising to 98% when CCFI values were outside an ambiguous intermediate range (i.e. <0.4 or >0.6). In an even larger study of 100 000 data sets, Ruscio et al. (Reference Ruscio, Walters, Marcus and Kaczetow2010) found that a CCFI threshold of 0.5 achieved an average of 94% accuracy for MAMBAC, MAXCOV and L-Mode applied individually, and 98% when the mean CCFI of the three procedures was used. A similar study of 10 000 data sets (Ruscio et al. Reference Ruscio, Ruscio and Meron2007) found that the CCFI strongly outperformed several previous fit indices and consistency tests. Use of simulated comparison data and the CCFI has become widespread, and it is therefore important to review whether this methodological development has had an influence on taxometric research findings.
Previous reviews of taxometric research have been qualitative and restricted to psychopathology-related constructs (Haslam, Reference Haslam2003, Reference Haslam2007), and they are now seriously outdated, the last comprehensive review (Haslam & Kim, Reference Haslam and Kim2002) covering less than one quarter of the taxometric articles published as of 2011. We therefore conducted a comprehensive quantitative review of all published empirical findings using multilevel logistic regression analysis, in an effort to ascertain the prevalence of taxonic findings and the factors associated with them. Although the primary focus of the study was on substantive factors (i.e. which latent variables are taxonic), we also examined methodological factors that might contribute to taxonic findings, potentially as sources of bias.
Method
Study sample
An exhaustive literature search was conducted using previous reviews, publication databases (Google Scholar, PsycINFO, Web of Science) and journal and publisher websites (search terms included taxometric*, taxon*, MAXCOV, MAMBAC, MAXEIG). Inclusion and exclusion criteria were as follows: research had to (1) be published in peer-reviewed journal articles; (2) be officially published or ‘in press’ by 1 April 2011; (3) address observed substantive latent variables rather than simulated data sets or those involving an experimental manipulation (Arnau et al. Reference Arnau, Thompson and Cook2001; Beauchaine & Waters, Reference Beauchaine and Waters2003; McGrath et al. Reference McGrath, Neubauer, Meyer and Tung2009); and (4) use at least one of the accepted taxometric procedures.
Each article could yield one or more pertinent empirical finding, defined as a conclusion about the latent structure of a single construct based on one or more taxometric procedures in a single sample. Different findings within one article could reflect the empirical investigation of more than one construct and/or be based on distinct samples. In this sense, when multiple taxometric procedures are used to analyse a single construct in a single sample, they contribute to a single finding. Similarly, parallel analyses of different sets of indicators of a single construct in a single sample were counted as contributing to a single finding. On these definitions, the 177 articles contained 311 findings (mean=1.76, range 1–11).
Coding
The 177 articles were coded by the first author on multiple characteristics under the headings of publication details, sample characteristics, measurement characteristics, data analysis, results, and construct. All coding was conducted at the level of the individual finding, except for codes related to publication details (i.e. publication year, journal, number of findings), which were conducted at the article level.
Sample characteristics
For each finding, we coded sample size, whether the sample was composed of undergraduates, whether it was drawn from a clinical or forensic sample, whether the sample was drawn from the general public, and whether it was composed primarily of children or adolescents (<18 years).
Measurement characteristics
We coded whether any of the data were based on self-report, ratings by observers, or interviews. With respect to indicator construction, we coded the number of indicators used in the analysis, whether any indicators were based on a single item rather than summed items, whether any indicators were dichotomous, and whether the validity of the indicators was reported in the manuscript (including quantification of indicator validities or any mention that they had satisfied a quantitative validation process).
Data analysis
Data-analytic methodology was coded in terms of the use or non-use of the MAXCOV, MAMBAC, MAXEIG, MAXSLOPE, L-Mode, and ‘other’ taxometric procedures. These codes were summed to produce a ‘number of procedures’ variable. Use of Ruscio's simulated comparison data technique was also coded.
Results
The overall conclusion for each finding was coded taxonic (1) or non-taxonic (0), based on the researchers' interpretation. Two ambiguous interpretations were coded as non-taxonic. For studies reporting CCFI values, or allowing them to be computed from an earlier fit index (fitRMSR), we coded the values for each taxometric procedure that was used to generate them. For each finding with at least one CCFI reported, a mean CCFI value was computed by taking the average across procedures.
Construct
The studied constructs were classified into 10 groupings, developed to reflect broad construct domains but also to recognize narrower domains that have received substantial taxometric attention. Seven groupings were psychopathology related, one referred to normal personality and two were residual groupings. The groupings and a listing of constructs examined in the article sample are presented in Table 1.
Table 1. Classification of constructs examined in taxometric research
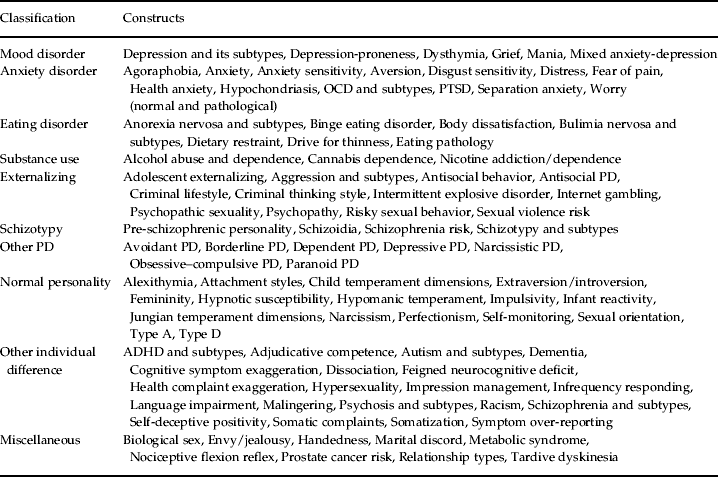
OCD, Obsessive–compulsive disorder; PTSD, post-traumatic stress disorder; PD, personality disorder; ADHD, attention deficit/hyperactivity disorder.
The ‘mood disorder’ grouping included mood disorders, proposed subtypes of these disorders, affective phenomena related to them (grief, mania), and diatheses for them (depression-proneness). The ‘anxiety disorder’ grouping similarly included a mix of disorders, proposed variants, specific kinds of anxiety or aversion, and relevant diatheses (anxiety and disgust sensitivity). The ‘eating disorder’ grouping followed the same pattern. The ‘substance use’ grouping included abuse and dependence on a variety of substances. The ‘externalizing’ grouping contained constructs involving antisocial and under-controlled conduct, including gambling and sexual behavior. A ‘schizotypy’ grouping was defined narrowly given the long-standing tradition of taxometric research on this topic, and was kept separate from an ‘other personality disorder’ grouping, which included studies of seven recognized or proposed PDs. The ‘normal personality’ grouping comprised personality or temperamental traits. The ‘other individual difference’ grouping contained diverse psychological constructs including response styles and biases, attitudes, and pathological phenomena that have received little taxometric attention and do not readily fit under the other groupings. The final ‘miscellaneous’ grouping contained constructs that either were not psychological (e.g. biological sex, metabolic syndrome) or did not have the individual as the unit of analysis (e.g. emotions, relationship types).
General considerations
Individuating distinct findings within studies was occasionally challenging, as multiple parallel analyses were sometimes reported using distinct indicator sets and/or different subsamples (e.g. men versus women). In these circumstances, if a single construct was being examined, we coded analyses based on the entire sample and not those based on subsamples, and we took an average value of all quantitative codes across the parallel analyses.
Coding agreement
The second author was assigned 30 randomly chosen articles containing 58 findings (18.6%), and coded them on all codes except authors and the specific CCFI values. Mean agreement across the codes was 94.8% [range 82.8% (any dichotomous indicators) to 100% (multiple codes)].
Results
The mean publication year was 2005.0 (range 1979–2011), but the rapid growth in taxometric research illustrated in Fig. 1 yields a median of 2007. Sample sizes for the 311 findings varied widely (mean=1999.7, median=934, range 130–80 304) and were log-transformed for later analyses. A summary of categorical finding-level codes is presented in Table 2, which indicates that most studies used clinical, forensic or undergraduate samples, used self-report data, and conducted MAMBAC and MAXCOV analyses with indicators of demonstrated validity. Methodological choices known to weaken indicator validity (i.e. single-item indicators) or bias findings towards taxonic conclusions (i.e. dichotomous indicators) were common, but taxometric procedures other than the MAMBAC, MAXCOV, MAXEIG and L-Mode were rare. On average, 2.12 distinct taxometric procedures were used in the analysis of each finding. CCFI values were reported or derivable for a substantial minority of findings.

Fig. 1. Publication year of the 177 articles (incomplete year for 2011).
Table 2. Descriptive statistics on finding-level codes (n=311)

MAMBAC, Mean above minus below a cut; MAXCOV, maximum covariance; MAXEIG, maximum eigenvalue; L-Mode, latent mode; MAXSLOPE, maximum slope; CCFI, comparison curve fit index.
A composite index of methodological quality was constructed from several codes in a way that subtracted points for known methodological weaknesses and added them for known strengths. One point was subtracted for findings based on samples of <300, using one-item indicators, or using dichotomous indicators. One point was added for findings with an above-median sample size, an above-median number of distinct taxometric procedures, and demonstrated indicator validity. The five items composing this scale yielded an index ranging from −3 to +3 (mean=0.76, s.d.=1.56), and all items intercorrelated positively (α=0.67).
Table 3 summarizes the distribution of taxonic findings and the mean CCFI values for the subset of findings reporting them. CCFI values derived from different procedures were highly similar (MAMBAC=0.37, MAXCOV=0.35, MAXEIG=0.36, L-Mode=0.36) and all intercorrelated strongly (mean r=0.64). A large minority (38.9%) of the 311 findings were taxonic and the mean CCFI for the 136 relevant findings was 0.37, indicating that these findings tend to be predominantly non-taxonic. Indeed, the distribution of mean CCFI values (see Fig. 2), which reveals an apparent bimodality, shows that only 20 (14.7%) findings exceeded the taxonic threshold (CCFI >0.5). According to more conservative guidelines, 102 (75%) findings were clearly dimensional (<0.4), 17 (12.5%) were clearly taxonic (>0.6), and 17 (12.5%) were ambiguous.

Fig. 2. Distribution of mean comparison curve fit index (CCFI) values for findings reporting them (n=136).
Table 3. Distribution of taxonic findings and use of the CCFI across construct types

CCFI, Comparison curve fit index; PD, personality disorder.
Table 3 indicates that the rate of taxonic findings differed widely across the construct domains [χ(9)2=56.73, p<0.0001]. Taxonic findings were relatively infrequent in the anxiety disorder, externalizing, other PD and normal personality domains, and much more common in the eating disorder, substance use, schizotypy and miscellaneous domains. Taxonic findings were also significantly more common in the broad psychopathology arena (i.e. the combination of the mood, anxiety, eating, substance use, externalizing, schizotypy, and other PD domains) than in the normal personality domain [38.7% v. 16.3%, χ(1)2=8.72, p<0.01].
Rates of use of the CCFI were also highly variable across construct domains [χ(9)2=68.90, p<0.0001] and the three domains with the highest rate of taxonic findings used the CCFI the least. Evidence of a further disjunction between rates of taxonic findings and CCFI evidence is revealed in Table 3. Only one of the four domains with a majority of taxonic findings has a mean CCFI consistent with predominant taxonicity.
In a first attempt to assess factors related to taxonic findings, we examined associations between taxonicity and three potential predictors. The findings reported above suggest that use of the CCFI is negatively associated with taxonic findings. The relative recency of the CCFI suggests that publication year might show the same negative association. Finally, the methodological quality of studies, assessed independently of the use of the CCFI, with which it correlated positively (r=0.63), might be associated with taxometric findings. Indeed, findings of analyses using the CCFI were much less likely to be taxonic (16.2%: 22 of 136) than those that did not (56.6%: 99 of 175), χ(1)2=52.54, p<0.0001. More recently published findings were also less likely to be taxonic (r=−0.36, p<0.001); 54.5% of findings published prior to the median article year were taxonic, compared to 23.2% of later findings, as were the findings of studies of higher methodological quality (r=−0.33, p<0.001). In short, more recent, methodologically stronger research that systematically compares the fit of taxometric output to simulated taxonic and non-taxonic data sets is substantially less likely to yield taxonic findings than older and methodologically weaker research that does not.
These findings indicate that methodological and temporal factors are systematically associated with taxometric findings. However, because these predictors are related [e.g. more recent findings were methodologically stronger (r=0.68) and more likely to use the CCFI (r=0.65)], and other methodological and construct factors also predict findings, multivariate analyses are required to assess their unique effects. The hierarchical structure of the data, with findings nested in articles, necessitates an analysis that takes these dependencies into account. We therefore used multilevel logistic regression analysis (Bryk & Raudenbush, Reference Bryk and Raudenbush1992; Snijders & Bosker, Reference Snijders and Bosker1999).
Multilevel logistic regression analyses
We first ran single-predictor two-level models with findings nested in articles. For ease of interpretation and because we are not primarily interested in within-article relationships between variables (Enders & Tofighi, Reference Enders and Tofighi2007), binary predictors were entered uncentered, and non-binary predictors and also the level-2 predictor (publication year) were entered grand-mean centered. All analyses relied on population-average models, with random intercepts but no random slopes. The fixed effects are presented in Table 4. At level 2 (article), publication year was negatively associated with taxonicity: more recent studies were less likely to yield taxonic findings. At level 1 (finding), several methodological predictors were significantly associated with taxonic findings. Findings based on smaller samples, fewer taxometric procedures and indicators that were dichotomous, lacking reported validity, and based on single items were more likely to be taxonic. These predictors all compose the methodological quality index, which was consequently strongly associated with non-taxonic findings. Findings in which the CCFI was reported were much less likely to yield taxonic findings, as were those based in part on the MAMBAC, MAXEIG and L-Mode procedures. The findings based in part on the MAXCOV procedure and on ‘other’ taxometric procedures were significantly more likely to be taxonic. Findings in the schizotypy and miscellaneous construct domains were more likely to be taxonic, and those in the externalizing domain were less likely.
Table 4. Findings of single-predictor multilevel logistic regression analyses predicting taxonic findings

MAXCOV, Maximum covariance; MAMBAC, mean above minus below a cut; MAXEIG, maximum eigenvalue; L-Mode, latent mode; MAXSLOPE, maximum slope; PD, personality disorder; s.e., standard error; df, degrees of freedom.
Many successful predictors from these single-predictor analyses were correlated. For this reason, further analyses were conducted to tease apart unique effects. With a view to reducing the number of predictors in the final analysis, the quality index was used in place of the five predictors that constituted it. When the effects of the individual taxometric procedures were tested in analyses that also included quality, year and use of the CCFI, only L-Mode [B=−1.144, s.e.=0.526, t(303)=2.18, p<0.05] and MAXSLOPE [B=2.101, s.e.=0.875, t(303)=2.40, p<0.05] were significant, so only these two procedure variables were retained for the final analysis. However, the 10 construct domains were retained (represented as nine dummy variables with the ‘miscellaneous’ domain as the baseline), given the theoretical interest in determining which substantive domains were more likely to contain taxa.
The final analysis therefore predicted taxonic effects with publication year at level 2, and quality, CCFI, L-Mode, MAXSLOPE and nine construct domain dummies at level 1. The results, shown in Table 5, indicate that the methodological quality effects obtained in the single-predictor analyses disappear when other variables are statistically controlled, and that only use of the CCFI is significantly associated with a lower likelihood of taxonic findings. Findings based at least in part on L-Mode were significantly less likely to be taxonic and those based in part on MAXSLOPE were more likely. The construct domain effects, which represent the likelihood of taxonic findings in each domain relative to the miscellaneous domain, indicate that taxonic findings are less prevalent in the domains of normal personality, abnormal personality (other than schizotypy), eating disorders and anxiety disorders, with marginal negative effects for mood disorders and other individual differences. An analogous model with all variables grand-mean centered (such that each fixed parameter reflects the effect relative to the average study), leads to the same conclusions. Three of these effects were moderated by publication year: taxonic findings were less likely to be obtained in more recent studies of normal personality [B=−0.204, s.e.=0.103, t(283)=1.98, p<0.05], externalizing disorders [B=−0.455, s.e.=0.183, t(283)=2.49, p<0.05] and schizotypy [B=−0.351, s.e.=0.104, t(283)=3.39, p<0.005].
Table 5. Findings of final multilevel logistic regression analysis predicting taxonic findings

L-Mode, Latent mode; MAXSLOPE, maximum slope; PD, personality disorder.
Monte Carlo evidence (Ruscio & Kaczetow, Reference Ruscio and Kaczetow2009; Ruscio et al. Reference Ruscio, Walters, Marcus and Kaczetow2010) indicates that the CCFI is a highly accurate method for discriminating between taxonic and dimensional data even under unfavourable measurement conditions. Our finding that use of the CCFI is associated with a markedly reduced rate of taxonic findings implies that some taxonic findings in which the index was not used may be spurious. The same may be true of studies of lower methodological quality, which were more common in early taxometric research and more likely to deliver taxonic findings. To estimate the ‘true’ prevalence of taxonicity in our sample of findings after controlling for these two potential sources of error, we ran a multilevel model using only the CCFI and quality index as predictors. The predictive equation, with a significant effect of CCFI (B=−1.576, p<0.001) and a marginal effect of quality (B=−0.201, p=0.087), was then used to estimate the probability of taxonic findings if the fit index was present (1) and quality was maximum (+3). The estimated probability (0.14) was markedly less than the proportion of taxonic findings reported in the literature (0.39). By implication, the taxometric literature substantially overstates the true frequency of taxa in its field of study.
Discussion
Our analysis of the taxometric literature has implications for taxometric methodology and for the latent structure of psychological phenomena. With regard to methodology, the analysis suggests that several factors that might plausibly influence taxometric research findings had no detectable effects. Different sample types (undergraduate, clinical, forensic, or child and adolescent) did not differ in rates of taxonic findings. The same was true of different data types (self-ratings, other-ratings and interview-based judgments) despite some concerns that self-report data may produce pseudo-taxonic findings (Beauchaine & Waters, Reference Beauchaine and Waters2003).
Other methodological factors that have been identified as potential sources of bias had systematic effects. Research using relatively small samples and few taxometric procedures was more likely to yield taxonic findings, consistent with Meehl's (Reference Meehl1995) view that modest samples are problematic and consistency testing essential. Use of dichotomous indicators was also associated with a higher rate of taxonic findings, as Ruscio (Reference Ruscio2000) contended, as was the use of indicator variables of questionable validity, such as those based on single items and those for which quantitative evidence of validity was not reported. Together these factors formed a reliable index of methodological quality that powerfully predicted rates of taxonic findings: methodologically stronger research tended not to find taxa. Unexpectedly, two taxometric procedures were also associated with taxonic findings, L-Mode negatively and MAXSLOPE positively. The latter effect may have limited importance because MAXSLOPE is rarely used.
Our review points to historical changes in taxometric practice. Methodological quality has improved over time, with recent findings more likely to use several distinct taxometric procedures, less likely to use small samples, and less likely to use indicators that are dichotomous, single-item, or lacking demonstrated validity. This rise in methodological quality has accompanied, and probably partly driven, a decline in taxonic findings. Clearly, the most important historical development in taxometric practice has been the analysis of simulated comparison data and use of the CCFI (Ruscio et al. Reference Ruscio, Ruscio and Meron2007). This technique has rapidly become dominant in taxometric research, serving as an objective supplement or alternative to visual curve inspection, and our analysis found it to be a powerful predictor of structural findings. Research that did not use the technique was almost 3.5 times as likely to yield taxonic findings as research that did (56.6% v. 16.2%), and this effect persisted even when potentially confounding methodological and construct-related factors were statistically controlled.
The use of simulated comparison data has been controversial, and it might be argued that its negative association with taxonic findings reflects a pro-dimensional bias rather than enhanced validity. We consider this argument to be baseless. First, use of the CCFI was strongly and positively associated with methodological quality, implying that it is a positive adjunct to research that is methodologically stronger in other respects. Second, research that was methodologically stronger in uncontroversial ways (e.g. larger samples, use of more convergent data-analytic procedures) was also associated with lower rates of taxonic findings. Third, substantial Monte Carlo evidence (Ruscio et al. Reference Ruscio, Ruscio and Meron2007, Reference Ruscio, Walters, Marcus and Kaczetow2010; Ruscio & Kaczetow, Reference Ruscio and Kaczetow2009) attests to the CCFI's high accuracy, its robustness over a wide range of unfavourable measurement conditions that occur in real research settings, and its lack of significant bias either for or against taxonic findings. For example, Ruscio et al.'s (Reference Ruscio, Walters, Marcus and Kaczetow2010) analysis of 100 000 simulated data sets found that the CCFI correctly identified taxonic and dimensional data with equally high accuracy.
The reorientation of taxometric research in favour of dimensional models that the simulated comparison data technique has brought about is substantial. Our findings indicate that methodologically stronger research that uses the CCFI is particularly unlikely to find taxa, whereas early, pre-CCFI taxometric research, which seemed to find many taxa, tended to be methodologically weaker. By implication, early taxometric research is likely to contain invalid taxonic findings. Our analysis estimated the rate of taxonic findings in our 177 articles to be 14% rather than the observed 39%, had the CCFI always been used in studies of high methodological quality. If this estimate is accurate, almost two-thirds of reported taxonic findings are invalid. Moreover, most of the invalid findings would be found among the 56.3% of findings (175 of 311) in which the CCFI was not used. The reported rate of taxonic findings in these studies was 56.6% (99 of 175), so if the ‘true’ rate of taxonicity was approximately 14% then three out of four taxonic findings generated by non-CCFI studies were probably spurious.
The way in which research using simulated comparison data and the CCFI has challenged earlier taxonic findings is well illustrated by anxiety sensitivity and antisociality (i.e. antisocial conduct, antisocial PD, criminal lifestyle and thinking styles, and psychopathy). The first seven articles on anxiety sensitivity, published between 2005 and 2007, did not use the CCFI and generated 11 exclusively taxonic findings. The next three, published from 2008 to 2011, used the CCFI and generated seven exclusively non-taxonic findings (mean CCFI=0.34). An equally stark reversal occurred for antisociality: four early articles (1994–2005) that did not use the CCFI yielded exclusively taxonic findings whereas 17 later articles (2006–2011) that did use the CCFI yielded 18 consistently non-taxonic findings (all CCFIs <0.40, mean=0.28). The CCFI data-analytic strategy can therefore sharply and replicably challenge early taxonic findings.
If the ‘true’ rate of taxonic findings in the 177 taxometric articles is only about 14%, then taxonic latent structure is rather rare in psychology and psychiatry, instead of being common as the 39% figure would suggest. If it is further assumed that taxometric researchers have selectively examined latent variables for which taxonic structure was plausible, and have attempted to replicate findings where such structure was initially supported (e.g. the 29 findings on schizotypy), then taxa may be even rarer in the domains of personality and psychopathology than the former figure suggests.
This conclusion regarding dimensional versus categorical latent variation complements the findings of a recent meta-analysis of continuous versus discrete observed variation (Markon et al. Reference Markon, Chmielewski and Miller2011). Continuous measures of psychopathology were on average 15% more reliable and 37% more valid than discrete measures, a superiority that was invariant across different construct types. The authors proposed that ‘in the absence of a specific rationale for the contrary, continuous measures of psychopathology should be preferred over discrete measures a priori.’ Our findings suggest that dimensional models of the latent structure of personality and psychopathology should similarly be preferred over categorical models a priori, with the proviso that if 14% of taxometric findings are validly taxonic then there may be specific rationales to the contrary in some cases. Our findings certainly do not justify blanket claims that all psychological variation is latently continuous.
The findings of this review help to identify where latent discontinuities exist. They strongly suggest, as expected, that taxa are unlikely to be found in the domains of normal personality and PDs, supporting the standard assumptions of trait theory on the one hand and much recent PD scholarship on the other (e.g. Clark, Reference Clark2007). The findings also suggest that taxa are scarce within the internalizing spectrum, such as in the mood, anxiety and eating disorders, and also in the general externalizing domain. The only domains in which taxonic findings were at least somewhat prevalent were schizotypy and substance use disorders. Neither set of findings was consistently taxonic, the substance use domain was not significantly associated with taxonicity in the single-predictor analysis, and neither domain yielded taxonic findings at a significantly higher rate compared to the miscellaneous domain or to the average study in the final analysis. Nevertheless, these two domains stood out as offering at least some support for the existence of taxa. The schizotypy domain finding is perhaps unsurprising given its long record of taxonic findings, dating back to Golden & Meehl (Reference Golden and Meehl1979). Both findings qualify the conclusion that abnormal personality and externalizing disorders tend to be latently continuous: schizotypy and substance use may be at least partial exceptions to these generalizations.
In deciding which specific latent variables may be taxonic, it seems prudent to give special weight to those taxonic findings in which the CCFI was used, given the apparent taxonic bias of research in which it was not. Fifteen such findings (excluding two from the miscellaneous domain) exceed a conservative criterion for taxonicity (CCFI >0.6). These findings predominantly represent the domains of substance use (seven) and other individual differences (four). The domains of mood (depression: Ruscio et al. Reference Ruscio, Brown and Ruscio2009), anxiety (social anxiety disorder: Weeks et al. Reference Weeks, Carleton, Asmundson, McCabe and Anthony2010), eating (binge-eating disorder: Hilbert et al. Reference Hilbert, Pike, Wilfley, Fairburn, Dohm and Striegel-Moore2011) and externalizing disorders (intermittent explosive disorder: Ahmed et al. Reference Ahmed, Green, McCloskey and Berman2010) are represented by one finding each. These solo findings do not provide a strong basis for inferring taxa as they are either unreplicated or run contrary to many non-taxonic findings for the same construct.
The existence of substance use taxa is supported by elevated CCFI values in five studies of alcohol abuse, misuse or dependence (Walters, Reference Walters2008, Reference Walters, Berry, Lanyon and Murphy2009a;Walters et al. Reference Walters, Hennig, Negola and Fricke2009c; Walters & Ruscio, Reference Walters and Ruscio2010; Green et al. Reference Green, Ahmed, Marcus and Walters2011) and in two studies of nicotine dependence (Goedeker & Tiffany, Reference Goedeker and Tiffany2008). Although several studies using the CCFI have yielded non-taxonic findings in this domain (alcohol abuse and dependence: Slade et al. Reference Slade, Grove and Teeson2009; Walters et al. Reference Walters, Diamond and Magaletta2010a; cannabis dependence: Denson & Earlywine, Reference Denson and Earleywine2006; nicotine dependence: Ginestet et al. Reference Ginestet, Mitchell and Wellman2008), there is at least some replicated evidence that substance use taxa exist.
In the ‘other individual differences’ domain, three of the four strongly taxonic findings relate to autism and its subtypes (Ingram et al. Reference Ingram, Takahashi and Miles2008; Frazier et al. Reference Frazier, Youngstrom, Sinclair, Kubu, Law, Rezai, Constantino and Eng2010). Although two of these findings came in the context of a study in which five other possible subtypes seemed to be non-taxonic (Ingram et al. Reference Ingram, Takahashi and Miles2008), there is now consistent support for the existence of taxa in the autism realm, including an additional study that did not use the CCFI (Munson et al. Reference Munson, Dawson, Sterling, Beauchaine, Zhou, Koehler, Lord, Rogers, Sigman, Estes and Abbott2008).
In sum, after 32 years of taxometric research, the methodologically strongest evidence for the existence of taxa is in the areas of alcohol- and nicotine-related substance use and autism. Schizotypy should probably be added because a clear majority of the findings addressing it have been taxonic, although the only ones to use the CCFI have not (Rawlings et al. Reference Rawlings, Williams, Haslam and Claridge2008). We therefore suggest that the taxometric literature clearly supports the existence of taxa only in the areas of schizotypy, autism and substance use disorders. Ideally, researchers should go beyond identifying such taxa and demonstrate that they have construct validity, such as predicted patterns of association with external variables (Hasin et al. Reference Hasin, Liu, Alderson and Grant2006; Prisciandaro & Roberts, Reference Prisciandaro and Roberts2011), in the absence of which evidence for taxonicity can be questioned (Watson, Reference Watson2003). We also acknowledge that taxometrics is just one statistical approach to the question of latent structure, and any comprehensive analysis of the existence and distribution of latent categories in personality and psychopathology must integrate its findings with those of other approaches, which may differ systematically.
Conclusions
Taxometrics was launched as a rigorous method for ‘solving’ (Meehl, Reference Meehl1995) a fundamental question in the study of personality and psychopathology. Three decades of research allow us to review its answers. The collected evidence of this review is that latent categories are very scarce in normal and abnormal personality and infrequent but not absent in the broad arena of psychopathology. Taxa are most likely few and far between in the domains of internalizing and externalizing disorders, with the partial exception of substance use conditions. They may also occur in additional forms of psychopathology, notably schizotypy and autism. Other taxa may exist, but taxometric evidence for them is preliminary and runs against the strongly dimensional tide of recent research. Early taxometric research findings performed a valuable role in raising the issue of latent structure and giving credence to the possibility of psychological taxa, but their findings may often have been spurious. Taxometric research supports the conclusion that most psychological variation is dimensional, with a few potentially important exceptions.
Declaration of Interest
None.