Introduction
Not yap wa' Hol. If asked to translate, speakers of Klingon (an alien language from the TV series, Star Trek) would tell you that “one language is not enough.” Many would agree with this sentiment, judging by the ubiquity of multilingualism and the popularity of language learning platforms such as Duolingo – which, incidentally, offers Klingon. Beyond the utility for building imaginary worlds (as with Klingon, Elvish, or Dothraki), artificial languages can provide insight into how we acquire natural languages in the world around us. In fact, Klingon itself has been used to assess language learning aptitude, as the ability to map Klingon sounds to symbols can predict English language proficiency (Kiss & Nikolov, Reference Kiss and Nikolov2005). Artificial languages have proven to be useful and versatile tools for studying language acquisition by helping to control for the immense variability that exists within natural languages.
Over 7,000 natural languages are used worldwide, spanning more than 140 language families (Simons & Fennig, Reference Simons and Fennig2018). Languages can vary at every level of representation, including how they are written (e.g., alphabetic vs. logographic), pronounced (e.g., tonal vs. non-tonal), syntactically ordered (e.g., subject-verb-object vs. verb-subject-object), and expressed (e.g., vocally vs. manually). There is also variability within languages (e.g., words that differ in concreteness, frequency, pronounceability), and across language users (e.g., proficiency, exposure, cognitive abilities). As a result, isolating the influence of any one factor can be difficult, if not impossible, when studying natural languages. One of the primary advantages of using artificial languages is the flexibility to carefully control not only the learners’ prior experience with a language, but also the properties of the language itself. It allows experimenters to customize their stimuli to fit the exact linguistic requirements of a particular study, while avoiding unwanted variability often found in natural languages (De Graaff, Reference De Graaff1997).
One may question, however, whether learning a language stripped of idiosyncrasies can truly inform us about learning real languages. And certainly, even a fairly well-formed artificial language is bound to pale in comparison to the rich and vivid constellation of sensory inputs, linguistic structures, motor programs, thoughts, beliefs, and memories that become associated with natural languages. However, just as physicists can collide particles to study the origins of the universe, psycholinguists can use artificial languages to examine the linguistic system. Furthermore, the substantial overlap between artificial and real languages in neural activation (Friederici, Steinhauer & Pfeifer, Reference Friederici, Steinhauer and Pfeifer2002), as well as behavioral measures of second language learning aptitude (Ettlinger, Morgan-Short, Faretta-Stutenberg & Wong, Reference Ettlinger, Morgan-Short, Faretta-Stutenberg and Wong2016), speak to the ecological validity of using artificial languages in bilingualism research.
Within the broad area of artificial language learning, the present paper focuses on two lines of inquiry relevant to understanding bilingual word learning: the influence of language input, and the impact of bilingual experience. Together, these two components represent the wide spectrum of extrinsic (e.g., language) and intrinsic (e.g., learner) sources of variability that determine successful learning. After a brief overview on the origin and function of artificial languages, we provide concrete examples of how artificial languages have been used to study the effects of language and learner characteristics, ultimately shedding light on the emergence and consequences of bilingualism. Lastly, we consider the advantages and limitations of using artificial languages to study natural language processes, and suggest resources for constructing artificial languages before concluding with a discussion of potential future directions.
The origin and function of artificial languages
Artificial languages have had a long history, starting with Lingua Ignota created in the 1100s by a German abbess named Hildegard von Bingen. The use of artificial languages in research came much later, with American psychologist Erwin Allen Esper conducting one of the earliest artificial language studies in 1925. Around the same time, the artificial language of Esperanto, deliberately created to have highly regular morphological and syntactic rules, began to be taught in parts of Europe to help students understand parts of speech in their mother tongue, as well as to learn other Romance languages (Eaton, Reference Eaton1927). In research, artificial languages are often created to examine specific levels of representation, such as the BROCANTO (Friederici et al., Reference Friederici, Steinhauer and Pfeifer2002) and BROCANTO 2 languages (Morgan-Short, Sanz, Steinhauer & Ullman, Reference Morgan-Short, Sanz, Steinhauer and Ullman2010; Morgan-Short, Steinhauer, Sanz & Ullman, Reference Morgan-Short, Steinhauer, Sanz and Ullman2012), which have artificial grammars suitable for studying morphosyntactic processing. Artificial languages have also been used to simulate the emergence of natural languages (Van Trijp, Reference Van Trijp2010). Lastly, research using artificial languages can systematically vary linguistic parameters to facilitate investigations into the specific characteristics of languages and learners that contribute to effective word learning.
Using artificial languages to study the role of language input in word learning
The value of artificial languages is perhaps most transparent when examining the influence of particular word properties on second language vocabulary acquisition. For example, to investigate whether similarity to the native language (L1) facilitates novel word learning, Bartolotti and Marian (Reference Bartolotti and Marian2017) created a “wordlike” and “unwordlike” version of an artificial language called Colbertian (named after Stephen Colbert, comedian and inventor of neologisms such as “truthiness”). The two versions were constructed to either follow or violate the English rules for combining letters and sounds into words (e.g., nish vs. gofp) by manipulating orthographic neighborhood size (many or few similarly-written English words) and orthotactic probability (high or low likelihood of observing the novel word's letter sequence based on English patterns). In all other respects, the two versions were matched (e.g., their English translations, word length, etc.). After training and testing native English speakers on one of the versions, the researchers found that recognition and production were faster and more accurate for the wordlike language (see Figure 1 for example training and recognition test trials). Artificial language studies have revealed that learning can also be influenced by properties of the novel word's translation, such as cognate status (de Groot & Keijzer, Reference de Groot and Keijzer2000), as well as word frequency (de Groot, Reference de Groot2006). Novel word forms are also more likely to be recalled if they are associated with concrete rather than abstract concepts (de Groot & Keijzer, Reference de Groot and Keijzer2000; de Groot, Reference de Groot2006), demonstrating that how well we learn new words depends on properties at multiple levels of representation, including the phonological, orthographic, lexical, and semantic characteristics of both known and unknown words.

Fig. 1. Examples of Colbertian (a) training and (b) recognition test trials in the “unwordlike” condition (Bartolotti & Marian, Reference Bartolotti and Marian2017).
Investigating the factors that facilitate word learning can serve a practical function by informing instructors and students about what is likely to be more or less difficult to learn. Perhaps more significant, however, are the theoretical insights that can be derived from observing the variables that help and hinder second language word learning. For instance, the finding that concrete words are learned more readily than abstract words may have implications for how we conceptualize the structure of bilingual memory. There is evidence that the relationship between L2 words and their concepts is lexically mediated during early stages of word learning, so L2 words initially activate conceptual information via connections to L1 word forms (e.g., “nish” “apple”
apple concept; see Kroll & Stewart's (Reference Kroll and Stewart1994) Revised Hierarchical Model). The fact that semantic properties (e.g., concreteness) can affect how easily a learner is able to access an L1 translation suggests that L2 words can also directly activate conceptual representations in parallel, or before, activating L1 translations (e.g., “nish”
apple concept
“apple”; Potter, So, Von Eckardt & Feldman, Reference Potter, So, Von Eckardt and Feldman1984).
Other cases of conceptual mediation have been observed in studies that manipulate the linguistic context in which artificial words are embedded (e.g., word sets, sentences, streams of speech). For instance, learning artificial words in semantically-related sets (e.g., apple, orange, grape) can make it more difficult to produce their L1 translations, suggesting that conceptual information can interfere with the retrieval of L1 lexical information (Finkbeiner & Nicol, Reference Finkbeiner and Nicol2003; Tinkham, Reference Tinkham1993). This result indicates that the way we are exposed to a word can significantly impact acquisition. Artificial languages are therefore often used to identify linguistic contexts that facilitate learning (e.g., Kersten & Earles, Reference Kersten and Earles2001), as well as to understand how words embedded in challenging contexts are learned at all. The latter is particularly relevant to understanding how children exposed to multiple languages manage to extract the linguistic structures contained in two different, potentially inconsistent sources of information. Researchers are able to gain insight into this process by seeing how learners track statistical regularities from continuous streams of artificial words (e.g., Mitchel & Weiss, Reference Mitchel and Weiss2010) and tones (e.g., Bartolotti, Marian, Schroeder & Shook, Reference Bartolotti, Marian, Schroeder and Shook2011). For instance, it has been found that learners can rely on nonlinguistic cues (such as the voices and faces of speakers) to distinguish between languages and learn the distributional properties of each one (Weiss, Gerfen & Mitchel, Reference Weiss, Gerfen and Mitchel2009; Mitchel & Weiss, Reference Mitchel and Weiss2010).
In sum, artificial languages have been used to demonstrate that the ease of acquiring novel vocabulary differs depending on characteristics of the language to be learned, such as similarity to known languages and concreteness, as well as the surrounding linguistic context, such as adjacent words and sounds. These findings, in turn, inform our understanding of the structures and processes that enable the acquisition of multiple languages. See Table 1 for a summary of the reviewed studies examining the influence of language input on word learning.
Table 1. Examples of studies examining effects of linguistic input on artificial language learning
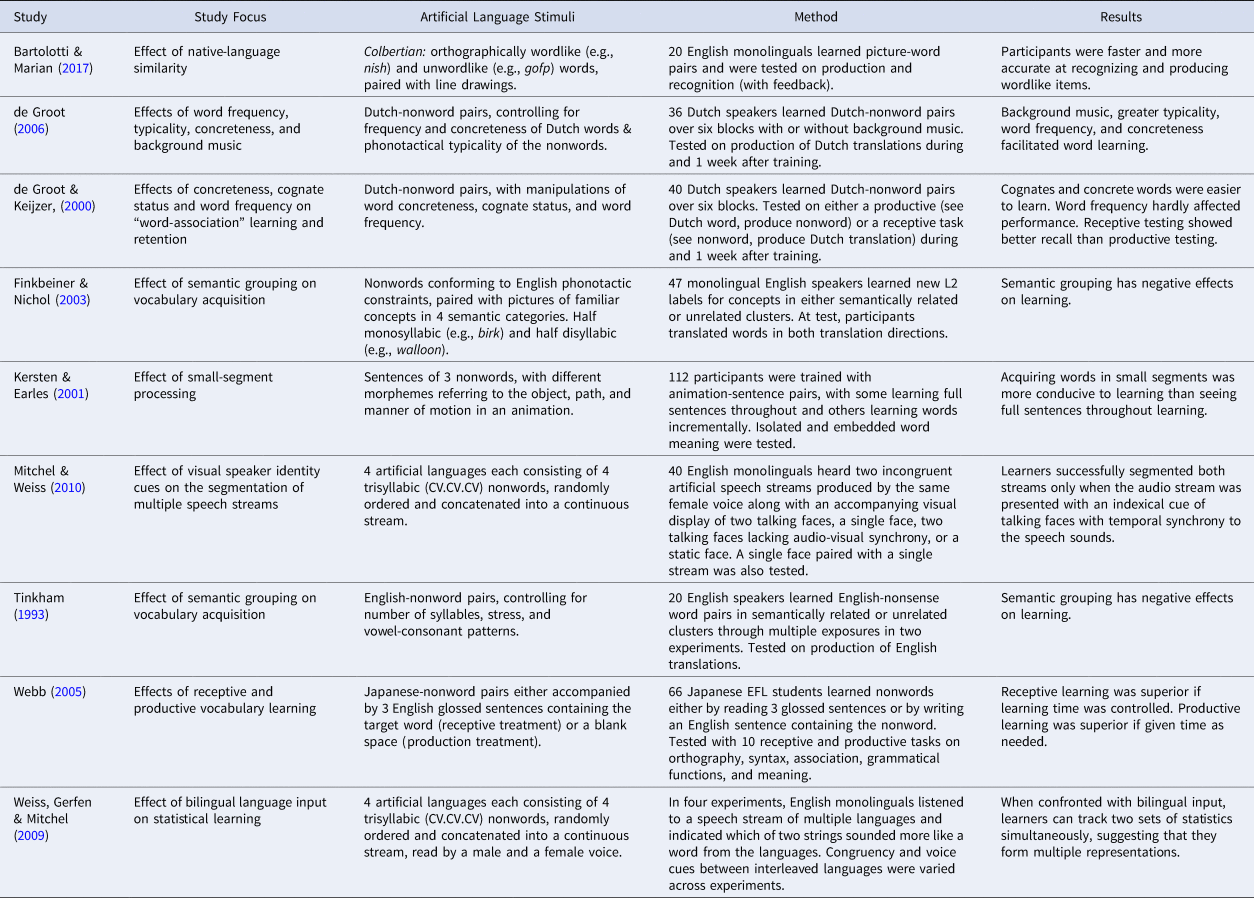
Using artificial languages to study the role of bilingual experience in word learning
Early in development, differences emerge in how monolingual and bilingual children learn words. When presented with a novel word and an array of possible referents, children have a strong tendency to infer that the label belongs to an unfamiliar, rather than a familiar object (i.e., “mutual exclusivity;” Markman & Wachtel, Reference Markman and Wachtel1988). However, studies using both artificial (Davidson, Jergovic, Imami & Theodos, Reference Davidson, Jergovic, Imami and Theodos1997) and natural languages (e.g., Byers-Heinlein & Werker, Reference Byers-Heinlein and Werker2009) have found that bilingual children are less constrained by mutual exclusivity, likely as a result of learning early on that objects often have two labels. Monolingual and bilingual children also rely on different sources of information to identify the referents of artificial words (Brojde, Ahmed & Colunga, Reference Brojde, Ahmed and Colunga2012). While monolinguals tend to focus on perceptual cues, such as an object's shape or color, bilinguals rely more on pragmatic cues, such as the eye gaze of people around them, possibly because of exposure to more complex social and linguistic environments. These findings demonstrate that bilingual experience can alter how children map words to meaning.
In addition to learning words differently, bilingual children and adults often learn words more effectively than monolinguals (e.g., Kaushanskaya & Marian, Reference Kaushanskaya and Marian2009a; Menjivar & Akhtar, Reference Menjivar and Akhtar2017; see Hirosh & Degani, Reference Hirosh and Degani2018 for a review). These advantages for language learning have been linked to both better phonological working memory and executive control (e.g., Antoniou, Liang, Ettlinger & Wong, Reference Antoniou, Liang, Ettlinger and Wong2015; Kaushanskaya & Marian, Reference Kaushanskaya and Marian2009a), as either could potentially increase an individual's ability to learn novel words (Kapa & Colombo, Reference Kapa and Colombo2014; Papagno, Valentine & Baddeley, Reference Papagno, Valentine and Baddeley1991). Greater phonological working memory and experience with diverse speech sounds could improve comprehension of foreign phonemes, and consequently, word learning (Kaushanskaya & Marian, Reference Kaushanskaya and Marian2009a), while greater cognitive control could help bilinguals inhibit interference from conflicting information. Take, for example, Yoshida, Tran, Benitez, and Kuwabara's (Reference Yoshida, Tran, Benitez and Kuwabara2011) finding that bilingual children outperform monolinguals on measures of both cognitive control and artificial adjective learning. The researchers interpreted this language learning advantage as stemming from more effective inhibition of word learning heuristics (such as the tendency to assume that labels refer to nouns). Similarly, Bartolotti and Marian (Reference Bartolotti and Marian2012) proposed that extensive experience managing conflict across multiple languages may make bilinguals especially adept at inhibiting lexical competition. In one study, Kaushanskaya and Marian (Reference Kaushanskaya and Marian2009b) had English monolinguals and English-Spanish bilinguals learn novel artificial language words with orthography-to-phonology mappings that differed from their native tongue. During control trials, participants would read an English word such as HOCKEY while listening to the novel word translation, pronounced /χf/. During critical trials, participants would additionally see the written form of the novel word, GEF, which did not correspond to its phonology based on English rules. While bilinguals generally outperformed monolinguals on subsequent vocabulary tests, this was especially the case for trials that included orthography-to-phonology mappings that conflicted with those in known languages. Yoshida et al. (Reference Yoshida, Tran, Benitez and Kuwabara2011) and Kaushanskaya and Marian (Reference Kaushanskaya and Marian2009b) thus demonstrate two different ways in which bilingual experience can enhance word learning in children and adults, respectively: the former by reducing interference from heuristics within a single language, and the latter by reducing interference from orthography-to-phonology mappings of other known languages.
As with studies explicitly examining the role of language input, using artificial languages to study group differences can help reduce unintended variation across stimuli and the likelihood of spurious effects. In Kaushanskaya and Marian (Reference Kaushanskaya and Marian2009b), using artificial languages made it possible to systematically manipulate the extent to which orthography-to-phonology mappings aligned with the learners’ known languages (while ensuring that other word properties, such as biphone and bigram probabilities, were equidistant from both known languages). An equally important function when comparing monolinguals to bilinguals, however, is the ability to ensure that neither group has had prior exposure to the novel language. This can be especially useful for experiments that include bilinguals from multiple different language backgrounds (as they often do). In Yoshida et al. (Reference Yoshida, Tran, Benitez and Kuwabara2011), for instance, using artificial words ensured that the stimuli were novel to all participants in their heterogeneous sample, whether their second language was Chinese, French, Spanish, Russian, Urdu, or Vietnamese. Converging evidence using natural languages (e.g., Van Hell & Mahn, Reference Van Hell and Mahn1997; Keshavarz & Astaneh, Reference Keshavarz and Astaneh2004), however, is valuable for ensuring that effects based on highly controlled, simplified languages are generalizable to richer, more complex languages. In sum, artificial languages have been used to shed light on how children learn words in different language environments, as well as why bilingual experience facilitates learning. See Table 2 for a summary of the reviewed studies examining the impact of bilingual experience on word learning.
Table 2. Examples of studies examining effects of bilingualism on artificial language learning

Conducting artificial language research: Limitations, opportunities, and resources
One limitation of both artificial and natural languages is that even strict controls cannot fully prevent the influence of unintended variables, as learning can be impacted by not only the word to be learned, but any word or concept associated with it. Note, however, that artificial languages still have an advantage over natural languages in this respect, as the latter introduce potential confounds for both novel and known languages. Yet studies using natural language stimuli can and do provide valuable insights, and their inherent complexity and richness can be an advantage in addition to an obstacle. Though artificial and natural language studies often converge upon the same conclusions, there may be cases in which discoveries based on natural language stimuli (e.g., French words) more reliably translate to real-world contexts (e.g., learning French in a lab vs. school), particularly given the impact that specific language properties can have on word learning. Highly-controlled artificial languages, on the other hand, are especially useful for reducing confounds and establishing causal mechanisms (e.g., enhanced inhibitory control), which can inform the generalizability of competence across different skill sets (e.g., learning language vs. music).
The utility of artificial languages for revealing causal mechanisms may additionally offer the opportunity to better understand cases in which bilinguals appear to have language learning difficulties, either due to legitimate language disorders, or less familiarity with the language being tested. Within the clinical field of Speech-Language Pathology, approaches such as Dynamic Assessment (Hasson, Camilleri, Jones, Smith & Dodd, Reference Hasson, Camilleri, Jones, Smith and Dodd2013) are often used to determine whether children who struggle on traditional language aptitude tests are able to acquire novel language rules (see Gutieérrez-Clellen & Penña, Reference Gutieérrez-Clellen and Penña2001). By controlling for potential confounds related to unintentional conflict or overlap with known languages, future research using artificial languages may help clinicians distinguish between language differences and language disorders among children from linguistically and culturally diverse backgrounds.
For individuals conducting their own word learning research using artificial languages, there are a number of useful resources available (Marian, Reference Marian2017). The CLEARPOND (Cross-Linguistic Easy-Access Resource for Phonological and Orthographic Neighborhood Densities) can be used to calculate and control for properties such as phonological and orthographic biphone and bigram frequencies, as well as neighborhood size and density, when creating words in an artificial language (Marian, Bartolotti, Chabal & Shook, Reference Marian, Bartolotti, Chabal and Shook2012). Databases such as SUBTLEX contain word frequency information in several languages including English (Brysbaert & New, Reference Brysbaert and New2009), German (Brysbaert, Buchmeier, Conrad, Jacobs, Bölte & Böhl, Reference Brysbaert, Buchmeier, Conrad, Jacobs, Bölte and Böhl2011), and Chinese (Cai & Brysbaert, Reference Cai and Brysbaert2010). Other tools such as the Simple Natural Language Processing tool (Crossley, Allen, Kyle & McNamara, Reference Crossley, Allen, Kyle and McNamara2014) can be used to analyze elements of discourse processing, such as syntactic complexity. While it should be noted that the majority of resources are based on English and other Indo-European languages, tools such as CLEARPOND allow users to upload custom lists to calculate lexical neighborhood statistics. These and other similar resources make it possible to use information about natural languages as a basis to generate artificial language stimuli with variable degrees of similarity to real languages (e.g., Bartolotti & Marian, Reference Bartolotti and Marian2017). They can also be used to control the natural language translations of artificial words (e.g., de Groot & Keijzer, Reference de Groot and Keijzer2000), as well as any natural language stimuli that may be integrated with artificial languages (e.g., Webb, Reference Webb2005).
Concluding remarks
At face value, learning a word may seem like a trivial task. Upon closer examination, it becomes apparent that the ability to learn a word belies an intricate system of perceptual schemas, cognitive functions, linguistic representations, and conceptual knowledge – made particularly complex with the introduction of multiple languages. With properly controlled stimuli, however, the ability to learn a word can provide significant insight into how the bilingual cognitive system functions. Because artificial languages allow fine-grained control over linguistic properties and prior language exposure, they can be useful tools to investigate how word learning is influenced by external factors, such as the language to be learned, as well as learner characteristics, such as language experience.
Understanding how words are learned has obvious practical utility, whether it is in service of diagnosing and treating language or learning disorders or in facilitating the acquisition of foreign languages. More fundamentally, however, how we learn words reflects our capacity to understand, generate, and see relationships among a system of symbols. This ability, which emerges early and universally in human development, is the very basis of complex reasoning and abstract thought – one that appears to out-pace all other animals and has proven difficult for artificial intelligence and machine learning. Though artificial languages, so far, have primarily been used to study how we learn rules and structures that are inherently tied to language (such as phonology, orthography, morphology, and syntax), future research may address the open question of whether artificial languages can be profited from to better understand the more generative side of language and the development of higher-order functions, such as analogical reasoning and event structure learning. With their capacity to distill complex processes down to their critical components, artificial languages may hold the key to understanding uniquely human abilities that continue to confound even the most advanced forms of artificial intelligence.
Acknowledgements
Preparation of this manuscript was supported by grant R01 HD059858 to Viorica Marian. We thank the members of the Bilingualism and Psycholinguistics Research Group for their helpful comments.