INTRODUCTION
Despite arguments of a “post-racial” America, race and ethnicity continue to occupy a central role in our understanding of politics. Indeed, questions of how racial/ethnic identity influences elections and politics may be more salient today than ever before. Yet efforts to measure, monitor, and address the racial/ethnic disparities in politics, and particularly elected office, are limited by the lack of systematic data identifying the race of candidates. As a result, we continue to know relatively little about the myriad ways in which candidate attributes shape campaigns, elections, and governance, and this problem is exacerbated for sub-national offices. Questions particularly relevant today that are difficult to answer include: when and why do local political offices serve as a political pipeline for women or racial/ethnic minorities? What draws persons of color into the electoral arena? What is the role of candidate quality in attaining local office and beyond? How does campaign fundraising and coalition-building differ for women and persons of color, and does it matter for electoral success?
Scholars of the politics of race and ethnicity routinely grapple with these questions. However, when tasked with answering them, it becomes clear that there is comparatively little data on the race and ethnicity—and gender—of candidates. Among candidates that lose elections, the problem of missing data is even worse. Individually, scholars have painstakingly “expert” coded thousands of candidates, but this method is limited and limiting in at least two ways. First, “expert” coding is costly in terms of resources. As an example, approximately 15,000 candidates ran for state legislative office in 2012. Juenke and Shah (Reference Juenke and Shah2015) coded 4,000 of these in 15 states, and estimate a combined 300 h spent coding the sample. Second, these costs limit the data available. Researchers generally create only small samples of data coded at one time point, and most often these small samples are of candidates running for higher level offices. Thus, questions about general patterns of descriptive representation of all candidates running for mayor across the United States, for example, are still largely unanswered.
The continued absence of systematically collected race and ethnicity data for candidates motivates this research note. First, we review two alternative methods of assigning race/ethnicity to individuals: one, a Bayesian analysis that utilizes Census surname lists and population distributions within a geography to estimate race; and two, an innovative crowdsourcing platform that allows many contributors to classify the racial identity of candidates. Second, we compare these methods against expert coding along two dimensions—cost and accuracy.
Our results show that these alternative methods are less costly in terms of time (and money, if individuals are paid to code candidates). Yet, the accuracy of these alternative methods varies depending on the race of the candidate being coded. In particular, we find that the Bayesian and crowdsourcing methods have the most agreement in determining the race of white candidates. When coding the race of Latino, Asian, Black, and Native American candidates these methods tend to be less accurate than expert coding, although there is interesting variation between the racial groups. We conclude with implications of these results for scholars of race and politics, and what these results mean for scholars interested in assigning race and ethnicity categories to individuals.
HYBRID GEOCODING AND SURNAMES
Geocoding links an individual's geography to a census measure of that geography's racial/ethnic population distribution, and uses that measure as a basis for inferring the individual's race/ethnicity. In particular, geocoding takes advantage of segregation patterns within cities, and thus the degree of correspondence between area and individual characteristics generally increases when smaller, more homogenous units are used, such as Census tracts (Enos Reference Enos2015). For example, knowing that a candidate resided in a Census Block Group where 90% of the residents are African American provides useful information for estimating that person's race.
Surname analysis infers race/ethnicity from surnames (last names) that are distinctive to particular racial/ethnic groups. Initially, surname analysis entailed using dichotomous dictionaries to identify Hispanics and various Asian nationalities (Census 1990, 2000). Construction of these lists emphasized high specificity (i.e., persons whose surnames appear on the list have a high probability of self-reported Hispanic ethnicity or Asian race, respectively). More detailed lists have been generated for Asian sub-nationalities (Lauderdale and Kestenbaum Reference Lauderdale and Bert2000) and Arab Americans (Morrison and Coleman Reference Morrison and Coleman2001).
Both surname and geocoding methods have recognized limitations. Surname lists often cannot distinguish between African Americans and non-Hispanic whites, and geocoding options often produce low probabilities that cannot guide the researcher to distinguish between Asians and Latinos. Others have also identified this problem, and have offered a hybrid Bayesian approach (see Elliott et al. Reference Elliott, Fremont, Morrison, Pantoja and Lurie2008) that we adopt.
We use the following Bayesian formulaFootnote 1 to calculate the conditional distributions of the posterior probability g that a candidate i with a given surname S is of one of the following racial groups G: White (non-Hispanic), Black/African American, Hispanic (not White or Black), Asian, or Native American.

We generate a “baseline racial prevalence” (prior probability, p i ) based on the racial/ethnic composition of the Census tract to which the residence of the individual was geocoded. We then use the surname lists to update the prior probabilities of membership in each of the categories. Taken together, we now have a posterior probability Pr(g i |S) of a candidate's race based on the probability that any given candidate belongs to racial group G given their surname and geocoded racial composition.
CROWDSOURCING
In addition to the Bayesian approach, we examine the accuracy of a crowdsourcing method of estimating race and ethnicity. Crowdsourcing is most simply defined as the process of leveraging public participation in or contributions to projects and activities. As Benoit et al. (Reference Benoit, Conway, Lauderdale, Laver and Mikhaylov2016) note:
The core intuition is that, as anyone who has ever coded data will know, data coding is “grunt” work. It is boring, repetitive and dispiriting precisely because the ideal of the researchers employing the coders is – certainly should be – different coders will typically make the same coding decisions when presented with the same source information.
Other disciplines have already acknowledged that research that relies upon data about the “natural world” is often hindered or rendered impossible by the high cost of data collection and analysis. This realization has lead to the development of a number of crowdsourced and citizen science projects to collect data on bird habitats (eBird), astronomical photographs (GalaxyZoo), and bee pollination (Great Sunflower Project). In each of these examples, the needed data are crowdsourced: data coding and collection tasks are undertaken by an individual or organization via a flexible open call.
Crowdsourcing in the social sciences is relatively nascent, in part because of concerns of reliability and validity. A number of recent studies have examined these concerns (see, e.g., Berinsky, Huber, and Lenz Reference Berinsky, Huber and Lenz2012; Benoit et al. Reference Benoit, Conway, Lauderdale, Laver and Mikhaylov2016; Horton, Rand, and Zeckhauser Reference Horton, Rand and Zeckhauser2011; Paolacci, Chandler, and Ipeirotis. Reference Paolacci, Chandler and Ipeirotis2010), and have found a general consistency between crowdsourced data and those derived from more “traditional” sources, but emphasize the necessity to ensure coder quality. We would argue, however, that while we do not often question “expert” coded data, these too require assessment of validity and reliability. Several empirical studies have found that while a single expert typically produces more reliable data, this performance can be matched and sometimes even improved, at much lower cost, by aggregating the judgments of several non-experts (Alonso and Baeza-Yates Reference Alonso, Baeza-Yates, Clough, Foley, Gurrin, Jones, Kraaij, Lee and Mudoch2011; Alonso and Mizzaro Reference Alonso and Mizzaro2009; Hsueh, Melville, and Sindhwani. Reference Hsueh, Melville and Sindhwani2009; Snow et al. Reference Snow, O'Connor, Jurafsky and Ng2008).
Moreover, the coding of race and gender might be best suited to multiple “experts.” Race as an identifying feature of other humans has become “common sense” (Omi and Winant Reference Omi and Winant1986), based on a number of factors, including language, physical characteristics, and behavior. As Rhodes, Hayward, and Winkler (Reference Rhodes, Hayward and Winkler2006) note, we are all face experts, with a remarkable ability to distinguish thousands of faces, despite their similarity. Moreover, we learn to “know” what surnames are distinctive (Nicoll, Bassett, and Ulijaszek Reference Nicoll, Bassett and Ulijaszek1986), and often use these to assign race as well.Footnote 2 A large body of psychology literature reports that for many people, expertise in coding race is greater for own-race faces than for other-race faces, resulting in less accurate judgments of age (Dehon and Brédart Reference Dehon and Brédart2001) and sex (O'Toole, Peterson, and Deffenbacher Reference O'Toole, Peterson and Deffenbacher1996) for other-race faces. These effects are robust for people from different races (Bothwell, Brigham, and Malpass Reference Bothwell, Brigham and Malpass1989) and can impair eyewitness memory for other-race faces (Meissner and Brigham Reference Meissner and Brigham2001). Scholars of psychology argue that this other-race effect may reflect reduced perceptual expertise in processing other-race faces (Chiroro and Valentine Reference Chiroro and Valentine1995; Furl, Phillips, and O'Toole Reference Furl, Phillips and O'Toole2002; Goldstone Reference Goldstone2003; Meissner and Brigham Reference Meissner and Brigham2001). Thus, it may be more advantageous, and lead to greater convergence on the “truth” to have multiple experts code race.
THE EXPERIMENT
Our primary objective here is to compare across three methods of measuring race: hybrid Bayesian estimates, crowdsourced data, and expert coding. Given the prevalence and history of using expert coding in the social sciences, we consider the measurements from this method as the baseline. We evaluate the accuracy of the Bayesian and crowdsourced methods against this baseline.
To carry out this experiment, we report the results of an experiment coding the race of candidates for mayor in CA in 2012. CA is a great test state for a number of reasons. First, CA has a large Latino population, and a sizable Black and Asian population, meaning that the likelihood of racial heterogeneity is greater than in other states. Second, the Sacramento State Institute for Social Research California Elections Data ArchiveFootnote 3 creates a list of all candidates running in local races every year. In all, 222 candidates ran for mayor in 87 cities in CA.
For the expert coding, a single expert was provided with the list of candidate names and locations, and asked to fill out a spreadsheet with race/ethnicity (White, Asian, Latino, Black, Native American), gender (Male and Female) and provide the source of the information (website with link to biography, picture, campaign website, newspaper article, etc.). To extend these coding tasks to the “crowd,” we set up a web-based interface. For this experiment, the crowd was limited to a set of undergraduate students at a large midwestern university. To ensure each candidate was coded a number of times, a list was divided among the students for coding. In all, each candidate was coded at least three times by the “crowd.” Last, we employed the hybrid geocoding/surname method. Following the formula in the preceding section, we collected Census data to inform racial group probabilities for the 220 candidates in our data candidate. To construct the prior probability for each candidate, we acquired voter registration data from the California Office of the Secretary of State, and matched registration information (primarily addresses) using the first and last name and city of each candidate in our dataset.Footnote 4 Next, we used geocoding services available online from Texas A&M Geoservices to code candidate street addresses to Census tracts. Using tract-level Census data on racial composition, the prior probability for each candidate was assigned.Footnote 5 Then, the Census surname list supplied the conditional probability used to update the prior. Where the conditional value was unavailable, typically due to a name not found in the surname list, the prior was not updated, and thus became the posterior probability of candidate race (53 of 220 candidates or 24%). These three approaches are summarized in Table 1.
Table 1. Summary of approaches

In order to compare results of each alternative method to the expert, each candidate received a single-race assignment. For the crowd method, race was coded by assigning the modal value of crowd responses.Footnote 6 For the Bayesian estimation, candidate i’s race was calculated simply by taking the largest probability of all the possible race/ethnicities.
In addition to evaluating the accuracy of alternatives to “expert” coding, we consider these approaches to have important differences in terms of their respective limitations. For researchers, data collection can be very time-consuming and therefore it presents an opportunity cost. Researchers also must consider technical skill limitations. Table 2 lists relative values for of these two dimensions of cost for each method.
Table 2. Limitations of approaches

* To process existing code using R.
We consider the “expert” coding approach to present the highest total cost. The time required for the researcher is relatively high when compared with the alternatives, since the researcher must evaluate every candidate record herself. This approach also requires a moderate technical skill. The “expert” must be able to evaluate the race of candidates accurately, meaning that the researcher should familiarize themselves with the process and establish qualitative coding practices to this end.
Relative to the “expert” method, the crowdsourced method presents the least amount of limitations. For the researcher, the time involved in initiating the data collection effort and monitoring the results is rather low. For each participant from the crowd, the time involved is also low. In our experiment, members of the “crowd” spent on average 17 min coding about 30 candidates.Footnote 7 The technical skill level of the researcher and each member of the crowd need not be as high as the single “expert” approach, as increasing the number of people in the crowd also increases the likely accuracy of the method. Members of the crowd need not develop qualitative coding practices.
The Bayesian Hybrid approach faces limitations less than the “expert” approach but greater than the crowdsourced method. The time required of the researcher to implement the approach is trivial, since the code already has been developed to implement the estimation of race using surname lists and geocoded information. As the number of candidates to be coded increases, the amount of processing time does not appreciably change. This is a great improvement over the “expert” and “crowd” methods, which require more time when the list of candidates increases. However, this trivial constraint on time is matched with a rather high constraint in technical skill, since understanding and implementing the prexisting code requires knowledge of the R programming language, access, and ability to geocode candidate records, and access to the surname list(s) relevant to the candidates in the sample. While not costly in terms of time, the setup of the Bayesian hybrid approach is an involved process.
EVALUATION
Tables 3 and 4 show the comparison of race coding between the expert method and each alternative. Figure 1 provides a visual representation of “Agreement of Expert.” Beginning with the Bayesian/Geocoding method, a number of points are noteworthy. First, this method of coding candidates performed best at coding White and Latino candidates: we find 94% correlation with the expert on White candidates, and 91% correlation on Latino candidates. Second, the Bayesian/Geocoding methods performed terribly in coding Asian, African American, and Native American candidate names. Indeed, all eight Black candidates were coded as White. Agreement is the proportion of the expert coded candidates that were coded “correctly” by each alternative method.

Figure 1. Method comparison by race.
Table 3. Comparison of expert and Bayesian methods

Note: Total indicates the number of candidates each method assigned to each race category.
Table 4. Comparison of expert and crowd methods

Note: Total indicates the number of candidates each method assigned to each race category. Agreement is the proportion of the expert coded candidates that were coded “correctly” by
A number of factors may have influenced the accuracy of the hybrid Bayesian estimation. The first is geographic specificity. Previous studies of the effectiveness of the method (Elliott et al. Reference Elliott, Fremont, Morrison, Pantoja and Lurie2008; Enos Reference Enos2015) had either Census tract-level data or actual addresses, which should dramatically improve the Bayesian results. Unfortunately, even in candidate databases such as the one created by Sacramento State, individual addresses are not provided. Thus, as we described above we were sometimes forced to use city-level demographics, which depreciated our ability to code Asians and Blacks in our sample.
Second, given that this method relies on strong segregation patterns within a city, racial, and ethnic groups that comprise a smaller proportion will suffer from less specificity. For example, even using tract-level Census data did not help the estimation of Black and Native American candidates. It is clear why the eight Black candidates identified by the expert method were not identified; the Bayesian estimation for probability that a candidate was Black ranged from zero to 15%, with a mean of about 4% in these CA cities. Therefore, none of the candidates would be picked as Black, since these values are too small to be the maximal probability.
Third, while the most recent Census surname list (released in 2007) is an improvement upon older lists, it continues to suffer from a number of weaknesses that are particularly acute for Asian, Black, and Native Americans. One issue is that the list is not geographically specific, and thus provides national averages for names. For example, none of the most common surnames for African Americans—Washington (90% Black), Jefferson (75%), Banks (54%), Jackson (53%)—occur in our dataset. This might be a function of a small dataset, but may also say something about regional variations in names.
Last is the issue of ethnic differences within groups, particularly Asian Americans. The top surnames on the Census list are Chinese, followed by Vietnamese. Thus, for example, the Indian surnames in our dataset (Akbari and Natarajan) are not picked up. Similarly, identifying a Native American from surname and residence remains difficult using national level lists and tract data. Surnames contribute almost no information; the most predictive Native American surname is Lowery, which indicates only a 4% chance of being AI/AN (Word et al. Reference Word, Coleman, Nunziata and Kominski2008). Residential location would likely be highly informative for a candidate living in one several Native American reservation areas in the southwest (Elliott et al. Reference Elliott, Fremont, Morrison, Pantoja and Lurie2008), but this is generally unavailable.
It is important to note that the hybrid Bayesian approach described here need not always succumb to the problems revealed by this experiment. The accuracy of the Bayesian approach is directly and inversely related to the coarseness of the input data. As population estimates use increasingly specific geocoding, the variation of the prior probabilities will increase, since it is possible to identify geographies where Black residents are more than 15% of the population.Footnote 8 The lack of candidate addresses in this analysis certainly exacerbated the problem, since the Census data for some individuals was even less precise. For other candidate lists this may not be a problem. Coarseness of surname lists, as suggested above, also presents a limitation to this analysis that is not necessarily a hindrance where regional lists or sub-group lists are available.
There are some potential remedies for the problems experienced using this type of hybrid approach. Several of the best examples of the hybrid Bayesian approach come from research on health care services. Elliott et al. (Reference Elliott, Fremont, Morrison, Pantoja and Lurie2008) establish a basic hybrid approach, which combines standard Census surname list and geocoded address data approaches to estimate the race and ethnicity of health care recipients. Elliott et al. (Reference Elliott, Fremont, Morrison, Pantoja and Lurie2008) make improvements on the original hybrid approach by updating the surname list used and applying a different approach in dealing with “other race” candidates, who were mostly Hispanic in their sample. The authors found that their improvements led to a 19% improvement in classification (Elliott et al. Reference Elliott, Fremont, Morrison, Pantoja and Lurie2008). The improved hybrid approach serves as the foundation of our Baysian hybrid method, and it is important to note that these studies also suffer from a coarseness of data problem.
The hybrid Bayesian approach a useful method for identifying the race of individuals for health care application purposes, but some racial categories are less well predicted relative to others (Adjaye-Gbewonyo et al. Reference Adjaye-Gbewonyo, Bednarczyk, Davis and Omer2014; Elliott et al. Reference Elliott, Fremont, Morrison, Pantoja and Lurie2008; Imai and Khanna Reference Imai and Khanna2016). Most studies find that Asian and American Indian or Alaskan Native categories, as well as multi-racial identification is comparatively more difficult to predict accurately. Adjaye-Gbewonyo et al. (Reference Adjaye-Gbewonyo, Bednarczyk, Davis and Omer2014) find that overall such improved Bayesian hybrid approaches are more accurate for males than females. Using voter file data, Imai and Khanna (Reference Imai and Khanna2016) indicate that adding additional characteristics of individuals, such as party registration could improve the accuracy of the Bayesian method in some cases.
Overall the research employing the improved Bayesian hybrid approach reveals that the approach is accurate enough to improve upon existing methods, such as surname list or geocoded data only approaches, contingent on data specificity. Imai and Khanna (Reference Imai and Khanna2016), for instance, have access to verified FL voter registration data, which include individual addresses. In our data, it was necessary to match address information to the candidates from the CA voter file. For over 16% of our candidates, there were no matches. This problem was not an issue for Imai and Khanna (Reference Imai and Khanna2016).
We contend that without finer geographic information, other variables such as gender are unlikely to considerably improve the hybrid approach as configured here. This is, however, an empirical question that future research could assess when more complete information about candidate addresses is available for states such as CA.
Turning to the crowdsourcing results, the findings presented in Table 4 and Figure 1 suggest that “crowd” performed relatively well, particularly for White and Black candidates. The largest degrees of discrepancy occurred around Latino and Asian candidates. In Table 5, we provide a list of the names for which there was disagreement between the crowd and expert, as well as the Bayesian coding. Again, a number of interesting patterns emerge. First, the crowd twice mistakenly coded the Asian candidates as Native Americans. This may be an anomaly of the “crowd” used in this pilot—students from a Midwestern city who may have had less experience with Asian last names or perhaps confused Indian and American Indian. A more expansive, nationally representative crowd should ameliorate this issue.
Table 5. Candidates coded differently between expert and crowd
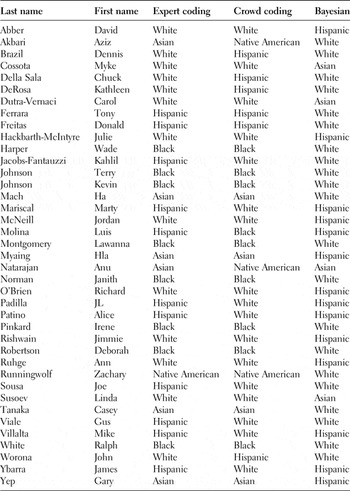
Second, the crowd had difficulty with differentiating the Latino candidates from the Italian candidates, of which there were quite a few in our sample dataset. Others have noted similar issues with Filipino surnames. In examining how the “crowd” determined race for these candidates, we find that most used pictures and surnames. Moving forward, these findings suggest that we may need to educate the crowd on the differences between Italian, Filipino, and Latino surnames, and the need to read into other detailed information such as biographies and newspaper stories to obtain additional information.
CONCLUSIONS
Scholars and community advocates continue to bemoan the underrepresentation of minorities, but are still struggling with understanding how the supply of minority candidates influence their representation (Juenke and Shah Reference Juenke and Shah2015). Political scientists interested in examining the relationship between candidate race and a number of other factors are often constrained by the available data; to date, there have been few systematic or sustained data collection efforts to gather candidates’ (as opposed to winners’) racial/ethnic characteristics.
That said, questions of how race and ethnicity intersect with political behaviors and institutions are tantamount in American politics. A large portion of race and politics research focuses specifically on the relationship between candidates and elected officials of color, and their constituents. Testing this relationship, however, requires scholars to know the race and ethnicity of both the candidates who won elections, and those candidates who lost; and while there continue to be significant improvements in the development of candidate lists (i.e., Ballotpedia, the New Organizing Institute, Who Leads Us?), to date these lists do not include the race or ethnicity of the candidate.
In this research note, we have examined two alternative methods of gathering race and ethnicity data, and compared them with expert coding. Our findings suggest that neither method is a perfectly interchangeable with expert coding, but can provide useful starting points for researchers interested in coding race and gender of individuals. Although expert coding continues to represent the gold standard, indirect methods offer a powerful and immediate alternative for estimating candidate race/ethnic status using more readily available data.
These alternative methods also have a relatively large benefit in terms of resources. Expert coding is inherently costly, since it takes the time and resources of an “expert” coder. Even with candidate lists as short as the one used here, the coding process is time-consuming. If more than one expert is used to check reliability, then these costs increase. The Bayesian approach requires less time, but imposes different costs in terms of resources. For this approach, the researcher must have an advanced knowledge of a statistical package such as R, and access to geocoding software. The process of estimating the race of the candidates utilizing the Bayesian approach, unlike the expert coding approach, is only minimally affected by the length of the candidate list.
The crowdsourcing option, however, presents a new technology to minimize both time and resource costs. Drawing on a vast pool of “experts” to carry out human judgments is a scalable, cost-effective, and efficient means to code data. Yes, there are start-up costs, but once paid, the crowdsourced method reduces the costs on the researcher to nearly zero. The researcher then only needs access to the aggregated and replicated data, and adjudicate discrepancies.
Questions about how race intersects with politics will be continue to be salient, and thus future work will be needed to continue to refine these methods. One possibility would be to develop regional sensitivity and specificity parameters for Census surname lists. Another possibility would be to gather Census racial/ethnic data within block groups that were restricted to ages that better matched the target population. Last, we believe crowdsourcing may provide many tools to scholars interested in gathering a number of demographic characteristics of candidates and voters.