1. Introduction
Finnish, like several other Finno-Ugric languages such as Hungarian (É. Kiss Reference É. Kiss1987, Reference É. Kiss2008), exhibits relatively few constraints on word order in a finite clause (e.g. Lindén Reference Lindén1947; Hakulinen Reference Hakulinen1975, Reference Hakulinen1976; Hakulinen & Karlsson Reference Hakulinen and Karlsson1979; Vilkuna Reference Vilkuna1989; Palander Reference Palander1991; Holmberg & Nikanne Reference Holmberg, Nikanne and Svenonius2002). Often all or nearly all mathematically possible word permutations are attested. Some are illustrated in (1)−(2).Footnote 1
-
(1)
-
(2)
As there is no independent evidence for radical nonconfigurationality in this language (van Steenbergen Reference van Steenbergen, Marácz and Muysken1989, Manninen Reference Manninen2003, Brattico Reference Brattico2019b), most studies have approached the phenomenon by relying on syntactic displacement, often using discourse features as drivers (Hakulinen Reference Hakulinen1975; Vainikka Reference Vainikka1989; Vilkuna Reference Vilkuna and É. Kiss1995; Koskinen Reference Koskinen1998; Nelson Reference Nelson1998; Holmberg Reference Holmberg and Svenonius2000; Kaiser Reference Kaiser2000, Reference Kaiser2006; Holmberg & Nikanne Reference Holmberg, Nikanne and Svenonius2002; Boef & Dal Pozzo Reference Boef and Dal Pozzo2012; Huhmarniemi Reference Huhmarniemi2012; Brattico Reference Brattico2018). Yet, no analysis exists that accounts for the free word order phenomenon as a whole. This has left many word order phenomena, reviewed in the next section, unaccounted for.
The following analysis of the Finnish free word order phenomenon will be proposed in this article. It will be argued that the human language parser can map both word order and rich morphosyntax into hierarchical order. Thus, in a language such as Finnish in which morphosyntax is rich, case suffixes guide arguments into their canonical positions. In a language with impoverished morphosyntax, canonical positions are recovered from order. A computational theory of the language comprehension, adopting ideas from the left-to-right minimalism (Phillips Reference Phillips1996, Reference Phillips2003; Chesi Reference Chesi2012; Brattico Reference Brattico2019a; Brattico & Chesi Reference Brattico and Chesi2020) and Dynamic Syntax (Kempson, Meyer-Viol & Gabbay Reference Kempson, Meyer-Viol and Gabbay2001, Cann, Kempson & Marten Reference Cann, Kempson and Marten2005) is developed that implements these properties (Section 4) and is tested against a corpus of 119,800 Finnish word order combinations by computer simulation (Section 5).
2. Finnish word order
The data in (1)−(2) could lead one to believe that all mathematically possible word order permutations are possible in Finnish. Indeed, some authors have proposed that Finnish is (either wholly or partially) nonconfigurational and obeys no or few syntactic constraints (for various proposals, see Välimaa-Blum Reference Välimaa-Blum1988; Vilkuna Reference Vilkuna1989; Sammallahti Reference Sammallahti2002, Reference Sammallahti2003; Helasvuo Reference Helasvuo, Luraghi and Parodi2013). Syntactic explanations are typically substituted with pragmatic ones, as the word order correlates with discourse interpretation. On the other hand, virtually all (or all) studies that have investigated the matter by using evidence outside of word order have concluded that Finnish is configurational (Hakulinen Reference Hakulinen1975; Vainikka Reference Vainikka1989; van Steenbergen Reference van Steenbergen, Marácz and Muysken1989; Koskinen Reference Koskinen1998; Nelson Reference Nelson1998; Holmberg Reference Holmberg and Svenonius2000; Holmberg & Nikanne Reference Holmberg, Nikanne and Svenonius2002; Kaiser Reference Kaiser2000, Reference Kaiser2006; Manninen Reference Manninen2003; Boef & Dal Pozzo Reference Boef and Dal Pozzo2012). Independent syntactic phenomena, such as binding, morphosyntax, operator movement, topicalization, ellipsis, control and quantifier scoping behave as if the language would exhibit structural asymmetries (Brattico Reference Brattico2019b). Furthermore, free word order is restricted to finite domains, with infinitival constructions operating under much less free regime. I will assume in this article that Finnish is configurational.
If Finnish is configurational, then (1)−(2) must emerge by means of grammatical displacement, and the problem is what the displacement operation is. Certain facts can be regarded as uncontroversial. The Finnish preverbal subject position is preceded by an operator position (glossed here as C/op) that is filled in by operator-like elements, such as interrogative pronouns, relative pronouns or contrastively focused phrases, and the filling mechanism is operator/A´-movement (Vainikka Reference Vainikka1989, Vilkuna Reference Vilkuna and É. Kiss1995, Huhmarniemi Reference Huhmarniemi2012, Huhmarniemi & Brattico Reference Huhmarniemi and Brattico2013). It can also be filled in by T-to-C head movement. Thus, only specifically marked or interpreted elements can occur in this position. It is not a ‘free’ position.
The operator position is followed by a preverbal subject position that can be filled in by a phrase of almost any type, which tends to receive a topic reading (Vilkuna Reference Vilkuna1989, Holmberg & Nikanne Reference Holmberg, Nikanne and Svenonius2002, Huhmarniemi Reference Huhmarniemi2019). The discourse-configurationality of the Finnish preverbal subject position together with the observation that it can be filled in by almost any kind of phrase accounts for some of the Finnish noncanonical word orders. The standard template, based on the evidence discussed in the sources mentioned and first proposed by Vilkuna (Reference Vilkuna1989, Reference Vilkuna and É. Kiss1995), is illustrated in (3).
-
(3)
Here the consensus ends, however. A mechanical generation and evaluation of all logically possible words orders in basic Finnish finite clauses, performed by the author, revealed a number of phenomena unexplained by (3) or by anything else found in the current literature. These inadequacies constitute the motivation for the present study. I will review them next.
First, Finnish head movement is not restricted to local domains. The fact that head movement is nonlocal creates word order variations that cannot be and have not been accounted for by the standard analysis above. Two examples of nonlocal head movement are provided in (4).
-
(4) Nonlocal head movement
While it is not trivial that these data exhibit long-distance head movement, I refer to them theory-neutrally as ‘nonlocal head movement constructions’ in this article.Footnote 2
There are also grammatical (if marginal) sentences in which a head has moved over another head into a position that does not exist in the standard model. Example (5) below illustrates this problem. Here the main verb moves over the negation into a position between C and Neg that is not posited by the standard model.
-
(5)
Let us consider the properties of this construction more closely. The negation e-i ‘not-3sg’ is either its own Neg head or occurs in a separate finiteness head, both above T. This is justified on the grounds that the negation agrees in ϕ-features with the grammatical subject and occurs above the tensed verb (Holmberg et al. Reference Holmberg, Nikanne, Oraviita, Reime, Trosterud, Holmberg and Nikanne1993, Holmberg & Nikanne Reference Holmberg, Nikanne and Svenonius2002, Thomas Reference Thomas2012). Whatever (e.g. Neg, finiteness head) hosts the negation is selected by C/op, which provides the position for the raised verb in (5). The subject must be in the specifier of C/op, as shown by the analysis (6a). The problem is that if a head moves to C/op, no phrase can fill its specifier (Vilkuna Reference Vilkuna1989, Reference Vilkuna and É. Kiss1995; Huhmarniemi Reference Huhmarniemi2012; Brattico et al. Reference Brattico, Huhmarniemi, Purma and Vainikka2013). This general and virtually exceptionless limitation is illustrated in (6b).
-
(6)
In addition, (5) is not interpreted so that ‘Jari’ constitutes the contrastive/corrective topic or focus, as it should were it located at Spec,C/op (Vilkuna Reference Vilkuna1989, Reference Vilkuna and É. Kiss1995). Therefore, example (5) is hard to reconcile with the standard analysis.
Let us leave the issue and address other problems of the standard analysis. The number of topics seem not to be limited in any principled way (7a), although an increase in the number of topics seems to correlate (in author’s judgment) with an increase in marginality; and much more if the topics are stacked above the negation (7b). To me, (7a) has a natural reading in which Pekka, the book and Merja are known from previous discourse and constitute topics. This phenomenon has been documented in previous literature (see, in particular, Vilkuna Reference Vilkuna1989), but no systematic analysis exists, to my knowledge.
-
(7) Several topics
These topics occupy position(s) that the standard analysis does not project. Notice that they occur above the auxiliary in (7a), so that the position of the lexical verb is irrelevant.
Fourth, it is possible to dislocate arguments towards the end of the clause, a phenomenon first discussed by Vilkuna (Reference Vilkuna1989) and later analyzed in more detail by Brattico (Reference Brattico2016, Reference Brattico2018), the latter which argues that the operation correlates with informational focus interpretation of the moved constituent. This is illustrated in (8).
-
(8) Nonlocal rightward movement
The operation is limited neither to the grammatical subject nor to the last position of the clause. It remains to be analyzed rigorously. One of the main goals of the present work is to fill in this gap.
Fifth, although the standard model explains how it is possible to topicalize several types of constituents, it does not explain why infinitival topics, such as those illustrated in (9), feel marginal.
-
(9)
Fronting of infinitival phrases (if this is indeed how these clauses are derived) creates further distortions in the ordering of the grammatical heads in the sentence. I find these constructions marginal but not ungrammatical.
Another observation, completely unaccounted for, is the fact that genitive arguments are much more resistant to movement than nominative or accusative arguments.Footnote 3 The pattern is illustrated by the two examples in (10). I judge (10a) to be ungrammatical; (10b) is marginal or ungrammatical. Compare (10) with (8).
-
(10)
Something prevents genitive arguments from moving into positions that are available for nominative, accusative or partitive arguments. Examination of the whole corpus of all word order permutations of certain basic Finnish construction types shows that the pattern is general. Genitive arguments resist noncanonical positioning, especially to the right, independent of the construction. This will become evident later in this article.
Completely unexplained and/or unexplored is also the fact that infinitival clauses accept multiple topics and/or subjects, at least to some degree:
-
(11)
It remains unclear (indeed, unaddressed) if such infinitival phrases contain left peripheral cartographies much like the finite clause. Huhmarniemi (Reference Huhmarniemi2012) shows that many infinitival phrases contain at least one A´ edge position. The semantic interpretation associated with these word order variations is unclear. They feel felicitous if interpreted as expressing contrastive focus or perhaps also topic, but the intuition is quite weak.
It is possible to combine the properties enumerated above, for example by topicalizing an infinitival clause with two topics (12a), perhaps even in a clause in which a head moves over another head (12b).
-
(12)
I judge both sentences to be grammatical (possible and understandable in some context) but quite marginal.Footnote 4
A final problem that I would like to address concerns the graded acceptability of many of the examples reported in the preceding text. The phenomenon is interesting in several ways. First, without a principled way of dealing with marginality it is hard to use marginal sentences as evidence. They come out as ‘unclear’ evidence, hence their status in any argument can be called into question. The second concern, often neglected, is the fact that marginality must be part of linguistic competence: as much as native speakers know that a sentence is grammatical or ungrammatical, they also know if it is marginal (assuming judgments does not fluctuate randomly, an assumption I will return to later). Finally, this author’s experiment with all mathematically possible word orders in Finnish suggests that something systematic is at play. Marginality is not at all uncommon: in a more systematic judgment task with random word order permutations, reported later in this article, I found that ~24% of the sentences were marginal in various ways (~75% ungrammatical). In addition, after examining thousands of scrambled sentences it is hard not to notice that there are patterns. For example, it seems to hold that nominative and accusative arguments produce marginality when they occur in noncanonical positions, if any deviance at all, whereas noncanonical positioning of genitive arguments produce ungrammaticality or extreme marginality. In addition, marginality seems to increase as a function of the number of specifiers and/or topics occurring in association with any given grammatical head. Whatever the source of marginality, it does not seem to constitute mere linguistic noise. Ideally we should try to find some principled way of approaching this phenomenon, too.
I will propose an analysis of Finnish word order in this article that addresses most of these concerns. The basic idea is that scrambled word orders are unnatural for the human parser and confuse language comprehension (see Braze Reference Braze2002, Hofmeister et al. Reference Hofmeister, Jaeger, Arnon, Sag and Snider2013). To examine this idea rigorously I adopt a parser-friendly top-down approach (Phillips Reference Phillips1996, Reference Phillips2003; Chesi Reference Chesi2012, Brattico Reference Brattico2019a; Brattico & Chesi Reference Brattico and Chesi2020) that has been further influenced by the dynamic syntax framework (Kempson et al. Reference Kempson, Meyer-Viol and Gabbay2001, Cann et al. Reference Cann, Kempson and Marten2005), and then apply it to the analysis of Finnish word order.
3. The framework
There are several reasons to distinguish grammatical competence, knowledge of language, from its use (Chomsky Reference Chomsky1965). One is that while any theory of language use must incorporate a theory of competence, the vice versa is not necessarily true. It is possible to construct a theory of grammatical well-formedness without taking performance factors, such as processing efficiency, accuracy, irrationality, or error recovery, into account. It does not follow from this, however, that performance is irrelevant to competence. As much as the human language faculty must operate within the boundaries set by the human conceptual-intentional system(s), it must also function within the boundaries set by sensory systems involved in comprehension. It could be that such limits are insignificant, but whether they are or are not must be determined by empirical inquiry.Footnote 5 One such inquiry is argued for in this article. I will argue that the explanation of free word order benefits from a shift from language production into comprehension.
Let us examine how the core computations of the language faculty might operate within the context of language comprehension. Let us assume that language incorporates an elementary recursive ability, call it Merge. Merge takes two elements α, β and yields [α β], a combination of the two (Chomsky Reference Chomsky1995:Chapter 4, Reference Chomsky, Freidin, Otero and Zubizarreta2008; Chomsky, Gallego & Ott Reference Chomsky, Gallego and Ott2019). This does not predict how α and β are selected, which is a matter of free will (Chomsky Reference Chomsky1959). What matters for the theory of competence is whether the combination of α and β is well-formed, grammatical, or an admissible operation. On the other hand, when the matter is looked from the perspective of language comprehension, Merge must construct phrase structures on the basis of a linear string of words that arrives to the language faculty through the one-dimensional PF-interface. This operation in the comprehension model substitutes ‘selection by free will’ in the production model. This shift is nontrivial.
Let us assume, following Phillips (Reference Phillips1996), that Merge can operate on the basis of a linear string of words. While Phillips assumes that the parsing operation that maps the input string into a phrase structure is also a theory of grammar/competence, hence that parsing = grammar, let us take a weaker position according to which the theory of Merge must be consistent with this scenario. The justification for the weaker thesis is that native speakers can parse sentences they receive through their sensory systems. It follows from this that because the input is linearized from left to right, Merge must be able to operate countercyclically by merging constituents in a top–down/left–right order. Suppose the input contains two words α + β. Suppose, in addition, that α + β arrive to the language faculty through the PF-interface, linearly ordered, after which they are matched with lexical entries in the lexicon (or surface vocabulary) and are then merged together to yield [α β]. I will assume, mirroring the standard linearization algorithm, that if β follows α at the PF interface, it will be merged to the right edge of the phrase structure (hence the operation could be called, following Phillips, ‘Merge Right’). ‘Right edge’ refers to the top node together with its right daughter, right granddaughter, and so on. To avoid any misunderstanding, I will refer to the Merge operation of this type as Merge–1. The superscript –1 refers to the fact that the operation performs an ‘inverse’ of the bottom-up Merge.
Suppose, to consider a more realistic example, that the input is a Finnish sentence Pekka käski heidän auttaa Merjaa ‘Pekka asked them to.help Merja’. This would generate (13), given in pseudo-English for simplicity, in which each line represents one step in the derivation.
-
(13)
The derivation proceeds from left to right and from top to bottom, following the incremental parsing process. On the other hand, derivation (13) assumes that each word is merged to the right of the bottom constituent. This assumption is unrealistic. If the subject were itself a complex constituent, the derivation could have a step in it in which the incoming word is merged to the top right node, not to the bottom right node. For example, if a previous derivation had generated [A man who asked them to help Merja], the next word could be merged to the top right position (as in ‘DP + laughed’). As a matter of fact, any merge site at the right edge between the top and bottom nodes must be available in principle. This means that instead of selecting a predetermined syntactic interpretation the model must access several possibilities and explore them in some order. To overcome this problem, I assume here, following Brattico (Reference Brattico2019a), that all accessible merge sites are ranked and that the ranking creates a search space that the parser-grammar explores recursively. If it finds a solution that does not work (i.e. encounters a garden path), it backtracks to an earlier point by using the search space generated from these rankings. If all options have been explored without reaching grammatical output, the sentence is categorized as ungrammatical. This operation provides the core of what I will call the ‘Phillips cycle’ in this article, in which words consumed from the input are merged (recursively, backtracking if needed) to the phrase structure into positions determined by the ranking. The cycle is illustrated in Figure 1.

Figure 1. Phillips cycle: a new word is consumed, which leads to the retrieval of the corresponding lexical item. Possible merge sites are ranked, after which the highest ranked site is selected, and the cycle begins anew. If there are no more words to be consumed, the output will be evaluated and the algorithm backtracks if the evaluation comes out as negative. If all legitimate branches get explored without a solution, the input is judged as ungrammatical.
One possibility is to rank the sites by means of some abstract geometrical property. For example, we could assume that the sites are always ranked and explored in a top–down order. This would be computationally inefficient and psycholinguistically unrealistic. We often know, just by looking at the elements that are about to be merged together, whether the operation is admissible or plausible (or impossible or implausible). For example, the fact that the direct object ‘Merja’ should be merged to the complement/sister position of the infinitival verb ‘to help’ in (13) is suggested by the fact that the transitive verb selects for a DP complement. Alternative solutions can be ruled out: finite or infinitival clauses cannot occur in the specifier position of DPs, so these solutions should not be prioritized. Thus, in Brattico (Reference Brattico2019a) and Brattico & Chesi (Reference Brattico and Chesi2020) it was assumed, following earlier work by Chesi (Reference Chesi2004, Reference Chesi2012), that top–down selectional features are implemented by means of lexical selection features. A transitive verb has a lexical selection feature comp:d which says that it selects for or ‘expects’ a DP-complement. Specifier selection was also assumed in the present study: D, for example, does not accept finite tensed clauses or infinitival clauses as its specifier, hence it is endowed with features −spec :t /fin and −spec:t. Lexical selection features were used to rank the merge sites: when they match, the solution is ranked higher, and if there is a mismatch, lower. Lexical features are also used to evaluate the output: if the output of the derivation does not satisfy lexical selection features of one or several lexical items, it will be rejected, and the parser will backtrack. Because the initial ranking is based on the satisfaction of selectional features, the output will typically satisfy them as well.Footnote 6 The internal operation of the Phillips cycle, as delineated here, will be subjected to a simulation test in Section 5.
Another approach to natural language that has inspired the present approach is the Dynamic Syntax (DS) framework (Kempson et al. Reference Kempson, Meyer-Viol and Gabbay2001, Cann et al. Reference Cann, Kempson and Marten2005). The DS framework starts from a parsing-oriented perspective similar to Phillips (Reference Phillips1996): both competence and performance are based on the ability to process linguistic input from the PF-interface. Accordingly, ‘knowing a language is knowing how to parse it’ (Cann et al. Reference Cann, Kempson and Marten2005:1). DS, like the current approach, models language comprehension as an incremental, dynamic operation, in which the phrase structure representation is constructed in a step-by-step manner on the basis of incoming words.
4. A top–down analysis of free word order
4.1 The problem of free word order
Consider a sentence in Finnish in which the grammatical subject is the last (rightmost) element of the clause. Let us assume (not unrealistically) that the bracketed phrase in (14) is what the parser-grammar has constructed so far, and that the next word to be consumed is Pekka ‘Pekka.nom’.
-
(14)
The correct canonical d-structure position for the subject, according to the widely accepted VP-internal subject hypothesis, is Spec,vP, indicated by ‘__1’ in the example above. The system elucidated in the previous chapter is not able to process these sentences correctly, as it will generate Pekka into a wrong position.
To solve this problem I suggest that the human parser reconstructs such orphan arguments by utilizing two cycles: a first pass parse, which reads and analyzes a chunk of words to create a tentative syntactic analysis by utilizing the Phillips cycle elucidated in the previous section, followed by adjunct reconstruction which reconstructs or ‘normalizes’ arguments to their canonical positions by using morphosyntax. Thus, the orphan argument is first attached to the phrase structure in a ‘tentative way’ during the first-pass parse and is then reconstructed, by using a grammatical operation, into the canonical position. To implement this idea formally, let us assume that rich case morphology licenses an operation in grammar in which thematic DP arguments are attached to the phrase structure as adjuncts (making them syntactically adverbials, see next section) and are then, by using case morphology as cues, reconstructed into canonical positions by means of adjunct reconstruction that forms adjunct chains.Footnote 7 The idea that thematic arguments are (or can be) attached to the structure as adjuncts was originally proposed by Jelinek (Reference Jelinek1984), Chomsky (Reference Chomsky1995:Section 4.7.3), Baker (Reference Baker1996) and Cann et al. (Reference Cann, Kempson and Marten2005) and was applied to Finnish by Brattico (Reference Brattico2016, Reference Brattico2018). Case features are utilized by the adjunct reconstruction by requiring that they match with the closest functional head of the type determined by the case feature itself. Matching consists of an inverse, top–down version of Agree (for the operation of Agree in the production theory, see Chomsky Reference Chomsky, Martin, Michaels and Uriagereka2000, Reference Chomsky and Kenstowicz2001, Reference Chomsky, Freidin, Otero and Zubizarreta2008). I call it Agree–1. The following condition on Agree–1 (15) is used in this study.
-
(15) Condition on Agree –1
If the parser-grammar detects a case feature that does not satisfy (15), it will promote the argument into an adjunct (i.e. assume that it has been so promoted by the speaker during production) and attempt to reconstruct it into a position that does satisfy (15). Reconstruction is implemented by locating the left edge of the minimal tensed finite clause and by searching downwards for a position that satisfies (15). The first position that satisfies the condition is selected.Footnote 8 The adjunct promotion-and-float cycle is then added to the Phillips architecture described in the previous section. The details of the adjunction operation will be elucidated in the next section.
4.2 Adjunct and head reconstruction
If richly case-marked thematic arguments are merged to the phrase structure as adjuncts, then what are adjuncts and adjunction? Manninen (Reference Manninen2003), building on the influential analysis of adjuncts by Cinque (Reference Cinque1999), assumes that Finnish manner adverbs are attached to the phrase structure as specifiers of expanded vP-projections. Chesi (Reference Chesi, Webelhuth, Sailer and Walker2013) develops the same idea within the context of the parser-friendly top–down grammar. These theories maintain that adverbials, like arguments, have fixed canonical positions. If, however, we use the theory of adjuncts to explain free word order, we cannot begin from a theory of adjuncts that posits canonical positions.
One analysis of adjuncts that is consistent with the present approach is that of Ernst (Reference Ernst2001), who proposes that adjuncts do not have canonical positions. Adjuncts are defined as nonheads that are not sisters to a head (i.e. they are noncomplements), such that they are furthermore not specifically designated as specifiers. I follow this proposal but assume that phrasal (or maximal) noncomplements are adjuncts if they are designated as such; otherwise they are specifiers (both formalizations are of course possible). I follow Chomsky (Reference Chomsky1995, Reference Chomsky and Belletti2004) and assume that the ‘adjunct Merge’ is distinguished minimally from argument Merge. Specifically, I assume that adjuncts constitute geometrical constituents of the phrase structure, being merged to the phrase structure as maximal categories, but are excluded from selection and label computations. These assumptions are illustrated in (16), in which WP constitutes the right branch of the node immediately below the highest XP but is not selected and does not project. We can imagine of the adjunct phrase as being ‘pulled out’ of the main structure and put into a secondary syntactic working space.
-
(16) [XP YP [XP [X0 ZP] ⟨WP⟩]] (⟨WP⟩ = adjunct)
Canonical adjunct positions are licensed by a formal linking mechanism that ensures that the adjunct can be linked with a suitable functional head (V for VP-adverbials, T for TP-adverbials, and so on).Footnote 9 In the case of argument adjuncts, the formal linking mechanism is based on dependencies between case features and features of local functional heads (e.g. ±fin), as explained earlier.Footnote 10
It was noted in Section 2 that the distribution of genitive arguments is not as free as that of nominative, accusative or partitive arguments. The simplest possible hypothesis, adopted as a tentative working hypothesis in this work, is to exclude genitive arguments from the adjunct promotion-and-float mechanism. Whether this simple stipulation suffices to capture the notion of possible word order in Finnish will be tested by means of concrete simulation.
The adjunct reconstruction mechanism described in Section 4 does not work correctly in the case of wh-interrogatives. They are regulated by A′- movement. I will follow Brattico & Chesi (Reference Brattico and Chesi2020) who propose a theory of A′-reconstruction in connection with a Phillips-style top–down theory. In Finnish, if the phrase in the operator position is morphologically unmarked, it will be interpreted as contrastively focused and is typically stressed prosodically (Vilkuna Reference Vilkuna1989). I represent prosodic stress by a prosodic feature foc in the input.Footnote 11 The reconstruction algorithm will return the focused phrase to the canonical position by A′-reconstruction (Vilkuna Reference Vilkuna and É. Kiss1995, Huhmarniemi Reference Huhmarniemi2012, Brattico & Chesi Reference Brattico and Chesi2020). If the C field is filled in by head (T-to-C) movement, then the prosodic feature is interpreted as representing the C morpheme itself (17). The parser-grammar will then reconstruct the head to the structure.
-
(17)
An additional complication is presented by the fact that many words arrive to the PF-interface as multimorphemic units, not as single morphemes. The existing algorithm that was available before this study began extracted complex heads in a process that resembled Matushansky’s m-merger approach (Matushansky Reference Matushansky2006), but which does not suffice to capture nonlocal head movement. I assume the mechanism proposed in Brattico (Reference Brattico2020). Suppose that the input string is Pekka antoi kirjan Merjalle ‘Pekka gave the book to Merja’. The verb antoi ‘give’ is decomposed in the morphological parser into three separate items, T/fin, v and V, which are fed to Merge one by one. They are first combined into a complex head (TvV)0, which is reconstructed by applying head reconstruction, an inverse of head movement. Head reconstruction takes the first/highest morpheme inside the complex head (i.e. vV in the case of (TvV)0) and locates the closest position in downward direction on the projectional spine of the sentence in which it can merge that head without violating lexical selection. Thus, the small verb (vV)0 is reconstructed into the position in which it is selected by T; V is reconstructed from within v into a position in which it is selected by v. The whole sequence creates the required T–v–V chain from the complex head.
4.3 Summary
A proposal to handle Finnish word order phenomenon was developed within the context of Phillips’ parsing-friendly minimalist theory (Phillips Reference Phillips1996), dynamic syntax (Cann et al. Reference Cann, Kempson and Marten2005) and our prior work developing the latter two approaches (Brattico Reference Brattico2019a, Brattico & Chesi Reference Brattico and Chesi2020). An input string is fed to the model as a linear string of phonological words, after which each word undergoes lexical processing. The result of successful lexical processing is the retrieval of a lexical item that is merged to the structure. The string is read from left to right, which means that lexical constituents are merged to the structure in a top–down fashion (‘Merge’ in Figure 2 below). Because the correct (or intended) position of any lexical constituent is unknown, all solutions are ranked and explored in the ranked order. They are explored only if the highest-ranking solution does not produce a legitimate parse (i.e. the model enters a garden-path). This process creates a tentative first pass structure for the input string, which is normalized by applying a sequence of operations: (i) complex heads are spread out (i.e. (CTvV)0 creates [C …[T …[v …V…]]]), (ii) adjuncts are reconstructed into canonical positions and (iii) A′/A-movement is reverse-engineered. The resulting structure is passed on to the LF-interface, which checks that it constitutes a legitimate, semantically interpretable LF-object. Failure at this stage results in recursive backtracking. It is assumed that all case-marked arguments, with the exception of the genitive, can be treated as adjuncts and are floated back into their canonical positions. Case forms are used to locate the canonical position by utilizing Agree–1, an inverse of the standard Agree. These assumptions, and the components of the theory, are illustrated in Figure 2.

Figure 2. Core components of the proposed language comprehension model illustrated in a nontechnical way. See main text for explanation.
It remains to be shown that the analysis captures the properties of Finnish word order. The usual method in the natural sciences for justifying a theory is to show by deductive calculation that it derives the observations. To do this, the model was formalized as a computer program that processes Finnish finite clauses. This procedure and the results are reported in Section 5.
5. Simulation experiment
5.1 Introduction
The correctness of the proposed hypotheses was assessed by means of computer simulation. Three questions were addressed: (i) whether the comprehension model delineated above is able to parse canonical Finnish finite sentences; (ii) if it is able, whether the same model can parse also noncanonical word orders; and, if it can, (iii) whether marginality, as judged by native speakers, correlates with computational complexity of the parsing process, supporting the notion that marginality emerges from the fact that such sentences are ‘unnatural’ for the human parser.
5.2 The algorithm
The source code embodying the model delineated in the two preceding sections together with technical documentation is available in the public domain and exists as a frozen branch of the master code.Footnote 12 The algorithm embodies the assumptions detailed in Sections 3 and 4 together with a few auxiliary conjectures (e.g. concerning morphological parsing, creation of head movement chains). The implementation was written in Python. The algorithm was provided with a lexicon that contains the required words and their lexical features. The lexicon was disambiguated and normalized. Prosodic emphasis was marked as a feature in the input (#foc). The input had no other grammatical annotation and consisted of a linear order of phonological words.
5.3 The output of the algorithm and raw data
The algorithm, which read and processed each sentence on a word-by-word basis, provided a parsing solution (structural analysis) for each input together with an overall well-formedness score and a log file documenting the computational steps consumed in the derivation. If no parsing solution was found, the input sentence was judged as ungrammatical. This notion of ungrammaticality was proposed by Cann et al. (Reference Cann, Kempson and Marten2005), who assume that for ‘a string to be ungrammatical, there must be no possible sequence of actions whereby a logical form can be constructed’ (p. 84).Footnote 13 The log and the result files constitute the raw machine-generated data of the present study. Both are available in public domain. All files used in the simulation are organized so that each input sentence is provided with a unique number identifier, and the corresponding items can be then found from the result and log files by searching for that number. Author’s judgments, which constitute the gold standard, are available in separate files. The author verified the grammatical analyses provided by the algorithm. Creation of the gold standard is detailed in Section 5.5. The organization of the input/output structure used in this study is illustrated by Figure 3.

Figure 3. Design of the study. The input corpus constitutes a separate text file that is fed to the algorithm sentence by sentence. Each sentence is processed one word at a time. If no parse is found, the input is classified as ungrammatical. Reasons for ungrammaticality are left to the derivational log file. If a solution is found, it is provided with a grammatical analysis. The log file then contains a step-by-step derivation ending up with the accepted solution. Author native speaker judgment is used as a gold standard against which the performance of the model is compared.
5.4 Materials and procedure
A small group of grammatical seed sentences (24 sentences in total) was first created which consisted of canonical Finnish finite clauses. These items were created by crossing four syntactic variables: valency (intransitive, transitive, ditransitive), polarity (negative, affirmative), embedding (no embedding, infinitival complementation) and the presence of an additional adverbial (yes, no) (a total of 3 × 2 × 2 × 2 = 24 construction types). The seed sentences are listed in Table 1.
Table 1. Seed sentences covering sentences in different valency (intransitive, transitive, ditransitive), polarity (affirmative, negative), with or without infinitival embedding and with or without an adverbial.
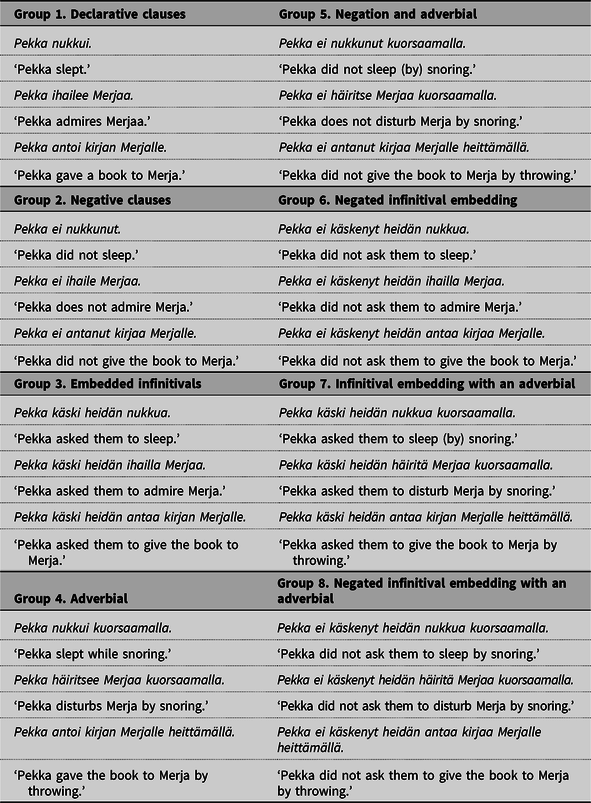
The main corpus was created by generating all mathematically possible and unique word order combinations from the seed sentences. For each sentence in the main corpus, an alternative was created which had the prosodic emphasis on the first word. This must be done because Finnish verb-initial clauses are grammatical if and only if the verb is stressed prosodically and has thus moved to C (with the exception of a few exceptional verb-initial constructions, not included in this study). The main corpus consisted of 119,800 word orders.
5.5 Creation of the gold standard
The output was compared with a gold standard that was based on the author’s native speaker judgment. Because of the vast volume of the judgments (approximately 5000 sentences were judged for grammaticality and marginality in this study), and because they required understanding of abstract linguistic features (e.g. foc), filtering of irrelevant parses and pragmatic anomalies and awareness of implicit contexts that are crucial in assessing the grammaticality of word orders, raw acceptability scores elicited from linguistically untrained participants were considered unreliable. Author’s judgments were performed twice, however, once before the algorithm was run (in June 2019) and then six months later (in January 2020).
I used the question ‘Could this sentence possibly be used in some context?’ as a guide in assessing grammaticality. Grammaticality was a binary choice: grammatical or ungrammatical. I used a four-level system for assessing marginality: ‘clear’, which meant that the clause was canonical; ‘mildly marginal’, which referred to sentences that felt noncanonical but otherwise normal and were marked with single question mark; ‘clearly marginal’, which referred to clauses that felt clumsy, strange or exceptional and were marked with double question mark; and ‘extremely marginal’, which was associated with sentences that were extremely marginal, borderline ungrammatical, or if it was unclear if the sentence was ungrammatical or grammatical. The latter were marked with ?*.Footnote 14 The line between extremely marginal and ungrammatical sentences proved to be difficult to draw, so not much significance will be put on this distinction in the analyses. These judgments were converted into a Likert-scale so that 1 = grammatical and clean, 5 = ungrammatical, and the three marginality grades between so that 2 = mildly marginal, 3 = clearly marginal and 4 = extremely marginal, borderline ungrammatical. The algorithm produced the same five-point classification, with 5 corresponding to the situation in which no parse was found. My ratings are provided in separate files associated with this article. Furthermore, my judgments should be interpreted as establishing a rank (not interval or categorical) scale. The point is to compare sentences in the corpus with each other rather than measuring them in some absolute sense.
I compared the machine-generated, predicted output from the model with the gold standard in two ways. All analyses were conducted by taking a representative random sample from the corpus (1000 sentences in most analyses). To check that the parsing solutions were correct, the machine-generated solution was verified by the author. In a second analysis, the author assessed the corpus first independently and the results were compared with the machine-generated output. The sample that was used to verify parsing solutions was different from the one the author used to perform the blind grammaticality and marginality judgments.
Use of mechanical deductive calculations in empirical justification in theoretical linguistics creates challenges due to scalability. Because the number of possible input sentences (here possible word orders) increases exponentially as a function of the number and complexity of the seed sentences, the size of the test corpus increases extremely fast. Addition of one more syntactic parameter into the present dataset would have increased the number of sentences into millions. While a computer can perform almost any number of calculations, the output must still be matched against a gold standard that is produced by native speaker(s). Yet, vast mechanically generated raw test corpora are not suitable targets for linguistically untrained informant judgment. Experimental materials must be carefully curated so that they measure, reliably and consistently, what they are intended to measure. In an ideal case collaborative work between computational modelling, corpus linguistics and experimental work should result in standardized test corpora that are accepted as normative gold standards in the field.
5.6 Results
5.6.1 Derivation of the canonical seed sentences: Study 1
A baseline was established by feeding the algorithm with the seed sentences. The model provided correct solutions for all canonical seed sentences, did so without errors, and as efficiently as possible without any garden-pathing (Table 2a and 2b). I use [,] for regular constituent and (,) for adjuncts.
Table 2a. Solutions provided by the algorithm for seed sentences in Groups 1–4 in Table 1.

Table 2b. Solutions provided by the algorithm for seed sentences in Groups 5–8 in Table 1.

Operator movement was tested by transforming each seed sentence into an interrogative by substituting one of its arguments with an interrogative pronoun and fronting it. The algorithm processed interrogatives correctly. Study 1 confirms that the proposed model produces plausible solutions for all Finnish seed sentences. The parsing process did not involve backtracking or unrealistic search: every solution was found immediately.
5.6.2 Noncanonical word orders: Study 2
The correctness of the model in connection with noncanonical word orders was examined next. A random sample of 1000 sentences were drawn from the whole corpus, which was first judged blindly by the author. The algorithm then processed the same 1000 sentences, after which the output was compared to the blind judgments by the author. Aggregate results from that comparison are provided in Table 3.
Table 3. Prediction error in a sample of 1000 sentences taken from the whole corpus.

Approximately 75% of the judgments produced by the model matched with the author’s blind judgment and 100% of the parsing solutions, when they were produced, were correct or at least plausible to this author’s linguistically informed judgment (based on a sample of 1000 sentences). Notice that most errors in the group A occurred when the algorithm was unable to distinguish ‘ungrammatical’ from ‘extremely marginal’, which suggests that the distinction might be difficult to draw or is perhaps unstable or does not exist. If we ignore these, then the prediction error is 15.9%.Footnote 15 I will analyze the nature of these errors further below. The distribution of author judgments is provided in Figure 4. This might be taken as an estimate for the freedom in Finnish word order in a corpus of relatively simple constructions (defined by the seed sentences in Table 1).

Figure 4. Distribution of native speaker grammaticality and marginality judgments. Judgments were generated by including all logically possible word orders from the seed sentences in Table 1 and by evaluating a random sample of 1000 sentences. Evaluation was done by the author. Distinction between adjacent categories should not be regarded as dichotomous or well-defined.
The log files, written by the algorithm as it processed the test sentences, show that the model’s success is based on the components elucidated in Section 4. The algorithm uses the adjunct promotion and the floating technique to reconstruct orphan arguments into their canonical positions by using case morphology. Limited distribution of genitive arguments came out correctly when it was assumed that their canonical positions were reconstructed on the basis of order alone. Genitive arguments in Finnish were therefore treated like accusative and nominative arguments in English. Head movement reconstruction was sufficient to reconstruct grammatical heads and was successful in pruning out ungrammatical (i.e. reversed or totally scrambled) head orders. Notice that the head reconstruction was a limited process: it was not able to reconstruct heads into ‘upward direction’, for example. Verb-initial clauses with prosodic stress were reconstructed correctly, and the same was the case with focused phrases, which were correctly normalized by A′/A-bar reconstruction. Example derivations and analyses are discussed in detail in the supplementary document ‘Analysis and derivation of Finnish finite clauses by the algorithm’.
The majority of errors were contributed by sentences in the Group A. Most of them (~70%) consist of word salad sentences that the author judged as extremely marginal (?*) but the model was unable to process and thus classified as ungrammatical. Example (18) illustrates examples from this category. The judgments are mine; the model judged them ungrammatical. Notice the feature #foc that indicates prosodic stress on the first constituent.
-
(18)
The sentences are extremely odd, perhaps ungrammatical, to a native speaker. Furthermore, the distinction between extremely marginal and ungrammatical is unclear and possibly non-dichotomous. An anonymous NJL reviewer, who is a native speaker of Finnish, classified these examples as ungrammatical. If they are ungrammatical, then the model performance is better. On the other hand, to draw any conclusions one has to judge all 1000 sentences. I will return to the nature of these errors at the end of this section.
Category B contained sentences that the model classified wrongly as grammatical. There were a few instances of nonlocal head movement that the model was able to reconstruct but which are not trivial for native speakers. An example of this phenomenon is provided in (19) (#211).
-
(19)
Are sentences of this type grammatical or ungrammatical? There are no systematic published studies of Finnish nonlocal head movement and thus very little to rely to settle the issue. I leave the problem for future research.
Category C involves prediction errors in marginality estimations that involves adjacent marginality categories. These errors have little or no meaning due to the overlap between the adjacent categories, further quantified below. Category D warrants closer examination. The total number of such errors was negligible, but approximately two thirds of these sentences were ones in which the model underestimated their marginality and was therefore too good in parsing them. This suggests that human comprehension is subject to limitations that the model was not capturing. Table 4 provides a sample of sentences in which the author and the model agreed on marginality, providing an overview of how the model handled marginality and what type of grammaticality–marginality–ungrammaticality continuum it (correctly) predicts to exit.
Table 4. A sample of sentences judged similarly by the author and the model, illustrating the grammaticality–marginality–ungrammaticality continuum predicted by the model.

S = main clause subject, V = main clause verb, O = main clause object, IO main clause indirect object, Adv = adverbial, s = infinitival subject, v = infinitival verb, n = negation. Sentence numbers (#) refer to the numbering in the test corpus.
The relationship between parsing complexity, as predicted by the model, and native speaker marginality judgments was examined next. Because the relevant numbers are meaningful only for sentences which the model judged as grammatical, only such input sentences are included in the analysis. Computational complexity increased linearly as a function of author’s marginality judgments, as shown in Figure 5.

Figure 5. Mean value of the total number of computational operations Merge and Move as a function of native speaker judgment (0 = grammatical, 1 = mildly marginal, 2 = clearly marginal, 3 = extremely marginal, 4 = ungrammatical). The main reason for the increase of complexity is due to occurrence of garden paths (parsing decisions that do not lead into a solution) in connection with more marginal sentences.
Spearman correlation between parsing complexity (number of computational steps required to provide a solution) and native speaker marginality judgment in sentences that the model judged grammatical was 0.38 (p < .001). ‘Number of computational operations’ was the sum of both Merge and Move. Thus, although parsing predicts variation in marginality judgments, it is not the only predicting factor. The hypothesis that one factor affecting marginality could be the number of extra specifiers or topics per grammatical head was also tested. The algorithm computed a discourse score for each sentence that was a linear function of the extra (>1) specifiers occurring in connection with any head(s) in the output analysis, which was then correlated with the native speaker judgment. The score is recorded as discourse plausibility in the raw output. A correlation (Spearman correlation 0.57, p < .001) was found. Thus, the initial suspicion that marginality could be predicted on the basis of the number of specifiers/topics per head, hence discourse complexity, was verified.
The nature of the errors in the Category A were difficult to discern reliably due to the fact that many of these sentences were excessively complex. To better understand the errors involved with Category A, and hence to examine the problems of the analysis, the model was evaluated by taking a random sample of 1000 sentences from the first four groups (Groups 1–4, Table 1) that were generated by crossing valency (intransitive, transitive and ditransitive) with construction type (simple declarative clauses, negative clauses, clauses with an embedded infinitival, and clauses with an adverbial). This sample contained simpler and shorter sentences. The author judged the sentences blindly and the result were compared with the model output. A summary comparison between author’s and model’s judgements is provided in Table 5.
Table 5. Prediction error in a sample of 1000 sentences constructed from Groups 1–4 in Table 1.

Category A could now be broken into three clear subcategories. The first (A1) contained ‘V…neg’ orders that the author judged marginal but the grammar was unable to parse (20a); the second (A2) contained ‘v…V’ orders (v = infinitival verb, V = main verb) that the author judged marginal but the model was not able to parse (20b); and (A3) contains ‘v…s’ orders some of which the author judged extremely marginal and the model ungrammatical. An example of each category is provided below. Judgments are from author; the model cannot parse them.
-
(20) (V = finite verb, Neg = negation, v = infinitival verb, s = infinitival subject)
Examples (20a, b) illustrate null head movement, in which V or v moves over something and lands in a position whose properties cannot be inferred from anything (i.e. there are no criterial or prosodic features). The algorithm was not allowed to generate null heads or reconstruct head movement from a null head. I rated many sentences of this type as grammatical, however. The required upward head movement reconstruction looks nontrivial. I leave this problem for future research.
Another group of ungrammatical sentences that the model classified wrongly as grammatical (Category B) contained multitopic constructions or very heavy ‘head-final constructions’ such as (21).
-
(21)
I judged (21) to be ungrammatical, while the model predicted extreme marginality.
It remains a possibility that author’s judgments reflect linguistic noise rather than a stable grammar. This was examined by evaluating one dataset twice: first before the algorithm was run, as described above, and then for a second time six months later. The Pearson correlation between the two ratings was high, 0.73 (p < .000). Figure 6 shows the distribution of the rating changes. Because the changes are evenly distributed into both directions, my overall rating leniency remained the same. T-test revealed no difference in the mean ratings between the two measurements (M 2019 = 3.578 and M 2020 = 3.573, t(999) = 0.142, p = .887).

Figure 6. Distribution of the differences between the two ratings together with normal distribution. There were 10 examples in the ±4 category, 41 in the ±3, 103 in ±2 and rest were in the range (−1, 0, 1), with more than half in the category of ‘no change’.
Normally distributed variation suggests that it represents noise in judging speed, parsing speed, reading speed, focus, attention, shifting judging criteria emphasis and other performance aspects (see Sprouse et al. Reference Sprouse, Yankama, Indurkhya, Fong and Berwick2018). Overall, however, my ratings reflect a stable grammar. This concerns both grammaticality and marginality, and further supports the notion that marginality is part of grammatical competence.
5.6.3 Noncanonical word order in English: Study 3
The model was tested with English sentences to verify that it captured the contrast between a free word order language such as Finnish and a frozen word order language such as English. In English, (most) thematic arguments are not associated with case suffixes; hence adjunct reconstruction is not available. All thematic arguments must be reconstructed by using A′/A-reconstruction. The matter was examined by translating the clauses from Group 1 into English, creating a corpus containing all possible word order permutations from these seeds, and running them through the parser-grammar. The results are summarized in Table 6. Notice that what in Finnish amounts to the OVS order will come out in English as a regular SVO order, but with the reversed thematic roles (e.g. John likes Mary, Mary likes John).Footnote 16
Table 6. Grammaticality judgments for noncanonical word orders in English, as provided by the model.

For comparison, Tables 7a and 7b list the grammaticality/marginality estimations (Table 7a) and output solutions (Table 7b) provided by the model for all possible word order permutations for the basic Finnish intransitive, transitive and ditransitive clauses in the Group 1.
Table 7a. Word order variations in basic finite clauses in Finnish and the grammatical/marginality estimations provided by the model.

Sentence numbers (#) refer to the numbering in the test corpus.
Table 7b. Analytic solutions provided by the model for sentences in Table 7a.

Sentence numbers (#) refer to the numbering in the test corpus.
The model captures the distinction between English and Finnish: in English, word order can be said to be frozen, whereas in Finnish, free. If the present hypothesis is correct, then the difference depends on the availability of adjunct reconstruction for arguments, available in Finnish on the basis of rich case suffixes but not in English. I propose that this is what explains the parametric distinction between rigid word order languages and free word order languages. Notice that Finnish genitive arguments were treated as if they were English DPs: only linear order in the input was used in reconstructing them.
6. Discussion and conclusions
A computational model of language comprehension, following and developing Phillips (Reference Phillips1996, Reference Phillips2003), Cann et al. (Reference Cann, Kempson and Marten2005), Brattico (Reference Brattico2019a) and Brattico & Chesi (Reference Brattico and Chesi2020) was proposed, in which language comprehension is incremental and uses the inverse versions of standard computational operations (i.e. Merge–1, Move–1, Agree–1). Words are merged to the phrase structure in tandem with consuming them from the one-dimensional input at the PF-interface. This model was augmented with several novel grammatical operations that were required on independent grounds on the basis of Finnish data. These mechanisms were as follows.
First, it was assumed that rich morphosyntax, and not only order, is used to infer hierarchical relations between words as they occur in the input. This was implemented by merging words into tentative positions during the first pass parse and then reconstructing them into the vicinity of functional heads on the basis of their case suffixes (e.g. nom = +fin, gen = −fin, acc/par = v/asp). This uses the operation Agree–1, perhaps pointing towards a possible functional motivation for case assignment. Genitive arguments were excluded from the mechanism and treated like arguments in a rigid word order language. Second, a separate head movement reconstruction (Move–1) was postulated that extracted constituents from within complex heads, as provided by the morphological parser, and reconstructed them into the first position in which they could be selected by a higher head (e.g. v was reconstructed from within T into a position in which it would be selected by T). Third, the occurrence of more than one specifier per head was used to generate an extra licensing head between the two phrases (e.g. C(wh) in the presence of a wh-phrase).
The model was formalized and implemented as a computer program. A corpus of Finnish finite clauses was created by crossing three grammatical variables, valency (intransitive, transitive, ditransitive), polarity (affirmative, negative), infinitival embedding (no, yes), presence of independent adverbial (no, yes), which created 3 × 2 × 2 × 2 = 24 basic seed sentences. A baseline was established by verifying that the parser-grammar was able to parse these sentences correctly and efficiently, after which it was fed with all mathematically possible word order combinations of the seed sentences (119,800 clauses in total). The proposed mechanisms were sufficient to classify grammatical sentences correctly as such and to provide them a plausible grammatical analysis; it was also able to predict marginality. Therefore, it can be argued that the model provides a useful analysis of the Finnish word order. More specifically, this study supports the notion that rich morphosyntax can substitute for order in inferring hierarchical relations between words, and that the marginality of many noncanonical word order permutations results from the fact that they constitute input strings that are unnatural, hence computationally costly, for the human parser.
Acknowledgements
This work was supported by IUSS as an internal research project ‘ProGraM-PC: A Processing-friendly Grammatical Model for Parsing and Predicting Online Complexity’. I would like to thank the three anonymous NJL reviewers for their valuable feedback and suggestions.
Supplementary material
To view supplementary material for this article, please visit https://doi.org/10.1017/S0332586520000098