Introduction
Overgeneral autobiographical memory (OGM) refers to the failure to retrieve a specific, event-level memory from one's personal past when asked and retrieval of a more general memory instead. A specific memory refers to a unique event that is spatiotemporally located (e.g. a 15th birthday spent with friends, or a particular day at work completing a particular task). In contrast, a general memory refers to events that are extended over more than 24 h in time (e.g. a holiday over summer), categoric in nature (e.g. going for walks in the neighbourhood once a week) or just semantically associated (e.g. generally feel happy). First described by Williams and Broadbent (Reference Williams and Broadbent1986), the OGM phenomenon has been established as a feature of clinical depression (e.g. Hitchcock, Nixon, & Weber, Reference Hitchcock, Nixon and Weber2014; Liu, Li, Xiao, Yang, & Jiang, Reference Liu, Li, Xiao, Yang and Jiang2013; Williams et al. Reference Williams, Barnhofer, Crane, Herman, Raes and Watkins2007; Wilson & Gregory, Reference Wilson and Gregory2018). It has been associated with a range of processes signifying impaired psychological functioning, such as poorer problem-solving ability, planning and decision-making (Dalgleish & Werner-Seidler, Reference Dalgleish and Werner-Seidler2014), and lower levels of social support (Barry, Vinograd, et al., Reference Barry, Vinograd, Boddez, Raes, Zinbarg, Mineka and Craske2019b). Therefore, as well as being a cognitive marker of clinical depression, it is a factor in a range of functional issues.
The primary instrument used to assess OGM has been the Autobiographical Memory Test (AMT) (Williams & Broadbent, Reference Williams and Broadbent1986). The AMT requires individuals to provide specific memories to a series of cue words, typically of different emotional valences. The responses are coded in terms of their specificity/generality, and typical indices of OGM are the number or proportion of responses that are non-specific or categorical in nature (overgeneral/categorical) or the number or proportion of specific memories (memory specificity). Therefore, OGM can be operationalised as more overgeneral memories or fewer specific memories.
Sumner, Griffith, and Mineka (Reference Sumner, Griffith and Mineka2010) published a meta-analytic review examining studies that assessed OGM as a predictor of the course of depressive symptoms over time using the AMT. This review was motivated by inconsistencies across studies that assessed OGM as a vulnerability factor for depression. In their review, they used indices of overgeneral/categorical memories and specific memories to assess OGM. They reported on studies that assessed the zero-order correlations between OGM at baseline and depressive symptoms at follow-up, and studies that used regression analyses to examine these relationships while controlling for baseline depressive symptoms. They found that more overgeneral/categorical memories were correlated with more severe depressive symptoms at follow-up (r = 0.13, p < 0.01) and that, in regression analyses, more overgeneral/categorical memories (β = 0.11, p < 0.05) and less specific memories (β = −0.17, p < 0.05) both predicted more severe depressive symptoms at a later time over and above baseline depressive symptoms. The zero-order correlation between specific memories and later depressive symptoms did not reach statistical significance (r = −0.10, p = 0.11). Another notable finding was that OGM was a stronger predictor of the course of depressive symptoms amongst samples with clinically-significant levels of psychopathology (β = −0.37, p < 0.001), relative to non-clinical samples (β = −0.10, p < 0.11).
The previous review by Sumner et al. (Reference Sumner, Griffith and Mineka2010) had few studies with which to estimate effect sizes (n between 4 and 8). Small numbers of studies can limit the precision of effect-size estimates. Furthermore, there were a small number of studies for moderator analyses which, as noted by the authors, was likely to result in low power to detect effects. Since the publication of their review, research interest into OGM and depression has grown, and further studies examining OGM and depression have been published. An updated review would provide more accurate effect-size estimates and help determine the robustness of the original findings. Further, increasing the number of samples would boost power for moderator analyses and improve our ability to examine the contribution of other variables that have emerged as possible moderators in the association between OGM and depression, such as participant age (Wilson & Gregory, Reference Wilson and Gregory2018) and gender (Young, Bodurka, & Drevets, Reference Young, Bodurka and Drevets2017).
Therefore, the aim of this study was to update the previous meta-analytic study by Sumner et al. (Reference Sumner, Griffith and Mineka2010). In order to facilitate comparisons between the reviews, their methodology was replicated in full. A systematic review was conducted from the end point of their systematic search (2009), and any new studies that were found were added to the pool of studies used in the original review and analyses. In terms of moderating variables, we assessed the contribution of clinical status of the sample (selected on basis of clinically-significant psychopathology v. not selected on this basis), rating method for depressive symptoms (clinician-rated v. self-rated), the valence of the cue words on the AMT, the length of follow-up and age of the sample, to the differences between study effect sizes. We examined two further moderators: number of cue words on the AMT and the gender of the sample. It was reasoned that the number of cue words could potentially increase the sensitivity of the AMT to detect effects on depressive symptoms. With respect to gender, some research has shown differences between males and females for OGM (e.g. Heron et al. Reference Heron, Crane, Gunnell, Lewis, Evans and Williams2012; Popovski & Bates, Reference Popovski and Bates2005; Ros et al. Reference Ros, Ricarte, Serrano, Nieto, Aguilar and Latorre2014), which might moderate its effects on depressive symptoms (Young et al., Reference Young, Bodurka and Drevets2017). We present a condensed version of Sumner et al.'s methodology below and refer readers to the original review for a more comprehensive description.
Method
The methodology of Sumner et al. (Reference Sumner, Griffith and Mineka2010) was replicated and is described below. Data on previously included studies were extracted from the tables presented in the previous meta-analysis. Some information was not reported in this previous analysis (e.g. gender composition, information about separate valence-related effects) and this was extracted from the source articles themselves where possible or authors were contacted.
Search strategy
In addition to articles selected from the previous meta-analysis, a systematic, computerised search of the literature was conducted on literature published between 2009 (the year up to which studies were included in the previous analysis) and the date of the present search, 6 January 2021. The search used the following databases: PubMed, PsycINFO, Social Sciences Abstracts, and ProQuest Dissertations and Theses. The reference lists of all articles included in the analyses were also searched for possible studies to include. The key word terms included were: overgeneral autobiographical memory, autobiographical memory specificity, and autobiographical memory and depression.
Inclusion and exclusion criteria
The following criteria were used to identify eligible articles: (1) OGM was measured using the AMT; (2) results for specific memories and/or overgeneral memories and/or categorical memories were reported; (4) depressive symptoms were measured at a minimum of two timepoints; (3) correlations between OGM and depressive symptoms at follow-up and/or the standardised regression coefficient for OGM predicting follow-up depressive symptoms with the initial level of depressive symptoms included as a covariate were reported; and (5) the publication was in English.
Figure 1 outlines the flow of articles retrieved for this review. The search and data extraction processes were conducted independently by two researchers (authors two and three). Any discrepancies that arose were discussed and resolved with the first author. In total, 1828 studies were identified from searching the electronic databases. After removing duplicates (n = 328), titles and abstracts of the remaining 1500 records were evaluated against the inclusion criteria, which led to further exclusion of 1215 studies. After complete reading of the remaining 285 studies, 268 studies were excluded for the following reasons: 105 studies did not use the AMT, 86 studies measured depressive symptoms at one timepoint only, 58 studies failed to include standardised regression coefficients or correlations between OGM and depressive symptoms at follow-up, nine studies involved an intervention, three studies were not published in English and eight studies were unavailable to assess. Where studies or data were unavailable or not reported, attempts were made to contact the authors. Following this process, 17 studies were included in this meta-analysis. When combined with the 15 studies from the previous meta-analysis, the total number of studies was 32.
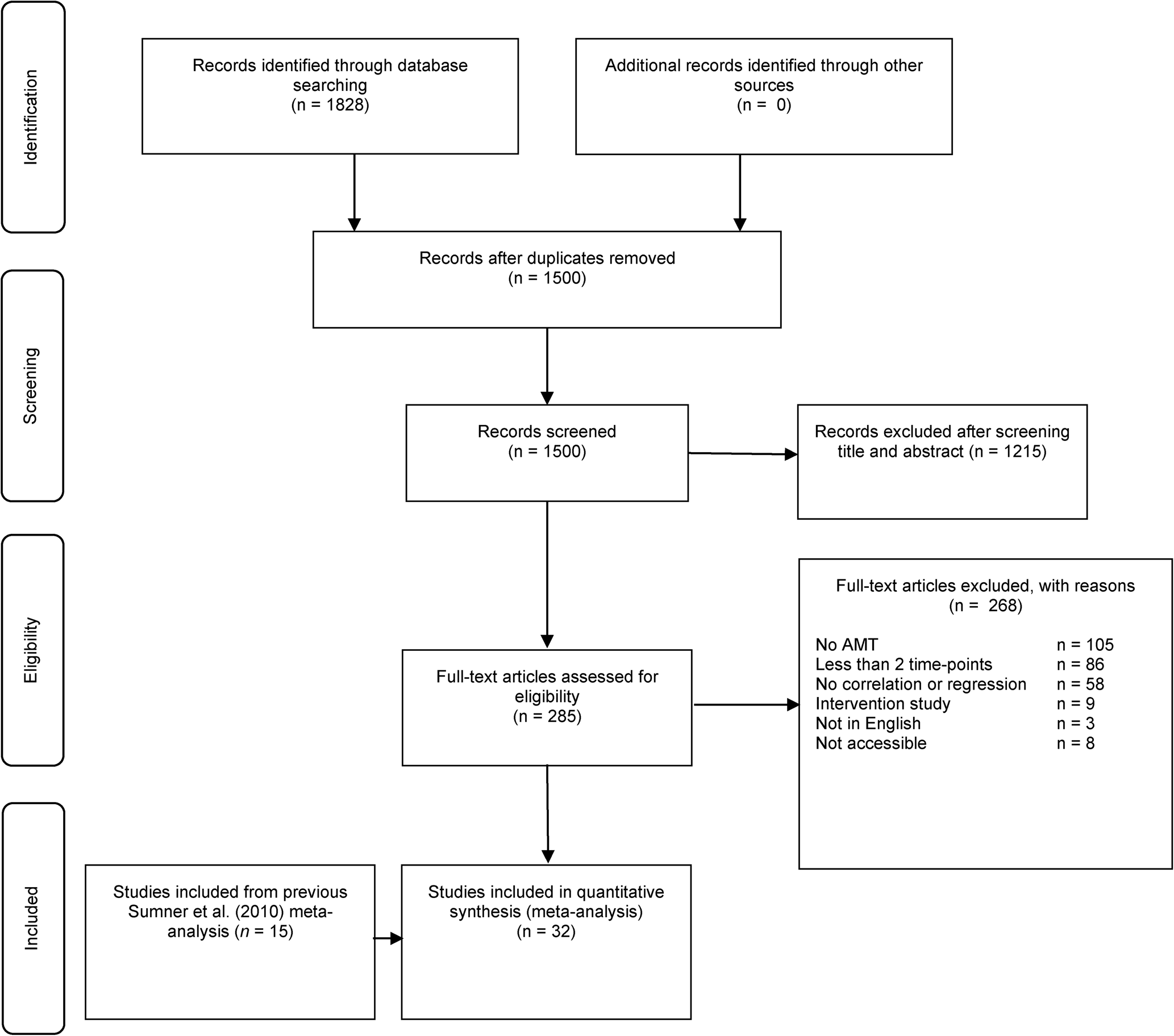
Fig. 1. PRISMA flowchart for systematic review.
Variables extracted from each study
The following data were extracted from each study: (1) publication date; (2) publication type (journal article, thesis or doctoral dissertation, unpublished data/report); (3) mean age of the sample; (4) percentage of females in the sample; (5) clinical status (selected on the basis of clinically-significant psychopathology or not); and (6) total sample size used in analysis.
AMT
Characteristics of the AMT procedure were also extracted, including (1) number of cue words; (2) valence of cue words (positive, negative, neutral, other); (3) cue word presentation method (oral, visual or both); (4) AMT response time limit (in seconds); and (5) OGM type (specific, overgeneral, categorical).
Depressive symptoms
The following aspects regarding the measurement of depressive symptoms were also extracted: (1) depressive symptom measure used (e.g. BDI); (2) depressive symptoms rating method (clinician-rated, self-rated); and (3) average time between baseline and follow-up assessments (in days). Each of these different measures was coded separately when studies reported results with multiple measures of depressive symptoms.
The second and third authors independently extracted the data and cross-checked their results. The first author then also checked these results against the included articles. Discrepancies were resolved through discussion and re-examination of the articles, and contacting authors was possible.
Computation and analysis of effect sizes
The current meta-analysis reported two effect-size indices: Fisher's Zr-transform and standardised regression (β) coefficient. Product-moment correlations were converted into Fisher's Zr-transform to be used for analysis in order to help mitigate problems due to standard error formulations (Lipsey & Wilson, Reference Lipsey and Wilson2001). Standard errors were also calculated, and each Fisher's Zr-transform effect size was weighted by the inverse of its variance, which consequently increased the impact of studies that had produced more reliable effect-size estimates. Standard errors and Fisher's Zr-transform estimates were calculated from sample size and product-moment correlations. Standard errors for standardised regression coefficients were based on reported standard errors, R 2 change values, p values and t tests.
Consistent with the previous meta-analysis, and research showing a one-factor model of autobiographical memory specificity within the AMT (Griffith et al., Reference Griffith, Sumner, Debeer, Raes, Hermans, Mineka and Craske2009), the current study used overall OGM, across cue valences, for the main analyses. Where studies reported separate valence-specific effect-size estimates instead of an overall OGM effect size, these effect-size estimates were averaged to calculate an overall OGM measure, with this averaged estimate and the sample size used to calculate the standard error. Some studies reported effect-size estimates of a single valence only (e.g. only negative, but not positive cues). In this case, single-valence effect-size estimates were averaged with an effect size of zero to compute a conservative overall effect-size estimate, and again this averaged estimate and sample size were used to calculate the standard error. An effect size of zero was selected in order to help correct for any bias resulting from only presenting statistically significant results of single-valenced cue word analyses.
Reporting multiple effect sizes per sample violates the independence assumptions of meta-analyses (Lipsey & Wilson, Reference Lipsey and Wilson2001). Therefore, in instances where studies reported more than one effect size for a particular OGM index (e.g. multiple depressive symptom measures), an average of the multiple effect-size estimates was calculated in order to present only one effect size per sample per OGM index (standard error calculated as above). In one study (Sumner, Mineka, & McAdams, Reference Sumner, Mineka and McAdams2013), two forms of the AMT were used which differed in their instructions. For consistency with the other studies, we opted to include the ‘traditional instructions’ version, as opposed to the version that provided less specific instructions for memory recall. In order to choose which time-point to use for studies that reported multiple time-points, we followed Sumner et al.'s method: Firstly, for all studies that included only one follow-up time-point, the average length of time to this follow-up was estimated (M = 8.76 months). Then, for studies with multiple time-points, the time-points closest to this average were selected to include in the meta-analysis.
All analyses used the mean effect-size estimate for each sample except for the moderator analysis that compared effect-size estimates for clinician-rated and self-rated measures of depressive symptoms. Both the clinician-rated and self-rated effect-size estimates for each depressive symptom measure were entered into this moderator analysis, thereby producing only one effect-size estimate from each sample in each class of the moderator variable. Even though effect sizes in the classes of the moderator variable are not independent, past research suggests that analyses of differences in groups of effect sizes that are potentially dependent likely generate conservative results (Hedges, Reference Hedges, Rao and Sinharay2007). All analyses used effect-size estimates based on the overall sample except for the moderator analysis examining participant clinical status. In this moderator analysis, two subsamples reported by Kleim and Ehlers (Reference Kleim and Ehlers2008) were considered to be separate studies, and both effect-size estimates were included.
Meta-analytic calculations were performed using Comprehensive Meta-Analysis, version 3 (Borenstein, Hedges, Higgins, & Rothstein, Reference Borenstein, Hedges, Higgins and Rothstein2013). Four separate analyses were conducted for: (1) correlations for overgeneral/categorical memories; (2) correlations for specific memories; (3) standardised regression coefficients for overgeneral/categorical memories; and (4) standardised regression coefficients specific memories. The current meta-analysis did not include any exactly replicated studies; therefore, we assumed a random-effects model for the analyses. This model accounts for variation in effect-size estimates by including a random component in addition to sampling error (Lipsey & Wilson, Reference Lipsey and Wilson2001). As per Sumner et al. (Reference Sumner, Griffith and Mineka2010), an analogue to ANOVA was used to analyse categorical moderator variables and meta-regression to analyse continuous moderator variables. These analyses used mixed-effects models in which variation in effect-size parameters was attributed to the moderator variable, subject-level sampling error and an additional random component. The same moderator variables were analysed as in Sumner et al. (Reference Sumner, Griffith and Mineka2010), with the addition of proportion of females in the samples and number of cue words. An α level of 0.05 was used for the results of the tests.
The Q statistic and I 2 index were used to assess between-study heterogeneity of effect sizes. A significant Q statistic indicates that factors outside of sampling error account for effect-size estimate variation, whereas a non-significant Q statistic suggests that differences in effect-size estimates between studies are likely to result from sampling error at the subject level (Lipsey & Wilson, Reference Lipsey and Wilson2001). The power of the Q statistic to detect statistically significant differences is based on the number of studies used in the meta-analysis. In contrast, the I 2 index does not rely on statistical significance, and is instead a percentage of total variation in a set of effect sizes that is due to heterogeneity between studies rather than chance (Higgins & Thompson, Reference Higgins and Thompson2002). Interpretations of I 2 indices of 25, 50 and 75% were taken as low, medium and high heterogeneity, respectively (Higgins, Thompson, Deeks, & Altman, Reference Higgins, Thompson, Deeks and Altman2003).
Duval and Tweedie's (Reference Duval and Tweedie2000) trim and fill method was used to identify and estimate the influence of publication bias. The trim and fill method graphs out a funnel plot in order to estimate the number of studies that are potentially missing from the meta-analysis as a result of publication bias. Effect-size estimates are plotted on the x-axis and the inverse of their standard error on the y-axis. Plots largely resemble a funnel, as less precise estimates are at the base of the funnel and estimates with the smallest standard errors are at the top. If there is no publication bias, the funnel plot will, hypothetically, be symmetrical. However, missing studies suppressed by publication bias may cause noticeable asymmetry in a funnel plot. The trim and fill method attempts to correct for publication bias by estimating these missing effect-size estimates and includes them in the analysis. The larger the difference between the original uncorrected effect-size estimate and the corrected trim and fill effect-size estimate, the greater the presumed influence of publication bias on the effect-size estimates based on only reported studies.
Results
Characteristics of the studies
With respect to the complete sample of studies included in this meta-analysis, the publication date ranged from 1993 to 2020. All articles were published in journals, with the exception of one unpublished dissertation and one unpublished report. Fourteen samples of participants were selected on the basis of clinically-significant psychopathology and 19 samples were not. The mean age of participants was 29.7 years (range 11–52.6), and the average proportion of females in the studies was 60.8%. Sample characteristics are provided in Table 1.
Table 1. Characteristics of studies included in analyses
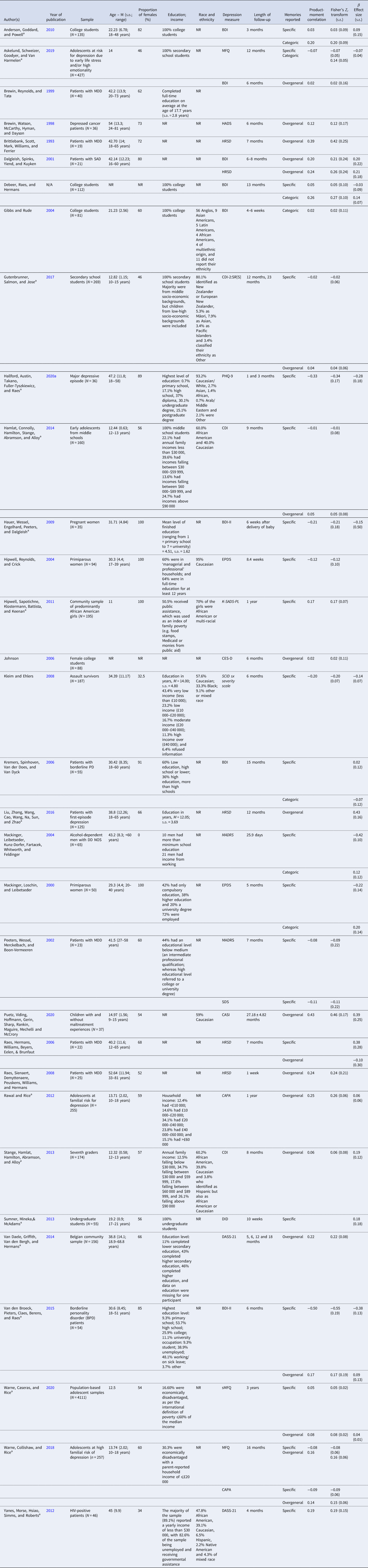
Note. Depression measure: BDI, Beck Depression Inventory; DASS-21, Depression Anxiety Stress Scales-21; PHQ-9, Patient Health Questionnaire; HADS, Hospital Anxiety and Depression Scale; HRSD, Hamilton Rating Scale for Depression; DID, Diagnostic Inventory for Depression; EPDS, Edinburgh Postnatal Depression Scale; CES-D, Center for Epidemiologic Studies Depression Scale; SCID sx severity scale, Structured Clinical Interview for DSM-IV symptom severity scale; MFQ, Mood and Feelings Questionnaire; sMFQ, 13-item version of the Mood and Feelings Questionnaire; CASI, Child and Adolescent Symptom Inventory; CAPA, Child and Adolescent Psychiatric Assessment; CDI, Child Depression Index; CDI-2:SR[S], 12-item Children's Depression Inventory-2 Self-Report Short Version; K-SADS-PL, Schedule for Affective Disorders for School-Age Children-Present and Lifetime Version; MADRS, Montgomery-Asberg Depression Rating Scale; SDS, Self-Rating Depression Scale.
Italicised depression measures are clinician-rated, whereas non-italicised measures are self-rated.
a Studies found in the current updated search between 2009 and 2020.
Correlation between OGM and depressive symptoms at follow-up
Table 2 presents the analyses of overall effects for correlations. The overall weighted mean effect size for correlations between overgeneral/categorical memories and depressive symptoms at follow-up was significant, 0.13 (p < 0.001, 95% CI 0.08–0.17), with low amounts of heterogeneity between studies. In contrast, the effect size between specific memories and depressive symptoms at follow-up was non-significant, −0.04 (p = 0.219, 95% CI −0.11 to 0.02). However, there was a significant Q statistic for specific memories, and moderate to high levels of heterogeneity. These findings are consistent with Sumner et al. (Reference Sumner, Griffith and Mineka2010) who also found that overgeneral/categorical memories, but not specific memories, correlated significantly with depressive symptoms at follow-up. One difference was that heterogeneity was higher in the present analysis for specific memories, indicating that there may be moderating factors that explain the variability in sampled effect sizes (see below).
Table 2. Results of overall meta-analysis of studies

OGM as a predictor of depressive symptoms over and above initial symptom levels
Table 2 presents the analyses of overall effects for regressions. The results indicated that overgeneral/categorical memories predicted higher depressive symptoms at follow-up, independent of depressive symptoms at baseline, with a statistically significant effect size of 0.09 (p = 0.003, 95% CI 0.03–0.15). Low amounts of heterogeneity were detected. A statistically significant result was also found for specific memories, which predicted lower levels of depressive symptoms at follow-up, with a small effect size of −0.16 (p = 0.001, 95% CI −0.25 to −0.06). Similar to the correlation analyses, a significant Q statistic and moderate levels of heterogeneity indicated there may be moderating factors of effect sizes for specific memories.
In order to examine the influence of the two unpublished studies on correlation and regression analyses, the analyses were re-run with the two studies removed. The results were almost identical (effect Δ ⩽ 0.01) with no substantive changes to p values.
Moderator analyses
Table 3 presents all results for moderator analyses.
Table 3. Results of analyses of categorical moderators
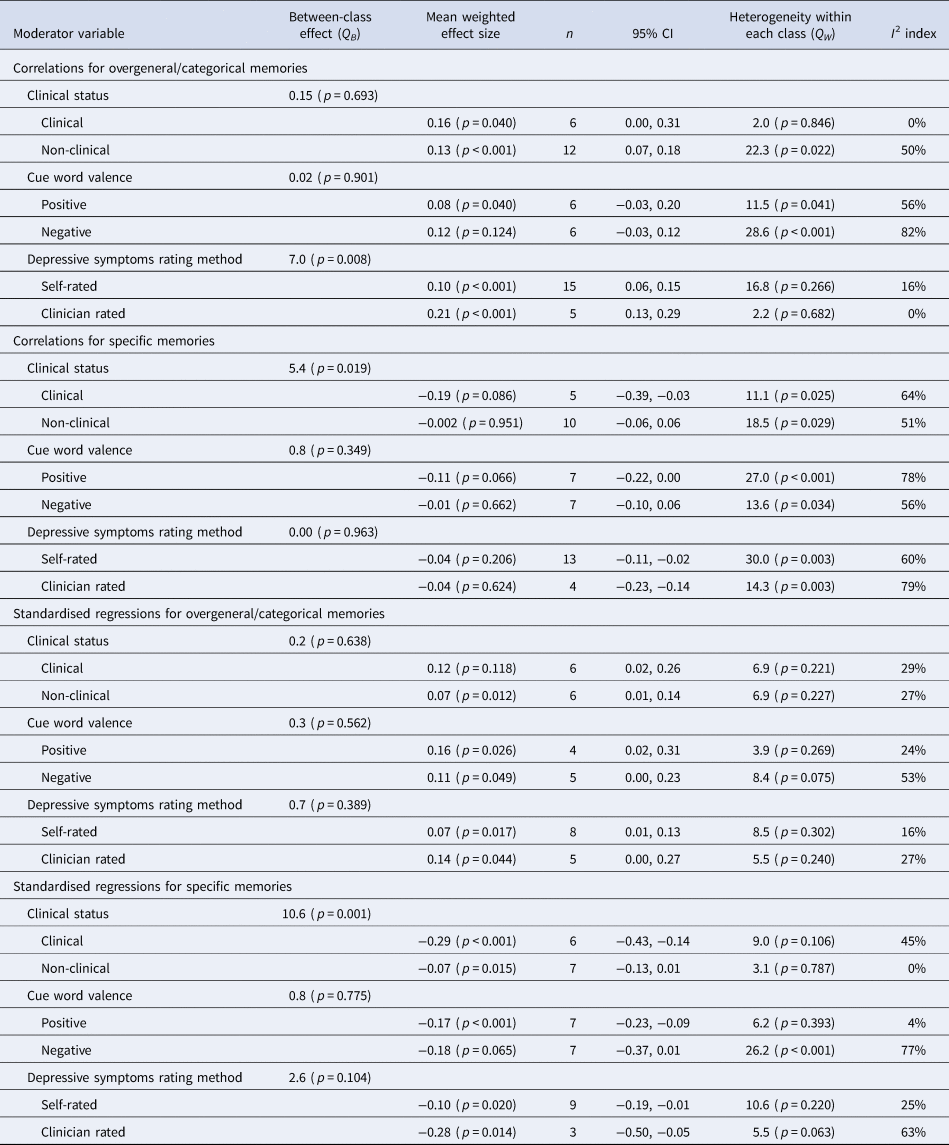
Clinical status
The clinical status of the samples was a significant moderator of the correlation (Q = 0.19, p = 0.016) and regression analyses (Q = 10.6, p = 0.001) between specific memories and depressive symptoms at follow-up, with this relationship stronger for clinical samples than non-clinical samples (−0.19 v. −0.002 and −0.29 v. −0.07, respectively). For overgeneral/categorical memories, clinical status was not a significant moderator in correlation or regression analysis (p's > 0.05). These findings are consistent with Sumner et al. (Reference Sumner, Griffith and Mineka2010) who also reported clinical status as a moderator for the effect of OGM assessed as specific memories, but not as overgeneral/categorical memories.
Rating method for depressive symptoms
The rating method (self-rated v. clinician-rated) was only a significant moderator for the correlation between overgeneral/categorical memories and depressive symptoms at follow-up (Q = 7.2, p = 0.007), whereby this correlation was stronger for clinician-rated methods relative to self-rated depressive symptoms (0.21 v. 0.09). For the correlation between specific memories and depressive symptoms, there was no moderating effect, with neither rating method showing a significant effect (p > 0.05). For regression analyses of overgeneral/categorical and specific memories, there were also no moderating effects (p > 0.05), with both indices of OGM being significantly associated with clinician- and self-rated depressive symptoms at follow-up.
Cue word valence
Cue word valence was not a significant moderator in the four sets of analyses (all p's > 0.05).
Length of follow-up
A longer length of follow-up (in days) was found to predict a smaller regression coefficient of overgeneral/categoric memories [Q(1) = 5.7, p = 0.016, b = −0.0001, p = 0.016) and regression coefficient of specific memories predicting follow-up depressive symptoms over baseline depressive symptoms [Q(1) = 6.6, p = 0.010, b = 0.0007, p = 0.010]. The findings were non-significant for the correlation between overgeneral/categorical memories and depressive symptoms [Q(1) = 1.7, p = 0.182, b = −0.0001, p = 0.182] and the correlation of specific memories [Q(1) = 1.9, p = 0.158, b = 0.0002, p = 0.158]. In summary, these findings are consistent with Sumner et al. (Reference Sumner, Griffith and Mineka2010) reporting that longer follow-up was associated with attenuated effect sizes for the regression of specific memories and extends this to the regression with overgeneral/categoric memories.
Age
The older the mean age of the sample was, the stronger the correlations were for specific memories and depressive symptoms [Q(1) = 4.1, p = 0.028, b = −0.006, p = 0.028], as well as the regression analysis of specific memories [Q(1) = 5.9, p = 0.014, b = −0.008, p = 0.014]. It was not a predictor of the correlation [Q(1) = 1.5, p = 0.210, b = 0.002, p = 0.210] or regression analysis [Q(1) = 1.3, p = 0.247, b = 0.003, p = 0.247] of overgeneral/categorical memories. In summary, there was some evidence that effect sizes for the association between specificity as an index of OGM and depressive symptoms were larger as the mean age of samples increased. This is largely consistent with Sumner et al. (Reference Sumner, Griffith and Mineka2010).
Number of cue words
A higher number of cues words was not a predictor of the correlation between overgeneral/categoric memories and depressive symptoms [Q(1) = 1.6, p = 0.194, b = 0.016, p = 0.194], nor the correlation between specific memories and depressive symptoms[Q(1) = 1.4, p = 0.230, b = −0.019, p = 0.230], or regression analyses of overgeneral/categorical memories [Q(1) = 0.1, p = 0.726, b = −0.005, p = 0.726] or specific memories [Q(1) = 1.7, p = 0.182, b = 0.0007, p = 0.182]. In summary, no evidence was found that the number of cues words in the AMT is related to effect sizes.
Sample gender
A higher proportion of females in samples was associated with a stronger correlation between overgeneral/categorical memories and depressive symptoms [Q(1) = 4.8, p = 0.027, b = 0.005, p = 0.027), but was not related to correlational effects for specific memories [Q(1) = 0.04, p = 0.843, b = −0.0003, p = 0.843], or regression analyses of overgeneral/categoric memories [Q(1) = 0.00, p = 0.960, b = −0.0001, p = 0.960) or specific memories [Q(1) = 0.86, p = 0.352, b = 0.0016, p = 0.352].
Consideration of publication bias
For the correlation analyses, six studies were trimmed for overgeneral/categorical memories, adjusting the point estimate to 0.08 (95% CI 0.06–0.11), and three studies were trimmed for specific memories, adjusting the point estimate to 0.01 (95% CI −0.08 to 0.05). For the regression analyses, four studies were trimmed for overgeneral/categorical memories, adjusting the point estimate to 0.06 (95% CI −0.005 to 0.13) and four studies for specific memories, adjusting the point estimate to −0.07 (95% CI −0.17 to 0.02). Funnel plots are presented in Figures 2–5. These results suggest there was some evidence of publication bias, but the adjusted effect-size estimates did not differ much, and the confidence intervals overlapped considerably with those of the unadjusted effect sizes. These findings are consistent with those of Sumner et al. (Reference Sumner, Griffith and Mineka2010) in showing some evidence of publication bias.

Fig. 2. Funnel plot of effect sizes of OGM correlations with standard error (Y-axis) by Fisher's z (X-axis).

Fig. 3. Funnel plot of effect sizes of OGM regressions with standard error (Y-axis) by point estimate (X-axis).

Fig. 4. Funnel plot of effect sizes of specificity correlations with standard error (Y-axis) by Fisher's z (X-axis).

Fig. 5. Funnel plot of effect sizes of specificity regressions with standard error (Y-axis) by point estimate (X-axis).
Discussion
The aim of this study was to further elucidate the predictive relationship between OGM and the course of depressive symptoms over time by updating and extending on a previous meta-analysis. The tighter confidence intervals across the analyses presented here indicate more precise estimates of these effects relative to those of the previous review. In general, the current findings replicated those of Sumner et al. (Reference Sumner, Griffith and Mineka2010), showing that OGM has a small but significant effect on the course of depressive symptoms. Importantly, this review also replicates the finding that OGM (assessed as overgeneral/categorical and specific memories) predicts the course of depressive symptoms independently of baseline depressive symptoms. Although specific memories did not have a significant zero-order association with depressive symptoms, the high heterogeneity among these studies was explained in part by the moderating effect of clinical status. On this point, there was evidence consistent with Sumner et al. that the association between specific memories and the course of depression is stronger in samples of individuals with clinically-significant psychopathology.
In addition to increasing confidence in previous findings that OGM predicts the course of depressive symptoms, this review extends on previous moderator analyses. Similar to Sumner et al., the rating method for depressive symptoms moderated effects for only one index of OGM, but this time it was correlations of overgeneral/categoric memories rather than regression analysis of specific memories. The results showed that the effects were stronger when clinician-rated approaches were used. The effect sizes in other analyses of OGM indicated that clinician-rated approaches were generally associated with larger effects too; however, the small number of studies means that the power to detect differences remained low. This indicates that ‘caseness’ for depression when determined by a clinician's judgment of diagnostic threshold seems to be associated with OGM more strongly than one's own impression of depressive symptoms. As with Sumner et al.'s review, the addition of more studies in the future may clarify whether this effect is robust across OGM measures.
Longer follow-up between the depression symptom ratings was associated with smaller effect sizes, with statistically significant findings or trends in the same direction across the OGM analyses. As suggested by Sumner et al. (Reference Sumner, Griffith and Mineka2010), these findings may reflect the fact that longer periods of time increase the likelihood that unmeasured variables influence or interact with either OGM or depression to attenuate their relationship. Given this, studies with short follow-ups are likely to find stronger associations relative to studies with longer-term follow-ups. Given that clinical status was associated with stronger effect sizes, and a proportion of people with diagnosed depression remit over time, either with or without treatment (Whiteford et al., Reference Whiteford, Harris, McKeon, Baxter, Pennell, Barendregt and Wang2013), changes for those with diagnosed depression might also partially explain reduced effects over time.
Age emerged as a more consistent predictor of effects across OGM indices than in Sumner et al., indicating that difficulty in retrieving specific memories might become a stronger predictor of the course of depression as people age. Cognitive decline in ageing, such as reduced executive functioning, may explain these stronger effects, particularly given that age is related to more pronounced OGM when assessed using the AMT (Wilson & Gregory, Reference Wilson and Gregory2018). One caveat here is that some recent evidence indicates that older people can outperform younger people when using the measures of OGM other than the AMT, and providing ample time and cues relating to lifetime periods rather than simple word cues might facilitate this retrieval performance (Barry, Gregory et al., Reference Barry, Gregory, Latorre, Ros, Nieto and Ricarte2020a). Regardless, the restricted age range of our samples, with the oldest sample being 52.6 years of age, precludes us from making strong inferences in relation to the current findings.
No evidence was found that cue word valence or the number of cue words in the AMT predicted the strength of effects between OGM and the course of depression. Previous meta-analytic cross-sectional research on OGM depression and other psychopathology has also failed to find significant differences in these characteristics of the AMT (e.g. Liu et al. Reference Liu, Li, Xiao, Yang and Jiang2013; van Vreeswijk & de Wilde, Reference van Vreeswijk and de Wilde2004). For cue valence, no significant effects were found across the different OGM analyses, and neither were there consistent trends in terms of differences of effect sizes for positive or negative cue words. The sample of studies was small though, and therefore not well powered to detect differences if they existed. For the number of cue words, Liu et al. (Reference Liu, Li, Xiao, Yang and Jiang2013) reported that a larger number of cue words was associated with a larger effect of depression on OGM for specific memories, but associated with a smaller effect for overgeneral/categorical memories. In the present study, there was no significant finding of any associations. Given the inconsistency between the current study and previous findings, no clear inferences can be drawn other than that the number of cues words is unlikely to be a reliable predictor of the effects of OGM.
A higher proportion of females in the samples was associated with a stronger correlation between overgeneral/categorical memories and depressive symptoms. However, other test results were clearly non-significant. It is unclear why this effect would be found for only one index of OGM, and only for correlation analyses, and warrants further investigation.
Some important limitations of this study should be noted. As above, the age range of the samples did not extend into older adults, and therefore inferences cannot be extended to this age group. Although OGM does appear to be a feature of depression in older adulthood (Wilson & Gregory, Reference Wilson and Gregory2018), how it predicts the course of depression requires further study. Such research might also examine cognitive functioning to assess whether this interacts with OGM in predicting depression. Another point is that publication bias was detected. Although it was not of a magnitude that changes the overall inferences of the study, the related adjustments tended to reduce the magnitude of effects. This highlights the need for researchers to report their findings regardless of outcomes, which may become more frequent given trends in increased pre-registrations of studies and open access to data. Although this updated review substantially increased the number of studies that were analysed, cell sizes were still typically under 10 studies in the cells of analyses of categorical moderators. This limits the power of the statistical analyses to detect small or moderate effects, and potentially decreases the robustness of findings due to the potential for single studies to exert influence on effect sizes. Studies with longer follow-ups stretching to over 5 years might also give a more thorough picture of how OGM affects the longer-term course of depressive symptoms. It is not entirely clear whether these findings are or are not applicable to specific ethnic/racial groups, as many studies did not report these demographic characteristics. Among those that did report them, more often than not it was a sample that identified predominantly as White/Caucasian. Similarly, with socioeconomic variables such as education and income, generalisability is difficult due to variable reporting. There was a number of samples that included people with low educational attainment and low income, and students.
Given the current findings, continued attention to how OGM is conceptualised as affecting the course of depression is warranted, if not other disorders also (Barry, Hallford, & Takano, Reference Barry, Hallford and Takano2020b). An earlier review of mechanisms of OGM noted some inconsistencies in findings (Sumner, Reference Sumner2012), and further inconsistencies have now emerged in the literature. For example, rumination was thought to be a possible contributor to OGM, and a mediator of OGM and depressive symptoms. However, recent meta-analytic research does not support an association between OGM and self-reported trait rumination (Chiu et al., Reference Chiu, Griffith, Lenaert, Raes, Hermans and Barry2018). One construct strongly related to OGM that is worthy of increased attention is specificity imagining future events. Future thinking appears to be reliant on information stored in autobiographical memory (Schacter, Addis, & Buckner, Reference Schacter, Addis and Buckner2007), and studies have established that specificity in future thinking is also impaired in clinical depression (Hallford, Austin, Takano, & Raes, Reference Hallford, Austin, Takano and Raes2018; Hallford, Austin, Takano, Fuller-Tyszkiewicz, & Raes, Reference Hallford, Barry, Austin, Raes, Takano and Klein2020b). We rely on simulations of the future to anticipate and prepare for problems that we might encounter, to regulate our emotions when faced with problems in the present, to form goals and plan actions, and motivate ourselves for continued goal-oriented living. In the presence of OGM, it may be difficult to engage in such processes and so the course of one's depressive symptoms may worsen. Given this, research may benefit from examining whether future thinking deficits also predict the course of depressive symptoms, and how this might be related to OGM.
Since the last review, research on interventions to increase memory specificity has advanced considerably. There now exists a series of trials of Memory Specificity Training (Raes, Williams, & Hermans, Reference Raes, Williams and Hermans2009), which indicate that specificity can be increased and that this is associated with a reduction in depressive symptoms, including in diagnosed clinical depression, at least in the short-term (Barry et al., Reference Barry, Sze and Raes2019a). Given the seemingly modest contribution of OGM to the course of depression, resource-light interventions such as this may be a feasible approach. Along these lines, studies have begun to examine whether simple, computerised interventions to improve memory specificity can remediate OGM (Martens, Barry, Takano, Onghena, & Raes, Reference Martens, Barry, Takano, Onghena and Raes2019a; Martens et al., Reference Martens, Takano, Barry, Goedleven, Van den Meutter and Raes2019b), with early signs of also alleviating depressive symptoms in clinical samples (Hallford et al., Reference Hallford, Austin, Takano, Fuller-Tyszkiewicz and Raes2020a).
Overall, the results of this updated meta-analysis are consistent with Sumner et al. in finding that OGM has a small effect on the course of depression. This effect seems to be stronger, moderate in size, for individuals with existing diagnoses of depressive disorders. Continued research into this modifiable cognitive process may help to provide an avenue to better understand and treat highly prevalent and impactful depressive disorders.
Financial support
The lead author received support for this research from Deakin University, as part of a 2020 Alfred Deakin Postdoctoral Research Fellowship.
Conflict of interest
None.