INTRODUCTION
Mild cognitive impairment (MCI) may be defined in various ways and comprises a clinically heterogeneous group with variable rates of progression to dementia (Brodaty et al., Reference Brodaty, Aerts, Crawford, Heffernan, Kochan, Reppermund and Trollor2017; Delano-Wood et al., Reference Delano-Wood, Bondi, Sacco, Abeles, Jak, Libon and Bozoki2009). While biomarkers such as magnetic resonance imaging, cerebrospinal fluid (CSF) measures and positron emission tomography ligands are being incorporated into the definition of MCI (Albert et al., Reference Albert, DeKosky, Dickson, Dubois, Feldman, Fox and Petersen2011), prodromal Alzheimer’s disease (AD) (Dubois et al., Reference Dubois, Feldman, Jacova, Cummings, DeKosky, Barberger-Gateau and Galasko2010) and AD (Jack et al., Reference Jack, Bennett, Blennow, Carrillo, Dunn, Haeberlein and Karlawish2018), their availability, cost and invasiveness may limit their widespread adoption. In contrast, clinical criteria remain essential (Dubois et al., Reference Dubois, Feldman, Jacova, Cummings, DeKosky, Barberger-Gateau and Galasko2010) and should be used in all clinical settings without the need for highly specialised investigations (Albert et al., Reference Albert, DeKosky, Dickson, Dubois, Feldman, Fox and Petersen2011). Accordingly, characterising the cognitive phenotype of AD along the continuum from its earliest clinical manifestations to frank dementia is fundamental to understanding the disease’s natural history.
Since Kral’s (Reference Kral1962) original description of benign senescent forgetfulness, there have been multiple iterations of the attempt to define the intermediate state between cognitive health and dementia (Albert et al., Reference Albert, DeKosky, Dickson, Dubois, Feldman, Fox and Petersen2011; American Psychiatric Association, 2013; Crook et al., Reference Crook, Bartus, Ferris, Whitehouse, Cohen and Gershon1986; Graham et al., Reference Graham, Rockwood, Beattie, Eastwood, Gauthier, Tuokko and McDowell1997; Levy, Reference Levy1994; Petersen et al., Reference Petersen, Smith, Waring, Ivnik, Tangalos and Kokmen1999; Winblad et al., Reference Winblad, Palmer, Kivipelto, Jelic, Fratiglioni, Wahlund and Petersen2004). Many modern definitions of the predementia state include objective cognitive impairment on psychometric assessment as a necessary criterion for diagnosis (American Psychiatric Association, 2013; Petersen et al., Reference Petersen, Smith, Waring, Ivnik, Tangalos and Kokmen1999; Winblad et al., Reference Winblad, Palmer, Kivipelto, Jelic, Fratiglioni, Wahlund and Petersen2004). However, impairment in these classifications is either not explicitly defined (Graham et al., Reference Graham, Rockwood, Beattie, Eastwood, Gauthier, Tuokko and McDowell1997; Winblad et al., Reference Winblad, Palmer, Kivipelto, Jelic, Fratiglioni, Wahlund and Petersen2004) or variably defined as 1 (Crook et al., Reference Crook, Bartus, Ferris, Whitehouse, Cohen and Gershon1986; Levy, Reference Levy1994), 1–1.5 (Albert et al., Reference Albert, DeKosky, Dickson, Dubois, Feldman, Fox and Petersen2011), 1.5 (Petersen et al., Reference Petersen, Smith, Ivnik, Tangalos, Schaid, Thibodeau and Kurland1995) or 1–2 (American Psychiatric Association, 2013) SD below the normative mean. This heterogeneity undermines the reliability of MCI as a construct and may compromise its predictive validity because consistent models of prediction cannot be developed (Bradfield & Ames, Reference Bradfield and Ames2020).
The clinical hallmark of typical AD is an impairment of episodic memory characterised by poor learning and rapid memory decay that is not, or only marginally, improved by cueing or recognition (Dubois et al., Reference Dubois, Feldman, Jacova, Cummings, DeKosky, Barberger-Gateau and Galasko2010; McKhann et al., Reference McKhann, Knopman, Chertkow, Hyman, Jack, Kawas and Mayeux2011; Weintraub, Wicklund, & Salmon, Reference Weintraub, Wicklund and Salmon2012). Accordingly, algorithms for predicting progression to clinically classified dementia should focus on performance on memory tests. As described above, many existing classifications adopt a cut-off to define abnormality. One problem with this method is that it introduces Type 1 errors. For example, nearly 16% of a healthy population will perform at or below 1 SD below the mean on any given test (Anastasi & Urbina, Reference Anastasi and Urbina1997). If a battery of neuropsychological tests is administered to a group of healthy adults, a substantial proportion will have at least one performance 1 or even 2 SD below the mean (Binder, Iverson, & Brooks, Reference Binder, Iverson and Brooks2009; Brooks, Iverson, Holdnack, & Feldman, Reference Brooks, Iverson, Holdnack and Feldman2008; Brooks, Iverson, & White, Reference Brooks, Iverson and White2007; Palmer, Boone, Lesser, & Wohl, Reference Palmer, Boone, Lesser and Wohl1998). In the context of MCI, the conventional method of defining impairment with reference to a cut-off on a single test subsumes a subgroup who are less likely to progress to AD dementia and show weaker associations with CSF biomarkers and apolipoprotein E (APOE) ϵ4 status (Clark et al., Reference Clark, Delano-Wood, Libon, McDonald, Nation, Bangen and Bondi2013; Edmonds et al., Reference Edmonds, Delano-Wood, Clark, Jak, Nation, McDonald and Salmon2015; Edmonds et al., Reference Edmonds, McDonald, Marshall, Thomas, Eppig, Weigand and Bondi2019; Eppig et al., Reference Eppig, Edmonds, Campbell, Sanderson-Cimino, Delano-Wood, Bondi and Initiative2017).
Where different tests measure the same aspect of cognition, such as delayed recall for example, true impairment should manifest on a number of those tests. Consequently, imposing a minimum base rate of impaired performance may improve the accuracy of diagnosing neurocognitive disorders (Mistridis et al., Reference Mistridis, Egli, Iverson, Berres, Willmes, Welsh-Bohmer and Monsch2015), including AD dementia (Clark et al., Reference Clark, Delano-Wood, Libon, McDonald, Nation, Bangen and Bondi2013; Edmonds et al., Reference Edmonds, Delano-Wood, Clark, Jak, Nation, McDonald and Salmon2015; Eppig et al., Reference Eppig, Edmonds, Campbell, Sanderson-Cimino, Delano-Wood, Bondi and Initiative2017; Jak et al., Reference Jak, Preis, Beiser, Seshadri, Wolf, Bondi and Au2016; Oltra-Cucarella et al., Reference Oltra-Cucarella, Sanchez-SanSegundo, Lipnicki, Sachdev, Crawford, Perez-Vicente and Ferrer-Cascales2018).
An alternative to a uniform diagnosis of MCI is to stratify individuals with MCI by criteria that putatively differentiate risk of progression to AD dementia. One such method was presented by Winblad et al. (Reference Winblad, Palmer, Kivipelto, Jelic, Fratiglioni, Wahlund and Petersen2004), who revised Petersen et al. (Reference Petersen, Smith, Ivnik, Tangalos, Schaid, Thibodeau and Kurland1995) criteria to include domains other than memory. Subcategories of MCI were established based on the pattern of cognitive domains affected: amnestic single-domain, amnestic multiple-domain, non-amnestic single-domain and non-amnestic multiple-domain (Winblad et al., Reference Winblad, Palmer, Kivipelto, Jelic, Fratiglioni, Wahlund and Petersen2004). Most studies show that multiple-domain amnestic MCI is associated with greater risk of progression to dementia than single-domain amnestic MCI (Bermejo-Pareja et al., Reference Bermejo-Pareja, Contador, Trincado, Lora, Sanchez-Ferro, Mitchell and Benito-Leon2016; Forlenza et al., Reference Forlenza, Diniz, Nunes, Memoria, Yassuda and Gattaz2009; Julayanont, Brousseau, Chertkow, Phillips, & Nasreddine, Reference Julayanont, Brousseau, Chertkow, Phillips and Nasreddine2014; Nordlund et al., Reference Nordlund, Rolstad, Klang, Edman, Hansen and Wallin2010; Palmer, Bäckman, Winblad, & Fratiglioni, Reference Palmer, Bäckman, Winblad and Fratiglioni2008; Summers & Saunders, Reference Summers and Saunders2012; Tabert et al., Reference Tabert, Manly, Liu, Pelton, Rosenblum, Jacobs and Devanand2006), although this finding is not universal (Damian et al., Reference Damian, Hausner, Jekel, Richter, Froelich, Almkvist and Visser2013; Lee et al., Reference Lee, Park, Lee, Moon, Chung and Kang2012; Ravaglia et al., Reference Ravaglia, Forti, Maioli, Martelli, Servadei, Brunetti and Mariani2006). Winblad et al. (Reference Winblad, Palmer, Kivipelto, Jelic, Fratiglioni, Wahlund and Petersen2004) criteria do not operationalise objective cognitive impairment, perhaps underlying the heterogeneity of results regarding progression to AD dementia.
Similarly, the Alzheimer’s Disease Neuroimaging Initiative (ADNI) study has stratified the MCI group into “early” and “late” based on education-adjusted performance on delayed free recall of the paragraph material of Logical Memory, with late MCI being more impaired than early MCI (Petersen et al., Reference Petersen, Aisen, Beckett, Donohue, Gamst, Harvey and Toga2010). However, this method is subject to the same criticisms described above pertaining to false positive classification when relying on a single test score (Edmonds et al., Reference Edmonds, McDonald, Marshall, Thomas, Eppig, Weigand and Bondi2019).
Our broad aim was to address the lack of operational definition for objective memory impairment in MCI.
To this end, we sought to identify ways of predicting incident AD dementia using neuropsychological tests of memory. One way to predict an outcome is to aggregate the total number of risk factors. For example, aggregating the total number of cardiovascular risk factors predicts incident AD (Luchsinger et al., Reference Luchsinger, Reitz, Honig, Tang, Shea and Mayeux2005). We aimed to determine whether an aggregate measure of the number of abnormal memory scores (NAMS) would predict incident AD dementia. We hypothesised that (1) NAMS would correlate positively with the rate of progression to AD dementia; (2) the area under the receiver operating characteristic (ROC) curve for NAMS would be significant and (3) the odds ratio for NAMS would be significant.
METHODS
Participants
Participants were recruited as part of the Australian Imaging, Biomarkers and Lifestyle (AIBL) Flagship Study of Ageing (Ellis et al., Reference Ellis, Bush, Darby, De Fazio, Foster, Hudson and Maruff2009). The AIBL Study is a multi-centre prospective longitudinal cohort study of ageing and AD that measures various biomarkers, cognitive parameters and lifestyle factors at 18-month intervals to allow prediction of future cognitive decline. The study was approved by the Human Research Ethics Committee at St Vincent’s Health (approval 028/06). The AIBL study employed strict exclusion criteria for neurological diseases and psychiatric disorders as well as alcohol use, past head injury, uncontrolled diabetes or obstructive sleep apnoea. With written informed consent, all participants completed an extensive evaluation including medical history, medication use, physical examination, blood samples, health and lifestyle questionnaires as well as a neuropsychological test battery (Ellis et al., Reference Ellis, Bush, Darby, De Fazio, Foster, Hudson and Maruff2009).
Cognitive Examination and Biomarkers
The NAMS variable was derived exclusively from baseline performance on neuropsychological tests of memory used in the AIBL study. These were the California Verbal Learning Test – Second Edition (Delis, Kramer, Kaplan, & Ober, Reference Delis, Kramer, Kaplan and Ober2000), Logical Memory (Wechsler, Reference Wechsler1945) and the Rey Complex Figure (Meyers & Meyers, Reference Meyers and Meyers1995). These three tests yielded 10 indices of memory including immediate recall, delayed recall, percentage retention and recognition (see Table 1). Consistent with the ADNI protocol (Petersen et al., Reference Petersen, Aisen, Beckett, Donohue, Gamst, Harvey and Toga2010), we administered Story A only of Logical Memory (Wechsler, Reference Wechsler1945). As described previously (Bradfield et al., Reference Bradfield, Ellis, Savage, Maruff, Burnham, Darby and Ames2018), we utilised normative data derived from the baseline performance of healthy controls in the AIBL cohort who remained classified as healthy controls at 3-year follow-up.
Table 1. Demographic, cognitive and APOE genotype at baseline and differences between participants who were or were not followed up

Normative data from Delis et al. (Reference Delis, Kramer, Kaplan and Ober2000), Bradfield et al. (Reference Bradfield, Ellis, Savage, Maruff, Burnham, Darby and Ames2018) and Meyers and Myers (Reference Meyers and Meyers1995). CVLT-II = California Verbal Learning Test-Second Edition; MMSE = Mini-Mental State Exam; NAMS = number of abnormal memory scores; t = two-tailed two-sample t-test; WMS = Wechsler Memory Scale; WTAR IQ = Wechsler Test of Adult Reading Intelligence Quotient; χ = Pearson’s chi square.
Performance on each memory index was converted to a z score using normative means. Abnormality on a memory test was defined in terms of performance equal to or greater than 1.5 SD below the normative mean. We chose the threshold of z ≤ −1.5 because it was the level originally described by Petersen et al. (Reference Petersen, Smith, Ivnik, Tangalos, Schaid, Thibodeau and Kurland1995).
Participants were stratified into five levels by years of education (i.e., 0–6, 7–8, 9–12, 13–15 and >15 years). Participants also completed the Wechsler Test of Adult Reading (WTAR) (Holdnack, Reference Holdnack2001) as an estimate of premorbid intelligence quotient (IQ) (Gladsjo, Heaton, Palmer, Taylor, Jeste, Gladsjo et al., Reference Gladsjo, Heaton, Palmer, Taylor and Jeste1999) and the Mini-Mental State Exam (MMSE) (Folstein, Folstein, & McHugh, Reference Folstein, Folstein and McHugh1975) as a measure of general cognitive functioning. Subjective memory complaint was determined by positive endorsement of the question “Do you have difficulties with your memory?” One 0.5 ml tube of whole blood was genotyped for number of APOE ϵ4 alleles (0, 1 or 2) (Ellis et al., Reference Ellis, Bush, Darby, De Fazio, Foster, Hudson and Maruff2009).
Participant Classification
A monthly clinical review panel meeting chaired by David Ames (DA) comprised old age psychiatrists, neurologists, geriatricians and neuropsychologists (with a minimum of three panellists including at least one medical practitioner and at least one neuropsychologist forming a quorum). The panel, which was blind to biomarker status, classified all participants at baseline as healthy control, MCI as defined in the AIBL cohort or AD dementia based on psychometric performance, subjective memory complaint status, informant report and functional performance. The previously described AIBL definition of MCI (Ellis et al., Reference Ellis, Bush, Darby, De Fazio, Foster, Hudson and Maruff2009) was disregarded in the present study, and these individuals were grouped with healthy controls as not having dementia at all time points.
At 3-year follow-up, the panel reviewed cases with a diagnosis of AD or AIBL-defined MCI, or healthy controls who showed psychometric impairment, relevant medical history, report of cognitive decline or use of medications that could affect cognition. The panel re-evaluated the classification of each of these participants as healthy control, AIBL-MCI (who were again classified in the present study as not having dementia) or AD dementia according to Diagnostic and Statistical Manual of Mental Disorders, fourth edition (DSM-IV) (American Psychiatric Association, 1994), International Classification of Diseases (ICD)-10 (World Health Organization, 1992) and National Institute of Neurological and Communicative Disorders and Stroke and the Alzheimer’s Disease and Related Disorders Association (NINCDS-ADRDA) (McKhann et al., Reference McKhann, Drachman, Folstein, Katzman, Price and Stadlan1984) criteria. At 3-year follow-up, the panel made its classification based on 3-year follow-up psychometric performance, subjective memory complaint, informant report and functional status as well as 18-month follow-up classification. At 3-year follow-up review, the panel was blind to baseline information including psychometric performances (i.e., those used to derive NAMS) and panel classification. It is possible that some panellists may have recalled a de-identified participant from 3 years previously, though given the large number of participants classified, the gap between classification at the time points studied and turnover in panel members from meeting to meeting, this seems unlikely to have been a common occurrence.
In conjunction with the panel classification, a neuropsychologist, who was also blind to the baseline performances used to generate the composite measure NAMS, completed the Clinical Dementia Rating scale (CDR) (Hughes, Berg, Danziger, Coben, & Martin, Reference Hughes, Berg, Danziger, Coben and Martin1982) for each participant based on psychometric performance, clinical interview and informant interview from 3-year follow-up time point only. The rating neuropsychologist was blind to all consensus panel classifications, APOE4 status and all previous psychometric performances. The AIBL study was designed to ensure that the neuropsychologist completing the CDR at each time point had not assessed that participant previously. Once completed by the neuropsychologist, DA reviewed each CDR rating (O’Connor et al., Reference O’Connor, Blessed, Cooper, Jonker, Morris, Presnell and Schaufele1996).
Statistical Analyses
First, we examined the association of each individual memory index (see Table 1) with AD using logistic regression models adjusting for age, sex and education. Each memory index that was significant at p < .005 (.05/10 Bonferroni corrected) was retained for further analyses. For each participant, we then summed the number of retained memory scores that were abnormal (i.e., NAMS).
Multiple collinearity refers to explanatory variables that are highly linearly related. This was not an issue with our current analysis because the memory indices, although correlated, were not entered simultaneously into any regression equation as explanatory variables. This methodology has precedence, for example, in terms of aggregating number of cardiovascular risk factors to predict incident dementia (Luchsinger et al., Reference Luchsinger, Reitz, Honig, Tang, Shea and Mayeux2005) and is analogous to cumulative risk models in developmental psychology (Hooper, Burchinal, Roberts, Zeisel, & Neebe, Reference Hooper, Burchinal, Roberts, Zeisel and Neebe1998).
For each level of NAMS (i.e., 0–10), we calculated the percentage that was diagnosed with AD dementia at follow-up and labelled this “progression rate”. We also calculated sensitivity and specificity for each cut-off level of NAMS. We then calculated positive and negative predictive values (PPV and NPV) for each cut-off level of NAMS based on the prevalence of AD dementia in the current cohort (7.5%). We also calculated PPV and NPV using Bayes’ theorem (Altman & Bland, Reference Altman and Bland1994) for the point prevalence of AD dementia in the general population over 65 years (4.4%) (Lobo et al., Reference Lobo, Launer, Fratiglioni, Andersen, Di Carlo, Breteler and Martinez-Lage2000), and in referrals to a tertiary memory clinic (20%) (Verhey et al., Reference Verhey, Jolles, Ponds, Rozendaal, Plugge, De Vet and Van der Lugt1993). PPV for these populations was defined as [Sensitivity × Prevalence]/[Sensitivity × Prevalence + (1 – Sensitivity) × (1 – Prevalence)] and NPV was defined as [Specificity × (1 – Prevalence)]/[Specificity × (1 – Prevalence) + (1 – Specificity) × Prevalence] (Parikh, Mathai, Parikh, Chandra Sekhar, & Thomas, Reference Parikh, Mathai, Parikh, Chandra Sekhar and Thomas2008).
We calculated Pearson’s r correlation coefficient between NAMS and progression rate after excluding NAMS = 0, the inclusion of which would have produced an intercept of the x axis and thus a negative probability of progressing to AD dementia. We calculated the regression equation for this association and used the slope of this line to represent the additional risk that each abnormal memory score would confer on the likelihood of developing AD dementia. Next, we calculated the area under the ROC curve for NAMS with the dependent variable being diagnosis of AD dementia or not at follow-up.
We performed logistic regression to control for demographic and other confounding variables. The dependent variable was the diagnosis of AD dementia at 3 years or not. The continuous explanatory variables were NAMS, age, WTAR-estimated Full Scale IQ and MMSE total score (MMSE score was multiplied by −1 for ease of interpretation). Categorical explanatory variables were sex, number of APOE ϵ4 alleles (0, 1 or 2), education and subjective memory complaint. We included all explanatory variables in the first logistic regression model and then ordered them by their magnitude of effect. We then performed a second analysis that utilised a manual stepwise regression procedure whereby each variable was added in turn beginning with the one with the greatest effect. The p values were set at .05 so that each variable was expected to increase the prediction of the model to determine at which point adding further variables did not improve its predictive accuracy.
To compare the agreement between CDR rating and consensus panel classification, Cohen’s (Reference Cohen1960) kappa coefficient was calculated. To this end, CDR score was binarised to “CDR less than 1” or “CDR equal to or greater than 1” and consensus panel classification was binarised to “not having AD dementia” and “having AD dementia”. Data were analysed using Stata (StataCorp; Stata/IC 15.1 for Mac).
RESULTS
There were 1166 participants recruited. Fifty-four people were excluded due to comorbid illness (Ellis et al., Reference Ellis, Bush, Darby, De Fazio, Foster, Hudson and Maruff2009), 211 were excluded due to a diagnosis of AD dementia and 5 were excluded due to missing all neuropsychological data, leaving 896 individuals whom had not been diagnosed with dementia at baseline. Of these 896 individuals, 249 (27.8%) had subjective memory complaint and impairment (i.e., z ≤ −1.5) on at least one memory measure, which would correspond to the classical definition of amnestic MCI.
A total of 722 (80.6%) participants were followed up at 3 years: 54 (7.5%) developed AD dementia, 3 (0.4%) developed vascular dementia, 1 (0.1%) developed Parkinson’s disease and 27 (3.0%) were deceased. The four participants who developed vascular dementia and the participant who developed Parkinson’s disease were classified as not having AD dementia in the outcome variable.
Compared to the participants who were followed up, the individuals lost to follow-up were older and had lower MMSE and memory scores, but had similar sex distribution, years of education, prevalence of subjective memory complaint, WTAR-IQ and APOE ϵ4 frequency (see Table 1). There was good agreement between consensus panel classification and CDR rating at 3-year follow-up, κ = .70 (95% CI .66–.74), p < .0001.
In logistic regression analyses examining each memory index adjusting for age, sex and education, all 10 memory indices had a significant odds ratio of developing AD dementia and thus met the criterion for consideration in further analyses (i.e., p < .005; see Table 2). Participants who developed AD dementia performed significantly more poorly on all 10 memory measures at baseline (all p < .001; see Table 3).
Table 2. Odds ratios for each individual memory index after adjusting for age, sex and education

CVLT-II = California Verbal Learning Test-Second Edition; WMS = Wechsler Memory Scale; WTAR-IQ = Wechsler Test of Adult Reading Intelligence Quotient.
Table 3. Differences between participants who developed AD dementia and those who did not on demographic variables and baseline psychometric performance

Normative data from Delis et al. (Reference Delis, Kramer, Kaplan and Ober2000), Bradfield et al. (Reference Bradfield, Ellis, Savage, Maruff, Burnham, Darby and Ames2018) and Meyers and Myers (Reference Meyers and Meyers1995). CVLT-II = California Verbal Learning Test-Second Edition; MMSE = Mini-Mental State Exam; NAMS=number of abnormal memory scores; t=two-tailed two-sample t-test; WMS = Wechsler Memory Scale; WTAR IQ = Wechsler Test of Adult Reading Intelligence Quotient; χ=Pearson’s chi square.
There was a strong positive correlation between NAMS and progression rate (r = .91, p = .0003; see Table 4). The simple linear regression to predict progression rate based on NAMS was significant (F (1,8) = 38.0, p < .001). Rate of progression increased by 9.8% with each abnormal memory score. Similar results were seen when the correlation was repeated with CDR as the outcome variable (r = .78, p = .0075).
Table 4. NAMS and rates of progression to AD dementia

AD = Alzheimer’s disease; CI=confidence interval; NAMS=number of abnormal memory scores.
Sensitivity, Specificity and ROC Analyses
For the various cut points for NAMS, sensitivity ranged from 3.7 to 87% and specificity ranged from 66.8 to 100% (see Table 5). Given the data were not binormally distributed, a non-parametric analysis of the ROC Area under the curve (AUC) was performed. The AUC for NAMS (see Figure 1) was 0.87 (95% CI 0.81–0.93), p < .001. The cut point of NAMS closest to (0,1) was 1.5. At this threshold, sensitivity was .80, specificity was .83 and AUC was .82. Similar results were seen when the ROC analysis was repeated with CDR as the outcome variable with AUC .84 (95% CI .77–.92), p < .001.
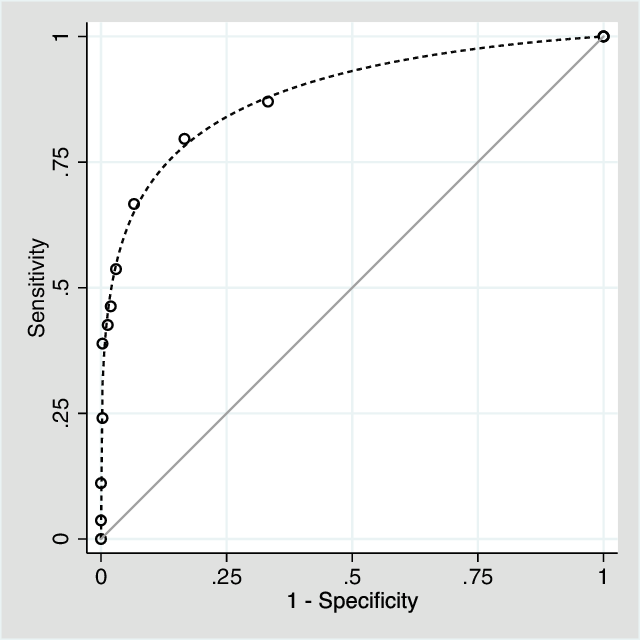
Fig. 1. Receiver operating characteristic curve for number of abnormal memory scores in predicting incident AD dementia.
Table 5. Sensitivity and specificity for each level of NAMS and progression to AD dementia

CI = confidence interval; NAMS = number of abnormal memory scores.
Table 6 shows the PPV and NPV for each level of NAMS according to three different prevalence rates: the general population, the present study and a tertiary memory clinic.
Table 6. Positive and negative predictive values for each level of NAMS according to different prevalence rates

CI = confidence interval; N/A = not applicable; NAMS = number of abnormal memory scores; NPV = negative predictive value; PPV = positive predictive value.
The four measures from the California Verbal Learning Test-Second Edition (CVLT-II) yielded larger beta coefficients than measures from the other memory tests. We conducted post hoc analyses with NAMS for the four CVLT-II memory variables. Accordingly, we calculated NAMS for the CVLT-II measures (range 0–4). The ROC AUC for CVLT-II NAMS (.81, 95% CI .74–.88) was significantly less than that for NAMS with all 10 memory indices, χ 2 (1, N = 722) = 5.42, p = .02.
Logistic Regression
The initial logistic regression model included age, sex, education, estimated IQ, MMSE, subjective memory complaint, APOE ϵ4 status and NAMS (see Table 7). The odds ratio for NAMS in the initial model was 1.74 (95% CI 1.44–2.10, p < .001). The AUC for this model was .97 (95% CI .95–.98). The odds ratio for NAMS in the final model was 1.67 (95% CI 1.40–2.01, p < .001) (see Table 8). The AUC for this model was .96 (95% CI .94–.98). Similar results were seen when the final logistic regression was repeated with CDR as the outcome variable, with the odds ratio for NAMS in the final model being 1.29 (95% CI 1.12–1.49), p < .001.
Table 7. Initial logistic regression model containing all variables

APOE = apolipoprotein E; CI = confidence interval; MMSE = Mini-Mental State Exam; NAMS = number of abnormal memory scores; SMC = subjective memory complaint; WTAR IQ = Wechsler Test of Adult Reading Intelligence Quotient. N.B. Constant term not reported.
Table 8. Final logistic regression model containing only retained variables

APOE = apolipoprotein E; CI = confidence interval; MMSE = Mini-Mental State Exam; NAMS = number of abnormal memory scores; SMC = subjective memory complaint. N.B. constant term not reported.
DISCUSSION
We have demonstrated in a prospective cohort study that individuals who were impaired on a greater number of memory tests were more likely to progress to AD dementia at 3-year follow-up. Each of the 10 individual neuropsychological measure of memory, which were set at a threshold of z ≤ − 1.5 to define abnormality, significantly predicted progression after correcting for age, sex and education (all p < .001). The number of impaired memory scores was aggregated to derive a composite measure of memory impairment (i.e., NAMS), which correlated with rate of progression and predicted incident AD dementia after correcting for age, sex, education, estimated IQ, subjective memory complaint, MMSE score and APOE ϵ4 status.
Our findings reinforce the notion that there is great heterogeneity within individuals demonstrating evidence of objective memory impairment (Brodaty et al., Reference Brodaty, Aerts, Crawford, Heffernan, Kochan, Reppermund and Trollor2017), with progression rates in our study ranging from 3.5 to 100% depending on NAMS. In the context of MCI, defining memory impairment using a single test identifies many individuals who will not progress to AD dementia (Clark et al., Reference Clark, Delano-Wood, Libon, McDonald, Nation, Bangen and Bondi2013; Edmonds et al., Reference Edmonds, Delano-Wood, Clark, Jak, Nation, McDonald and Salmon2015; Eppig et al., Reference Eppig, Edmonds, Campbell, Sanderson-Cimino, Delano-Wood, Bondi and Initiative2017; Jak et al., Reference Jak, Preis, Beiser, Seshadri, Wolf, Bondi and Au2016; Oltra-Cucarella et al., Reference Oltra-Cucarella, Sanchez-SanSegundo, Lipnicki, Sachdev, Crawford, Perez-Vicente and Ferrer-Cascales2018). Previous research has shown that aggregating abnormally low scores on cognitive tests can predict incident AD dementia (Oltra-Cucarella et al., Reference Oltra-Cucarella, Sanchez-SanSegundo, Lipnicki, Sachdev, Crawford, Perez-Vicente and Ferrer-Cascales2018), but our study is the first to do so using specifically tests of memory and provides PPV and NPV relevant to prevalence in the general elderly population as well as in tertiary memory clinic referrals. For example, an individual referred to a tertiary memory clinic may have one memory score z ≤ − 1.5 on the neuropsychological battery in this study. While such an individual may be classified as MCI according to various taxonomies (Albert et al., Reference Albert, DeKosky, Dickson, Dubois, Feldman, Fox and Petersen2011; American Psychiatric Association, 2013; Petersen et al., Reference Petersen, Smith, Ivnik, Tangalos, Schaid, Thibodeau and Kurland1995), our results suggest such an individual would have a 95% CI (91.2–97.6) chance of remaining free of AD dementia at 3-year follow-up. The majority of the sample (453/722 = 67.2%) had zero abnormally low memory scores and these individuals were unlikely to develop AD dementia during 3-year follow-up period (1.5%; 95% CI 0.6–3.2).
It is interesting to note that MMSE scores were also useful in predicting incident AD dementia. This finding may well reflect the large weighting the MMSE has on orientation, which itself predicts the development of AD dementia (Defrancesco et al., Reference Defrancesco, Marksteiner, Deisenhammer, Kemmler, Djurdjevic and Schocke2013; Lee et al., Reference Lee, Ritchie, Yaffe, Stijacic Cenzer and Barnes2014). The MMSE, however, has little role as a standalone instrument in the prediction of progression from MCI to AD dementia (Arevalo-Rodriguez et al., Reference Arevalo-Rodriguez, Smailagic, Roqué i Figuls, Ciapponi, Sanchez-Perez, Giannakou and Cullum2015).
The CVLT-II measures yielded the largest beta coefficients for predicting incident AD dementia (see Table 2). It would be convenient to have a single memory test to predict incident dementia. Although generating NAMS for the CVLT-II yielded a significant ROC AUC, it was not as accurate as the NAMS derived from all 10 memory indices, which suggests that a battery of memory tests better predicted progression to AD dementia than did the CVLT-II as a standalone test. Future studies may wish to analyse the predictive utility of the CVLT-II as a standalone measure or in conjunction with other measures such as the MMSE.
Taken together, our findings suggest that NAMS is a useful way of predicting development of incident AD dementia. It follows that NAMS could be a useful way of operationalising objective memory impairment in MCI. We have now demonstrated that individuals can be stratified for their risk of progressing to AD dementia according to the severity of memory impairment (Bradfield et al., Reference Bradfield, Ellis, Savage, Maruff, Burnham, Darby and Ames2018) and aggregation of NAMS. This provides further support for a conceptual shift away from defining MCI as a categorical diagnosis to one that may be graded by severity.
A limitation to the present study is that it was a retrospective analysis of a prospective cohort study. As such, it may be subject to confirmation bias. To address this, an additional data set from an expansion cohort from the AIBL Study will be examined to test the conclusions of this study when sufficient individuals have been followed up for 3 years. Another limitation of the study was that the panel for consensus classification was not entirely blind to psychometric performance and therefore there may be an element of circularity and criterion contamination. However, the construct of NAMS was only conceived after the AIBL data were collected. Furthermore, baseline psychometric performances, from which NAMS was derived, were not used at follow-up classification by the panel. Nonetheless, to redress any potential influence of this lack of blinding, key analyses were repeated with CDR, which was rated by a neuropsychologist who was completely blind to the baseline data used to derive NAMS. These CDR analyses were all consistent with the results generated by the panel classification and also supported all hypotheses.
One limitation of aggregating all memory performances into single composite measure is that it does not allow distinction between encoding-, consolidation- or retrieval-based memory difficulties. Another limitation of this study relates to its generalisability. A clinician administering a different neuropsychological battery to that utilised by the present study may find it difficult to apply explicitly the current findings to predict probability of progression to AD dementia. However, Logical Memory, CVLT-II and the Rey Complex Figure are commonly administered tests. A future study could employ different memory measures to determine whether these results are generalisable to a different neuropsychological battery.
If replicated, these findings would have important implications for clinicians working with individuals presenting with amnestic MCI in settings such as a memory clinic. A brief and non-invasive neuropsychological assessment battery of memory tests would be useful for providing prognosis in such a setting. Normal performance on the memory battery should reassure the clinician and individual that he or she is unlikely to develop AD dementia in the coming years. On the other hand, an individual who performs poorly on a majority of memory tests may warrant more detailed investigations, frequent follow-up and ensuring medicolegal affairs are in order while decision-making capacities are still retained. Another potential benefit of refining the prognostic utility of MCI is to identify individuals who may be selected for future research on the natural history of AD. By extension, it may also allow selection of individuals who are good candidates for recruitment into clinical trials.
CONCLUSION
Memory impairment in MCI that manifests on a range of psychometric memory tests may reduce the likelihood of false positive classification and improve the predictive validity of MCI by allowing better prediction of progression to AD dementia. As such, aggregating the NAMS may be a useful way to operationalise objective memory impairment in MCI. Similarly, cognitive parameters such as NAMS and severity of memory impairment are a simple way to provide differential prognostication within the heterogeneous group defined by current MCI criteria. These findings have important implications for prognosis and help us understand the natural history of AD, which could have benefits both in research and clinical settings. Psychometric examination is an affordable, non-invasive, widely available and low risk method for identifying cognitive health and stratifying individuals by their likelihood of developing AD dementia.
Acknowledgments
We thank all those who took part as participants in the study as well as the entire AIBL team for their commitment and dedication to helping advance research into the early detection and causation of AD.
CONFLICT OF INTEREST
The authors declare no conflict of interest.
FINANCIAL SUPPORT
Core funding for the AIBL study was provided by Commonwealth Scientific Industrial Research Organization (CSIRO), which was supplemented by in-kind contributions from the study partners (see http://www.aibl.csiro.au/): University of Melbourne, Neurosciences Australia Ltd (NSA), Edith Cowan University (ECU), Mental Health Research Institute (MHRI), Alzheimer’s Australia (AA), National Ageing Research Institute (NARI), Austin Health, University of WA (UWA), CogState Ltd., Macquarie University, Hollywood Private Hospital and Sir Charles Gairdner Hospital.