Introduction
Images of mutilated bodies and lifeless children proliferated across WhatsApp group chats in northern India in 2018, allegedly resulting from an organized kidnapping network. In response to these messages, a young man mistaken for one of the kidnappers was mobbed and brutally beaten by villagers. The images, however, were not from a kidnapping network but rather from a chemical weapons attack in Ghouta, Syria in 2013. Mob lynchings such as this have become a prominent problem in India since 2015, when a Muslim villager in Uttar Pradesh was killed by a mob after rumors spread that he was storing beef in his house. Such misinformation campaigns are often developed and run by political parties with nationwide cyber armies, targeting political opponents, religious minorities, and dissenting individuals (Poonam and Bansal Reference Poonam and Bansal2019). The consequences of such rumors are as extreme as violence, demonstrating that misinformation is a matter of life and death in India and other developing countries.
What tools, if any, exist to combat the misinformation problem in developing countries? Nearly all of the extant literature on combating misinformation focuses on the United States and other developed democracies, where misinformation spreads via public sites such as Facebook and Twitter. Interventions in these contexts are not easily adapted for misinformation distributed on encrypted chat applications such as WhatsApp, where no one, including the app developers themselves, can see, read, or analyze messages. Encryption necessitates that the the burden of fact-checking falls solely on the user and therefore, the more appropriate solutions in such contexts are bottom-up, user-driven learning and fact-checking to combat misinformation.
This study is one such bottom-up effort to counter misinformation with a broad pedagogical program. I investigate whether improving information-processing skills changes actual information processing in a partisan environment. The specific research question asked in this paper is whether in-person, pedagogical training to verify information is effective in combating misinformation in India. To answer this question, I implemented a large-scale field experiment with 1,224 respondents in the state of Bihar in India during the 2019 general elections, when misinformation was arguably at its peak. In an hour-long intervention, treatment group respondents were taught inoculation strategies and concrete tools to verify information, and they were given corrections to false stories. After a two-week period, respondent households were revisited to measure their ability to identify misinformation.
My experiment shows that an hour-long educative treatment is not sufficient to help respondents combat misinformation. Importantly, the average treatment effect is not significantly distinguishable from zero. Finding that an in-person, hour-long, and bottom-up learning intervention does not move people’s prior attitudes is testimony to the tenacity and destructive effects of misinformation in low-education settings such as India. These findings also confirm qualitative evidence about the distinctive nature of social media consumers in developing states who are new to the Internet, lending them particularly rife and vulnerable to misinformation.
While there is no evidence of a nonzero average treatment effect, there are significant treatment effects among subgroups. Bharatiya Janata Party (BJP) partisans (those self-identifying as supporters of the BJP, the national right-leaning party in India) who receive the treatment are less likely to identify pro-attitudinal stories as false. That is, on receiving counterattitudinal corrections, the treatment backfires for BJP respondents while simultaneously working to improve information processing for non-BJP respondents. This is consistent with findings in American politics on motivated reasoning, demonstrating that respondents seek out information reinforcing prior beliefs and that partisans cheerlead for their party and are likely to respond expressively to partisan questions (Gerber and Huber Reference Gerber and Huber2009; Prior, Sood, and Khanna Reference Prior, Sood and Khanna2015; Taber and Lodge Reference Taber and Lodge2006). These findings also challenge the contention that Indians lack consolidated, strong partisan identities (Chhibber and Verma Reference Chhibber and Verma2018). I demonstrate that party identity in India is more polarized than previously thought, at least with BJP partisans and during elections.
This study hopes to spark a research agenda on the ways to create an informed citizenry in low-income democracies through testing and implementation of bottom-up measures to fight misinformation. I also seek to contribute to the empirical study of partisan identity in India, revisiting the conventional wisdom of party identities being unconsolidated and fluctuating.
What is Misinformation and How Do We Fight It?
I define misinformation as claims that contradict or distort common understanding of verifiable facts (Guess and Lyons Reference Guess, Lyons, Persily and Tucker2020) and fabrications that are low in facticity (Li Reference Li2020; Tandoc, Lim, and Ling Reference Edson, Lim and Ling2018).
The literature on misinformation identifies three key components of false stories: (1) low levels of facticity, (2) journalistic presentation, and (3) intent to deceive (Egelhofer and Lecheler Reference Egelhofer and Lecheler2019; Farkas and Schou Reference Farkas and Schou2018). Given my focus on misinformation in India, my definition does not include the format of the news. In India, misinformation is spread via WhatsApp where much of it is in the form of text messages, with the content copied and pasted into the body of the message such that it exists as standalone content. Therefore, this cannot mimic legitimate news websites and is rarely presented in a journalistic format. Further, while the creation of falsehoods in the Indian context can stem from organized attempts by political parties with the intention to deceive, users in WhatsApp groups who are the victims of such campaigns may further propagate falsehoods inadvertently or unintentionally. Thus, my definition also leaves out the intention to deceive, defined in the literature as “disinformation” (Tucker et al. Reference Tucker, Guess, Barberá, Vaccari, Siegel, Sanovich and Stukal2018).
A predominant model of misinformation comes from Gentzkow, Shapiro, and Stone (Reference Gentzkow, Shapiro, Stone, Anderson, Waldfogel and Strömberg2015). They posit that consumption of misinformation is a result of preferences for confirmatory stories rather than the truth because of the psychological utility from such stories. We tend to seek out information that reinforces our preferences, counterargue information that contradicts preferences, and view pro-attitudinal information as more convincing than counterattitudinal information (Taber and Lodge Reference Taber and Lodge2006). Thus individuals’ preexisting beliefs strongly affect their responses to corrections (Flynn, Nyhan, and Reifler Reference Flynn, Nyhan and Reifler2017). Importantly, a number of contextual and individual moderators of such motivated reasoning predispose subsets of the population to be more vulnerable to misinformation.
The two primary political factors that contribute to the vulnerability to misinformation effects are political sophistication and ideology (Wittenberg and Berinsky Reference Wittenberg, Berinsky, Persily and Tucker2020). More politically sophisticated individuals (including political knowledge and education) are more likely to be resistant to corrections (Valenzuela et al. Reference Valenzuela, Halpern, Katz and Miranda2019) and are the least amenable to updating beliefs when misinformation supports their existing worldviews (Lodge and Taber Reference Lodge and Taber2013). Additionally, differences in informedness can affect how well corrective information helps individuals develop knowledge about current events: Li and Wagner (Reference Li and Wagner2020) find that uninformed individuals are more likely to update their beliefs than misinformed individuals after exposure to corrective information. Further, ideology and partisanship are associated with differences in response to corrections. Although everyone is vulnerable to misinformation to a certain extent, worldview backfire effects are more visible for Republicans but not Democrats, given that the insular nature of the conservative media system is more conducive to the spread of misinformation (Ecker and Ang Reference Ecker and Ang2019; Faris et al. Reference Faris, Roberts, Etling, Bourassa, Zuckerman and Benkler2017; Nyhan and Reifler Reference Nyhan and Reifler2010).
Apart from political factors, research highlights age as a key demographic variable influencing both exposure to misinformation as well as responses to it. Studies find that older adults are more likely than others to share misinformation (Grinberg et al. Reference Grinberg, Joseph, Friedland, Swire-Thompson and Lazer2019) and that the relationship between age and vulnerability to misinformation persists even after controlling for partisanship and ideology.
But despite the growing attention to misinformation in media and scholarship, empirical literature finds that the online audience for misinformation is a small subset of the total online audience. Those consuming false stories are a small, disloyal group of heavy internet users (Nelson and Taneja Reference Nelson and Taneja2018): Grinberg et al. (Reference Grinberg, Joseph, Friedland, Swire-Thompson and Lazer2019) find that 1% of Twitter users in their sample account for 80% of misinformation exposures; Guess, Nyhan, and Reifler (Reference Guess, Nyhan and Reifler2018) find that almost 6 in 10 visits to fake websites came from the 10% of people with the most conservative online information diets. However, though people online are not clamoring for a continuous stream of false stories, misinformation in a multifaceted and fast-paced online environment can command people’s limited attention (Guess and Lyons Reference Guess, Lyons, Persily and Tucker2020). Such misinformed beliefs are especially troubling when they lead people to action, as these skewed views may well alter political behavior (Hochschild and Einstein Reference Hochschild and Einstein2015).
A large research agenda has tested interventions to reduce the consumption of misinformation. These interventions can be grouped into reactive, or top-down efforts that are implemented after misinformation is seen, and proactive, or bottom-up efforts that seek to fight misinformation before it has been encountered.
Examples of top-down interventions include providing corrections, warnings, or fact-checking, and consequently measuring respondents’ perceived accuracy of news stories. For instance, in 2016 Facebook began adding “disputed” tags to stories in its newsfeed that had been previously debunked by fact-checkers (Mosseri Reference Mosseri2017); it then switched to providing fact-checks underneath suspect stories (Smith, Jackson, and Raj Reference Smith, Jackson and Raj2017). Chan et al. (Reference Chan, Jones, Jamieson and Albarracín2017) find that explicit warnings can reduce the effects of misinformation; Pennycook, Cannon, and Rand (Reference Pennycook, Cannon and Rand2018) test and find that disputed tags alongside veracity tags can lead to reductions in perceived accuracy; Fridkin, Kenney, and Wintersieck (Reference Fridkin, Kenney and Wintersieck2015) demonstrate that corrections from professional fact-checkers are more successful at reducing misperceptions.
Bottom-up interventions to combat misinformation rely on inoculation theory, the idea of preparing people for potential misinformation by exposing logical fallacies inherent in misleading communications a priori (Compton Reference Compton, Dillard and Shen2013). To this end, Tully, Vraga, and Bode (Reference Tully, Vraga and Bode2020) and Vraga, Bode, and Tully (Reference Vraga, Bode and Tully2020) conduct experiments where treatment-group respondents were reminded to be critical consumers of the news via tweets encouraging people to distinguish between high- and low-quality news. Wineburg and McGrew (Reference Wineburg and McGrew2019) demonstrate that lateral reading (i.e., cross-checking information with additional sources) led to more warranted conclusions relative to vertical reading (i.e., staying within a website to evaluate its reliability). Cook, Lewandowsky, and Ecker (Reference Cook, Lewandowsky and Ullrich2017) inoculated respondents against misinformation by presenting mainstream scientific views alongside contrarian views.
Closer in design to the present study, Guess et al. (Reference Guess, Lerner, Lyons, Montgomery, Nyhan, Reifler and Sircar2020) evaluate a digital literacy intervention in India and the United States using “tips” provided by WhatsApp to measure whether they are effective at increasing the perceived accuracy of true stories. In Hameleers (Reference Hameleers2020), similar tips to spot misinformation are paired with fact-checking in a bundled treatment.
Moving beyond the Western context, a small but burgeoning literature looks at misinformation on WhatsApp in developing countries. In Brazil, Rossini et al. (Reference Rossini, Stromer-Galley, Baptista and Oliveira2020) compare misinformation sharing dynamics on WhatsApp and Facebook and find that those who are more engaged in political talk are significantly more likely to have shared misinformation. In Zimbabwe, Bowles, Larreguy, and Liu (Reference Bowles, Larreguy and Liu2020) use WhatsApp to disseminate messages intended to target COVID-19 misinformation and find that the intervention resulted in significantly greater knowledge about best practices. In Indonesia, Mujani and Kuipers (Reference Mujani and Kuipers2020) find that younger, better-educated, and wealthier voters were more likely to believe misinformation. Finally, in India Garimella and Eckles (Reference Garimella and Eckles2020) use machine learning algorithms to analyze WhatsApp messages and determine that image misinformation is highly prevalent on public WhatsApp groups.
Despite the inroads made in the study of misinformation in developing countries, the problem of fake news in these contexts continues to grow. The next sections outline the challenge posed by misinformation in developing countries and the need for solutions and interventions specific to those contexts.
Dissemination of Misinformation in India: The Supply
This study was conducted in May 2019 during the general election in India, the largest democratic exercise in the world. The 2019 contest was a reelection bid for Narendra Modi, leader of India’s Hindu nationalist Bharatiya Janata Party (BJP).
This election was distinctive because it allowed for campaigning to be conducted over the Internet, and chat-based applications such as WhatsApp became a primary communication tool for parties. For example, the BJP drew plans to have WhatsApp groups for each of India’s 927,533 polling booths. A WhatsApp group can contain a maximum of 256 members, so this communication strategy potentially reached 700 million voters. This, coupled with WhatsApp being the social media application of choice for over 90% of Internet users, led the BJP’s social media chief to declare 2019 the year of India’s first “WhatsApp elections” (Uttam Reference Uttam2018). Survey data from this period in India find that one sixth of the respondents said they were members of a WhatsApp group chat started by a political leader or party (Kumar and Kumar Reference Kumar and Kumar2018).
Unlike the United States where the focus has been on foreign-backed misinformation campaigns, political misinformation circulating in India appears to be largely domestically manufactured. The information spread on such political WhatsApp groups is not only partisan but also hate-filled and often false (Singh Reference Singh2019). This trend is fueled by party workers themselves: ahead of the 2019 election, national parties hired armies of volunteers “whose job is to sit and forward messages” (Perrigo Reference Perrigo2019). Singh (Reference Singh2019) reports that the BJP directed constituency-level volunteers to sort voters into groups created along religious and caste lines, even location, socioeconomic status, and age, such that specific messages could be targeted to specific WhatsApp groups. Then BJP President Amit Shah underscored this during a public address in 2018: “We can keep making messages go viral, whether they are real or fake, sweet or sour” (The Wire Staff 2018). Misinformation is inherently political in India, and the creators of viral messages are often parties themselves.
Vulnerability to Misinformation in India: The Demand
WhatsApp group chats morph into havens for misinformation in India. Four characteristics make their users vulnerable to misinformation.
First, literacy and education rates are low across the developing world. India’s literacy and formal education rates are relatively low compared with those of other developing countries where misinformation has been shown to affect public opinion (Figure 1). Further, the sample site for this study—the state of Bihar in India—has historically had one of the lowest literacy rates in the country. Research has demonstrated a strong relationship between levels of education and vulnerability to misinformation. While people with higher levels of education have more accurate beliefs (Allcott and Gentzkow Reference Allcott and Gentzkow2017), motivated reasoning gives them better tools to argue against counterattitudinal information (Nyhan et al. Reference Nyhan, Porter, Reifler and Wood2019). We should thus expect that vulnerability to misinformation is influenced by lower literacy and education.

Figure 1. India Has Low Levels of Literacy and Education
Second, Internet access has exploded in the developing world. India, particularly, is digitizing faster than most mature and emerging economies, driven by the increasing availability and decreasing cost of high-speed connectivity and smartphones, and some of the world’s cheapest data plans (Kaka et al. Reference Kaka, Madgavkar, Kshirsagar, Gupta, Manyika, Bahl and Gupta2019). Internet penetration in India increased exponentially over the past few years and Bihar—the sampling site for this study—saw an Internet connectivity growth of over 35% in 2018, the highest in the country (Mathur Reference Mathur2019).
In India, 81% of users now own or have access to smartphones and most of these users report obtaining information and news through their phones (Devlin and Johnson Reference Devlin and Johnson2019). Paradoxically, this leap in development coupled with the novelty and unfamiliarity with the Internet could make new users more vulnerable to information received online. The example of Geeta highlights this aspect. Geeta lives in Arrah, Bihar and recently bought a smartphone with Internet. I asked her if she thought information received over WhatsApp was factually accurate:
This object [her Redmi phone] is only the size of my palm but is powerful enough to light up my home. Previously we would have to walk to the corner shop with a TV for the news. Now when this tiny device shines brightly and tells me what is happening in a city thousands of kilometers away, I feel like God is directly communicating with me. [translated from Hindi]Footnote 1
Geeta’s example demonstrates that the novelty of digital media could increase vulnerability to all kinds of information. Survey data shows that countries like India have several “unconscious” users who are connected to the Internet without an awareness that they are going online (Silver and Smith Reference Silver and Smith2019). Such users may be unaware of what the Internet is in a variety of ways. The expansion of Internet access and smartphone availability in India thus generate the illusion of a mythic nature of social media, underscoring a belief that if something is on the Internet, it must be true.
Third, online information in developing countries is disproportionately consumed on encrypted chat-based applications such as WhatsApp. India is WhatsApp’s biggest market in the world (with about 400 million users in mid-2019), but an important reason contributing to the app’s popularity is also at the heart of the misinformation problem: WhatsApp messages are private and protected by encryption. This means that no one, including the app developers and owners themselves, have access to see, read, filter, and analyze text messages. This feature prevents surveillance by design, such that tracing the source or the extent of spread of a message is close to impossible, making WhatsApp akin to a black hole of misinformation. Critically, this means that top-down and platform-driven solutions are impractical in the case of private group chats on WhatsApp, suggesting that bottom-up interventions are more promising.
Finally, the format of misinformation in India is mainly visual: much of what goes viral on WhatsApp constitutes photo-shopped images and manufactured videos (Garimella and Eckles Reference Garimella and Eckles2020). Misinformation in graphical and visual form is found to have increased salience, capable of retaining respondent attention to a higher degree (Flynn, Nyhan, and Reifler Reference Flynn, Nyhan and Reifler2017). On WhatsApp, false stories are almost never shared with a link; images are forwarded as is to thousands of users, making the original source unknown and difficult to trace.
Research on WhatsApp in India finds that a large fraction of groups belong to political parties or causes supporting political parties (Garimella and Eckles Reference Garimella and Eckles2020). Despite this, evidence on the power of partisanship and ideology as polarizing social identities in India is mixed. India’s party system is not historically viewed as ideologically structured. Research finds that parties are not institutionalized (Chhibber, Jensenius, and Suryanarayan Reference Chhibber, Jensenius and Suryanarayan2014), elections are highly volatile (Heath Reference Heath2005), and the party system itself is not ideological (Chandra Reference Chandra2007; Kitschelt and Wilkinson Reference Kitschelt and Wilkinson2007; Ziegfeld Reference Ziegfeld2016). More recent literature, however, argues for the idea that Indians are reasonably well sorted ideologically into parties and that politics might be becoming more programmatic among certain groups (Chhibber and Verma Reference Chhibber and Verma2018; Thachil Reference Thachil2014). Despite this, we know little about the origins of partisanship in India—whether it stems from transactional relationships with parties, affect for leaders, ties to social groups, ideological leanings—or its stability.
Despite these findings, I argue that party identities will likely moderate attitudes in India. This is largely because of the nature of the BJP’s appeals. The recent BJP administration under the leadership of Prime Minister Narendra Modi represents a departure from traditional models of voting behavior in India, highlighting that Modi’s rule is a form of personal politics in which voters prefer to centralize political power in a strong leader and trust the leader to make good decisions for the polity (Sircar Reference Sircar2020). Some have concluded that under Modi, polarization in India is more toxic than it has been in decades, showing no signs of abating (Sahoo Reference Sahoo2020). To add to this, misinformation is India is inherently political in nature, with disinformation campaigns often stemming from party sources themselves (Singh Reference Singh2019). Finally, partisan identities tend to be more salient during elections, when citizen attachments to parties are heightened (Michelitch and Utych Reference Michelitch and Utych2018). Taken together, these factors indicate that BJP partisans are more likely to respond expressively to the partisan treatment and engage in motivated reasoning in the face of counterattitudinal information.
Media Literacy Intervention
I designed a pedagogical, in-person media literacy treatment with educational tools to address misinformation in the Indian context.
The concept of media literacy captures the skills and competencies that promote critical engagement with messages produced by the media, needed to successfully navigate a complex information ecosystem (Jones-Jang, Mortensen, and Liu Reference Jones-Jang, Mortensen and Liu2019). Research finds that media literacy can bolster skepticism toward false and misleading information, making it particularly suitable to address the spread of misinformation (Kahne and Bowyer Reference Kahne and Bowyer2017). Experimental studies promoting media literacy initiatives against misinformation operationalize media literacy by increasing the salience of critical thinking (Vraga, Bode, and Tully Reference Vraga, Bode and Tully2020), gauging respondent knowledge about media industries and systems (Vraga and Tully Reference Vraga and Tully2021), or going a step further by providing tips to spot misinformation (Guess et al. Reference Guess, Lerner, Lyons, Montgomery, Nyhan, Reifler and Sircar2020).
But simply nudging respondents to be more critical consumers or providing tips asking them to be more aware may be insufficient to help counter misinformation in contexts where respondents are not armed with the tools to apply such advice to the information they encounter. These tools can be thought of as a set of instructions or concrete steps that can be used to spot or correct misinformation.
In contexts such as India where respondents may be unconscious Internet users who are unaware of misinformation, tips (such as accuracy nudges) may not be sufficient to change behavior. Such nudges might prime the concept of accuracy, but if respondents do not know how to apply the information in those nudges, in spite of having the desire to, they may fall short in reaching their accuracy goals.
Experimental Design
The intervention for the treatment group respondents was, by design, a bundled treatment incorporating several elements, drawing on research demonstrating that the most promising tools to fight misinformation are fact-checking combined with media literacy (Hameleers Reference Hameleers2020). The intervention consisted of surveying a respondent in their home and undertaking the following activities in a 45–60 minute visit:
-
1. Pretreatment survey: Field enumerators administered survey modules to measure demographic and pretreatment covariates including digital literacy, political knowledge, media trust, and prior beliefs about misinformation.Footnote 2
-
2. Pedagogical intervention: Next, respondents went through a learning module to help inoculate against misinformation. This included an hour-long discussion on encouraging people to verify information along with concrete tools to do so:
-
• Performing reverse image searches: A large part of misinformation in India comprises misleading photos and videos, often drawn from one context and used to spread misinformation about another context or time. Reverse searching such images is an easy way identify their origins. As one focus group discussion conducted before the experiment revealed: “the time stamp on the photo helped me realize that it is not current news; if this image has existed since 2009, it cannot be about the 2019 election.”Footnote 3 Respondents can see the original source and time stamp on an image once it is fed back into Google, making this technique a uniquely useful and compelling tool given the nature of visual misinformation in India. The enumerators discussed this tool with respondents.
-
• Navigating a fact-checking site: Focus group discussions also revealed that while a minority of those surveyed knew about the existence of fact-checking websites in India, even fewer were able to name one. The second concrete tool involved demonstrating to respondents how to navigate a fact-checking website, www.altnews.in, an independent fact-checking service in India.
-
-
3. Corrections and tips flyer: Enumerators next helped respondents apply these tools to fact-check four false stories. To do so enumerators displayed a flyer to respondents, the front side of which had descriptions of four recent viral political false stories. For each story, enumerators systematically corrected the false story, explaining in each case why the story was untrue, what the correct version was, and what tools were used to determine veracity. The back side of the flyer contained six tips to reduce the spread of misinformation. The enumerator read and explained each tip to respondents, gave them a copy of the flyer, and exhorted them to make use of it.
These tools were demonstrated to treatment group respondents only. Control group respondents were shown a placebo demonstration about plastic pollution and were given a flyer containing tips to reduce plastic usage.
-
4. Comprehension Check: Last, the enumerators administered a comprehension check to measure whether the treatment was effective in the short term.
For this study, the respondents were randomized into one of three groups, two treatment and one placebo control. Table 1 summarizes the three groups.
Table 1. Experimental Treatments

Respondents in both treatment groups received the pedagogical intervention. However, one group received corrections to four pro-BJP false stories and the other received corrections to four anti-BJP false stories. Besides differences in the stories that were fact-checked, the tips on the flyer remained the same for both treatment groups. Respondents in the placebo control group received a symmetric treatment where enumerators spoke about plastic pollution and were given a flyer on tips to reduce plastic usage. The false stories included in the treatment group flyers were drawn from a pool of stories that had been fact-checked for accuracy by altnews.in and boomlive.in. The partisan slant of each story was determined by a Mechanical Turk pretest. To ensure balance across both treatment groups, stories with similar salience and subject matter were picked. The back of treatment flyers contained the same tips on how to verify information and spot false stories. The entire intervention was administered in Hindi. Figures C.1, C.2, and C.3 present the English-translated version of flyers distributed to respondents.
To control for potential imbalance in the sample, a randomized block design was used. The respondents who identified with the BJP were one block, and those who identified with any other party were another block. Within each block, the respondents were randomly assigned to one of the three experimental groups described in Table 1. This design ensured that each treatment condition had an equal proportion of BJP and non-BJP partisans. Overall, the sample was equally divided between the two treatment and placebo control groups (i.e., one third of the sample in each of the three groups).
Sample and Timeline
The sample was drawn from the city of Gaya in the state of Bihar in India. Bihar has both the lowest literacy rate in the country and the highest rural penetration of mobile phones, making it a strong test-case for the intervention.
Respondents were selected through a random walk procedure. Within the sampling area, a random sample of polling booths (smallest administrative units) were selected to serve as enumeration areas. Within each enumeration area, enumerators were instructed to survey 10–12 households following a random walk procedure. This method was chosen over traditional survey-listing techniques to minimize enumerators’ time spent in the field during the elections and because of a lack of accurate census data for listing (Lupu and Michelitch Reference Lupu and Michelitch2018). Each field enumerator was assigned to only one polling booth, so the paths taken during each selection crossed each household only once, increasing the likelihood of a random and unbiased sample.
Once a household was selected, respondents could qualify for the study based on three preconditions designed to maximize familiarity with the Internet: respondents were required to have their own cellphone (i.e., not a shared household phone), working Internet for six months prior to the survey, and WhatsApp downloaded on the phone. If multiple members of a household qualified based on the preconditions, a randomly selected adult member was requested to participate in the study.
Of note, only 20% of all households sampled had respondents who met the criteria for recruitment into the study. In Bihar, where only 20–30% of citizens have access to the Internet, this is unsurprising. Despite this, the study had a high response rate: of all those who were eligible for the study, 94.5% agreed to participate. The final sample included 1,224 respondents.Footnote 4
Trained enumerators administered the intervention in a household visit that was rolled out in May 2019. Approximately two weeks after the intervention, the same respondents were revisited to conduct an endline survey and measure the outcomes of interest. Critically, the respondents voted in the election between the two enumerator visits. Figure 2 summarizes the timeline for this study.

Figure 2. Experimental Timeline (May 2019)
The study included multiple steps in survey design and implementation to minimize exogenous shocks from election results. The timeline ensured that though respondents voted in the general election after the intervention, making voter turnout posttreatment, the endline survey to measure outcomes was conducted before election votes were counted and results were announced.Footnote 5 This timeline had the double advantage of ensuring that the outcome measures were not affected by the exogenous shock of the election results while also ensuring that the respondents received the intervention before they voted, when political misinformation was arguably at its peak. At the end of the baseline survey, enumerators collected addresses and mobile numbers of respondents for subsequent rounds of the study and then immediately separated this contact information from the main body of the survey to maintain the respondents’ privacy.
Dependent Variables
In the endline survey, the enumerators revisited respondents after they had voted. The same set of enumerators administered the intervention and the endline survey. However, the enumerators were given a random set of household addresses for the endline survey to minimize the possibility of the same enumerator systematically interviewing the same respondent twice. Further, addresses and contact information were separated immediately from baseline survey data to ensure that enumerators only had contact information about respondents. During the baseline survey, 1,309 respondents were administered the intervention. The enumerators successfully located 1,224 of these respondents, resulting in an attrition rate of 6.5%. Importantly, nobody who was administered the intervention refused to answer the endline survey; the attrited group comprised only respondents who enumerators were unable to contact at home after three attempts.
The key outcome of interest is whether the intervention positively affected respondents’ ability to identify misinformation. To this end, respondents were shown a series of 14 news stories.Footnote 6 These stories varied in content, salience, and critically, partisan slant. Half of the stories were pro-BJP in nature and the other half anti-BJP.Footnote 7 Each respondent saw all the 14 stories, but the order in which they were shown was randomized.Footnote 8 A list of the 14 stories shown to respondents is presented in Table D.1. Following each story, the key primary dependent variable measured perceived accuracy of stories, with the following question:Footnote 9
“Do you believe this news story is false?” (binary response, 1 if yes, 0 otherwise).
Hypotheses and Estimation
I hypothesize there will be a positive effect of the intervention for respondents assigned to any arm of the treatment group relative to the placebo control. I also hypothesize that the individual effect of being assigned to each treatment will be positive relative to the placebo control:
Hypothesis 1: Exposure to the media literacy intervention will increase ability to identify misinformation relative to the control.
Hypothesis 2a: Exposure to media literacy and pro-BJP corrections will increase ability to identify misinformation.
Hypothesis 2b: Exposure to media literacy and anti-BJP corrections will increase ability to identify misinformation.
I estimate the following equations to test the main effect of the intervention:


In the equations, i represents the respondent, the Intervention variable in Equation 1 represents pooled assignment to the media literacy intervention (relative to control). In Equation 2, the dependent variable is regressed on separate indicators for having received the intervention and pro-BJP corrections or the intervention and anti-BJP corrections, with the control condition as the omitted category. The dependent variable MisinformationId counts the number of stories accurately identified as true or false. MisinformationId has been coded such that a positive estimated
$ {\beta}_1 $
indicates an increase in discernment ability.
Beyond the average treatment effect, I expect treatment effects to differ conditional on a single factor previously identified in the literature as a significant predictor of information consumption: partisan identity. In line with the literature on partisan-motivated reasoning (Nyhan and Reifler Reference Nyhan and Reifler2010), I expect that the treatment effect will be larger for politically incongruent information than for politically congruent information, relative to the control condition. A politically congruent condition manifests when corrections are pro-attitudinal—that is, BJP partisans receiving corrections to anti-BJP false stories, or non-BJP partisans receiving corrections to pro-BJP false stories.
Hypothesis 3: Effectiveness of the intervention will be higher for politically incongruent information than for politically congruent information, relative to the control condition.
To determine whether partisan identity moderates treatment effects, I test Hypothesis 3 with the following model:

In Equation 3, PartyID is an indicator variable that takes on the value of 1 if the respondent self-identified as a BJP supporter. The choice to code party identity as dichotomous was based on the nature of misinformation in India where false stories are perceived as either favoring or not favoring the BJP. A positive coefficient estimate for
$ {\beta}_2 $
indicates an increase in discernment ability among BJP partisans due to the treatment.
However, while partisanship might moderate attitudes, the role of other theoretical moderators such as age is unclear in the Indian context. Owing to the lack of priors in the Indian context, I do not form preregistered hypotheses about demographic moderators. Instead, I examine their relationships with misinformation through the following exploratory research questions:
RQ1: What is the relationship between age and vulnerability to misinformation? Does this relationship change as a function of the treatment?
RQ2: Does age correlate negatively with digital literacy, as in the American context? Are more digitally literate respondents likely to learn better from the treatment?
RQ3: What is the relationship between political sophistication (measured both by education and political knowledge) and vulnerability to misinformation?
Data and Results
This section begins with descriptive analyses that demonstrate the extent of belief in misinformation as well as partisan polarization in this belief.Footnote 10
Figure 3 lists the 12 false stories used in the dependent variable measure in this study. This figure plots the share of respondents in the sample who believed each story to be true. Two aspects of the figure are striking. First, general belief in misinformation is low. For half of the 12 false stories, less than 10% of the sample thought they were true. Second, belief in pro-BJP misinformation appears to be stronger, possibly alluding to its increased salience (Jerit and Barabas Reference Jerit and Barabas2012), frequency of appearance on social media (Garimella and Eckles Reference Garimella and Eckles2020), or the presence of a higher proportion of BJP supporters in the sample. Overall, across the 12 false stories, respondents correctly classified an average of 9.91 stories.

Figure 3. Percentage of Sample Who Believes Rumors
Figure 4 plots respondent belief in stories by partisan identity. For 10 out of the 12 partisan stories, we see a correspondence between respondent party identity and pretested political slant of the story. Though there is partisan sorting on belief in political rumors, the gap between BJP and non-BJP partisans in their beliefs is not as large as in the American case: the biggest gap appears in the case of the Unclean Ganga river rumor, where non-BJP partisans showed about 9 percentage points more belief in the rumor relative to BJP supporters. In contrast, Jardina and Traugott (Reference Jardina and Traugott2019) demonstrate that differences between Democrats and Republicans in their belief of the Obama birther rumor can be as large as 80 percentage points.

Figure 4. Belief in Rumors by Respondents’ Party ID
To identify differences between subpopulations in vulnerability to misinformation, I analyze the correlates of misinformation among control group respondents (N = 406). This analysis provides the baseline rate of identification ability in the absence of the intervention. In the regression analysis in Table 2, the dependent variable is the number of stories accurately classified as true or false by control group respondents.
Table 2. Misinformation Identification in Control Group

Note: *p < 0.05; **p < 0.01; ***p < 0.001.
First, we observe that BJP supporters were significantly better at discernment between true and false stories. This observational result is striking—on the one hand, pro-BJP rumors are more likely to be believed by respondents, in line with descriptions of a right-wing advantage in producing misinformation (supply side). However, demand-side results demonstrate that BJP supporters are better at identifying misinformation.
This finding concurs with observations that incentives to spread partisan misinformation has led parties like the BJP to form “cyberarmies” to disseminate information. Thus, while it is possible that BJP respondents are more aware of party-driven supply of misinformation, thereby being able to identify rumors at greater rates, their partisanship also makes them expressively believe pro-attitudinal rumors. These observational findings suggest the presence of partisan-motivated reasoning in the Indian context.
Parsing this result further, it appears that BJP supporters’ better discernment is driven by their ability to identify anti-BJP stories as fake. I find that while there is no difference in discernment of pro-BJP stories between BJP and non-BJP partisans, the true difference in means is significantly greater than 0 for anti-BJP stories, with BJP respondents correctly identifying these stories at a higher rate. Thus the finding that BJP respondents identify stories at a higher rate is driven only by their identification of anti-BJP messages as false.Footnote 11
Next, we observe interesting findings with respect to age and digital literacy. While findings on misinformation in the United States suggest that older adults are most likely to engage with fake sources (Grinberg et al. Reference Grinberg, Joseph, Friedland, Swire-Thompson and Lazer2019), this data demonstrates that older age is associated with better discernment between true and false stories, highlighting that the vulnerability of older adults to misinformation differs dependent on whether the outcome of interest is sharing behavior or perceived accuracy. The result that older adults are better at discernment may be attributed to findings that older adults may strategically decide to share information despite their beliefs, and that their vast knowledge base might help information processing despite cognitive declines (Brashier and Schacter Reference Brashier and Schacter2020).
Further, I find that increases in digital literacy are associated with lower discernment, contrary to findings in the American context that people who are less digitally literate are more likely to fall for misinformation and clickbait (Munger et al. Reference Munger, Luca, Nagler and Tucker2018). Finally, I find that while political knowledge is not correlated with misinformation identification, education is associated with significant increases in discernment.Footnote 12
I now move to discussing experimental results. I estimate effects of the treatment on outcomes in a between-subjects design. All estimates are ordinary least square (OLS) regressions, and empirical models are specified relying on random treatment assignment to control for potential confounders. First, I analyze data for the main effect of the intervention. While research predicts that in-person and field interventions on media effects are likely to have stronger effects (Flynn, Nyhan, and Reifler Reference Flynn, Nyhan and Reifler2017; Jerit, Barabas, and Clifford Reference Jerit, Barabas and Clifford2013), my findings from misinformation-prone India are less encouraging. Even with an in-person intervention, where enumerators spend close to one hour with each respondent to debunk and discuss misinformation and where respondents understood the intervention, I do not see significant increases in the ability to identify misinformation as a function of teaching respondents media literacy tools.
Results are shown in Table 3. The key dependent variable is the number of stories that a respondent correctly classified as true or false. To estimate the pooled effect of the intervention, I construct a variable that takes on the value of 1 if a respondent received any literacy and fact-checking treatment (relative to 0 if the respondent was in the placebo control group). The effect of this pooled treatment is estimated in model 1. In model 2, I split the treatment into the pro-BJP corrections and the anti-BJP corrections (note both treatment conditions receive the same literacy intervention).
Table 3. Effect of Treatment on Ability to Identify Misinformation

Note: *p < 0.05; **p < 0.01; ***p < 0.001.
Table 3 demonstrates that the intervention did not increase misinformation identification ability on average. Splitting the treatment into its component parts (each compared with the placebo control) yields similar results. I find no evidence that an hour-long pedagogical intervention increased ability to identify misinformation among respondents in Bihar, India. The ability to update one’s priors in response to factual information is privately and socially valuable, so the fact that a strong, in-person treatment does not change opinions demonstrates the resilience of misinformation in India. Priors about misinformation in this context appear resistant to change, but, as I demonstrate below, this does not preclude moderating effects of partisan identity.
I now turn to the analysis of heterogeneous effects of partisan identity. Results are striking: I find that the interaction effect of the treatment on BJP partisans produces a negative effect on the ability to identify misinformation (Table 4).
Table 4. Effect of Treatment x Party (Discernment Measure)

Note: *p < 0.05; **p < 0.01; ***p < 0.001.
This indicates that the treatment made BJP supporters worse at identifying misinformation. However, since the main dependent variables pools together all stories—true and false, pro-BJP and not—I now examine whether the negative coefficient on the interaction term obtains for pro-attitudinal and counterattitudinal stories separately. In Table 5, I limit the dependent variable to the set of false stories. In Column 1, I estimate the effect of receiving the treatment for BJP supporters on ability to identify pro-BJP false stories; Column 2 does the same with anti-BJP false stories. The treatment variable for both models pools across receiving any treatment relative to control.
Table 5. Effect of Treatment x Party (False Stories)
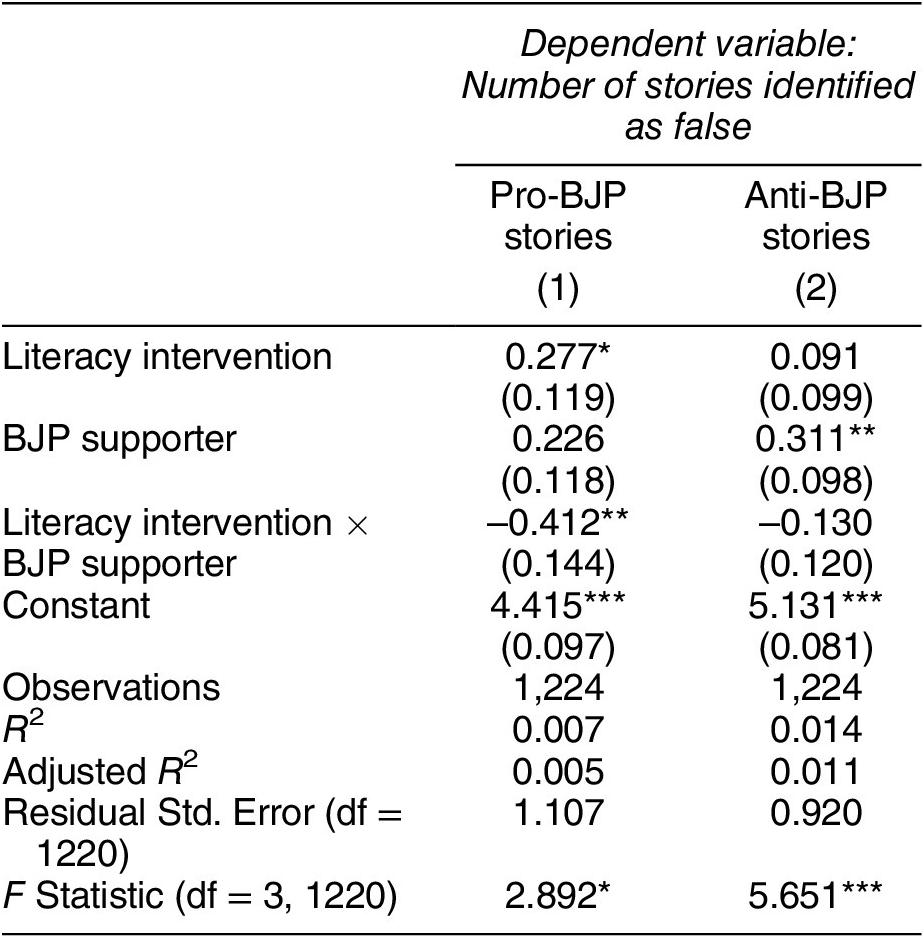
Note: *p < 0.05; **p < 0.01; ***p < 0.001.
The results show that while there was no average treatment affect, the interaction effect of the treatment on BJP partisans produces a negative effect on the ability to identify misinformation. For pro-BJP stories, the treatment effect for non-BJP supporters was 0.277, indicating that those who did not support the BJP and received the treatment identified an additional 0.277 stories. However, the treatment effect for BJP supporters was -0.135, indicating that those who supported the BJP and received the treatment identified 0.135 fewer stories.
Visualizing this interaction effect in Figure 5, where I graph the predicted values from the interaction model in Equation 3, it appears that the treatment had contradictory effects conditional on party identity (for the set of pro-BJP stories). The intercept for BJP partisans is higher, demonstrating better identification skills ex ante in the absence of the treatment. However, treatment group respondents who identify as BJP partisans show a significant decrease in their ability to identify false stories, while treatment group respondents who do not identify as BJP partisans show an increase in their ability to identify false stories. Thus, the treatment was successful with non-BJP partisans and backfired for BJP partisans. Importantly, these effects obtain only for the set of false stories that is pro-BJP in slant (implying that their corrections could be perceived as pro-attitudinal for non-BJP partisans). In Figure 6, I graph the interaction for the set of dependent variable stories that are anti-BJP in slant. While the relationships in this graph are directionally similar, they are smaller in magnitude and not significant. Importantly, fact-checking is much more effective for anti-BJP stories than for pro-BJP stories (note that the effects are much larger). Pro-BJP stories are more likely to be identified as false in the control, but the treatment is weaker for this subset of stories. Taken together, these results imply that non-BJP respondents were able to successfully apply the treatment to identify pro-attitudinal corrections. But for BJP partisans, given that these corrections are not consistent with their partisan identity, the treatment backfires. Finding that higher levels of identification (in the control group for BJP respondents) were made worse as a function of the treatment demonstrates the existence of partisan-motivated reasoning in the Indian context. I examine this result further in the Discussion.

Figure 5. Predicted Identification of Pro-BJP Stories

Figure 6. Predicted Identification of Anti-BJP Stories
Moving beyond experimental results, I find that younger adults in the sample are less likely to be able to identify misinformation and that higher levels of digital literacy are associated with greater vulnerability to misinformation, contrary to findings in the United States (Grinberg et al. Reference Grinberg, Joseph, Friedland, Swire-Thompson and Lazer2019; Munger et al. Reference Munger, Luca, Nagler and Tucker2018). I also find that while political knowledge does not correlate with perceptions of stories, more educated respondents are better at spotting false stories. I explore these associations in Online Appendices F and G.
Discussion
The most striking finding to emerge from this study demonstrates that the intervention improved misinformation identification skills for one set of respondents (non-BJP respondents) but not another (BJP partisans). Paralleling results seen in developed contexts, the perceptual screen (Campbell et al. Reference Campbell, Converse, Miller and Stokes1960) of BJP partisanship shaped how respondents interacted with this treatment, with BJP partisans demonstrating a tendency to cheerlead for their party and discredit pro-party stories despite their being false (Gerber and Huber Reference Gerber and Huber2009; Prior, Sood, and Khanna Reference Prior, Sood and Khanna2015). These findings of motivated reasoning demonstrate that citizen attachments to political parties are heightened during elections (Michelitch and Utych Reference Michelitch and Utych2018) and that strong partisans engage in strategic ignorance, pushing away information and facts that get in the way of feelings (McGoey Reference McGoey2012).
This finding is also surprising, given that there is little evidence of such backfire effects in the American context (Wood and Porter Reference Wood and Porter2019). However, several other associations in the American context do not hold in this data: I find a positive correlation between increasing age and ability to discern true from false stories, a negative correlation between increasing digital literacy and discernment, and no association with political knowledge.Footnote 13 The nature of these findings underscores that what we know about misinformation comes largely from Western contexts and may not easily apply to other settings. It highlights that we need more theorizing and more data from non-Western contexts. Thus, while I do find some backfire effects in the data, more needs to be done to establish the robustness of these findings. Future work should examine treatments such as this one in nonelectoral contexts where the salience of partisanship may be lower, resulting in smaller differences between parties. Nevertheless, my findings suggest that even in democracies with weaker partisan identification, citizens still engage in motivated reasoning. This has important implications beyond the study of fact-checking and extends more broadly to how Indian citizens make political judgements.
It is important to underscore that the intervention worsened misinformation identification only for the pro-BJP set of false stories. This effect does not appear for anti-BJP false stories. This highlights key differences in partisan identities in this data. First, though traditionally India has been described as a nonideological system, the recent years under the Modi-led BJP governments have led some to conclude that tribalism and psychological attachments to political parties (Westwood et al. Reference Westwood, Iyengar, Walgrave, Leonisio, Miller and Strijbis2018) are more salient now than ever before (Sircar Reference Sircar2020). Importantly, such partisan attachments seem to have arisen in response to the personal popularity of Narendra Modi, with no comparable cult of personality on the political left. Thus it stands to reason that partisanship is stronger for BJP supporters. Second, the way the party identity variable is operationalized in the data further emphasizes this point. I cluster BJP supporters into one block and non-BJP supporters into another, but the non-BJP block is a heterogeneous group of respondents from several different parties. Thus we should expect that citizen attachments to political stories, true and false, will be perceived very differently for both political blocks. Third, political disinformation campaigns in India seem to emanate largely from the right-wing. This is underscored in my data by pro-BJP stories being believed to a much greater extent than anti-BJP stories, alluding to the fact that pro-BJP stories are more salient in the minds of respondents (Figure 3).
As a consequence of these factors, there is an inherent lack of symmetry between the two sets of stories that comprise the dependent variable measure. Pro-BJP stories are more salient and believed to a greater extent, so there is likely more room for the treatment to move attitudes on the stories (as it does, for non-BJP supporters). On the contrary, the majority of anti-BJP stories were believed by less than 10% of the sample; this high ceiling might make it difficult for the treatment to work for anti-BJP stories.
Conclusion
Misinformation campaigns have the capacity to affect opinions and elections across the world. In this paper, I present new evidence on belief in popular misinformation stories in India in the context of the 2019 general elections. I design a pedagogical intervention to foster bottom-up skills training to identify misinformation. Using tools specifically designed for the Indian context such as reverse image searches, I administer in-person skills training to 1,224 respondents in Bihar, India in a field experiment. I find that this grassroots-level pedagogical intervention has little effect on the respondents’ ability to identify misinformation on average. But, the partisanship and polarization of BJP supporters appears stickier than that of their out-partisans. Non-BJP supporters in the sample receive the treatment and apply it to identify misinformation at a higher level, demonstrating that cognitive skills can be improved as a function of the treatment. However, for BJP partisans, receiving the treatment leads to a significant decrease in identification ability, but only for pro-attitudinal stories.
The presence of motivated reasoning is a surprising result in a country with traditionally weak party ties and nonideological party systems. Democratic citizens have a stake in dispelling rumors and falsehoods, but in societies with polarized social groups, individuals also have a stake in maintaining their personal standing in social groups that matter to them (Kahan et al. Reference Kahan, Peters, Dawson and Slovic2017). The finding that the intervention worked on a subset of respondents underscores the fact that the training was not strong enough to overcome the effects of group identity for BJP respondents. Theoretically, this result is similar to research that finds that identity-protective cognition, a type of motivated reasoning, increases pressure to form group-congruent beliefs and steers individuals away from beliefs that could alienate them from others to which they are similar (Giner-Sorolla and Chaiken Reference Giner-Sorolla and Chaiken1997; Sherman and Cohen Reference Sherman and Cohen2006). Practically, the result calls for a revision of findings on party identity in India, as it demonstrates the presence of motivated reasoning in electoral settings.
Despite these findings on partisanship, I consider some reasons for the null average treatment effect and some limitations of the study and future avenues for research. First, the two-week gap between the intervention and the measurement of outcomes is atypical for studies of this kind, where dependent variables are measured in close proximity to treatments. Thus, it is possible that a first-stage effect decayed over time such that it was not captured by the study. But while this design does not measure immediate effects, its results suggest that such treatments may have limited long-term durability.
Further, it is worth noting that this was an explicitly political intervention. Recent research demonstrates that news literacy messages paired with a political talk show host advancing an incongruent political argument increases the odds that people will rate the literacy message as biased (Tully and Vraga Reference Tully and Vraga2017). Thus, the political nature of the treatment itself likely activated motivated reasoning (Groenendyk and Krupnikov Reference Groenendyk and Krupnikov2021).
Next, the timing of the intervention during a contentious election meant not only that partisan identities were more salient (Michelitch and Utych Reference Michelitch and Utych2018) but also that the presence of several election officials, campaigning party workers, and GOTV efforts meant that respondents in the area had their door knocked on several times a day by different interest groups. Thus, it is possible that the marginal effect of an additional house visit by the enumeration team for this study made the in-person intervention less salient.
Additionally, the design oversampled false news stories in the outcome measure.Footnote 14 While this was done to maximize belief reduction in false stories with perilous consequences, future research should systematically change the balance of true and false stories to study how this factor shapes the efficacy of these types of campaigns.
Finally, while the outcome measured the perceived accuracy of news stories, it did not measure whether the participants used fact-checking tools between the intervention and the follow up. Future studies of this nature would benefit from tests that allow the participants to directly apply the tools learned in the intervention at the moment of identification of false news stories. This would allow us to measure a number of intermediary steps including whether participants recalled the training and chose to apply it to identify misinformation. Thus, a valuable prospect for future work would be to validate the usage and frequency of procedural tools before measuring beliefs.
But providing educative tools alone may be insufficient to help move attitudes, as media literacy is distinct from its application (Vraga, Bode, and Tully Reference Vraga, Bode and Tully2020). Several additions to such a design could be undertaken to ensure respondents not only are given tools but also are motivated to apply them. As Tully, Vraga, and Bode (Reference Tully, Vraga and Bode2020) note, the one-shot nature of such interventions was may be insufficient to change behavior in a fast-paced and overwhelming social media landscape. On social media, news literacy messages have to compete with many other pieces of information, and any one piece of information may get lost in the crowd. This may also be a factor in my study, as even though the intervention was fairly detailed, it was just a single training session, and multiple in-person visits might be needed to reinforce these skills and provide respondents with the opportunity to practice them. One such strategy could be to make misinformation and digital literacy training a part of school curriculums. Further, highlighting social responsibility might motivate citizens to use the tools at their disposal. Vraga, Bode, and Tully (Reference Vraga, Bode and Tully2020) suggest that a more citizen-oriented framing might persuade people relative to a more personal framing, as it connects information to democratic duties. Similarly, Mullinix (Reference Mullinix2018) shows that heightening a sense of civic duty (i.e., citizens have an obligation to get the facts right) reduces partisan-motivated reasoning, and a similar logic could apply here. Thus, a related corollary would be to investigate whether accuracy motivations could also improve the applicability of these tools.
The findings from this study indicate local average treatment effects, dependent heavily on the locality where this experiment was conducted: the low-education, low-internet environment of semiurban Bihar at a time when politics was salient and political misinformation was rife. New internet users, such as those in this sample, not only face greater barriers in learning new technology, but the uptake of interventions designed to combat misinformation might be harder to implement with samples that are more rural and as a result have mobile-only and less-stable internet connections. My results, along with those from similar studies (Guess et al. Reference Guess, Lerner, Lyons, Montgomery, Nyhan, Reifler and Sircar2020) suggest that different techniques will be needed to reach diverse populations in the developing world. For better-educated and more digitally literate populations, simpler tools may prove to be effective. But for new internet users, richer interventions that provide not only tools but also boosts to motivation may be necessary.
Thus, whether these findings would hold–or change–outside of this sample remains an open empirical question. Consequently, I caution about interpreting these null results to mean that interventions of this kind do not work, as thorough future work must look into replicating such a design in different contexts and times. Thus, while this study was necessarily context-dependent, it is nevertheless an important first step towards tempering the human cost of misinformation in India.
Supplementary Materials
To view supplementary material for this article, please visit http://dx.doi.org/10.1017/S0003055421000459.
DATA AVAILABILITY STATEMENT
Research documentation and data that support the findings of this study are openly available at the APSR Dataverse: https://doi.org/10.7910/DVN/ITKNX5.
ACKNOWLEDGMENTS
This study was preregistered with Evidence in Governance and Policy and received IRB approval from the University of Pennsylvania (832833). For comments and feedback on this study, the author thanks Guy Grossman, Matthew Levendusky, Marc Meredith, Devesh Kapur, Neelanjan Sircar, Brendan Nyhan, Tariq Thachil, Douglas Ahler, Milan Vaishnav, Adam Ziegfeld, Devra Moehler, Jeremy Springman, Emmerich Davies, Simon Chauchard, Simone Dietrich, and the anonymous referees. Pranav Chaudhary and Sunai Consultancy provided excellent on-ground and implementation assistance. This research was funded by the Center for the Advanced Study of India (CASI) at the University of Pennsylvania and the Judith Rodin Fellowship. The author also thanks seminar participants at the NYU Experimental Social Sciences Conference, MIT GOV/LAB Political Behavior of Development Conference, Penn Development and Research Initiative, the Harvard Experimental Political Science Conference, APSA 2020, SPSA 2020, and the UCLA Politics of Order and Development lab.
FUNDING STATEMENT
This research was funded by the Center for the Advanced Study of India (CASI) and the Judith Rodin Fellowship, both at the University of Pennsylvania.
CONFLICTS OF INTEREST
The author declares no ethical issues or conflicts of interest in this research.
ETHICAL STANDARDS
The author declares that the human subjects research in this article was reviewed and approved by the Institutional Review Board at the University of Pennsylvania and certificate numbers are provided in the text. The author affirms that this article adheres to the APSA’s Principles and Guidance on Human Subject Research.
Comments
No Comments have been published for this article.