Introduction
Mood dysregulation is present in diverse forms of mental illness and severe dysregulation occurs in 1–3.5% of children and adolescents (Hudziak et al. Reference Hudziak, Althoff, Derks, Faraone and Boomsma2005; Althoff et al. Reference Althoff, Verhulst, Rettew, Hudziak and van der Ende2010). It can be described as a dimensional trait in child development in terms of irritability (Wiggins et al. Reference Wiggins, Mitchell, Stringaris and Leibenluft2014). Furthermore, it is a common phenomenon in many psychiatric disorders like attention-deficit/hyperactivity disorder (ADHD), oppositional defiant disorder (ODD) (Angold et al. Reference Angold, Costello and Erkanli1999), substance use disorders and suicidality (Holtmann et al. Reference Holtmann, Buchmann, Esser, Schmidt, Banaschewski and Laucht2011b ). The presence of mood dysregulation in childhood was associated with increased rates of adult anxiety disorders, mood disorders and disruptive behaviour (Althoff et al. Reference Althoff, Verhulst, Rettew, Hudziak and van der Ende2010).
However, mood dysregulation is a heterogeneous dimensional construct that has been defined and measured in several ways. Closely related terms used in recently published studies are: ‘ Deficient emotional self-regulation ’ measured by an aggregate cut-off score of >180 but <210 on the Anxiety/Depression, Aggression and Attention scales of the Child Behavior Checklist (CBCL) (Spencer et al. Reference Spencer, Faraone, Surman, Petty, Clarke, Batchelder, Wozniak and Biederman2011; Biederman et al. Reference Biederman, Petty, Day, Goldin, Spencer, Faraone, Surman and Wozniak2012). ‘ Mood dysregulation ’ measured by the ARI (Affective Reactivity Index) (Stringaris et al. Reference Stringaris, Goodman, Ferdinando, Razdan, Muhrer, Leibenluft and Brotman2012). This scale contains six symptom items and one impairment item about irritability. Stringaris (Reference Stringaris2011) chose the items based on a broad definition of irritability as a mood of easy annoyance and touchiness characterised by anger and temper outbursts. ‘ Severe irritability ’ measured by 3 items from the CBCL/6–18 version: ‘temper tantrums’, ‘stubborn, sullen or irritable’, ‘sudden changes in mood’ (Leibenluft & Stoddard, Reference Leibenluft and Stoddard2013; Wiggins et al. Reference Wiggins, Mitchell, Stringaris and Leibenluft2014). ‘ Emotional lability ’ measured by parent rated Conner's 10-item-scale under consideration of four items: ‘easily frustrated’, ‘cries easily’, ‘mood changes quickly and drastically’, ‘temper outbursts and unpredictable behaviour’ (Banaschewski et al. Reference Banaschewski, Jennen-Steinmetz, Brandeis, Buitelaar, Kuntsi, Poustka, Sergeant, Sonuga-Barke, Frazier-Wood, Albrecht, Chen, Uebel, Schlotz, van der Meere, Gill, Manor, Miranda, Mulas, Oades, Roeyers, Rothenberger, Steinhausen, Faraone and Asherson2012; Merwood et al. Reference Merwood, Chen, Rijsdijk, Skirrow, Larsson, Thapar, Kuntsi and Asherson2014). As mentioned above behavioural phenomena described under these terms are similar, and thus allow a rough comparison between studies.
A broader phenotype of dysregulation, the ‘Dysregulation Profile’ (DP), firstly measured as the Child Behavior Checklist-Dysregulation Profile (CBCL-DP), has been investigated in epidemiological and clinical studies for the last two decades (Biederman et al. Reference Biederman, Wozniak, Kiely, Ablon, Faraone, Mick, Mundy and Kraus1995; Holtmann et al. Reference Holtmann, Bölte, Goth, Döpfner, Plück, Huss, Fegert, Lehmkul, Schmeck and Postka2007; Ayer et al. Reference Ayer, Althoff, Ivanova, Rettew and Waxier2009; Althoff et al. Reference Althoff, Verhulst, Rettew, Hudziak and van der Ende2010; Holtmann et al. Reference Holtmann, Buchmann, Esser, Schmidt, Banaschewski and Laucht2011b ; Althoff et al. Reference Althoff, Ayer, Crehan, Rettew, Baer and Hudziak2012; Maoz et al. Reference Maoz, Goldstein, Axelson, Goldstein, Fan, Hickey, Monk, Sakolsky, Diler, Brent, Iyengar, Kupfer and Birmaher2014; Geeraerts et al. Reference Geeraerts, Deutz, Deković, Bunte, Schoemaker, Espy, Prinzie, van Baar and Matthys2015; Deutz et al. Reference Deutz, Geeraerts, van Baar, Deković and Prinzie2016). Its score is based on the following scales of the Child Behavior Check List (CBCL): aggressive behaviour, anxious/depressive and attention problems (AAA-scales). The DP can also be derived from the Strengths and Difficulties Questionnaire (SDQ). The SDQ-dysregulation profile (SDQ-DP) has been validated to screen the DP in a clinical setting. It was characterised by the five SDQ-DP items ‘restless’ (2), ‘many worries’ (8), ‘fights often’ (12), ‘often unhappy’ (13) and ‘steals’ (22). It mirrors the AAA-scales captured by the CBCL-DP well (Holtmann et al. Reference Holtmann, Becker, Banaschewski, Rothenberger and Roessner2011a ; Carballo et al. Reference Carballo, Serrano-Drozdowskyj, Nieto, De Neira-Hernando, Pérez-Forminaya, Molina-Pizarro, De León-Martínez and Baca- García2014). Carballo et al. (Reference Carballo, Serrano-Drozdowskyj, Nieto, De Neira-Hernando, Pérez-Forminaya, Molina-Pizarro, De León-Martínez and Baca- García2014) investigated the SDQ-DP in a clinical sample of 623 children and adolescents. They corroborated that SDQ-DP seems to be a valuable clinical assessment tool for identifying children with poor self-regulation and severe functional psychosocial impairments.
Little is known about the developmental trajectory of the DP from middle childhood (9–13 years of age) to adolescence although this is a highly dynamic phase of change. Caprara et al. (Reference Caprara, Paciello, Gerbino and Cugini2007) found that irritability development from adolescence to adulthood could follow one of four trajectories: low stable, medium declining, medium stable or high stable in 500 youths followed from 12 to 20 years of age in a clinical setting. Wiggins et al. (Reference Wiggins, Mitchell, Stringaris and Leibenluft2014) investigated mood dysregulation in terms of irritability in a large, population based cohort of children predominantly from low-income households. They found five main trajectory types of irritability across toddlerhood and middle childhood. Maternal depression and exposure to violence, maternal drug and alcohol abuse, as well as paternal depression and alcohol abuse were identified as risk factors for membership in the more severe irritability classes. So far, no study focused on the development of children in middle childhood to early adolescence. However, this is an important period of development, considering that during normal development there is a trend toward the use of more cognitively guided self-regulation strategies during this time (Garnefski & Kraaij, Reference Garnefski and Kraaij2006; Stegge & Terwog, Reference Stegge, Terwog and Gross2007).
In 2015 Caro-Cañizares et al. reviewed the literature concerning biological and environmental predictors of the DP in children and adolescents (Caro-Cañizares et al. Reference Caro-Cañizares, García-Nieto and Carballo2015). They revealed a lack of studies focusing on predictors of the DP in children and adolescents. Only one large longitudinal community-based study that investigated predictors of the DP was reported: Dougherty et al. (Reference Dougherty, Smith, Bufferd, Carlson, Stringaris, Leibenluft and Klein2014) assessed disruptive mood dysregulation disorder (DMDD) in 6-year-old children (n = 462) using a parent-reported structured clinical interview. Lower effortful control, parental lifetime substance use disorders, and higher parental hostility at age 3 were related to DMDD at age 6.
In our study characteristics were selected based on known determinants (risk or protective factor) of the onset and course of mental health problems as available from the BELLA data (Wille et al. Reference Wille, Bettge and Ravens-Sieberer2008): child related clinical factors (SDQ-impact score, internalising problems, externalising problems); environmental and psychosocial factors (socioeconomic status, quality of life, parent's mental health problems, dysfunctional family climate).
To address the mentioned gaps in literature, the present study pursued the following three objectives: (1) elucidating dimensional aspects of dysregulation development from middle childhood to early adolescence (children aged 9–13 years) to find developmental trajectories of SDQ-DP; (2) investigating factors that predict these SDQ-DP trajectory classes in terms of children's psychosocial and clinical characteristics; (3) identifying gender associated trajectory-classes of individuals by examining differences using a person-centred approach.
Method
Subjects
Our analyses are based on data from the BELLA study, which is the mental health module of the National Health Interview and Examination Survey for children and adolescents (KiGGS) (cf. Becker et al. Reference Becker, Rothenberger and Sohn2015). The KiGGS study and its Mental Health Module BELLA are the combination of a cross-sectional and a longitudinal study that could be methodologically characterised as a cohort sequence study. Conceptualization, design, methods and ethical approval of BELLA are described in detail in Ravens-Sieberer et al. (Reference Ravens-Sieberer and Kurth2008) as well as Ravens-Sieberer et al. (Reference Ravens-Sieberer, Otto, Kriston, Rothenberger, Döpfner, Herpertz-Dahlmann, Barkmann, Schön, Hölling, Schulte-Markwort and Klasen2015). A random selection of 4199 families from the KiGGS sample with children and adolescents aged 7–17 was initially asked to participate in the BELLA study. The sample is representative in terms of age, gender, regional and citizenship structure of the population living in Germany. Data collection was carried out between May 2003 and June 2006 at baseline.
The present study analysed data from the BELLA-cohort focussing on the first three measurement points (baseline, 1- and 2-year follow-ups) for reason of sample size. The statistical analyses of this study are based on a representative BELLA subsample comprising complete data sets over all three measurement points. We focused on the analyses of the range from 9 to 13 years of age investigating the parent version of the SDQ. Available data for child related clinical and environmental/psychosocial factors were included. This resulted in a sample of n = 564 participants, 267 were female and 297 were male (age: T0 = 9−11 years; T1 = 10–12 years; T2 = 11–13 years). In each family, one parent was interviewed concerning the child's behaviour using a standardised computer-assisted telephone interview. In addition, the parents were asked to fill in a set of questionnaires sent by mail.
Measures
Outcome variable SDQ-DP
The SDQ was completed by the parents. It is a short screening instrument of 25 items that contains five different subscales measuring ‘emotional symptoms’, ‘conduct problems’, ‘hyperactivity-inattention’, ‘peer relationship problems’ and ‘prosocial behaviour’ (Goodman, Reference Goodman1999). Each of the items of the SDQ is scored on a 3-point scale with 0 = ‘not true’, 1 = ‘somewhat true’ and 2 = ‘certainly true’. Higher scores are indicating more serious problems, except for ‘prosocial behaviour’, where higher scores indicate more positive behaviour. The psychometric properties of the SDQ are satisfactory to good, and its subscales are corresponding to the major categories and criteria of the current psychiatric classification systems (Becker et al. Reference Becker, Woerner, Hasselhorn, Banaschewski and Rothenberger2004). Concerning the SDQ-DP a dichotomisation of the data as ‘normal’ or ‘abnormal’ was realised (scale values <5 = ‘normal’; scale values ⩾5 = ‘abnormal’) (Holtmann et al. Reference Holtmann, Becker, Banaschewski, Rothenberger and Roessner2011a ). This is different from the other SDQ-Scales, for which three ranges, ‘normal’, ‘borderline’ and ‘abnormal’ are defined. Holtmann et al. (Reference Holtmann, Becker, Banaschewski, Rothenberger and Roessner2011a ) computed the SDQ-DP cut-off ⩾5 based on the CBCL-DP sum scores (T-score of the relevant scales ⩾210) following Hudziak et al. (Reference Hudziak, Althoff, Derks, Faraone and Boomsma2005).
Environmental and psychosocial factors
-
(1) The participants’ socioeconomic status was quantified using the Winkler-Index as reference (Winkler & Stolzenberg, Reference Winkler and Stolzenberg1999) based on net household income, parental level of education and occupational status. This allows for a differentiation between lower, middle and upper socioeconomic class (Lange et al. Reference Lange, Kamtsiuris, Lange, Schaffrath-Rosario, Stolzenberg and Lampert2007; Klasen et al. Reference Klasen, Otto, Kriston, Patalay, Schlack and Ravens-Sieberer2015). For the KiGGS study this multidimensional aggregated index was fundamentally revised following critical assessment. The calculation basis was adjusted to accommodate income inflation, increased educational participation and structural occupational changes (Lampert & Kroll, Reference Lampert, Kroll, Richter and Hurrelmann2009).
-
(2) Quality of life was measured using KINDL-R questionnaire, one frequently used generic measure for children and adolescents developed by Ravens-Sieberer & Bullinger (Reference Ravens-Sieberer and Bullinger1998). The 24 items resulted in six subscales: physical well-being, emotional well-being, self-esteem, family, friends and everyday functioning (school or nursery school/kindergarten). Each item is measured on a 5-point scale (1 = ‘none at all’ to 5 = ‘always’) and can be combined to produce a sum score. All scales were transformed so that values range from 24 to 120, with higher values representing better quality of life (Bullinger et al. Reference Bullinger, Brütt, Erhart and Ravens-Sieberer2008).
-
(3) Parent's mental health problems regarding the symptom dimensions somatisation, obsessive-compulsive, interpersonal sensitivity, depression, anxiety, hostility, phobic anxiety, paranoid ideation and psychoticism were determined using the short form with 9 items of the German translation of the Symptom Check List 90 (SCL-90-R, cf. Derogatis et al. Reference Derogatis, Lipman, Rickels, Uhlenhuth and Covi1974; Short Version, SCL-S-9, cf. Klaghofer & Braehler, Reference Klaghofer and Braehler2001). The 5-point Likert-scale (1 = ‘none at all’ to 5 = ‘very severe’) leads to a Global Severity Index ranges from 9 to 45 with higher values indicating more severe psychopathologic symptoms.
-
(4) Dysfunctional family climate was reported using a modified version of the German Family Climate Scale (FCS), which is the German adaption of the Family Environmental Scale (FES). The BELLA study used 12 items on a 4-point Likert-scale concerning activities within the family and caring for each other offering answering options from 1 = ‘not true’ to 4 = ‘exactly true’. The sum score ranges from 12 to 48 and a higher mean over the respective items indicates a more positive family climate (Klasen et al. Reference Klasen, Otto, Kriston, Patalay, Schlack and Ravens-Sieberer2015). In our study, we converted this factor to negative to address it as a possible risk factor.
Child related clinical factors
-
(1) The SDQ-impact score was used to define the severity of impairment by using items on overall distress that are summed to generate an impact score that ranges from 0 to 10 (Goodman, Reference Goodman1999).
-
(2) Externalising problems were investigated using the two subscales of the CBCL, aggressive and dissocial behaviour, analysed according to the recommendation of the German working group (Arbeitsgruppe Deutsche Child Behavior Checklist, 1998). Externalising problems were measured with 20 items assessing aggressive behaviour and 13 items assessing delinquent behaviour on a 3-point scale (0 = ‘not true’, 1 = ‘somewhat true’ and 2 = ‘certainly true’). The sum score ranges from 0 to 66 with higher scores indicating more serious externalising problems.
-
(3) Internalising problems were investigated by joining two scales (SCARED + CES) that were oriented on diagnostic criteria for anxiety and depression (ICD-10 and DSM IV) used in the BELLA study: SCARED (Screen for child anxiety related emotional disorders, parent report (Birmaher et al. Reference Birmaher, Khetarpal, Brent, Cully, Balach, Kaufmann and Neer1997; Birmaher et al. Reference Birmaher, Brent, Chiappetta, Bridge, Monga and Baugher1999), analysed according to the recommendation of Birmaher et al. (Reference Birmaher, Khetarpal, Brent, Cully, Balach, Kaufmann and Neer1997)); CES (Center for Epidemiological Studies Depression Scale for Children, parent report (Faulstich et al. Reference Faulstich, Carey and Ruggiero1986)). SCARED were measured with 41 items on a 3-point scale (0 = ‘not true’, 1 = ‘somewhat true’ and 2 = ‘certainly true’). CES were measured with 20 item on a 4-point scale (0 = ‘not at all’, 1 = ‘a little’, 2 = ‘some’ and 3 = ‘a lot’). The sum score ranges from 0 to 142 with higher scores indicating more serious internalising problems.
Statistical analysis
The structure of our statistical analysis including the design of tables and figures followed the study of Isensee et al. (Reference Isensee, Castelao and Kröner-Herwig2016). We first gender-specifically examined the descriptive characteristics of outcome variable (SDQ-DP from Waves 1 to 3) and predictors (Wave 1). Differences in gender outcome variables and predictors were examined by an independent-samples t test. Changes in SDQ-DP value over time were evaluated in a descriptive manner by a series of paired-samples t tests among three sets of samples: total, boys and girls.
Next, we investigated the possible heterogeneity in the development of SDQ-DP by adopting the person-centred method Latent Class Growth Analysis (LCGA). This semi-parametric, group-based modelling strategy helps to determine whether there are different developmental subgroups in the population (Nagin, Reference Nagin1999; Reference Nagin2005) and identifies heterogeneous trajectories that differ in terms of intercept (initial level) and slope (average growth). LCGA is a special type of Growth Mixture Modelling (GMM), whereby the variance and covariance estimates for the growth factors within each class are assumed to be fixed to zero instead of being freely estimated (Jung & Wickrama, Reference Jung and Wickrama2008). Therefore, LCGA reduces computational burden and avoids problem of convergence. Taking account of the databases on gender differences in SDQ-DP, we conducted separate analyses for boys and girls to capture their unique characteristic in SDQ-DP development. The analytical approach was based on an accelerated longitudinal design, in which age, rather than wave of assessment, was the time unit (Duncan et al. Reference Duncan, Duncan and Hops1996; Reference Duncan, Duncan and Strycker2001; Reference Duncan, Duncan and Strycker2006). In this design, each adolescent has five responses of SDQ-DP (age 9–13). Two of them were missing values dealt with Full Information Maximum Likelihood (FIML). Three of them were true responses from consecutive observations. The accelerated longitudinal design maximises the advantages of the cohort design in the BELLA Study, obtaining the broader age span.
To find the optimal latent class growth model with best goodness of fit with the examined data, models with different numbers of classes were compared with a combination of different model selection criteria. First, Lo–Mendell–Rubin test (LMRT) and a Bootstrap Likelihood Ratio Test (BLRT) were used to compare the k–1 and the k class models. A significant p value in LMRT and BLRT represents a statistically significant improvement in model fit with the inclusion of one more class. Second, we examined the Bayesian Information Criterion (BIC) and the Sample-size Adjusted BIC (ABIC) in which lower (i.e. closer to 0) value indicates better balance between model fit and parsimony. Third, we examined the entropy index that ranges from 0 to 1. Entropy closer to 1 represents a more accurate classification. Finally, considering validity and usefulness we set a minimum value requirement of group size of 5%.
To examine the predictive value of wave 1 risk factors for the SDQ-DP development, we conducted gender-specific multinomial logistic regression analyses with wave 1 risk factors as predictors to examine their influence on SDQ-DP trajectory group membership. As recommended by Muthén (Reference Muthén2004), we used a conditional model, including these predictors while modelling the latent class growth models, to consider their influence on class membership.
The LCGA and multinomial logistic regression were performed using Mplus version 7.3 (Muthén & Muthén, Reference Muthén and Muthén1998–2015). Other analyses were carried out using SPSS version 23. Individuals with missing data on outcome variables were dealt with using the FIML method in Mplus. However, this procedure cannot be used for missing data on predictors and was deleted from the analyses (Muthén & Muthén, Reference Muthén and Muthén1998–2015).
Results
A total of 267 girls and 297 boys took part in this study. Overall, the following number of children matched criteria for the SDQ-DP concerning a cut-off ⩾5 points at each time point of measurement: T1 (total sample 3.01%, girls 1.87% and boys 4.04%); T2 (total sample 2.84%, girls 1.87% and boys 3.70%); T3 (total sample 3.01%, girls 1.87% and boys 4.04%). Table 1 presents the group characteristics with the available sample size, the means and standard deviation for the three outcome measures of SDQ-DP and the predictor variables. Both genders scored at low level concerning the SDQ-DP at all three waves. For the total sample, there were no significant differences between the scores of the SDQ-DP over time (all p > 0.05). At all three assessment points, both genders differed significantly with respect to their SDQ-DP. Boys showed a higher level of dysregulation at T1 (p = 0.015; d = 0.21), T2 (p = 0.001; d = 0.27) and T3 (p = 0.012; d = 0.21). Concerning the predictor variables, externalising problems differed significantly between boys and girls (p = 0.022; d = 0.20). In contrast, no significant gender differences were found in SDQ-impact score, internalising problems, quality of life, parent mental health problems, socioeconomic status and dysfunctional family climate (all p > 0.05).
Table 1. Descriptive statistics of gender-specific outcome variables and predictors

M, Mean; s.d., Standard deviation; Min, Minimum; Max, Maximum; DP1, DP2, DP3, dysregulation profile at T1, T2 and T3; SES, socioeconomic status; QUA, quality of life; PMP, parent mental health problems; DYSFC, dysfunctional family climate; IMP, SDQ impact score; EXT, externalising problems; INT, internalising problems.
Note. The total sample size is 564.
Conditional model for girls
The three-class solution was identified as the best-fitting model for the subsample of girls. The BIC decreased with a three-class model and increased again with a four-class solution (BIC1 = 12 568.571; BIC2 = 216.575; BIC3 = 2019.273; BIC4 = 2034.670;). The classification accuracy was deemed satisfactory (Entropy = 0.978).
A significant value of the BLRT (p < 0.001) indicated that the three-class model was favourable to the two-class solution. The largest class in the girls’ subsample (67%; ‘low SDQ-DP class’; Fig. 1) showed a very low level of SDQ-DP at the first assessment point and across time, without any changes in slope (i = 0.596, p < 0.001; s = −0.021, p = 0.900). The second largest class (28.0%; ‘moderate SDQ-DP class’) displayed a moderate level of SDQ-DP across all waves, again without any changes in time (i = 2.001, p < 0.001; s = −0.450, p = 0.257). The third group of girls (5.0%; ‘high SDQ-DP class’) had the highest SDQ-DP level at first assessment, which was close to the cut-off score of 5. Again, in this class there was no significant change in slope over time (i = 4.066, p < 0.001; s = 0.514, p = 0.753). However, on a descriptive level an increase in SDQ-DP scores was observed from age 9 to 13 years. The subgroup proportions for girls and boys showed in Fig. 1 and Table 3 are different because the former were based on an estimated model while the latter were based on each individual's most likely latent class membership.

Fig. 1. Trajectories of dysregulation profile in boys (n = 297) and girls (n = 267).
For the multinomial logistic regression analyses, ‘low SDQ-DP’ was chosen as the reference class (Table 2). Results showed that externalising problems [odds ratios (OR) 4.83], as well as internalising problems (OR 1.98), significantly increased the risk of being categorised into the ‘moderate SDQ-DP class’ when compared with the ‘low SDQ-DP class’. Quality of life was (OR 0.36) also significant and functioned as a protective factor.
Table 2. Results from multinomial logistic regression analyses

OR, odds ratios; l95% CI, lower 95% confidence intervals; u95% CI, upper 95% confidence intervals; DP1, DP2, DP3, dysregulation profile at T1, T2 and T3; SES, socioeconomic status; QUA, quality of life; PMP, parent mental health problems; DYSFC, dysfunctional family climate; IMP, SDQ impact score; EXT, externalising problems; INT, internalising problems.
Note. Odds ratios of IMP in girls are not reported due to unconvergence of the model.
With regards to the ‘high SDQ-DP class’, externalising problems (OR 5.79) and internalising problems (OR 1.98) differentiated between this class and the ‘low SDQ-DP class’. Quality of life (OR 0.36) again functioned as a protective factor just like the socioeconomic status (OR 0.00). Both showed significance in prediction. ORss of SDQ impact score in girls are not reported due to non-convergence of the model. Also, regarding girls, parental mental health problems and dysfunctional family climate showed no validity for prediction.
Conditional model for boys
In the boys’ subsample, the BIC declined from the two-class to the three-class solution (BIC2 = 2526.001; BIC3 = 2461.658). Fit indices [entropy (0.871) and BLRT] for the three-class model were good. In contrast, the four-class models showed instable results and lower values of model fit.
For the three-class solution, which was chosen as the optimal model, the largest class in the boys’ subsample (59.5%; ‘low SDQ-DP class’; Fig. 1) had a very low level of SDQ-DP at baseline, as well as across all assessment points, without changes in slope (i = 0.531, p < 0.001; s = 0.143, p = 0.502). The second largest class (31.7%; ‘moderate SDQ-DP class’) showed a moderate level of SDQ-DP across all waves, again without any changes across time (i = 1.953, p < 0.001; s = 0.092, p = 0.813). The third group of boys (8.8%; ‘high-SDQ-DP class’) had the highest level of SDQ-DP, close to the cut-off score of five (Fig. 1). Boys of this class did not show changes in their SDQ-DP level over the time of assessment (i = 4.845, p < 0.001; s = −1.456, p = 0.143). Only on a descriptive level, a considerable decrease of the SDQ-DP scores was observed in this class.
Results of multinomial logistic regression analyses revealed that externalising problems (OR 1.20), dysfunctional family climate (OR 1.24), as well as internalising problems (OR 1.14), increased the risk of belonging to the ‘moderate SDQ-DP class’ compared with the ‘low SDQ-DP class’. In contrast quality of life (OR 0.88) was a protective factor.
Concerning the ‘high SDQ-DP class’, the SDQ impact score (OR 2.79), externalising problems (OR 1.37), as well as internalising problems (OR 1.26) discriminated between the high class and the reference class. Regarding the boys, the variables socioeconomic status, quality of life, parent mental health problems and dysfunctional family climate did not predict a membership of an affected class as compared to the low SDQ-DP class.
We additionally compared the mean scores of the variables in the three SDQ-DP classes low, moderate, and high for each gender. Considering the direction of coding (potential protective or risk factor) a comprehensible trend could be shown for all variables with higher mean scores for risk factors and lower mean scores for protective factors from the ‘low SDQ-DP class’ up to the ‘high SDQ-DP class’ (Table 3).
Table 3. Comparison of predictors in the low, moderate and high SDQ-DP classes
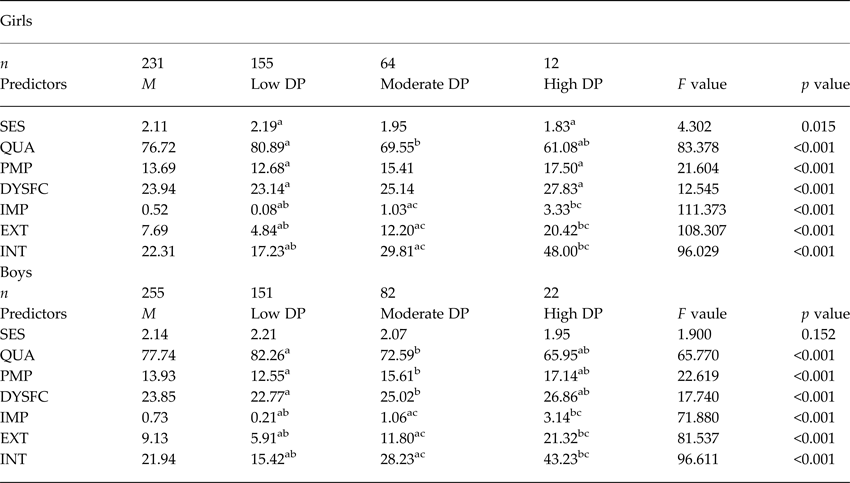
M, Mean; DP1, DP2, DP3, dysregulation profile at T1, T2 and T3; SES, socioeconomic status; QUA, quality of life; PMP, parent mental health problems; DYSFC, dysfunctional family climate; IMP, SDQ impact score; EXT, externalising problems; INT, internalising problems.
Note. the same pair of superscripts represents pairs of values with significant differences (i.e. for SES, girls in the low DP and high DP class have the same superscript ‘a’, showing significant differences in SES means between low DP and high DP classes).
Discussion
This large scale epidemiological study identified gender associated developmental trajectories of the SDQ-DP. Furthermore it analysed the influence of risk and protective factors on the courses of the revealed trajectories from 9 to 13 years of age.
In the first step of our analysis we found a low level of SDQ-DP scores for the total sample including both genders (Table 1). In our study sample 3% (T1 = 3.01, T2 = 2.84, T3 = 3.01%) of all children met the SDQ-DP criteria with recommended cut-off ⩾5 points (Holtmann et al. Reference Holtmann, Becker, Banaschewski, Rothenberger and Roessner2011a ) at each point of measurement. This is in accordance with the results of Holtmann et al. (Reference Holtmann, Becker, Banaschewski, Rothenberger and Roessner2011a ) who detected a prevalence of the SDQ-DP of 2.6% evaluating a German epidemiological sample of children and adolescents. Our results are also confirmed by previous findings with a prevalence of the DP (usually assessed by the CBCL-DP) of about 1–3.8% in community-based samples (Hudziak et al. Reference Hudziak, Althoff, Derks, Faraone and Boomsma2005; Volk & Todd, Reference Volk and Todd2007; Althoff et al. Reference Althoff, Verhulst, Rettew, Hudziak and van der Ende2010) and 6–28% in child psychiatric clinical samples (Holtmann et al. Reference Holtmann, Goth, Wockel, Poustka and Bolte2008; Holtmann et al. Reference Holtmann, Becker, Banaschewski, Rothenberger and Roessner2011a ; Carballo et al. Reference Carballo, Serrano-Drozdowskyj, Nieto, De Neira-Hernando, Pérez-Forminaya, Molina-Pizarro, De León-Martínez and Baca- García2014). There were no statistically significant differences between the mean scores of the SDQ-DP considering the three points of measurement. Concerning the severity level of SDQ-DP in our study, boys were more affected than girls at all three points of measurement. Externalising behaviour showed a significant influence on this gender associated difference. Gender differences in prevalence and severity level of the DP were reported in previous studies with inconsistent results. Some studies indicated that DP was more prevalent in girls [e.g. nationwide representative general population sample of 2856 children and adolescents aged 4–18 years (Holtmann et al. Reference Holtmann, Bölte, Goth, Döpfner, Plück, Huss, Fegert, Lehmkul, Schmeck and Postka2007)]. Another study showed no gender difference [epidemiological cohort study with 325 young adults, birth to age 19 years (Holtmann et al. Reference Holtmann, Buchmann, Esser, Schmidt, Banaschewski and Laucht2011b )], and most studies indicated that boys were overrepresented corresponding with our results: 2076 children from 13 Dutch birth cohorts 4–16 years of age sampled every 2 years (Althoff et al. Reference Althoff, Verhulst, Rettew, Hudziak and van der Ende2010); 9024 patients aged 4–18 (Jucksch et al. Reference Jucksch, Salbach-Andrae, Lenz, Goth, Döpfner, Poustka, Freitag, Lehmkul, Lehmkul and Holtmann2011); longitudinal data on 8013 Dutch twin pairs (Boomsma et al. Reference Boomsma, Rebollo, Derks, Van Beijsterveldt, Althoff, Rettew and Hudziak2006). However, the different findings may in part be attributed to sampling differences and examination of different age spans.
Based on our statistical analyses with a combination of different model selection criteria the second step of our analyses identified three distinct trajectories for each gender. The three gender associated classes could be identified, with good separation of classes and a stable nature of dysregulation over time. They corresponded to low, moderate and high symptom scores for the outcome variable. Thus, the DP-results fit in the psychometrical nature of the basic SDQ scales with the assignment of symptoms to normal, borderline and abnormal.
As expected children with very low symptom scores formed the largest group (girls 67% and boys 60%). They showed no or low signs of SDQ-DP with stable trajectories and a symptom score level below one in both genders and thus can be considered as non-affected and age-appropriate (cf. Becker et al. Reference Becker, Rothenberger and Sohn2015). The second largest group displayed a moderate level of SDQ-DP across all waves, again without any changes over time (girls 28% and boys 32%) revealing a stable symptom level. These findings correspond with other studies that detected similar group sizes of children with stable low and low to moderate symptom course (Hudziak et al. Reference Hudziak, Althoff, Derks, Faraone and Boomsma2005; Althoff et al. Reference Althoff, Verhulst, Rettew, Hudziak and van der Ende2010).
The third group was the smallest (girls 5% and boys 9%) and showed high symptom levels of SDQ-DP close to the cut-off. In the boys’ high SDQ-DP trajectory symptom scores tended to decrease with age. This may be due to the findings of other studies that externalising children's neuronal functions and their behaviours may change rapidly over time (Rothenberger et al. Reference Rothenberger, Woerner and Blanz1987; Woerner et al. Reference Woerner, Rothenberger and Lahnert1987). Furthermore, Hudziak et al. (Reference Hudziak, van Beijsterveldt, Bartels, Rietveld, Rettew, Derks and Boomsma2003) described that aggression and dysregulation diminishes in boys and girls during development (Stanger et al. Reference Stanger, Achenbach and Verhulst1997). Also, genetic and environmental influences on behaviour may change with development. Thus, an interesting finding in our study is the girls’ high SDQ-DP trajectory, where the DP-symptom scores tended to increase over time, although, as a general group effect, dysregulation usually decreases during this developmental period (Hudziak et al. Reference Hudziak, van Beijsterveldt, Bartels, Rietveld, Rettew, Derks and Boomsma2003; Leibenluft & Stoddard, Reference Leibenluft and Stoddard2013). Further, analysing trajectories of subgroups may help to better understand why the latter is not the case in some children. This could nurture a more personalised treatment approach.
In the third step of our study, we investigated the influence of psychosocial and child-related clinical predictors on the SDQ-DP trajectories. For girls and boys, internalising as well as externalising problems increased the risk to fall into the moderate or high SDQ-DP class. The comparison of both genders (see Table 2) revealed that the predictive value of externalising problems was considerably higher in the girls’ moderate and high classes (OR 4.83 and 5.79) compared to the boys’ moderate and high classes (OR 1.20 and 1.37). Although the mean scores for externalising problems are similar in different classes of boys and girls, this finding hypothetically expresses a higher impact of externalising behaviour in girls on the SDQ-DP compared with boys. Remarkably, the same tendency was found for the influence of internalising problems on the SDQ-DP comparing both genders (girls OR 1.98 and 1.98; boys OR 1.14 and 1.20). Most likely this indicates a higher risk for girls with mainly externalising but also internalising problems concerning their mental health development during this period in comparison with boys. Our results add information concerning gender aspects to the findings of Stringaris & Godman (Reference Stringaris and Goodman2009). They investigated cross-sectional data were taken from a national mental health survey in the UK and found that mood lability was particularly strongly associated with comorbidity between internalising and externalising disorders.
We examined parental mental health problems, the SDQ impact score and dysfunctional family climate as possible risk factors concerning the course of SDQ-DP in children. Our study found a significant influence of the SDQ impact score (boys’ low v. high SDQ-DP class), increasing the risk to fall into the high affected class. A significant influence of dysfunctional family climate was shown only for the boys’ low v. moderate SDQ-DP class. However, comparing the mean scores in the low, moderate and high SDQ-DP classes (Table 3) all three characteristics revealed a trend in the expected direction, suggesting that the SDQ impact score, dysfunctional family climate and parental mental health problems are risk factors for children's mental health.
On the other hand, we could show that good quality of life decreased the risk to fall into moderate and high SDQ-DP classes in boys and girls. However, the socioeconomic status did not show the expected influence. Only in the girls’ high SDQ-DP class it functioned as a significant protective factor reducing the probability to be categorised as part of the high SDQ-DP class. Whether the socioeconomic status only serves as a significant protective factor for girls in comparison to boys, has yet to be examined.
There exist some limitations of this study. First, the analytical approach led to missing values (3 data values, 2 missing values), but maximises the advantages of the cohort design in the BELLA Study, obtaining the broader age span. This procedure possibly reduced the validity of the trajectories. Therefore, we were cautious in the interpretation of these results and described developmental trends only on a descriptive level. Second, for the predictor variable SDQ impact score in the girls’ subsample, our model did not converge. In consequence, we could not report a valid result on multinomial logistic regression analyses as discussed above. However, ANOVA results were presented on a more descriptive level in Table 3. Third, the data on SDQ-DP in this study were based solely on parent-reports. Parents might have a different, limited estimation of the child's experience, especially concerning internalising behaviour. This might have reduced the validity of the presented results. Future research could benefit from including both parent- and self-report.
The psychometric validity of the SDQ-DP was investigated by Holtmann et al. (Reference Holtmann, Becker, Banaschewski, Rothenberger and Roessner2011a ) in a clinical setting. On this basis, Carballo et al. (Reference Carballo, Serrano-Drozdowskyj, Nieto, De Neira-Hernando, Pérez-Forminaya, Molina-Pizarro, De León-Martínez and Baca- García2014) added evidence of the clinical value of the SDQ-DP in their clinical study. Our study is the first investigating the SDQ-DP in a population based sample. The extent to which the SDQ-DP utilised in this study maps onto the CBCL-DP and similarly predicts future mental problems, may still require corroboration given the fact that the SDQ-DP subscale comprises only five items compared with the CBCL's 44 items included in the CBCL-DP. Winsper & Wolke (Reference Winsper and Wolke2014) stated that a 15-item scale of the SDQ utilising full information garnered by three SDQ subscales (negative emotions, conduct problems and hyperactivity) may be more appropriate for community population to ensure sufficient sensitivity when detecting sub-clinical levels of behavioural and emotional dysregulation. However, our study served as substantiation of the concept of the SDQ-DP as validated equivalent of the CBCL-DP (Holtmann et al. Reference Holtmann, Becker, Banaschewski, Rothenberger and Roessner2011a ) adding valuable findings to the existing literature on DP.
The main strenght of this study are the use of a highly complex and valuable statistical method, the general community based longitudinal study-design with large sample size and the chosen age-span. We investigated gender associated trajectory classes of SDQ-DP and the predictive value of clinical, environmental and psychosocial characteristics. Findings from this study added three distinguishable trajectories of SDQ-DP in boys and girls to the known clinical and scientific value of the SDQ-DP. Mainly externalising also internalising problems were pointed out as risk factors being associated with an undesirable course of SDQ-DP. Good quality of life functioned as a protective factor on the SDQ-DP course.
Using the SDQ-DP as outcome variable our study may support the need for comprehensive screening and consecutive counselling, monitoring and treatment. It may contribute to the growing number of studies concerning trans-diagnostic sharing of aetiological and pathophysiological factors in child psychiatry consistent with the Research Domain Criteria Initiative (Shaw et al. Reference Shaw, Stringaris, Nigg and Leibenluft2014; Sonuga-Barke et al. Reference Sonuga-Barke, Cortese, Fairchild and Stringaris2015; NIMH Research Domain Criteria, 2016).