Posttraumatic stress disorder (PTSD) is a debilitating psychiatric disorder characterized by a prolonged and maladaptive response to traumatic events (American Psychiatric Association, 2013). It has a significant toll on both society and the individual and is associated with significant psychological dysfunction and health impairments (Stein et al., Reference Stein, Walker, Hazen and Forde1997; Kessler, Reference Kessler2000; Deykin et al., Reference Deykin, Keane, Kaloupek, Fincke, Rothendler, Siegfried and Creamer2001; Westphal et al., Reference Westphal, Olfson, Gameroff, Wickramaratne, Pilowsky, Neugebauer, Lantigua, Shea and Neria2011). While lifetime prevalence is estimated at 6% (Goldstein et al., Reference Goldstein, Smith, Chou, Saha, Jung, Zhang, Pickering, Ruan, Huang and Grant2016), for military veterans, a highly trauma-exposed population, prevalence rates are even higher, reaching 23% (Fulton et al., Reference Fulton, Calhoun, Wagner, Schry, Hair, Feeling, Elbogen and Beckham2015), with increased severity and comorbidity rates (Tsai et al., Reference Tsai, Harpaz-Rotem, Armour, Southwick, Krystal and Pietrzak2015).
Despite extensive research, PTSD symptom structure is still highly debated (Hoge et al., Reference Hoge, Yehuda, Castro, McFarlane, Vermetten, Jetly, Koenen, Greenberg, Shalev, Rauch, Marmar and Rothbaum2016; McNally, Reference McNally2016, Reference McNally2017; Armour et al., Reference Armour, Fried, Deserno, Tsai and Pietrzak2017; McNally et al., Reference McNally, Heeren and Robinaugh2017a), as reflected in the ongoing discussion surrounding its diagnosis following the publication of the Diagnostic and Statistical Manual of Mental Disorders-5 (DSM-5; Galatzer-Levy and Bryant, Reference Galatzer-Levy and Bryant2013; Hoge et al., Reference Hoge, Riviere, Wilk, Herrell and Weathers2014; Hoge et al., Reference Hoge, Yehuda, Castro, McFarlane, Vermetten, Jetly, Koenen, Greenberg, Shalev, Rauch, Marmar and Rothbaum2016), which presented marked differences in the diagnostic criteria of PTSD, (Hoge et al., Reference Hoge, Riviere, Wilk, Herrell and Weathers2014; Hoge et al., Reference Hoge, Yehuda, Castro, McFarlane, Vermetten, Jetly, Koenen, Greenberg, Shalev, Rauch, Marmar and Rothbaum2016) including, among others, a more restrictive definition of criterion A and marked changes in the number and nature of individual symptoms and symptom-clusters required for diagnosis (Armour et al., Reference Armour, Fried, Deserno, Tsai and Pietrzak2017). Importantly, this has led to lower PTSD prevalence rates compared with DSM-IV (Kilpatrick et al., Reference Kilpatrick, Resnick, Milanak, Miller, Keyes and Friedman2013). Noticeable diagnostic differences also exist between DSM-5 and the International Classification of Diseases-11 (ICD-11; World Health Organization, 2018), which includes only six mandatory symptoms assumed to reflect core PTSD symptoms not shared by other disorders (Cloitre et al., Reference Cloitre, Garvert, Brewin, Bryant and Maercker2013; Maercker et al., Reference Maercker, Brewin, Bryant, Cloitre, Reed, van Ommeren, Humayun, Jones, Kagee, Llosa, Rousseau, Somasundaram, Souza, Suzuki, Weissbecker, Wessely, First and Saxena2013; Mitchell et al., Reference Mitchell, Wolf, Bovin, Lee, Green, Rosen, Keane and Marx2017). This difference has resulted, once more, in striking differences in PTSD rates (Hoge et al., Reference Hoge, Riviere, Wilk, Herrell and Weathers2014; Stein et al., Reference Stein, McLaughlin, Koenen, Atwoli, Friedman, Hill, Maercker, Petukhova, Shahly, van Ommeren, Alonso, Borges, de Girolamo, de Jonge, Demyttenaere, Florescu, Karam, Kawakami, Matschinger, Okoliyski, Posada-Villa, Scott, Viana and Kessler2014; Hansen et al., Reference Hansen, Hyland, Armour, Shevlin and Elklit2015; Friedman et al., Reference Friedman, Kilpatrick, Schnurr and Weathers2016; Guina, Reference Guina2016; Hoge et al., Reference Hoge, Yehuda, Castro, McFarlane, Vermetten, Jetly, Koenen, Greenberg, Shalev, Rauch, Marmar and Rothbaum2016; Wisco et al., Reference Wisco, Miller, Wolf, Kilpatrick, Resnick, Badour, Marx, Keane, Rosen and Friedman2016b; Mitchell et al., Reference Mitchell, Wolf, Bovin, Lee, Green, Rosen, Keane and Marx2017), found to be 10%-to-30% lower according to ICD-11, compared to DSM-5, among trauma-exposed individuals (Hansen et al., Reference Hansen, Hyland, Armour, Shevlin and Elklit2015; Wisco et al., Reference Wisco, Miller, Wolf, Kilpatrick, Resnick, Badour, Marx, Keane, Rosen and Friedman2016b). While different treatments for PTSD are available, including psychotherapy and pharmacotherapy (Bradley et al., Reference Bradley, Greene, Russ, Dutra and Westen2005; Sullivan and Neria, Reference Sullivan and Neria2009; Steckler and Risbrough, Reference Steckler and Risbrough2012), research consistently shows that more than one-third of PTSD patients never fully remit, even if treated (Difede et al., Reference Difede, Olden and Cukor2014). As importantly noted in the guidelines of the National Institute for Health and Care Excellence (NICE) PTSD, treatment efficacy can only be achieved if the disorder is first accurately recognized (National Institue for Clinical Excellence, 2005; Spoont et al., Reference Spoont, Williams, Kehle-Forbes, Nieuwsma, Mann-Wrobel and Gross2015).
Recently, a novel network perspective on psychopathology has emerged, offering an alternative approach for understanding and conceptualizing symptomatology, which may be of use also in discerning the central and fundamental features of PTSD (McNally, Reference McNally2016, Reference McNally2017; Fried et al., Reference Fried, van Borkulo, Cramer, Boschloo, Schoevers and Borsboom2017; Mitchell et al., Reference Mitchell, Wolf, Bovin, Lee, Green, Rosen, Keane and Marx2017; Fried et al., Reference Fried, Eidhof, Palic, Costantini, Huisman-van Dijk, Bockting, Engelhard, Armour, Nielsen and Karstoft2018). According to the network perspective, psychopathology is not the result of underlying latent variables, but rather is the constitution of symptoms associated in dynamic and causal relations, interacting and possibly self-reinforcing each other (Borsboom et al., Reference Borsboom, Mellenbergh and van Heerden2003; Cramer et al., Reference Cramer, Waldorp, van der Maas and Borsboom2010b; Borsboom and Cramer, Reference Borsboom and Cramer2013; Schmittmann et al., Reference Schmittmann, Cramer, Waldorp, Epskamp, Kievit and Borsboom2013; Boschloo et al., Reference Boschloo, van Borkulo, Rhemtulla, Keyes, Borsboom and Schoevers2015; McNally et al., Reference McNally, Robinaugh, Wu, Wang, Deserno and Borsboom2015; McNally, Reference McNally2016, Reference McNally2017; Fried et al., Reference Fried, van Borkulo, Cramer, Boschloo, Schoevers and Borsboom2017). To use an example put forward by Birkeland and Heir (Reference Birkeland and Heir2017), in the aftermath of traumatic event, intrusive memories of the event might occur, leading to hyperarousal and sleep difficulties, which in turn lead to concentration deficiencies, which can be then looped back to the intrusive memories, creating feedback loops of symptoms that maintain each other. In the corresponding network structure, symptoms are represented by nodes, and the associations or connections between pairs of symptoms/nodes are represented by edges, establishing a specific disorder (Jones et al., Reference Jones, Heeren and McNally2017). The importance of each node within a specific network is quantified by measures of node centrality. As nodes are activated, their activation is transmitted to connected nodes, via the connecting edges, and an episode of a disorders unfolds (McNally et al., Reference McNally, Robinaugh, Wu, Wang, Deserno and Borsboom2015). Thus, network findings can guide clinicians and researchers in measuring disorders and their symptoms, and eventually, in refining the nosology and classification of different psychopathologies. In PTSD, network analysis has been explicitly suggested as a vital tool for selection of diagnostic criteria, and for assessing its occurrence and severity (Phillips et al., Reference Phillips, Wilson, Sun and Morey2018).
Extensive research implementing network models in PTSD among trauma-exposed adult populations, including several in veterans, has been conducted over the last several years, aiming to elucidate the symptom structure of PTSD (McNally et al., Reference McNally, Robinaugh, Wu, Wang, Deserno and Borsboom2015; Knefel et al., Reference Knefel, Tran and Lueger-Schuster2016; Armour et al., Reference Armour, Fried, Deserno, Tsai and Pietrzak2017; Birkeland and Heir, Reference Birkeland and Heir2017; Bryant et al., Reference Bryant, Creamer, O'Donnell, Forbes, McFarlane, Silove and Hadzi-Pavlovic2017; Haag et al., Reference Haag, Robinaugh, Ehlers and Kleim2017; Mitchell et al., Reference Mitchell, Wolf, Bovin, Lee, Green, Rosen, Keane and Marx2017; Spiller et al., Reference Spiller, Schick, Schnyder, Bryant, Nickerson and Morina2017; Afzali et al., Reference Afzali, Sunderland, Batterham, Carragher, Calear and Slade2017a, 2017b; McNally et al., Reference McNally, Heeren and Robinaugh2017a; Benfer et al., Reference Benfer, Bardeen, Cero, Kramer, Whiteman, Rogers, Silverstein and Weathers2018; Epskamp et al., Reference Epskamp, Borsboom and Fried2018; Fried et al., Reference Fried, Eidhof, Palic, Costantini, Huisman-van Dijk, Bockting, Engelhard, Armour, Nielsen and Karstoft2018; Greene et al., Reference Greene, Gelkopf, Epskamp and Fried2018; Moshier et al., Reference Moshier, Bovin, Gay, Wisco, Mitchell, Lee, Sloan, Weathers, Schnurr, Keane and Marx2018; Papini et al., Reference Papini, Rubin, Telch, Smits and Hien2018; Phillips et al., Reference Phillips, Wilson, Sun and Morey2018; Ross et al., Reference Ross, Murphy and Armour2018; Sullivan et al., Reference Sullivan, Smith, Lewis and Jones2018; Vanzhula et al., Reference Vanzhula, Calebs, Fewell and Levinson2018; von Stockert et al., Reference von Stockert, Fried, Armour and Pietrzak2018; Price et al., Reference Price, Legrand, Brier and Hebert-Dufresne2019). While some similarities in findings across studies exist, important disparities emerge (Birkeland and Heir, Reference Birkeland and Heir2017, Phillips et al., Reference Phillips, Wilson, Sun and Morey2018). For example, while amnesia is found to be the least central symptom in most PTSD network studies (McNally et al., Reference McNally, Robinaugh, Wu, Wang, Deserno and Borsboom2015, Armour et al., Reference Armour, Fried, Deserno, Tsai and Pietrzak2017, Birkeland and Heir, Reference Birkeland and Heir2017, Bryant et al., Reference Bryant, Creamer, O'Donnell, Forbes, McFarlane, Silove and Hadzi-Pavlovic2017, Mitchell et al., Reference Mitchell, Wolf, Bovin, Lee, Green, Rosen, Keane and Marx2017, Spiller et al., Reference Spiller, Schick, Schnyder, Bryant, Nickerson and Morina2017, Afzali et al., Reference Afzali, Sunderland, Batterham, Carragher, Calear and Slade2017a, McNally et al., Reference McNally, Heeren and Robinaugh2017a, Benfer et al., Reference Benfer, Bardeen, Cero, Kramer, Whiteman, Rogers, Silverstein and Weathers2018, Fried et al., Reference Fried, Eidhof, Palic, Costantini, Huisman-van Dijk, Bockting, Engelhard, Armour, Nielsen and Karstoft2018, Moshier et al., Reference Moshier, Bovin, Gay, Wisco, Mitchell, Lee, Sloan, Weathers, Schnurr, Keane and Marx2018, Papini et al., Reference Papini, Rubin, Telch, Smits and Hien2018, Phillips et al., Reference Phillips, Wilson, Sun and Morey2018, Ross et al., Reference Ross, Murphy and Armour2018, Vanzhula et al., Reference Vanzhula, Calebs, Fewell and Levinson2018, von Stockert et al., Reference von Stockert, Fried, Armour and Pietrzak2018), symptoms emerging as most central vary considerably across studies, including, among others, getting emotionally upset due to trauma reminders, hypervigilance, intrusive thoughts, concentration difficulties, nightmares, future foreshortening, negative trauma related emotions, detachment, loss of interest, emotional numbing, physical reactions to trauma reminders, and flashbacks (Phillips et al., Reference Phillips, Wilson, Sun and Morey2018). Recently, it has been suggested that these mixed findings might be the result of corresponding differences in traumatic events, such as, for example, man-made trauma v. natural disasters, as this has been shown to affect PTSD presentation, and hence, network structure (Ross et al., Reference Ross, Murphy and Armour2018; Sullivan et al., Reference Sullivan, Smith, Lewis and Jones2018). Interestingly, when limiting past research to include only veteran samples, a more consistent result pattern emerges, demonstrating high centrality of getting emotionally upset by trauma reminders (Armour et al., Reference Armour, Fried, Deserno, Tsai and Pietrzak2017; Mitchell et al., Reference Mitchell, Wolf, Bovin, Lee, Green, Rosen, Keane and Marx2017; Fried et al., Reference Fried, Eidhof, Palic, Costantini, Huisman-van Dijk, Bockting, Engelhard, Armour, Nielsen and Karstoft2018; Moshier et al., Reference Moshier, Bovin, Gay, Wisco, Mitchell, Lee, Sloan, Weathers, Schnurr, Keane and Marx2018; von Stockert et al., Reference von Stockert, Fried, Armour and Pietrzak2018). Other reasons implicated as responsible for observed inconsistencies across studies are differences in sample size, sample type (treatment-seeking, survey data), participants' clinical status (PTSD, probable PTSD, sub-threshold PTSD, trauma-exposed healthy participants), and the PTSD measure used for network construction (self-report, clinician-administered).
While extant network research in PTSD has advanced our knowledge in the field, important gaps still remain, which we aim to address in the present study. First, no study to date has used a sample comprised exclusively of treatment-seeking veterans with PTSD, while also basing analyses on clinician-rated measures only. Importantly, in clinical assessments, self-report measures do not allow for additional inquiries of ambiguous or vague answers (Trull et al., Reference Trull, Widiger and Burr2001), pose a risk for response biases, and are subjected to wording-effects (Moskowitz, Reference Moskowitz1986; Schwarz, Reference Schwarz1999). In PTSD, self-reports can only result in a diagnosis of ‘probable’ PTSD (Wisco et al., Reference Wisco, Marx, Miller, Wolf, Mota, Krystal, Southwick and Pietrzak2016a), imperfectly capturing the examined pathology (McNally, Reference McNally2016). Finally, while a recent study comparing PTSD network structures based on clinician-rated and patient-rated data reported similar network structures, results also showed that their global structure was not statistically identical and that networks also differed on node-clustering (Moshier et al., Reference Moshier, Bovin, Gay, Wisco, Mitchell, Lee, Sloan, Weathers, Schnurr, Keane and Marx2018). Indeed, most PTSD network studies have mentioned both using self-reports and non-clinical samples as limitations necessitating additional research (McNally et al., Reference McNally, Robinaugh, Wu, Wang, Deserno and Borsboom2015; Knefel et al., Reference Knefel, Tran and Lueger-Schuster2016; Armour et al., Reference Armour, Fried, Deserno, Tsai and Pietrzak2017; Birkeland and Heir, Reference Birkeland and Heir2017; Mitchell et al., Reference Mitchell, Wolf, Bovin, Lee, Green, Rosen, Keane and Marx2017; Spiller et al., Reference Spiller, Schick, Schnyder, Bryant, Nickerson and Morina2017; Afzali et al., Reference Afzali, Sunderland, Batterham, Carragher, Calear and Slade2017a, Reference Afzali, Sunderland, Teesson, Carragher, Mills and Slade2017b; Greene et al., Reference Greene, Gelkopf, Epskamp and Fried2018; Ross et al., Reference Ross, Murphy and Armour2018; Sullivan et al., Reference Sullivan, Smith, Lewis and Jones2018; Vanzhula et al., Reference Vanzhula, Calebs, Fewell and Levinson2018; von Stockert et al., Reference von Stockert, Fried, Armour and Pietrzak2018).
Second, while sample size is crucial for establishing a reliable network structure, with robust estimation of edges and centrality metrics powered to detect even small coefficients (Epskamp et al., Reference Epskamp, Borsboom and Fried2018; Epskamp and Fried, Reference Epskamp and Fried2018; Fried et al., Reference Fried, Eidhof, Palic, Costantini, Huisman-van Dijk, Bockting, Engelhard, Armour, Nielsen and Karstoft2018), most studies used small sample sizes (McNally et al., Reference McNally, Robinaugh, Wu, Wang, Deserno and Borsboom2015; Knefel et al., Reference Knefel, Tran and Lueger-Schuster2016; Armour et al., Reference Armour, Fried, Deserno, Tsai and Pietrzak2017; Birkeland and Heir, Reference Birkeland and Heir2017; Haag et al., Reference Haag, Robinaugh, Ehlers and Kleim2017; Spiller et al., Reference Spiller, Schick, Schnyder, Bryant, Nickerson and Morina2017; Afzali et al., Reference Afzali, Sunderland, Batterham, Carragher, Calear and Slade2017a; McNally et al., Reference McNally, Heeren and Robinaugh2017a; Epskamp et al., Reference Epskamp, Borsboom and Fried2018; Greene et al., Reference Greene, Gelkopf, Epskamp and Fried2018; Ross et al., Reference Ross, Murphy and Armour2018; Vanzhula et al., Reference Vanzhula, Calebs, Fewell and Levinson2018). For adequate power, sample size should be considerably larger than the number of parameters estimated in analyses, which given the number of variables in PTSD-related networks, tends to be relatively high (Spiller et al., Reference Spiller, Schick, Schnyder, Bryant, Nickerson and Morina2017; Afzali et al., Reference Afzali, Sunderland, Batterham, Carragher, Calear and Slade2017a, 2017b; McNally et al., Reference McNally, Heeren and Robinaugh2017a, Fried et al., Reference Fried, Eidhof, Palic, Costantini, Huisman-van Dijk, Bockting, Engelhard, Armour, Nielsen and Karstoft2018)Footnote 1Footnote †. To date, six studies used large samples of about 1000 participants (Bryant et al., Reference Bryant, Creamer, O'Donnell, Forbes, McFarlane, Silove and Hadzi-Pavlovic2017; Fried et al., Reference Fried, Eidhof, Palic, Costantini, Huisman-van Dijk, Bockting, Engelhard, Armour, Nielsen and Karstoft2018; Phillips et al., Reference Phillips, Wilson, Sun and Morey2018; Sullivan et al., Reference Sullivan, Smith, Lewis and Jones2018; von Stockert et al., Reference von Stockert, Fried, Armour and Pietrzak2018; Price et al., Reference Price, Legrand, Brier and Hebert-Dufresne2019). Still, four assessed trauma-exposed participants with low-to-none rates of probable PTSD (Bryant et al., Reference Bryant, Creamer, O'Donnell, Forbes, McFarlane, Silove and Hadzi-Pavlovic2017; Sullivan et al., Reference Sullivan, Smith, Lewis and Jones2018; von Stockert et al., Reference von Stockert, Fried, Armour and Pietrzak2018; Price et al., Reference Price, Legrand, Brier and Hebert-Dufresne2019), the fifth pooled data from four datasets differing on country of origin, symptoms assessed, and assessment language and tools (Fried et al., Reference Fried, Eidhof, Palic, Costantini, Huisman-van Dijk, Bockting, Engelhard, Armour, Nielsen and Karstoft2018), and, as stated previously, all six studies assessed PTSD symptoms based on self-report measures only.
Finally, PTSD and depression symptoms frequently co-occur (Neria and Bromet, Reference Neria and Bromet2000; Lapierre et al., Reference Lapierre, Schwegler and LaBauve2007; Au et al., Reference Au, Dickstein, Comer, Salters-Pedneault and Litz2013), with approximately 50% comorbidity rates between PTSD and major depressive disorder (MDD; Kessler et al., Reference Kessler, Sonnega, Bromet, Hughes and Nelson1995; Elhai et al., Reference Elhai, Grubaugh, Kashdan and Frueh2008; Rytwinski et al., Reference Rytwinski, Avena, Echiverri-Cohen, Zoellner and Feeny2014; Flory and Yehuda, Reference Flory and Yehuda2015). Compared to PTSD alone, patients with PTSD-MDD exhibit greater distress, higher suicide risk, and poorer treatment outcome (Blanchard et al., Reference Blanchard, Buckley, Hickling and Taylor1998; Oquendo et al., Reference Oquendo, Friend, Halberstam, Brodsky, Burke, Grunebaum, Malone and Mann2003; Green et al., Reference Green, Krupnick, Chung, Siddique, Krause, Revicki, Frank and Miranda2006; Ramsawh et al., Reference Ramsawh, Fullerton, Mash, Ng, Kessler, Stein and Ursano2014; Flory and Yehuda, Reference Flory and Yehuda2015), with co-morbid patients shown to be more likely to drop out from treatment, to be non-responders to prolonged exposure treatment, and to necessitate longer psychotherapy (Gillespie et al., Reference Gillespie, Duffy, Hackmann and Clark2002; Bryant et al., Reference Bryant, Moulds, Guthrie, Dang and Nixon2003; McDonagh et al., Reference McDonagh, Friedman, McHugo, Ford, Sengupta, Mueser, Carney-Demment, Fournier, Schnurr and Descamps2005; Stein et al., Reference Stein, Dickstein, Schuster, Litz and Resick2012). Thus, understanding the underlying structure of the PTSD-MDD comorbidity can enhance extant efforts to better assess and treat PTSD-MDD patients (Flory and Yehuda, Reference Flory and Yehuda2015; Afzali et al., Reference Afzali, Sunderland, Teesson, Carragher, Mills and Slade2017b). Past research aiming to explore the PTSD-MDD comorbidity has mainly focused on shared features or similarities between disorders. For example, some have suggested that both disorders are high on a psychopathology-related diagnostic dimension of general distress, leading to increased comorbidity rates (i.e. the quadripartite model of psychopathology; Watson, Reference Watson2009), while others have focused on more specific content-overlap between disorders (Elhai et al., Reference Elhai, Grubaugh, Kashdan and Frueh2008; Elhai et al., Reference Elhai, Carvalho, Miguel, Palmieri, Primi and Frueh2011). Research efforts undertaken to support these ideas have employed, among others, exploratory (Blanchard et al., Reference Blanchard, Buckley, Hickling and Taylor1998; Grant et al., Reference Grant, Beck, Marques, Palyo and Clapp2008; Gros et al., Reference Gros, Simms and Acierno2010; Contractor et al., Reference Contractor, Elhai, Fine, Tamburrino, Cohen, Shirley, Chan, Liberzon, Galea and Calabrese2015; Price and van Stolk-Cooke, Reference Price and van Stolk-Cooke2015) and confirmatory factor analytic approaches (Simms et al., Reference Simms, Watson and Doebbeling2002; Armour and Shevlin, Reference Armour and Shevlin2010; Miller et al., Reference Miller, Wolf, Harrington, Brown, Kaloupek and Keane2010). However, an important limitation shared by all of these analyses is that they all assume symptoms to be independent constructs, related only via their corresponding factor, thereby missing important facets of the interplay between specific symptoms of different disorders (Afzali et al., Reference Afzali, Sunderland, Teesson, Carragher, Mills and Slade2017b; Price et al., Reference Price, Legrand, Brier and Hebert-Dufresne2019). Conversely, as described above, the network approach views symptoms as being in dynamic and causal associations, interacting and possibly self-reinforcing each other, hence tapping this important aspect. In line with this view, the network approach conceptualizes comorbidity as the overall constellation of symptom-level associations, including those that exist between symptoms of different disorders (Cramer et al., Reference Cramer, Waldorp, van der Maas and Borsboom2010a; Borsboom et al., Reference Borsboom, Epskamp, Kievit, Cramer and Schmittmann2011; Afzali et al., Reference Afzali, Sunderland, Teesson, Carragher, Mills and Slade2017b; Price et al., Reference Price, Legrand, Brier and Hebert-Dufresne2019). These between-disorder symptom associations are termed bridge nodes, and their importance is measured using centrality measures in a similar fashion to that used for within-disorder nodes. Thus, network analysis can be readily used to examine the role of more specific causes for high PTSD-MDD comorbidity rates such as between-disorder overlapping symptoms as sleep and concentration difficulties, which are part of both depression and PTSD (Spitzer et al., Reference Spitzer, First and Wakefield2007; Cramer et al., Reference Cramer, Waldorp, van der Maas and Borsboom2010a; Afzali et al., Reference Afzali, Sunderland, Teesson, Carragher, Mills and Slade2017b). Importantly, the topic of overlapping symptoms gained renewed attention following the addition of Cluster D (i.e. Negative alterations in cognitions and mood associated with the traumatic event) to PTSD diagnosis per DSM-5 (American Psychiatric Association, 2013), which increased the number of potential overlapping symptoms between PTSD and depression (Mitchell et al., Reference Mitchell, Wolf, Bovin, Lee, Green, Rosen, Keane and Marx2017; Price et al., Reference Price, Legrand, Brier and Hebert-Dufresne2019; but see O’Donnell et al. (Reference O'Donnell, Alkemade, Nickerson, Creamer, McFarlane, Silove, Bryant and Forbes2014) showing similar PTSD-MDD comorbidity rates based on DSM-IV and DSM-5). Still, while providing a novel approach to conceptualize and understand the PTSD-MDD comorbidity, to date, only four studies included depressive symptoms in their network analysis of PTSD. Yet, one measured lifetime occurrence, not current major depressive disorder (MDD) symptoms (Afzali et al., Reference Afzali, Sunderland, Teesson, Carragher, Mills and Slade2017b), and three used self-reported measures of PTSD and depression (Armour et al., Reference Armour, Fried, Deserno, Tsai and Pietrzak2017; Mitchell et al., Reference Mitchell, Wolf, Bovin, Lee, Green, Rosen, Keane and Marx2017; Price et al., Reference Price, Legrand, Brier and Hebert-Dufresne2019).
In line with the above-mentioned gaps in knowledge, here we examined PTSD symptom structure in a sample of clinically-diagnosed, treatment-seeking Israel Defense Forces combat-exposed veterans. Our goal was to extend current knowledge in the following ways: (1) analyze PTSD symptom networks using data from structured clinician-administered interviews; (2) use a large sample (N = 1489); (3) re-estimate network structure when including clinician-rated depressive symptoms; (4) compare the PTSD network to that of trauma-exposed (TE) treatment-seeking combat veterans not meeting PTSD diagnostic criteria; and (5) explore potential directionality among symptoms including depressive symptoms and a daily functioning measure, as the DSM classification system specifically requires significant impairment in one or more areas of functioning for a PTSD diagnosis. Several hypotheses were made. First, due to lack of consensus in the literature on central symptoms, we hypothesized that re-experiencing symptoms would emerge as highly central based on findings of most network studies focusing on trauma-exposed veterans (Armour et al., Reference Armour, Fried, Deserno, Tsai and Pietrzak2017; Mitchell et al., Reference Mitchell, Wolf, Bovin, Lee, Green, Rosen, Keane and Marx2017; Fried et al., Reference Fried, Eidhof, Palic, Costantini, Huisman-van Dijk, Bockting, Engelhard, Armour, Nielsen and Karstoft2018; Moshier et al., Reference Moshier, Bovin, Gay, Wisco, Mitchell, Lee, Sloan, Weathers, Schnurr, Keane and Marx2018; von Stockert et al., Reference von Stockert, Fried, Armour and Pietrzak2018). In line with numerous network studies across different traumatic populations, we also predicted low centrality for amnesia. Second, based on previous studies incorporating depressive symptoms in the network structure of military veterans (Armour et al., Reference Armour, Fried, Deserno, Tsai and Pietrzak2017; Mitchell et al., Reference Mitchell, Wolf, Bovin, Lee, Green, Rosen, Keane and Marx2017), we hypothesized that while the introduction of depressive symptoms would result in several significant bridge nodes, this would have little effect on the PTSD symptom structure. Third, we hypothesized greater overall connectivity in the clinical PTSD group, compared with the trauma-exposed (TE) treatment-seeking patients not meeting full criteria for PTSD, which in accordance with the conceptualization of psychopathology per the network approach and with the only study to date that examined this possibility in veterans with PTSD compared with subthreshold PTSD (Phillips et al., Reference Phillips, Wilson, Sun and Morey2018). Still, we would like to emphasize at this juncture that the current study is the first to employ a network analysis approach using a large sample comprised exclusively of treatment-seeking veterans with PTSD, while also basing analyses on clinician-rated measures only. Thus, present analyses should be regarded as partly exploratory in nature. Put differently, as previous research has implicated numerous factors (e.g. sample size, sample type, participants' clinical status, trauma type, and the PTSD measure used for network construction) in results heterogeneity across studies, current hypotheses are in part extrapolations based on extant findings in the field.
Methods
Participants
Participants were 1795 male veterans seeking treatment from the Israel Defense Forces Unit for Treatment of Combat-Related PTSD (UTC-PTSD), an outpatient clinic specializing in assessing and treating combat-related PTSD. Upon contacting the clinic, veterans were first phone-screened to ascertain past exposure to a combat-related event. Veterans fulfilling this requirement were then invited for a formal clinical assessment by one of the clinic's assessors, namely, psychiatrists, clinical psychologists, or social workers with 12 or more years of experience in PTSD diagnosis in veterans. Importantly, as assessors also completed the mandatory Israel Defense Forces service, they were familiar with the language, manners, behavioral norms, belief system, and rituals of Israeli military culture (Levi et al., Reference Levi, Bar-Haim, Kreiss and Fruchter2016; Levi et al., Reference Levi, Shoval-Zuckerman, Fruchter, Bibi, Bar-Haim and Wald2017). Next, semi-structured diagnostic interviews based on DSM-IV-TR were administered as part of the routine admission process to the clinic. Interviews followed the UTC-PTSD assessment manual, developed within the UTC-PTSD, which comprises an anamnestic section to collect socio-demographic and personal background, as well as general medical information, and a diagnostic section using both clinician-rated measures (i.e. the clinician-administered PTSD Scale (CAPS-IV; Blake et al., Reference Blake, Weathers, Nagy, Kaloupek, Gusman, Charney and Keane1995) and the Montgomery–Asberg Depression Rating Scale (MADRS; Montgomery and Asberg, Reference Montgomery and Asberg1979)) and self-report questionnaires (for full measures description see Measures below). Next, each patient's assessment record was presented in the clinic's staff meeting, and final decisions relating to diagnoses (and ensuing treatment course) were made (Levi et al., Reference Levi, Bar-Haim, Kreiss and Fruchter2016). Veterans with active or past psychosis or current severe substance use disorder were not accepted for treatment at the clinic and were referred out to specialized clinics. Veterans with high levels of active suicidality, as indicated by concrete suicidal plans, were taken to a psychiatric emergency room for immediate intervention. Finally, veterans receiving psychotherapy or pharmacotherapy elsewhere were also excluded from the clinic's care. The present study analyzed the assessment-data collected from 1795 male veterans that were admitted to the clinic's care between 2006-to-2014. Of note, about 15% of patients were still part of the military, but only as reserve forces, during their assessment.
In total, of the 1795 treatment-seeking male veterans, 1489 met diagnostic criteria for PTSD while 306 did not (See Table 1 for demographic characteristics of the two groups), with all being exposed to combat-related traumatic events (i.e. DSM-IV criterion A). Specifically, these events included: (1) participating in direct combat, including incoming mortar and rocket attacks, while participating in one of Israel's major wars (e.g. ‘Second Lebanon War’); (2) receiving incoming small fire-arms and/or experiencing detonations of improvised explosive devices (IEDs) while participating in one of Israel's combat operations (e.g. ‘Operation Cast Led’); (3) routine security measures including clearing and searching operations while undergoing extensive Molotov cocktail and/or stone throwing attacks; and (4) personally knowing someone seriously injured or killed in one of the aforementioned wars or combat operationsFootnote 2. A clinical diagnosis of PTSD was established using CAPS-IV (Blake et al., Reference Blake, Weathers, Nagy, Kaloupek, Gusman, Charney and Keane1995), based on the relatively lenient F1/I2 item rule (i.e. Frequency ⩾ 1, Intensity > 2; Blake et al., Reference Blake, Weathers, Nagy, Kaloupek, Gusman, Charney and Keane1995). Accordingly, PTSD diagnosis necessitated endorsing at least one re-experiencing symptom, three avoidance symptoms, and two hyperarousal symptoms. For the purpose of the present study, those not meeting this requirement were regarded as trauma-exposed (TE) treatment-seeking patients not meeting full criteria for PTSD. Of note, of the 306 TE patients, exactly half (n = 153) met criteria for subthreshold PTSD, defined as fulfilling symptom-requirement of two out of the three symptom-clusters (Keane and Barlow, Reference Keane, Barlow and Barlow2002; Phillips et al., Reference Phillips, Wilson, Sun and Morey2018). The study was approved by the Israel Defense Forces Medical Corps Ethics Committee.
Table 1. Demographic characteristics by group
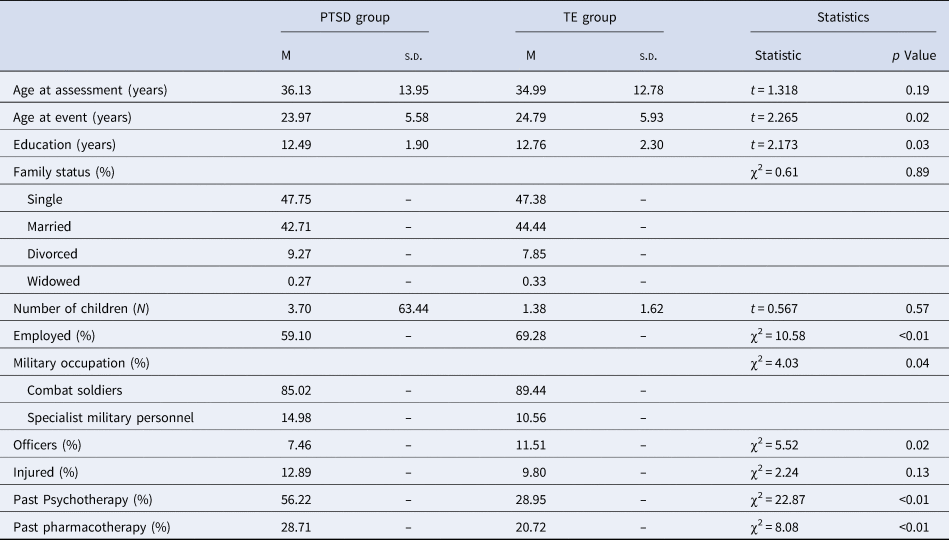
PTSD, posttraumatic stress disorder; TE, trauma-exposed.
Measures
Clinician-rated PTSD symptoms
Severity of PTSD symptoms was measured using CAPS-IV (Blake et al., Reference Blake, Weathers, Nagy, Kaloupek, Gusman, Charney and Keane1995), a 17-item structured interview used for diagnosing PTSD based on DSM-IV criteria, considered the gold standard in PTSD assessment. Each item/symptom is rated separately on frequency and intensity on a 0-to-4 scale, for an item total score of 0-to-8. Summed item scores yield an estimate of three symptom clusters (i.e. Cluster B, Re-experiencing; Cluster C, Avoidance, and Numbing; and Cluster D, Hyperarousal) and an overall PTSD severity score. The CAPS has excellent reliability, convergent and discriminant validity, diagnostic utility and sensitivity to clinical change in military veterans and other populations (Weathers et al., Reference Weathers, Keane and Davidson2001; Pupo et al., Reference Pupo, Jorge, Schoedl, Bressan, Andreoli, Mello and Mari2011). Cronbach's α in the current sample was 0.91.
Clinician-rated depressive symptoms
Clinician-evaluated levels of depressive symptoms were measured using the Montgomery–Asberg Depression Rating Scale (MADRS; Montgomery and Asberg, Reference Montgomery and Asberg1979), a 10-item questionnaire assessing core depressive symptoms during the past week, each rated on a 0-to-6 scale (no evidence to pervasive evidence). It has high inter-rater reliability, convergent validity, and similar rates of sensitivity to change compared to other measures of depression (Montgomery and Asberg, Reference Montgomery and Asberg1979; Khan et al., Reference Khan, Khan, Shankles and Polissar2002; Khan et al., Reference Khan, Brodhead and Kolts2004). Cronbach's α in the current sample was 0.90.
Functioning
Functioning in different life domains were assessed using the Psychotherapy Outcome Assessment and Monitoring System-Trauma Version (POAMS-TV) Assessment Questionnaire (Green et al., Reference Green, Lowry and Kopta2003). This self-report includes 10 items, each rated on a 0-to-5 scale (extreme distress/dissatisfaction to optimal functioning/satisfaction). A global functioning score is derived by averaging scores across items (Levi et al., Reference Levi, Bar-Haim, Kreiss and Fruchter2016; Levi et al., Reference Levi, Shoval-Zuckerman, Fruchter, Bibi, Bar-Haim and Wald2017). The POAMS-TV has been shown to be reliable in other samples of Israel Defense Forces veterans (Svetlicky et al., Reference Svetlicky, Solomon, Benbenishty, Levi and Lubin2010). Cronbach's α in the current sample was 0.81.
Data analysis
Network analysis
Network models estimating the associations between symptoms are usually constructed using the Graphical Gaussian Model (GGM; Epskamp and Fried, Reference Epskamp and Fried2016), through the R-package qgraph (Epskamp et al., Reference Epskamp, Cramer, Waldorp, Schmittmann and Borsboom2012). Within the graphical network, each node depicts a symptom, and edges depict regularized partial correlations between two symptom nodes. Line thickness reflects association strength; blue and red edges represent positive and negative associations, respectively.
Here, as we had two different samples of participants (i.e. PTSD patients and TE participants) for which we constructed the same network structure based on the CAPS 17-symptom-items, we used the fused graphical lasso (FGL; Danaher et al., Reference Danaher, Wang and Witten2014). The FGL is a network estimation technique, which is an extension of the graphical lasso, that allows estimating several GGMs jointly, yielding a more accurate estimation of network structures than estimating each network individually using separate GGMs (Danaher et al., Reference Danaher, Wang and Witten2014; Costantini and Epskamp, Reference Costantini and Epskamp2017; Fried et al., Reference Fried, Eidhof, Palic, Costantini, Huisman-van Dijk, Bockting, Engelhard, Armour, Nielsen and Karstoft2018). Thus, we used FGL to plot the network structure for the PTSD and TE samples using the CAPS 17-symptom-items (CAPS-items and TE-CAPS-items networks, respectively). For the PTSD sample, we used GGM to plot the network structure incorporating both the CAPS 17-symptom-items and the MADRS depressive symptom-items (CAPS + MADRS-items network), as this network was conducted only for the PTSD sample.
Node centrality measures
Within-network symptoms importance was characterized using the one-step expected influence (EI) centrality measure indexing node strength (Robinaugh et al., Reference Robinaugh, Millner and McNally2016), as it takes into account negative as well as positive edges (Wang et al., Reference Wang, Jones, Dreier, Elliott and Grilo2018). It is considered the most reliable, stable and accurate measure of node centrality compared with other centrality measures, such as betweenness and closeness (Fried et al., Reference Fried, Eidhof, Palic, Costantini, Huisman-van Dijk, Bockting, Engelhard, Armour, Nielsen and Karstoft2018; Wang et al., Reference Wang, Jones, Dreier, Elliott and Grilo2018), which were recently deemed less suitable as measures of node importance in psychological networks (Bringmann et al., Reference Bringmann, Elmer, Epskamp, Krause, Schoch, Wichers, Wigman and Snippe2018; Hallquist et al., Reference Hallquist, Wright and Molenaar2019).
EI analyses used the R-package bootnet (Epskamp et al., Reference Epskamp, Borsboom and Fried2018) and included the one-step expected influence measure (Robinaugh et al., Reference Robinaugh, Millner and McNally2016), which reflects the summed weight of positive and negative edges with the neighboring nodes in the network (Heeren et al., Reference Heeren, Jones and McNally2018). Expected influence was computed for all networks. For the CAPS + MADRS-items network, EI was also computed for bridge nodes (nodes which have symptom-level connections with nodes of the other disorder), reflecting between-disorder symptom associations (Jones et al., Reference Jones, Ma and McNally2019). For all measures, higher values of EI reflect greater node centrality (McNally, Reference McNally2016; Bringmann et al., Reference Bringmann, Elmer, Epskamp, Krause, Schoch, Wichers, Wigman and Snippe2018; Fried et al., Reference Fried, Eidhof, Palic, Costantini, Huisman-van Dijk, Bockting, Engelhard, Armour, Nielsen and Karstoft2018; Hallquist et al., Reference Hallquist, Wright and Molenaar2019).
Network robustness (accuracy and stability)
Network accuracy was computed using the R-package bootnet (Epskamp et al., Reference Epskamp, Borsboom and Fried2018) employing a non-parametric bootstrap approach. We estimated edge weights accuracy at a 95% confidence intervals (CIs) by sampling the data 1000 times (with replacement), thereby generating a distribution of edge weights. Network stability was assessed using a bootstrap person-dropping procedure (Costenbader and Valente, Reference Costenbader and Valente2003) providing a correlation stability (CS)-coefficient. While CS-coefficient ⩾0.25, and preferably ⩾0.50, reflects adequate network stability, those under 0.25 reflect insufficient network stability (Epskamp et al., Reference Epskamp, Borsboom and Fried2018).
Network comparison
For network comparison analysis we first randomly sampled 306 PTSD patients from the original PTSD sample to ensure an even number of participants in the PTSD and TE samples. Next, a Network Comparison Test (NCT R-package; van Borkulo and Millner, Reference van Borkulo and Millner2016) was used to compare the CAPS-items and TE-CAPS-items networks. We used an omnibus test evaluating invariance of the network structure across groups, and also tested the differences in global strength between the networks, comparing the sum of absolute edge weight values.
Directed acyclic graph (DAG) analysis
The DAG is a Bayesian network approach that models a network in which edges are directed and noncircular. Thus, the DAG gives us information about both the strength and the direction of connections between symptoms, which are regarded as clues as to which associations between symptoms might be causally important, that is, which symptoms may play a causal role in creating other symptoms (Jones et al., Reference Jones, Mair, Riemann, Mugno and McNally2018). Here, a Bayesian network analysis applying the R-package bnlearn (Scutari, Reference Scutari2010) was used to produce DAGs aiming to discern directionality among nodes from cross-sectional data (McNally, Reference McNally2016). For the present study, we used the completed partially DAG (CPDAG) which accounts for some of the shortcomings of equivalent separate DAGs (Scutari and Denis, Reference Scutari and Denis2014). DAG analysis considered CAPS and MADRS single-items as well as the daily functioning total score.
See Supplemental Material for a more comprehensive description of data analyses including the original analytic code (R-scripts) and the underlying co-variance matrices of the different presented networks.
Results
Psychopathology measures
Mean scores of the PTSD sample were 81.77 (s.d. = 17.21) for the CAPS, 29.23 (s.d. = 10.825) for the MADRS, and 2.07 (s.d. = 0.74) for the POAMS-TV. In the trauma-exposed (TE) treatment-seeking patients not meeting full criteria for PTSD sample, mean scores were 33.95 (s.d. = 18.26), 25.31 (s.d. = 9.68), and 2.67 (s.d. = 0.76), respectively. Independent-samples t tests revealed significant group differences on all measures, all ps<0.0001. Of the PTSD group, 57% also met diagnostic criteria for MDD (i.e. a PTSD-MDD comorbidity), while only 32% of the TE sample met MDD criteria.
CAPS-items network
The CAPS-items network of the PTSD sample is depicted in Fig. 1a (for the TE-CAPS-items network see Fig. 1b and section below). The stability of the estimated network (CS-coefficient) was 0.75 for expected influence (Fig. 2a), reflecting a highly stable network. For edge-weight accuracy, see Fig. 2b.

Fig. 1. Symptom-items Networks. (a) The clinician-administered PTSD Scale (CAPS-IV) 17-symptom-items network among PTSD patients (CAPS-items network). Positive edges appear in blue and negative appear in red. The stronger and saturated edges represent stronger regularized partial correlations. The item label abbreviations for the CAPS are: AMN, amnesia; ANG, irritability; AVS, avoidance of situations; AVT, avoidance of thoughts; CON, concentration deficits; DIS, disinterest in activities; DRE, nightmares; DTA, detachment from others; FLA, flashbacks; FUT, foreshortened future; HYP, hypervigilance; INT, intrusions; NUM, emotional numbing; PHY, physiological reactivity; SLE, sleep disturbance; STR, startle response; and UPS, upset by reminders. (b) The clinician-administered PTSD Scale (CAPS-IV) 17-symptom-items network among treatment-seeking patients who did not meet full criteria for PTSD (TE-CAPS-items network). Positive edges appear in blue and negative appear in red. The stronger and saturated edges represent stronger regularized partial correlations. The item label abbreviations for the CAPS are the same. (c) The network of the clinician-administered PTSD Scale (CAPS-IV) 17-symptom-items combined with the 10-symptom-items of the clinician-rated Montgomery–Asberg Depression Rating Scale (MADRS) among PTSD patients. The items from the CAPS-IV are colored red and the items from the MADRS are colored blue (CAPS + MADRS-items network). Positive edges appear in blue and negative appear in red. The stronger and saturated edges represent stronger regularized partial correlations. The item label abbreviations for the CAPS (red circles) are: AMNES, amnesia; ANGER, irritability; AVSIT, avoidance of situations; AVTHT, avoidance of thoughts; CONC, concentration deficits; DISINT, disinterest in activities; DREAM, nightmares; DTACH, detachment from others; FLASH, flashbacks; FUTRE, foreshortened future; HYPER, hypervigilance; INTRU, intrusions; NUMB, emotional numbing; PHY, physiological reactivity; SLEEP, sleep disturbance; STRTL, startle response; and UPSET, upset by reminders. The item abbreviation labels for the MADRS (blue circles) are: APPSAD, apparent sadness; REPSAD, reported sadness; TENSION, Inner Tension; RESLEEP, reduced sleep; REAPP, reduced appetite; CONCDIFF, concentration difficulties, LASS, lassitude; FEEL, inability to feel; PESS, pessimistic thoughts; SUI, suicidal thoughts.

Fig. 2. Network Robustness Measures (Accuracy and Stability). (a) Bootstrapped node expected influence of the clinician-administered PTSD Scale (CAPS-IV) 17-symptom-items network among PTSD patients. (b) Bootstrapped confidence intervals of all edge weights of the clinician-administered PTSD Scale (CAPS-IV) 17-symptom-items network among PTSD patients. The red line represents sample values, the black line represents bootstrap means, and the gray area is the bootstrapped CIs. Each horizontal line represents one edge of the network, ordered from the edge with the highest edge-weight to the edge with the lowest edge-weight.
The strongest edges were physiological reactivity by trauma reminders (B5)-to-feelings of detachment from others (C5); physiological reactivity by trauma reminders (B5)-to- getting emotionally upset by trauma reminders (B4); getting emotionally upset by trauma reminders (B4)-to-intrusive thoughts (B1); and flashbacks (B3)-to-avoidance of thoughts (C1).
The standardized estimate of the expected influence centrality measure is depicted in Fig. 3. Flashbacks (B3) and getting emotionally upset by trauma reminders (B4) emerged as the most influential symptoms, with amnesia (C3) and irritability (D2) emerging as the least influential.

Fig. 3. Network Centrality – Expected Influence. The expected influence measure for the clinician-administered PTSD Scale (CAPS-IV) 17-symptom-items network among PTSD patients.
See online Supplementary Figs S1 and S2 for significant tests between edge weights and nodes, respectively.
CAPS + MADRS-items network
CAPS and MADRS items formed two separate clusters within the co-morbidity network (Fig. 1c). Network stability remained high for expected influence (0.75; online Supplementary Fig. S3A) reflecting a highly stable network. For edge-weight accuracy, see online Supplementary Fig. S3B.
Within the PTSD cluster, same edges as in the CAPS-item network emerged as strongest. Within the depressive symptoms cluster, strongest edges were suicidality (Q10)-to-pessimistic thoughts (Q9); reported sadness (Q2)-to-apparent sadness (Q1); and lassitude (Q7)-to-concentration difficulties (Q6).
The standardized estimate of expected influence for the CAPS + MADRS-items network is depicted in online Supplementary Fig. S4. Getting emotionally upset by trauma reminders (B4) and flashbacks (B3) emerged once more as the two most influential PTSD symptoms in the network. Depressive symptoms of reported sadness (Q2), lassitude (Q7), and inner tension (Q3) also demonstrated high expected influence. As in the CAPS-item network, amnesia (C3) and irritability (D2) were found to be the lowest on EI. Reduced appetite (Q5) and detachment from others (C5) also demonstrated low EI.
See online Supplementary Figs S5 and S6 for significant tests between edge weights and nodes, respectively.
Considering only bridge-nodes (online Supplementary Fig. S7), results showed that the most influential bridge-nodes were (depression-related) reduced sleep (Q4), reported sadness (Q2), inner tension (Q3), (PTSD-related) disturbed sleep (D1), avoidance of thoughts (C1), and getting emotionally upset by trauma reminders (B4). Reduced appetite (Q5) and physiological reactions from trauma reminders (B5) were the least influential bridge-nodes.
TE-CAPS-items network (v. CAPS-items network)
The stability of the TE network showed a lower CS-coefficient of 0.52 compared with the PTSD-related networks (0.75 for both the CAPS-items and the CAPS + MADRS-items). However, this score is still above the recommended score of 0.5, reflecting a stable enough network (online Supplementary Fig. S8A). For edge-weight accuracy, online Supplementary Fig. S8B.
The strongest positive edges in the TE network were intrusive thoughts (B1)-to-flashbacks (B3); anhedonia (C4)-to-detachment from others (C5); irritability (D2)-to-concentration deficits (D3); and hypervigilance (D4)-to-startle response (D5).
The standardized estimate of the expected influence centrality measure is depicted in online Supplementary Fig. S9. Intrusive thoughts (B1), flashbacks (B3), irritability (D2), and nightmares (B2) were the strongest nodes, with the weakest being amnesia (C3), foreshortened future (C7), and disturbed sleep (D1).
See online Supplementary Figs S10 and S11 for significant tests between edge weights and nodes, respectively.
The two networks differed significantly on structure (p = 0.0018) and global strength (p = 0.0262). As seen in Fig. 1, the network structure of the PTSD sample (Fig. 1a) is more interconnected relative to the TE sample (Fig. 1b).
Directed acyclic graph (DAG) analysis
In the DAG analysis (Fig. 4), flashbacks (B3) emerged once more as the most pivotal network node. While not being triggered by any higher located nodes, it triggered nine other PTSD symptoms, with the strongest edges being with intrusive thoughts (B1) and physiological reactivity by trauma reminders (B5), with all three triggering getting emotionally upset by trauma reminders (B4), hypervigilance (D4), and avoiding trauma-related thoughts (C1). The most downstream PTSD symptoms (i.e. triggered nodes that trigger no other nodes) were nightmares (B2), irritability (D2) and foreshortened future (C7) with concentration difficulties (D3) being mostly triggered by other nodes.

Fig. 4. Directed Acyclic Graph (DAG). Directed acyclic graph (DAG) of the clinician-administered PTSD Scale (CAPS-IV) 17-symptom-items (PTSD), the clinician-rated Montgomery–Asberg Depression Rating Scale (MADRS) 10-symptom-items (depression), and the psychotherapy outcome assessment and monitoring system-trauma version (POAMS-TV) total score reflecting overall functioning levels (functioning) among PTSD patients. Nodes represent symptoms and edges represent directed connections between symptoms. The item label abbreviations for the CAPS are: AMNES, amnesia; ANGER, irritability; AVSIT, avoidance of situations; AVTHT, avoidance of thoughts; CONC, concentration deficits; DISINT, disinterest in activities; DREAM, nightmares; DTACH, detachment from others; FLASH, flashbacks; FUTRE, foreshortened future; HYPER, hypervigilance; INTRU, intrusions; NUMB, emotional numbing; PHY, physiological reactivity; SLEEP, sleep disturbance; STRTL, startle response; and UPSET, upset by reminders. The item abbreviation labels for the MADRS are: APPSAD, apparent sadness; REPSAD, reported sadness; TENSION, Inner Tension; RESLEEP, reduced sleep; REAPP, reduced appetite; CONCDIFF, concentration difficulties, LASS, lassitude; FEEL, inability to feel; PESS, pessimistic thoughts; SUI, suicidal thoughts. The item abbreviation label for the POAMS-TV is FUNC.
Of note, PTSD and depressive symptoms, while showing some between-disorders edges in the DAG, were once more clustered separately from each other. Importantly, most of the existing between-disorders edges were PTSD-to-depressive symptoms edges, with almost none of the opposite direction. Daily functioning was directly influenced by several PTSD-related symptoms, including disturbed sleep (D1), anhedonia (C4), and numbness (C6), but only by one depressive symptom of reduced sleep (Q4). In turn, daily functioning showed a triggering effect on foreshortened future (C7), concentration difficulties (D3), and irritability (D2), all PTSD-related symptoms.
Discussion
This study examined PTSD network structure in treatment-seeking veterans with PTSD (n = 1489). To our knowledge, this is the largest single-sample PTSD network study, and the first to include participants meeting full diagnostic criteria for PTSD, while basing network structure exclusively on clinician-administered measures. Network structure was further assessed after introducing depressive symptoms, and again using a DAG analysis, including also depressive symptoms and a functioning measure, to better discern association directionality (McNally, Reference McNally2016; McNally et al., Reference McNally, Heeren and Robinaugh2017a).
Flashback and getting emotionally upset by trauma reminders emerged as the most central symptoms across all PTSD-related networks, highlighting their importance in the clinical presentation of PTSD among treatment-seeking veterans, echoing prior results in various populations (Spiller et al., Reference Spiller, Schick, Schnyder, Bryant, Nickerson and Morina2017; Afzali et al., Reference Afzali, Sunderland, Batterham, Carragher, Calear and Slade2017a; Epskamp et al., Reference Epskamp, Borsboom and Fried2018; Fried et al., Reference Fried, Eidhof, Palic, Costantini, Huisman-van Dijk, Bockting, Engelhard, Armour, Nielsen and Karstoft2018; Greene et al., Reference Greene, Gelkopf, Epskamp and Fried2018), including veterans (Armour et al., Reference Armour, Fried, Deserno, Tsai and Pietrzak2017; Mitchell et al., Reference Mitchell, Wolf, Bovin, Lee, Green, Rosen, Keane and Marx2017; Fried et al., Reference Fried, Eidhof, Palic, Costantini, Huisman-van Dijk, Bockting, Engelhard, Armour, Nielsen and Karstoft2018; von Stockert et al., Reference von Stockert, Fried, Armour and Pietrzak2018), for which their clinical importance was specifically emphasized (Armour et al., Reference Armour, Fried, Deserno, Tsai and Pietrzak2017). Amnesia emerged as the least central symptom across all networks, reaffirming previous findings (Birkeland and Heir, Reference Birkeland and Heir2017; Bryant et al., Reference Bryant, Creamer, O'Donnell, Forbes, McFarlane, Silove and Hadzi-Pavlovic2017; Mitchell et al., Reference Mitchell, Wolf, Bovin, Lee, Green, Rosen, Keane and Marx2017; Spiller et al., Reference Spiller, Schick, Schnyder, Bryant, Nickerson and Morina2017; Afzali et al., Reference Afzali, Sunderland, Batterham, Carragher, Calear and Slade2017a; McNally et al., Reference McNally, Heeren and Robinaugh2017a; Fried et al., Reference Fried, Eidhof, Palic, Costantini, Huisman-van Dijk, Bockting, Engelhard, Armour, Nielsen and Karstoft2018; Moshier et al., Reference Moshier, Bovin, Gay, Wisco, Mitchell, Lee, Sloan, Weathers, Schnurr, Keane and Marx2018; Phillips et al., Reference Phillips, Wilson, Sun and Morey2018; Ross et al., Reference Ross, Murphy and Armour2018; von Stockert et al., Reference von Stockert, Fried, Armour and Pietrzak2018), which have led some to question amnesia as a core PTSD symptom (Armour et al., Reference Armour, Fried, Deserno, Tsai and Pietrzak2017). Low centrality of amnesia is also in line with factor-analysis studies (Yufik and Simms, Reference Yufik and Simms2010; Armour et al., Reference Armour, Tsai, Durham, Charak, Biehn, Elhai and Pietrzak2015; Forbes et al., Reference Forbes, Lockwood, Elhai, Creamer, Bryant, McFarlane, Silove, Miller, Nickerson and O'Donnell2015) and memory research demonstrating similarities in the clearness and vividness of traumatic and non-traumatic memories, with the former found to be more potent amongst PTSD patients compared with trauma-exposed-non-PTSD individuals (Porter and Birt, Reference Porter and Birt2001; Megias et al., Reference Megias, Ryan, Vaquero and Frese2007; Ross et al., Reference Ross, Murphy and Armour2018).
The physiological reactivity by trauma reminders-to-getting emotionally upset by trauma reminders-to-intrusive thoughts edges (all re-experiencing symptoms), as well as three additional edges involving re-experiencing nodes (physiological reactivity by trauma reminders-to-detachment, flashbacks-to-avoidance of thoughts, and physiological reactivity by trauma reminders-to-startle response) were found to be of the strongest in the network. The DAG analysis further underscored the importance of re-experiencing symptoms, as physiological reactivity by trauma reminders and intrusive thoughts emerged as additional influential nodes, with flashbacks found to drive both these symptoms, with all three driving getting emotionally upset by trauma reminders. These results are in line with previous networks studies emphasizing the importance of re-experiencing symptoms in PTSD (Knefel et al., Reference Knefel, Tran and Lueger-Schuster2016; Armour et al., Reference Armour, Fried, Deserno, Tsai and Pietrzak2017; Bryant et al., Reference Bryant, Creamer, O'Donnell, Forbes, McFarlane, Silove and Hadzi-Pavlovic2017) and with research using structural equation modeling reporting a positive association between trauma-exposure and re-experiencing symptoms severity in veterans (Miller et al., Reference Miller, Wolf, Martin, Kaloupek and Keane2008). The importance of re-experiencing symptoms as maladaptive reactivity to trauma-reminders in the symptom structure of PTSD is also in accordance with early and more recent theoretical accounts of PTSD (Brewin and Holmes, Reference Brewin and Holmes2003). For example, the stress response theory (Horowitz, Reference Horowitz1976; Horowitz, Reference Horowitz1986), one of the earliest theoretical formulations of PTSD, suggests that a failure in assimilating an overflow of new trauma-related information with prior knowledge results in persistent posttraumatic reactions, as trauma memories continuously break into consciousness (i.e. re-experiencing symptoms such as intrusions and flashbacks). As a defensive response, the individual employs avoidance of trauma reminders. Thus, trauma information continues to repeatedly intrude and be avoided, creating a re-experiencing and avoidance cycle (Horowitz, Reference Horowitz1986). Interestingly, in addition to the importance of re-experiencing symptoms in the PTSD network structure, results of the DAG analysis further showed most avoidance symptoms (e.g. avoidance of thoughts, anhedonia, detachment) to be directly triggered mostly by re-experiencing symptoms (or by other avoidance symptoms connected to re-experiencing symptoms), echoing the suggestion of the stress response theory. A more recent theory, the dual representation theory of PTSD (Brewin et al., Reference Brewin, Dalgleish and Joseph1996; Brewin, Reference Brewin2001; Brewin et al., Reference Brewin, Gregory, Lipton and Burgess2010), also assign importance to re-experiencing symptoms in PTSD. According to this theory, posttraumatic pathological responses, and especially uncontrollable re-experiencing symptoms, emerge when traumatic memories are dissociated and stored separately from ordinary memories. Specifically, trauma stimuli that receive insufficient processing to be stored as regular autobiographical memories in the ‘verbally accessible memory’ (VAM), are stored instead in the parallel operating, but separate, image-based ‘situationally accessibly memory’ (SAM) system. In the context of trauma reminders, these trauma-related memories give rise to uncontrollable intrusions and to maladaptive psychological and physiological responses (Brewin and Holmes, Reference Brewin and Holmes2003). Another recent theory acknowledging the importance of re-experiencing symptoms in PTSD is Ehlers and Clark's cognitive model (Ehlers and Clark, Reference Ehlers and Clark2000). Per this model, a pathological reaction following a traumatic event unfolds when the processing of traumatic information produces a continuous current sense of threat. As in the dual process theory, a trauma-related memory that lacks elaboration, that is not given a complete context, and that is inadequately integrated into the autobiographical memory system results in a memory that is hard to retrieve intentionally, leading to re-experiencing symptoms. The model further suggests that retrieval of trauma-related information from the associative memory system is mainly cue-driven and unintentional, priming the individual to trauma-reminders and leading directly to re-experiencing symptoms in response to relevant cues (Brewin and Holmes, Reference Brewin and Holmes2003). Importantly, in comparison to avoidance and hyperarousal symptoms, also implicated in other psychopathologies (Joiner et al., Reference Joiner, Steer, Beck, Schmidt, Rudd and Catanzaro1999; Chawla and Ostafin, Reference Chawla and Ostafin2007), re-experiencing symptoms are regarded as more unique features of PTSD, less overlapping with other disorders (Chemtob et al., Reference Chemtob, Roitblat, Hamada, Carlson and Twentyman1988; Brewin and Holmes, Reference Brewin and Holmes2003; Ehlers, Reference Ehlers2015). Thus, in conjunction with previous findings and theories, current results could be interpreted as suggesting re-experiencing symptoms, and especially flashbacks and getting emotionally upset by trauma reminders, as potential treatment targets for veterans with PTSD. Still, as the present study is cross-sectional in nature, this possibility should be taken with cautious and rigorously explored in future studies (Fried and Cramer, Reference Fried and Cramer2017; Dablander and Hinne, Reference Dablander and Hinne2018; Phillips et al., Reference Phillips, Wilson, Sun and Morey2018).
The comorbidity network revealed several important results. First, a distinct clustering of PTSD and depressive symptoms emerged, creating two discrete sub-networks. This differential clustering is in line with previous network research showing similar clustering when including depressive symptom as single nodes (Mitchell et al., Reference Mitchell, Wolf, Bovin, Lee, Green, Rosen, Keane and Marx2017), and no change in the PTSD network structure after including depression as a single-node covariate (Armour et al., Reference Armour, Fried, Deserno, Tsai and Pietrzak2017). Second, same edges emerged as strongest in the CAPS-items network and within the PTSD cluster of the CAPS + MADRS-items network, suggesting minimal influence for the introduction of depression items on PTSD symptoms connectedness. Also, only slight changes in node centrality of PTSD symptoms within the co-morbid network were found, with flashbacks and getting emotionally upset by trauma reminders remaining the most central nodes, and amnesia and irritability the least central nodes. Finally, the DAG analysis yielded the same distinct clustering of PTSD and depressive symptoms. Together with findings of previous network studies, current results suggest that within-disorder (i.e. PTSD and depression) symptom connectivity is greater than between-disorder connections, even when co-occurrence of disorders is high. This suggestion is in accordance with previous research exploring the PTSD-MDD comorbidity using different analytic approaches, which supported the notion that while PTSD and MDD are highly correlated among traumatized individuals, they are also two distinct, independent and distinguishable constructs/responses to traumatic events (Blanchard et al., Reference Blanchard, Buckley, Hickling and Taylor1998; Grant et al., Reference Grant, Beck, Marques, Palyo and Clapp2008; Gros et al., Reference Gros, Simms and Acierno2010; Post et al., Reference Post, Zoellner, Youngstrom and Feeny2011). Clinically, these findings might suggest that treatment of co-morbid PTSD-MDD should include components specifically addressing each pathology. Indeed, the above-cited research suggested to incorporate specific evidence-based treatment components for MDD into extant PTSD protocols to enhance treatment outcomes (Gros et al., Reference Gros, Simms and Acierno2010), as PTSD-MDD patients, compared to patients with PTSD alone, exhibit poorer treatment response following PTSD-focused treatment (Bernardy and Friedman, Reference Bernardy and Friedman2015), necessitating novel treatment strategies for PTSD-MDD (Flory and Yehuda, Reference Flory and Yehuda2015).
Examining bridge nodes to explore between-disorders connections revealed that sleep difficulties per PTSD and depression emerged as major bridging nodes, replicating previous network studies (Mitchell et al., Reference Mitchell, Wolf, Bovin, Lee, Green, Rosen, Keane and Marx2017; Afzali et al., Reference Afzali, Sunderland, Teesson, Carragher, Mills and Slade2017b). This fact is not surprising considering the high comorbidity rates of sleep disturbances with psychopathology in general, and more specifically with anxiety- and depression-related pathologies, with about 50–80% of psychiatric patients reporting sleep difficulties (Morin and Ware, Reference Morin and Ware1996). Moreover, many DSM-based diagnoses, including PTSD and depression, entail sleep difficulties as one of their potential symptoms (American Psychiatric Association, 2000, 2013). Thus, the bridging role of sleep difficulties is in line with research considering overlapping symptoms as origins of high comorbidity in PTSD (Boschloo et al., Reference Boschloo, van Borkulo, Rhemtulla, Keyes, Borsboom and Schoevers2015; Afzali et al., Reference Afzali, Sunderland, Teesson, Carragher, Mills and Slade2017b), supporting the notion that the PTSD-MDD comorbidity might reflect imprecisions in symptom classification into two discrete categorical diagnoses, suggesting a nosology artifact (Flory and Yehuda, Reference Flory and Yehuda2015). However, avoidance of thoughts as well as getting emotionally upset by trauma reminders also emerged as a key bridge-nodes, replicating previous findings (Mitchell et al., Reference Mitchell, Wolf, Bovin, Lee, Green, Rosen, Keane and Marx2017). Importantly, these symptoms are not overlapping symptoms, reflecting more unique pathways between PTSD and depression. Indeed, research has shown that removing overlapping symptoms from network analysis does not necessarily collapse the comorbidity networks (Afzali et al., Reference Afzali, Sunderland, Batterham, Carragher, Calear and Slade2017a, Reference Afzali, Sunderland, Teesson, Carragher, Mills and Slade2017b), and that overlapping symptoms cannot exclusive explain the PTSD-MDD association (Blanchard et al., Reference Blanchard, Buckley, Hickling and Taylor1998; Elhai et al., Reference Elhai, Grubaugh, Kashdan and Frueh2008; Elhai et al., Reference Elhai, Carvalho, Miguel, Palmieri, Primi and Frueh2011; Boschloo et al., Reference Boschloo, van Borkulo, Rhemtulla, Keyes, Borsboom and Schoevers2015). Thus, the high centrality of non-overlapping bridge symptoms supports an alternative conceptualization of the PTSD-MDD comorbidity, namely, that this comorbidity is not a nosology artifact, but rather a distinct trauma-related phenotype, or even a subtype of PTSD, reflecting a fundamental dimension of risk for psychopathology following trauma exposure (Flory and Yehuda, Reference Flory and Yehuda2015). On a more speculative note, current findings can be also viewed in light of research examining the temporal order of PTSD and MDD, in general and more specifically in combat-related PTSD, trying to ascertain whether pre-existing MDD is a risk factor for developing PTSD following trauma-exposure, whether MDD is a reaction to PTSD, considering PTSD as a risk factor for MDD, or whether there is a more complex, bi-directional relationship between PTSD and MDD, with shared risk factors and vulnerabilities (Stander et al., Reference Stander, Thomsen and Highfill-McRoy2014; Angelakis and Nixon, Reference Angelakis and Nixon2015). While not addressing these options directly, due to the cross-sectional nature of our data, the differential clustering of PTSD and depressive symptoms in the CAPS + MADRS network, the results of the DAG analysis showing a similar differential clustering of PTSD and depressive symptoms, and the emergence of significant non-overlapping bridge symptoms, all allude to the conceptualization of the PTSD-MDD comorbidity as a trauma-related reaction comprised of mutual influential, yet distinct, symptom structures.
Addressing functioning in network analyses is important as the DSM classification system specifically requires significant impairment in functioning for a PTSD diagnosis (Ross et al., Reference Ross, Murphy and Armour2018). The DAG network revealed that functioning was directly triggered by the PTSD symptoms of disturbed sleep, anhedonia, and emotional numbness, and by one overlapping depressive symptom of reduced sleep. This is in line with research showing that sleep disturbances independently aggravate PTSD daytime symptoms, contributing to poorer functioning (DeViva et al., Reference DeViva, Zayfert and Mellman2004; Germain et al., Reference Germain, Buysse and Nofzinger2008), and that anhedonia and emotional numbness lead to deficient social activity and restriction of psychological resources in PTSD (Kashdan et al., Reference Kashdan, Elhai and Frueh2006, Reference Kashdan, Elhai and Frueh2007). Our results also align with the only network study that focused specifically on functional impairment in veterans with PTSD showing anhedonia to have the highest bridge centrality/EI measure (Ross et al., Reference Ross, Murphy and Armour2018). Thus, it seems that impaired functioning is directly influenced by depressive-like PTSD symptoms.
The network structure of the trauma-exposed (TE) treatment-seeking patients not meeting full criteria for PTSD was markedly less connected compared to the PTSD network, with different central edges and nodes, replicating a previous network study conducting similar analyses comparing US veterans with PTSD and subthreshold PTSD (Phillips et al., Reference Phillips, Wilson, Sun and Morey2018). This aligns with the networks approach's theoretical framing of psychopathology, namely, that a certain disorder will transpire when the required number of symptoms are activated for a sufficient duration, reaching the disorder's ‘tipping point’ (van de Leemput et al., Reference van de Leemput, Wichers, Cramer, Borsboom, Tuerlinckx, Kuppens, van Nes, Viechtbauer, Giltay, Aggen, Derom, Jacobs, Kendler, van der Maas, Neale, Peeters, Thiery, Zachar and Scheffer2014; Hofmann et al., Reference Hofmann, Curtiss and McNally2016; McNally, Reference McNally2016, Reference McNally2017). Still, this trauma-exposed population should not be taken lightly, especially among treatment-seeking veterans. Research has shown the detrimental effects of sub-threshold PTSD (Stein et al., Reference Stein, Walker, Hazen and Forde1997; Mylle and Maes, Reference Mylle and Maes2004; Jakupcak et al., Reference Jakupcak, Conybeare, Phelps, Hunt, Holmes, Felker, Klevens and McFall2007; Pietrzak et al., Reference Pietrzak, Goldstein, Southwick and Grant2011; Mitchell et al., Reference Mitchell, Mazzeo, Schlesinger, Brewerton and Smith2012; Schnurr, Reference Schnurr2014; Jung et al., Reference Jung, Chang and Kim2016), suggesting it as a risk condition at the prodromal phase of PTSD (Schnurr, Reference Schnurr2014). Current results could assist therapists treating treatment-seeking trauma-exposed veterans with no ‘formal’ PTSD diagnosis by highlighting the need to address specific symptoms and connection between symptoms, which are different from those characterizing PTSD patients, aiming to weaken the corresponding network, and, potentially, halt the progression towards clinical PTSD.
While our findings corroborate previous research in PTSD, differences also emerged, potentially due to differences in various methodological features. This has been acknowledged by most network studies which have implicated potential differences in sample size, type (treatment-seeking, survey data), and status (clinical, probable, sub-threshold, trauma-exposed), and PTSD measures (self-report, clinician-administered) used for network construction (Armour et al., Reference Armour, Fried, Deserno, Tsai and Pietrzak2017; Birkeland and Heir, Reference Birkeland and Heir2017; Bryant et al., Reference Bryant, Creamer, O'Donnell, Forbes, McFarlane, Silove and Hadzi-Pavlovic2017; Mitchell et al., Reference Mitchell, Wolf, Bovin, Lee, Green, Rosen, Keane and Marx2017; Spiller et al., Reference Spiller, Schick, Schnyder, Bryant, Nickerson and Morina2017; McNally et al., Reference McNally, Heeren and Robinaugh2017a; Fried et al., Reference Fried, Eidhof, Palic, Costantini, Huisman-van Dijk, Bockting, Engelhard, Armour, Nielsen and Karstoft2018; Ross et al., Reference Ross, Murphy and Armour2018; Sullivan et al., Reference Sullivan, Smith, Lewis and Jones2018) in results heterogeneity. Gender may also contribute to observed differences (Mitchell et al., Reference Mitchell, Wolf, Bovin, Lee, Green, Rosen, Keane and Marx2017), especially as we have explored an all-male sample. Finally, trauma type has been suggested as influencing PTSD expression/presentation (Norris et al., Reference Norris, Friedman and Watson2002a, Reference Norris, Friedman, Watson, Byrne, Diaz and Kaniasty2002b; Chung and Breslau, Reference Chung and Breslau2008; Kelley et al., Reference Kelley, Weathers, McDevitt-Murphy, Eakin and Flood2009; McNally, Reference McNally2009), and, accordingly, network structure (Armour et al., Reference Armour, Fried, Deserno, Tsai and Pietrzak2017; Birkeland and Heir, Reference Birkeland and Heir2017; Phillips et al., Reference Phillips, Wilson, Sun and Morey2018; Ross et al., Reference Ross, Murphy and Armour2018; Sullivan et al., Reference Sullivan, Smith, Lewis and Jones2018; von Stockert et al., Reference von Stockert, Fried, Armour and Pietrzak2018). Indeed, comparing networks across three different traumatic events revealed corresponding differences in network structures (Benfer et al., Reference Benfer, Bardeen, Cero, Kramer, Whiteman, Rogers, Silverstein and Weathers2018). Importantly, while seven studies explored veteran samples (Armour et al., Reference Armour, Fried, Deserno, Tsai and Pietrzak2017; Mitchell et al., Reference Mitchell, Wolf, Bovin, Lee, Green, Rosen, Keane and Marx2017; Fried et al., Reference Fried, Eidhof, Palic, Costantini, Huisman-van Dijk, Bockting, Engelhard, Armour, Nielsen and Karstoft2018; Moshier et al., Reference Moshier, Bovin, Gay, Wisco, Mitchell, Lee, Sloan, Weathers, Schnurr, Keane and Marx2018; Phillips et al., Reference Phillips, Wilson, Sun and Morey2018; Ross et al., Reference Ross, Murphy and Armour2018; von Stockert et al., Reference von Stockert, Fried, Armour and Pietrzak2018), trauma was restricted to being combat- or deployment-related only in three (Mitchell et al., Reference Mitchell, Wolf, Bovin, Lee, Green, Rosen, Keane and Marx2017; Fried et al., Reference Fried, Eidhof, Palic, Costantini, Huisman-van Dijk, Bockting, Engelhard, Armour, Nielsen and Karstoft2018; Phillips et al., Reference Phillips, Wilson, Sun and Morey2018), with results being mostly similar to the present study, namely, high centrality of getting emotionally upset by trauma reminders and low centrality for amnesia and irritability.
Limitations should be considered. First, data from this study is cross-sectional and as such cannot directly examine the temporal nature and directed influences of associations between symptoms (Fried and Cramer, Reference Fried and Cramer2017; Dablander and Hinne, Reference Dablander and Hinne2018; Phillips et al., Reference Phillips, Wilson, Sun and Morey2018). While trying to address this issue using the DAG analysis, which provides preliminary clues of directionality in cross-sectional data, DAG analysis has some strict assumptions curbing potential inferences (McNally et al., Reference McNally, Heeren and Robinaugh2017a, Reference McNally, Mair, Mugno and Riemann2017b). Primarily, for psychopathology network, including DAG, one must assume that no important variable has been omitted from the network (Jones et al., Reference Jones, Heeren and McNally2017). While attempting to address this by including depressive symptoms and a functioning measure, many other non-symptoms may play a causal role in PTSD, as well as in bridging PTSD and other disorders (Jones et al., Reference Jones, Heeren and McNally2017). These may include, among others, threat-related cognitive biases (Lazarov et al., Reference Lazarov, Suarez-Jimenez, Abend, Naim, Shvil, Helpman, Zhu, Papini, Duroski, Rom, Schneier, Pine, Bar-Haim and Neria2018, Reference Lazarov, Suarez-Jimenez, Tamman, Falzon, Zhu, Edmondson and Neria2019), neural abnormalities (O'Doherty et al., Reference O'Doherty, Chitty, Saddiqui, Bennett and Lagopoulos2015; Koch et al., Reference Koch, van Zuiden, Nawijn, Frijling, Veltman and Olff2016), social functioning (Wilcox, Reference Wilcox2010), and other factors which have been implicated in PTSD such as age (Konnert and Wong, Reference Konnert and Wong2015) and gender (Lehavot et al., Reference Lehavot, Katon, Chen, Fortney and Simpson2018). Thus, future network-based research should include additional non-symptom nodes to enrich our understanding of PTSD (Jones et al., Reference Jones, Heeren and McNally2017). In addition, one must also assume that there are no feedback loops between symptoms, as in DAG activation flow is uni-directional. While thick edges (as those connecting re-experiencing symptoms; see Fig. 4) increase our confidence in directionality, future longitudinal studies could better assist in clarifying temporal sequences and potential causal relations between symptoms (Bringmann et al., Reference Bringmann, Vissers, Wichers, Geschwind, Kuppens, Peeters, Borsboom and Tuerlinckx2013; Bryant et al., Reference Bryant, Creamer, O'Donnell, Forbes, McFarlane, Silove and Hadzi-Pavlovic2017; Greene et al., Reference Greene, Gelkopf, Epskamp and Fried2018). Second, the PTSD-related network structures were modeled using the CAPS 17-symptom-items, which are the same symptoms/items used to select/create the study groups (i.e. PTSD and TE samples). Importantly, when using covariance-based methods, this selection might bias the resultant covariance structure leading to spurious correlations, a bias known as Berkson's bias (Cole et al., Reference Cole, Platt, Schisterman, Chu, Westreich, Richardson and Poole2010; Berkson, Reference Berkson2014; Phillips et al., Reference Phillips, Wilson, Sun and Morey2018; de Ron et al., Reference de Ron, Fried and Epskamp2019). Although there is currently not a clear best-practice guide for how to deal with this in network analysis in psychopathology-related research (Phillips et al., Reference Phillips, Wilson, Sun and Morey2018), we wish to emphasize that selection per PTSD diagnosis was established based on the relatively lenient F1/I2 item rule (i.e. Frequency ⩾ 1, Intensity ⩾ 2), and not on a clinical significance cutoff score (Blake et al., Reference Blake, Weathers, Nagy, Kaloupek, Gusman, Charney and Keane1995). Third, the present study used DSM-IV criteria in diagnosing PTSD and in constructing the different PTSD-related networks. Importantly, in DSM-5 three additional symptoms were added (for a total of 20 symptoms as opposed to the 17 symptoms in DSM-IV) with the addition of a forth symptom-cluster, following the division of the DSM-IV avoidance cluster into avoidance and negative alterations in cognitions and mood associated with the traumatic event (American Psychiatric Association, 2013; Hoge et al., Reference Hoge, Riviere, Wilk, Herrell and Weathers2014). Specifically, the negative alterations in cognitions and mood symptom-cluster introduces three ‘new’ depressive-like symptoms which were not included in DSM-IV, namely, negative feeling about oneself, others and the world (Symptom D2), self-blame (Symptom D3), and persistent negative emotional state (Symptom D4). Thus, constructing the PTSD-MDD comorbidity network using DSM-5 might have yielded different results. Still, as network analysis is data-driven, including depressive symptoms and a functioning measure provide vital information on symptom structure, partly covering the above-mentioned DSM-5 additional symptoms. Finally, although representing an important sub-population of patients, the sample's homogeneity (i.e. male veterans with combat-related PTSD) impedes result-generalizability to other trauma-exposed populations.
Despite these limitations, the present study has several key strengths that outweigh its limitations and contribute to extant knowledge in the field. Results indicate a pivotal role for flashbacks and psychological reaction to trauma reminders, with strong connections to other re-experiencing symptoms, in the clinical presentation of combat-exposed veterans with PTSD. Depressive symptoms had little effect on the PTSD network structure, reflecting two separate diagnostic entities, but with meaningful between-disorder connections, suggesting independent yet mutually-influential systems.
Supplementary material
The supplementary material for this article can be found at https://doi.org/10.1017/S0033291719002034.
Financial support
This work was supported by the National Institute of Mental Health T32-MH020004 (Amit Lazarov), T32-MH015144 (Benjamin Suarez-Jimenez), K01MH118428 (Benjamin Suarez-Jimenez), and R01MH105355 (Yuval Neria). The funding agency had no role in the study design; in the collection, analysis and interpretation of data; in the writing of the report; or in the decision to submit the article for publication.
Conflict of interest
None.
Ethical standards
The authors assert that all procedures contributing to this work comply with the ethical standards of the relevant national and institutional committees on human experimentation and with the Helsinki Declaration of 1975, as revised in 2008.