Introduction
The ever-increasing population and reduction of cultivable land are frequently posing a serious burden to the maintenance of desirable food security, better working conditions and sound biodiversity for any country's agricultural sector, especially for developing countries (Krupnik et al., Reference Krupnik, Schulthess, Ahmed and McDonald2017; Shew et al., Reference Shew, Durand-Morat, Putman, Nalley and Ghosh2019). Most developing countries are trying to mitigate their progressive food and fiber demands by adopting intensifying agricultural production (Ali, Reference Ali2007; Roy and Chan, Reference Roy and Chan2012). The intensification of agricultural production and massive global food demand have threatened the most crucial factors of production and led to landfill issues, increase in the use of synthetic chemicals in the forms of fertilizers and pesticides, and most importantly, detritions of the environment and global biodiversity (Butchart et al., Reference Butchart, Walpole, Collen, Van Strien, Scharlemann, Almond, Baillie, Bomhard, Brown and Bruno2010; Garcia et al., Reference Garcia, Cabeza, Rahbek and Araújo2014; Asrat and Simane, Reference Asrat and Simane2018). Global warming and climate change seriously hinder production, thus creating substantial pressure for agricultural sectors to curb food insecurity and malnutrition (Siegel, Reference Siegel2016; Shah et al., Reference Shah, Dulal and Awojobi2019). Agricultural production has undergone a dramatic transformation over time. Particularly, after the Second World War, food and fiber production efficiency increased markedly due to modern innovation and technological advancement (Harwood, Reference Harwood1990). Synthetic fertilizer and pesticide use, as well as production intensifications, has indeed improved significantly. This further poses a significant threat to natural soil fertility, damaging environmental ecology and creating hazardous health conditions (Savci, Reference Savci2012). Paradoxically, there is a rise in agricultural production and a rise in poverty and hunger. Growing global production processes are disrupted because we are damaging the very basis of agriculture through unsustainable activities. Conventional agriculture might have contributed to the situation, especially with climate change and ecological balance degradation (Warner et al., Reference Warner, Hamza, Oliver-Smith, Renaud and Julca2010; Israel et al., Reference Israel, Amikuzuno and Danso-Abbeam2020). In the meantime, developing countries would suffer immensely from global warming and climate change; in particular, smallholder farmers would suffer greatly. Although Bangladesh is a mostly agro-based economy, most of the farmers in this country are still in a trap of poverty and hunger, which makes them more vulnerable in terms of climate change and global warming (Agrawala et al., Reference Agrawala, Ota, Ahmed, Smith and Van Aalst2003; Agwu et al., Reference Agwu, Bakayoko, Jimoh and Stefan2018; Tessema and Simane, Reference Tessema and Simane2019). Urgent transformation should be needed to assess the sustainable environment, as current research indicates that climate change, especially global temperature, is dramatically rising, leading to unpredictable weather and exacerbating the circumstances that contribute to soil degradation, water contamination, threats of floods and famine. The main priority of sustainable food production is to meet people's present, fundamental food and fiber requirements, thus not narrowing the opportunity of future populations to meet their particular requirements (Adnan et al., Reference Adnan, Nordin and Bin Abu Bakar2017; Amare and Simane, Reference Amare and Simane2018; Ruttan, Reference Ruttan2019; Heylen et al., Reference Heylen, Meunier, Peeters, Ek, Neang, Hean and Peanh2020). Sustainable agriculture (SA) practitioners incorporate the following three key goals into their research: climate safety, social supportability and economic sustainability (Koohafkan et al., Reference Koohafkan, Altieri and Gimenez2012; Reytar et al., Reference Reytar, Hanson and Henninger2014; Whitehead et al., Reference Whitehead, MacLeod and Campbell2020).
Rapid urbanization, natural disasters, inadequate soil management and coercive farming methods are all threatening agricultural output in Bangladesh, the world's most heavily populated nation (Rahman and Mikuni, Reference Rahman and Mikuni1999). Seemingly, the Bangladeshi agricultural sector still lags in improved technological interactions, maintaining effective usage of land and water resources (Islam and Shirazul, Reference Islam and Shirazul2009; Raihan et al., Reference Raihan, Onitsuka, Basu, Shimizu and Hoshino2020). It is also experiencing decreasing productivity due to inappropriate agrochemical utilization, threatening its traditional agricultural systems (Faroque et al., Reference Faroque, Kashem and Bilkis2011). Furthermore, the high percentage of illiteracy among farmers (approximately 80%) and weak awareness level worsen the situation (Rasul and Thapa, Reference Rasul and Thapa2004). As a result of this vicious loop, farmers with large households typically ignore the possible advantages of agricultural expansion under sustainability themes (Rasul and Thapa, Reference Rasul and Thapa2003). Finally, the government of Bangladesh is working to foster SA, with a focus on ensuring effective usage of on-farm resources and reducing reliance on off-farm inputs (Sultana et al., Reference Sultana, Ahmed and Shiratake2020). Interestingly, farmers' interpersonal behavior regarding sustainable agricultural practices in Bangladesh is still not fully understood by previous studies.
In the study's scope, we utilized the generalized theory of planned behavior (TPB) to analyze behavioral factors' influence on farmers' intentions to adopt SA. TPB is among the most common frameworks for forecasting and interpreting behavioral intentions (Feng et al., Reference Feng, Fu, Zheng and Mu2010; Borges et al., Reference Borges, Foletto and Xavier2015; Borges and Lansink, Reference Borges and Lansink2016; Senger et al., Reference Senger, Borges and Machado2017; Rezaei et al., Reference Rezaei, Mianaji and Ganjloo2018; Mohammadinezhad and Ahmadvand, Reference Mohammadinezhad and Ahmadvand2020). TPB implies that the action of a person is dictated by his or her insistence to indulge in a particular outcome (Rhodes and Courneya, Reference Rhodes and Courneya2003; Rezaei et al., Reference Rezaei, Safa, Damalas and Ganjkhanloo2019), which includes motivations comprising behavioral perceptions, social expectations and assumed cultural influence (Chen, Reference Chen2016). Similar studies concluded that although the main aim of TPB is to provide self-identification to perform any specific behavior, it could be suitable for predicting psychological motivations to perform or not to perform that behavior (Smith et al., Reference Smith, Terry, Manstead, Louis, Kotterman and Wolfs2007). In addition, recent empirical studies tend to demonstrate that behavioral motivation is not a feature of a discrete collection of behavioral factors but comprises a dynamic interdependency system (Adnan et al., Reference Adnan, Nordin, Bahruddin and Tareq2019, Reference Adnan, Nordin and Anwar2020). Thus, the study extends the psychological motivation framework by evaluating the interrelationship among the associated factors to explore the complexity of the paradigm that has not been formally studied. SA is not a new idea, but this innovative farming mechanism has not been explored sufficiently within the behavioral dimensions of farmers. Particularly, farmers' opinions on adopting SA have not previously been explored within the context of a developing country. The important innovation in our study is to explore the adoption tendencies of SA among Bangladeshi farmers. Moreover, to quantify a structural representation of the evaluations, we employed the TPB, which is also relatively rare within these dimensions. To the best of our knowledge, this study will be one of the first attempts to explore the interpersonal behavioral component of young farmers within the Bangladeshi agriculture sector.
The rest of the paper is portrayed as follows: in the next section, we introduced and explored all the literature to develop the associated hypothesis. Section ‘Materials and methods’ presents the materials and methods, and section ‘Results and discussions’ portrays the results and discussion. Section ‘Conclusion’ denotes the conclusions of the study.
Literature review and hypothesis developments
As stated earlier, ever-increasing environmental pollution and global warming have had enormous negative impacts on agricultural productivity (Ruane et al., Reference Ruane, Major, Winston, Alam, Hussain, Khan, Hassan, Al Hossain, Goldberg and Horton2013), especially concerning irrigational water deficiency as a significant decisive factor for agricultural production (Misra, Reference Misra2014). A sufficient set of literature could be traced that explored sustainable agricultural practices within various directions. Many of those studies are comprised of SA with a large variety of ecological, natural, socio-economic and socio-emotional advantages, involving enhancing productivity, enhanced economic freedom, nutritious eating, better health and balanced agricultural practices, the fewer burden of labor, relatively demanding and mentally pleasurable daily tasks, and healthier family ties (Hobbs et al., Reference Hobbs, Sayre and Gupta2008; Scherr and McNeely, Reference Scherr and McNeely2008; Hayati et al., Reference Hayati, Ranjbar, Karami and Lichtfouse2010). For instance, Garnett et al. (Reference Garnett, Appleby, Balmford, Bateman, Benton, Bloomer, Burlingame, Dawkins, Dolan, Fraser, Herrero, Hoffmann, Smith, Thornton, Toulmin, Vermeulen and Godfray2013) and Tilman et al. (Reference Tilman, Balzer, Hill and Befort2011) evaluated the themes of SA to mitigate the adverse effects of intensifications in agricultural production, and Rockstrom et al. (Reference Rockstrom, Williams, Daily, Noble, Matthews, Gordon, Wetterstrand, DeClerck, Shah, Steduto, de Fraiture, Hatibu, Unver, Bird, Sibanda and Smith2017) utilized the concepts of SA. To quantify societal and global development, Busby et al. (Reference Busby, Soman, Wagner, Friesen, Kremer, Bennett, Morsy, Eisen, Leach and Dangl2017) portrayed the effects of SA practices toward plant microbiomes and conservation and agricultural sustainability. Seemingly, SA adoption could be analyzed in terms of two major approaches. The first creative aspect emphasizes the generation, extension, transformation and exchange of SA information as a complete framework (Hassanein and Kloppenburg Jr, Reference Hassanein and Kloppenburg1995, p. 1; Berg et al., Reference Berg, Zachow, Müller, Philipps and Tilcher2013; Dwyer, Reference Dwyer2013). The second aspect of SA is the interactive phase concerned with the following two questions: ‘What leads farmers to adopt sustainable agriculture?’ and ‘Why adopt this sustainable form of agriculture?’ The theoretical design of perceived value for prime agricultural users (farmers) (Bagheri, Reference Bagheri2010; Bernués et al., Reference Bernués, Tello-García, Rodríguez-Ortega, Ripoll-Bosch and Casasús2016) and behavioral dimensions (Bopp et al., Reference Bopp, Engler, Poortvliet and Jara-Rojas2019; Adnan et al., Reference Adnan, Nordin and Anwar2020) has also been traced within the past few decades (for more details, see Zeweld et al. (Reference Zeweld, Van Huylenbroeck, Tesfay and Speelman2017), Menozzi et al. (Reference Menozzi, Fioravanzi and Donati2014), Karami and Mansoorabadi (Reference Karami and Mansoorabadi2008) and Savari and Gharechaee (Reference Savari and Gharechaee2020)).
However, as the prime objectives of the study are to evaluate young farmers' motives or intentions to adopt SA practices, we employed the TPB and the expansion of social identity theory (SIT) to quantify the motives or intentions suggested by Savari and Gharechaee (Reference Savari and Gharechaee2020) and Tan (Reference Tan2013). The TPB and SIT have been found to offer an excellent foundation and evaluate the willingness to adopt new approaches by using several distinct determinants (Fekadu and Kraft, Reference Fekadu and Kraft2002; Fielding et al., Reference Fielding, McDonald and Louis2008). In predicting SA adoption preferences, it is necessary to evaluate farmers' perceptions about SA practices. The essential elements of TPB are attitude, subjective norms and perceived behavioral control (PBC), which shape an individual's behavioral intentions (Ajzen, Reference Ajzen1991, Reference Ajzen2005). When people assume that a particular action's conduct can create a favorable result, they can establish a favorable outlook about actions (Ajzen and Fishbein, Reference Ajzen and Fishbein1980). Throughout the SA framework, perceptions regarding environmentally friendly farming strategies affect adoption intentions (Fairweather and Campbell, Reference Fairweather and Campbell2003; Tilahun et al., Reference Tilahun, Angassa and Abebe2017). Intentions are moral decisions of how people would act in the long term, which provides a bridge through a person's attitude, perceptions, references and collective actions (Daxini et al., Reference Daxini, O'Donoghue, Ryan, Buckley, Barnes and Daly2018; Buyinza et al., Reference Buyinza, Nuberg, Muthuri and Denton2020), which can address any person's behavior profoundly (Daxini et al., Reference Daxini, Ryan, O'Donoghue and Barnes2019; Grzelak et al., Reference Grzelak, Guth, Matuszczak, Czyżewski and Brelik2019; Adnan et al., Reference Adnan, Nordin and Anwar2020; Buyinza et al., Reference Buyinza, Nuberg, Muthuri and Denton2020). An attitude comprises several emotional reactions, ideologies and comportments toward any specific person, circumstance, mechanism, framework or action. Attitudes seem to comprise profound accumulations of knowledge, experience, expectations and obligations, which can shape strong impacts for measuring any set of behavioral actions (Palacios, Reference Palacios2005; Wauters et al., Reference Wauters, Bielders, Poesen, Govers and Mathijs2010). Especially toward any new mechanism or situation, attitude provides specific information crucial to formulating a measurement framework (Orduño Torres et al., Reference Orduño Torres, Kallas and Ornelas Herrera2020). Former research within the context of similar approaches indicated that the potential attitude toward any particular mechanism works as a predictive indicator for predicting any prospective actions (Daxini et al., Reference Daxini, O'Donoghue, Ryan, Buckley, Barnes and Daly2018; Rezaei et al., Reference Rezaei, Safa, Damalas and Ganjkhanloo2019; Buyinza et al., Reference Buyinza, Nuberg, Muthuri and Denton2020). Positive attitudes concerning SA may possess a significant impact on social references. Based on this discussion, we propose hypothesis 1 as follows:
H1: Attitudes regarding sustainable agriculture (ATS) positively impact behavioral intentions to adopt sustainable agriculture practices (AI).
Social referencing enriches and controls any individual's interconnectivity with the social setting that can shape any specific behavior by predicting how social partners will appraise that behavior (Bandura, Reference Bandura and Feinman1992; Walle et al., Reference Walle, Reschke and Knothe2017). Previous research traced the degree to which a farmer believes that reference groups and information channels impact a farmer's behavior in adopting any novel tactics (Roling and Wagemakers, Reference Roling and Wagemakers2000; Lee, Reference Lee2005; Zeweld et al., Reference Zeweld, Van Huylenbroeck, Tesfay and Speelman2017). Social pressure is another form of social referencing that usually forces farmers to adopt particular strategies set by the government to safeguard societal benefits (Maertens and Barrett, Reference Maertens and Barrett2013; Adenle et al., Reference Adenle, Wedig and Azadi2019; Nguyen and Drakou, Reference Nguyen and Drakou2021). Simultaneously, a person is far more willing to behave in compliance with specified perceptions if these perceptions are compatible with everyone else's views in society (Zeweld et al., Reference Zeweld, Van Huylenbroeck, Tesfay, Azadi and Speelman2020). Moreover, farmers' comprehensive, positive and command perceptions of SA practices may lead them to refer practices to other farmers (Adnan et al., Reference Adnan, Nordin, Bahruddin and Tareq2019; Zhang et al., Reference Zhang, Yang, Ni and Xie2019; Li et al., Reference Li, Feng, Luo and Guan2020). Therefore, we propose hypothesis 2.
H2: Social references (SR) positively impact behavioral intentions to adopt sustainable agriculture practices (AI).
PBC denotes any individual's ability to accomplish a specific behavior (Ajzen and Madden, Reference Ajzen and Madden1986; Barlett, Reference Barlett and Barlett2019; Eanes and Zhou, Reference Eanes and Zhou2020). Ajzen (Reference Ajzen1991) defines PBC as the personal attributes and attitude regarding any specific situation, which can trigger the personal decision-making process. For example, it is apparent that if farmers possess favorable confidence regarding SA, there will be enough possibilities to adopt sustainable practices within their core farming methods (Adnan et al., Reference Adnan, Nordin and Bin Abu Bakar2017; Waseem et al., Reference Waseem, Mwalupaso, Waseem, Khan, Panhwar and Shi2020). Therefore, PBC has profound moderating roles in shaping a person's decisions and mostly quantifies the particular attitude regarding the circumstances (Martinez and Lewis, Reference Martinez and Lewis2016). Therefore, we propose hypothesis three.
H3: Perceived behavioral control (PBC) positively impacts the behavioral intentions to adopt sustainable agriculture practices (AI).
Self-identity is a generous approach to a person's persuasive behavior that can trigger and be triggered by the attitude of an individual possessed (Sparks and Shepherd, Reference Sparks and Shepherd1992; Sparks, Reference Sparks, Terry and Hogg2000; Li et al., Reference Li, Feng, Luo and Guan2020). Possible opportunities to flourish farmers' self-identity and influence created by other farmers' self-identities greatly impact changing their attitudes toward new situations (Cullen et al., Reference Cullen, Ryan, O'Donoghue, Hynes, hUallacháin and Sheridan2020). In contrast, those impacts have been traced mostly within visionary farmers (Sulemana and James, Reference Sulemana and James2014). Moreover, past studies have demonstrated that the psychological impact of family and community can affect how important it is to undertake actions of significance (Kauppinen et al., Reference Kauppinen, Vesala and Valros2012; Van Thanh and Yapwattanaphun, Reference Van Thanh and Yapwattanaphun2015). Therefore, it is logical to assume that persons' trust throughout the decision to adopt SA practice might be affected by the views of friends, family members and society itself. It may also be concluded that farmers who have a positive perspective about SA intend to adopt sustainable farming practices (Ansari and Tabassum, Reference Ansari and Tabassum2018; Cullen et al., Reference Cullen, Ryan, O'Donoghue, Hynes, hUallacháin and Sheridan2020; Silva et al., Reference Silva, Mauad, Domingues, Marques and Borges2020). In addition, farmers' moral concern for the environment can be attributed to a presumed societal obligation to fulfil their actions. For example, when others share clear viewpoints that SA is socially acceptable and economically advantageous to the community, it is likely fair to assume those socially beneficial actions that quantify the farmer's self-obligations to participate in SA (Adnan et al., Reference Adnan, Nordin and Bin Abu Bakar2017). Farmers may promote environmentally friendly behavior if they have enough information and resources to do so. In other words, the more opportunities and resources farmers have, the higher their expression of self-identity and behavioral control will be with respect to SA (Sadati et al., Reference Sadati, Fami, Asadi and Sadati2010). In the simulation of such interactions, we propose hypothesis four.
H4: Perceived self-identity (PSI) positively impacts behavioral intentions to adopt sustainable agriculture practices (AI).
Individuals can influence the way they perceive and respond to the situation that has been known to them as socially appraisable (Bretherton, Reference Bretherton and Feinman1992), which creates a positive attitude toward performing the actions. Therefore, it is expected that if SA will be socially acceptable and if the peer farmer also appraises SA practices, the farmers will possess a positive attitude and vice versa (Sherif et al., Reference Sherif, Sherif and Nebergall1965; Saliba et al., Reference Saliba, Callieris, D'Agostino, Roma and Scardigno2018; Ramborun et al., Reference Ramborun, Facknath and Lalljee2020). Similarly, it could be expected that there might be some connections between attitude reformation and PBC for measuring any person's decisions. For example, if a farmer possessed a positive attitude toward any particular action, they were likely to construct a positive esteem of self-identity. Seemingly, they feel high self-esteem when they think they are good enough to perform those actions and vice versa (Stangor, Reference Stangor2014a, Reference Stangorb). Therefore, it is fair to expect that behavioral components such as attitude or perceptions, social referents influence, PBC and perceived self-identity may positively impact farmers' adoption behavior toward SA and be interconnected. In the context of the above discussion, we evaluated the following additional hypotheses:
H5: Factors regarding the influences of attitudes (ATS) and social reference influence (SR) are positively interconnected to quantify behavioral intentions to adopt sustainable agriculture practices (AI).
H6: Factors regarding the influences of attitudes (ATS) and perceived behavioral control (PBC) are positively interconnected to quantify the behavioral intentions to adopt sustainable agriculture practices (AI).
H7: Factors regarding the influences of attitudes (ATS) and perceived self-identity (PSI) are positively interconnected to quantify behavioral intentions to adopt sustainable agriculture practices (AI).
H8: Factors regarding the influences of social referencing (SR) and perceived behavioral control (PBC) are positively interconnected to quantify the behavioral intentions to adopt sustainable agriculture practices (AI).
H9: Factors regarding the influences of social referencing (SR) and self-identity (PSI) are positively interconnected to quantify the behavioral intentions to adopt sustainable agriculture practices (AI).
H10: Factors regarding the influences of perceived behavioral control (PBC) and self-identity (PSI) are positively interconnected to quantify the behavioral intentions to adopt sustainable agriculture practices (AI).
Materials and methods
Study area, respondents and survey instruments
The study's prime objectives are to measure the young farmer's intention to adopt SA practices within a developing nation's framework and measure the interconnection among the behavioral principles. We collected empirical pieces of information to evaluate the behavioral principles regarding adopting SA approaches. Within the context of the study, we randomly choose 400 farmers listed under the Teesta Barrage Irrigation Project (TBIP) located in four districts of the lower Teesta River Basin of Bangladesh, namely, Gainbandha, Lalmonirhat, Nilphamari and Kurigram (see Fig. 1 for a visual map of the study area). TBIP is one of the most significant irrigation projects in Southeast Asia and the largest irrigation marvel of Bangladesh. It is considered one of the most exceptional initiatives to foster sustainability in the Bangladeshi agriculture sector (Sarker et al., Reference Sarker, Pramanik, Zerin and Ara2011; Mukherjee and Saha, Reference Mukherjee and Saha2016). So the study area is most appropriate for the study. The survey instrument was developed according to Planned Behavior's theory proposed by Fishbein and Ajzen (Reference Fishbein and Ajzen1975). A well-structured questionnaire with a five-point Likert scale evaluation criterion was employed to collect empirical data that can foster the evaluation process. In this study, we define a young farmer as a person who engaged in farming activities ranging from 21 to 30 years. Measuring young farmers' engagements is aligned with the existing literature (Balezentis et al., Reference Balezentis, Ribasauskiene, Morkunas, Volkov, Streimikiene and Toma2020; Munim and Noor, Reference Munim and Noor2020). Therefore, the selected respondent's age range in the article could be recognized as young farmers. As the survey was conducted in Bangla, we modified some technical terms to keep the assumption to the point.

Fig. 1 Study area.
Moreover, we simplified some technical terms and several practices for a better understanding of the respondents. All the respondents were well informed about several basic sustainable agricultural practices before the responses were taken (e.g., crop rotation, permaculture, cover crops, soil enrichment, natural pest management, biointensive integrated pest management and better water management). Please check the supplementary material for more details. We evaluate only the farmers who possessed at least the basic idea about SA. We also conducted a pilot test to ensure the best fit of the survey methods, as suggested by Sezen and Çankaya (Reference Sezen and Çankaya2013). After receiving feedback from the pilot test (with 30 farmers), we made the necessary adjustment and finalized the instrument. After a preliminary evaluation of the filled questionnaire, a complete set of 157 fully useable (with complete information and fit to our research objectives) feedback was found and used for further analysis. As a multidimensional approach, SEM does not require strict sample size and data normalization criteria (Wong, Reference Wong2013; Sarkar et al., Reference Sarkar, Qian and Peau2020). Likewise, there is no universal criterion to predict a sufficient sample size for SEM. Thus, maintaining an appropriate sample size is a challenging task for the investigation. Hence, 100 responses could be the base point for running the path estimation.
Interestingly, Marcoulides and Saunders (Reference Marcoulides and Saunders2006) proposed observing the maximum number of arrows pointing toward the proposed model's latent variable to maintain a minimum sample size for satisfactory SEM estimation. They recommended that if two arrows pointed toward the latent variables, the lowest sample size should be 52. If it is 5, then the sample should be 70, and if the arrow count is 10, then the lowest number of the sample should be comprised of at least 91 respondents. Therefore, the final dataset of 157 young farmers passed by the above-discussed parameters of minimum sample size.
Analytical framework
We utilized various empirical approaches for evaluating farmers' intentional behavioral principles to adopt SA practices to feed the study's prime objectives. This study used AMOS tools to determine the assumption's consistency and efficiency, which also portrayed the model's structural representation and SEM estimation. SEM is a multidimensional approach for testing and evaluating multivariate structural linkages between theory and data that has driven modeling (Kelloway, Reference Kelloway1995; Moreira et al., Reference Moreira, Sotiropoulos, Silva, Takashima, Sousa, Leite-Almeida and Costa2016). SEM differs from other modeling approaches, as they test the direct and indirect effects on pre-assumed causal relationships (Fan et al., Reference Fan, Chen, Shirkey, John, Wu, Park and Shao2016; Thirupathi and Vinodh, Reference Thirupathi and Vinodh2016; Sarkar et al., Reference Sarkar, Qian and Peau2020). SEM is a combined analytical approach of confirmatory factor analysis (CFA) and path mapping. CFA (based on psychometrics) intends to evaluate latent psychological factors that cannot be assessed by single variables such as attitude and satisfaction.
In contrast, path mapping (based on biometrics) is derived from the prospects of evaluating the causal interrelationship among the associated indicators by generating a path illustration. On the other hand, critics often indicate pitfalls in statistical design, a weak ability to check the external validity of some limited models, and difficulty mastering within the aspects of SEM (Tarka, Reference Tarka2018). We implement CFA to assure the validity of the selected model and allocate the factor loading of the model by evaluating the reliability and validity of the elements involved. The study validated the proposed model and evaluated all the associated hypotheses (H1–H10) by structural equation modeling (SEM) tactics powered by AMOS. The study chose AMOS-based SEM, as it is more compatible with small observations, a shortage of available theory, and greater statistical compatibility than most other SEM tactics, for example, covariant base (CB-SEM), partial least squares based (PLS-SEM), EQS and LISREL (Byrne, Reference Byrne2001; Thakkar, Reference Thakkar and Thakkar2020). We have adopted the maximum likelihood estimation tactics and covariance matrix as empirical inputs. To assess whether the model is fit or not, a set of fit models is constructed, such as the normed fit index (NFI), goodness of fit (GFI), root mean square error (RMSEA) and comparative fit index (CFI), as suggested by Tan (Reference Tan2013).
Results and discussion
Confirmatory factor analysis
We carried out a preliminary assessment using CFA to provide a comprehensive, precise and accurate representation of the selected framework. Moreover, two profound testing criteria of construct reliability (CR) and convergent validity (VE) should be implemented to ensure the reliability and validity of the proposed framework, as acclaimed by Fornell and Larcker (Reference Fornell and Larcker1981). Table 2 shows that the CR and VE values of every construct were >0.5, which confirmed that the chosen latent variables are reliable and valid, as recommended by Wong (Reference Wong2013) and Bagozzi and Yi (Reference Bagozzi and Yi1988). However, the interpretation of discriminant validity has been completed by using VE outcomes to evaluate the convergent validity. In addition, the average variance extracted (AVE) of all essential constraints was carefully calculated.
Table 2. Findings of confirmatory factor analysis

L, factor loadings; VE, convergent validity; CR, construct reliability.
Furthermore, AVE measures of all the indicators are higher than the minimum accepted value of 0.5, which secures the convergent validity suggested by Bagozzi and Yi (Reference Bagozzi and Yi1988), Munim and Noor (Reference Munim and Noor2020) and Sarkar et al. (Reference Sarkar, Qian and Peau2020). After that, the discriminant validity of the model should be evaluated (Byrne, Reference Byrne2001). To do so, the square root of AVE measures should be utilized to confirm the discriminant validity, and it should be less than the AVE value, as referred to by Fornell and Larcker (Reference Fornell and Larcker1981). In terms of our current study, the square of all the correlational values was less than the AVE value. The conceptual structures thus had distinguishing value, demonstrating that the frameworks seemed to be dynamic and interconnected. The endogenous correlations of the respective hypothesized frameworks have been evidently merged in their corresponding influences. The metrics are thus validated to demonstrate the strongest variable weights on a particular framework. Factors were then excluded from additional analyses if a particular element was largely or single factor leadenness occurred.
Structural model
In terms of adequate interpretation of the structural equation model, there is a lack of a well-established framework that can quantify standardization, and the comparing standards are also very diverse. Various researchers have used various tactics for various research dimensions, as SEM is a relatively complex and dynamic methodology. Prior research usually postulates a series of alternate models to explore the relationships among the experimental factors based on objectives, hypotheses and the collected dataset. According to Götz et al. (Reference Götz, Liehr-Gobbers, Krafft, Esposito Vinzi, Chin, Henseler and Wang2010) and Barrett (Reference Barrett2007), model selection, as well as assessment, should be centered on a selective synthesis of practical and theoretical concerns, numerical simulation, fitness value, partial fit index, representativeness test, and finally, the results should be compared with similar models (Table 3).
Table 3. Descriptive statistics of key variables

As per construct validity, the research framework of the study suitably matched the fit index. The model comprises a GFI of 0.921, which secures values higher than the acceptable value of 0.9, and the standardized root means that the square error value accounted for 0.052, which was significantly lower than the standard value of 0.08. The CFI evaluates the fitness of the framework with the help of measuring the difference between surveyed data and the conceptual framework, while the adjustments of the concerns for the integral of sampling size within the mechanism of χ2 evaluation for securing the credibility of the framework (Gatignon, Reference Gatignon and Gatignon2010) as well as by the evaluation of NFI (Bentler, Reference Bentler1990). The CFA is standard between 0 and 1, whereas the large values denote with more accuracy, and thus 0.95 or higher standard considered as most preferable suggested by Hu and Bentler (Reference Hu and Bentler1999). The investigated framework produced NFI = 0.952, IFI = 0.981 and CFI = 0.981, which denote sufficient accuracy of the framework.
The SEM model embraces a relatively concentrated and complex criterion, whereas the assessment mostly depends on the underlined theoretical views. Moreover, the sample size can greatly quantify the model setup. This creates some shortcomings regarding model viability, resulting in a paradoxical indication (La Du and Tanaka, Reference La Du and Tanaka1989; Marsh et al., Reference Marsh, Hau and Grayson2005). To provide a better solution, Mulaik et al. (Reference Mulaik, James, Van Alstine, Bennett, Lind and Stilwell1989) provided well-structured evolutionary criteria popularly known as parsimony of fit indexes, the Parsimony Goodness-of-Fit Index (PGFI), and the Parsimonious Normed Fit Index (PNFI). The PNFI is a widely adopted tactic for assessing latent and existing variables (Sivo et al., Reference Sivo, Fan, Witta and Willse2006). Parsimony is quantified throughout the design under which the evaluation criterion is set as per the combination of principles of independence, levels of freedoms and regression equations (Table 4). The value of PNFI is zero to one, whereas the higher the value is, the better the parsimonious capacity to derive the fit index (Hooper et al., Reference Hooper, Coughlan and Mullen2007). Within the study context, the study's output showed a PNFI value of 0.839, which portrayed a well-established correlational matrix and provided adequate measurement capacity among the exogenous and endogenous factors. We fail to reject H1 since attitudes concerning SA significantly positively impact interpersonal preferences (standardized coefficient = 0.251, t = 3.309), indicating that farmers with a more favorable attitude toward SA are more likely to pursue SA in the future. The more favorable the attitude about the benefit of SA farmers holds, the greater they tend to adopt SA practices, which denotes parallel findings of Bultena and Hoiberg (Reference Bultena and Hoiberg1992) and Tatlıdil et al. (Reference Tatlıdil, Boz and Tatlidil2009). One of the surveyed farmers stated that ‘Actually the attitude regarding sustainable agriculture in our regions is relatively new, while we the framers usually consider any novel tactics it fosters us economically’. His fellow farmer added that ‘I usually like the approaches of better tillage management, cover crops, improved soil management and integrated pest management as those approaches may improve productivity’. According to one of the agricultural extension officers of the surveyed regions, the advantages that mostly trigger farmers to shape favorable practices largely depend on ecological, natural, socio-economic and socio-emotional advantages, including enhanced productivity, enhanced economic freedom, nutritious eating, better health and balanced agricultural practices, a lower burden of labor, relatively demanding and mentally pleasurable daily tasks and healthier family ties.
Table 4. Regression weights

***P < 0.05.
Hypothesis testing indicated that social referent does not influence the adoption behavior of SA practices (standardized coefficient = 0.079, t = 0.954). As a result, we reject hypothesis two (H2). Interestingly, in a study of Iowa farmers and agriculture professionals, Carolan (Reference Carolan2006) also found the weakness of social referencing. However, Mishra et al. (Reference Mishra, Gyawali, Paudel, Poudyal, Simon, Dasgupta and Antonious2018), Kabii and Horwitz (Reference Kabii and Horwitz2006), and Baumgart-Getz et al. (Reference Baumgart-Getz, Prokopy and Floress2012) found that social referencing could be influential in shaping adopting behavior. This could have happened because those studies represent farmers from technologically advanced countries. One of the farmers highlighted the lack of information-sharing platforms and access to agricultural values chain activities to utilize SA practices' potentialities effectively.
In line with the findings of Fairweather and Campbell (Reference Fairweather and Campbell2003), Cristea et al. (Reference Cristea, Bozgă, Tiţa and Munteanu2019), Heylen et al. (Reference Heylen, Meunier, Peeters, Ek, Neang, Hean and Peanh2020), Bernués et al. (Reference Bernués, Tello-García, Rodríguez-Ortega, Ripoll-Bosch and Casasús2016), and Borges et al. (Reference Borges, Foletto and Xavier2015), the study also finds that PBC leads to willingness and regulates actions to shape behavior (standardized coefficient = 0.192, t = 2.299), which indicates that we fail to reject hypothesis three (H3). For Bangladeshi farmers, the shortage of resource supply and initial capital expenditure created huge barriers toward facilitating SA. In other words, the more wealth and incentives that farmers possess, the much more positive behaviors they will quantify, which is supported by the research of Floress et al. (Reference Floress, Reimer, Thompson, Burbach, Knutson, Prokopy, Ribaudo and Ulrich-Schad2018). One farmer indicates that ‘till now, there are no incentives or subsidies facilities has not taken by the government’. Moreover, ‘easy access to the financial and risk-sharing network will be more helpful for us to adopt SA practices’, his fellow farmer added. The research has indicated greater ambitions for the farmer who considered himself more ecologically responsible than the individual who has not aligned himself with such resources (standardized coefficient = 0.283, t = 3.778). The analysis showed that certain environmental impacts could shape farmers' behavior and ambitions to adopt SA practices. Recent studies have also found similar findings that farmers largely intended to adopt SA practices, as they are well concerned about environmental safety (Bopp et al., Reference Bopp, Engler, Poortvliet and Jara-Rojas2019), and they are also found to exercise environmentally friendly farming practices regarding seedlings, land preparation, pesticide usage for harvesting and waste disposal (Daxini et al., Reference Daxini, Ryan, O'Donoghue and Barnes2019). Table 4 denotes that we fail to reject hypotheses five (H5) to ten (H10) as per the analysis, as a positive interconnection was traced among the associated psychosocial factors. This outcome supported the previous results that a reciprocal association occurs between psychosocial factors (Brigance et al., Reference Brigance, Soto Mas, Sanchez and Handal2018; Caffaro et al., Reference Caffaro, Roccato, Cremasco and Cavallo2019; Zeweld et al., Reference Zeweld, Huylenbroeck, Tesfay and Speelman2019; Foguesatto et al., Reference Foguesatto, Borges and Machado2020). In terms of adopting SA, perceptions or attitudes toward SA practices have been closely interlinked to shape other farmers' views and the desire to engage in SA (PBC) to provide strong influence and potential options for recognition (perceived self-identity) with correlation coefficients of 0.529, 0.214 and 0.308, respectively.
Simultaneously, social referencing impacts toward SA have a stronger correlational impression to shape belief, expectation and controlling power (PBC). It also possessed a strong and positive interconnection toward shaping farmers' self-identity, with correlation coefficient values of 0.451 and 0.409. In particular, as per the correlation coefficient values of 0.379, it has been shown that there is a relatively positive association between high control of perceived behavior on SA and the opportunities to build self-identity as ecological perceptions. According to the graphical illustration portrayed within Figure 2, the interconnections among the psychosocial factors have been crucial for better understanding the prediction powered by the TPB. An increasing rate of approximately 33% has been traced within the accumulated variances of adopting the behavior (Table 5).
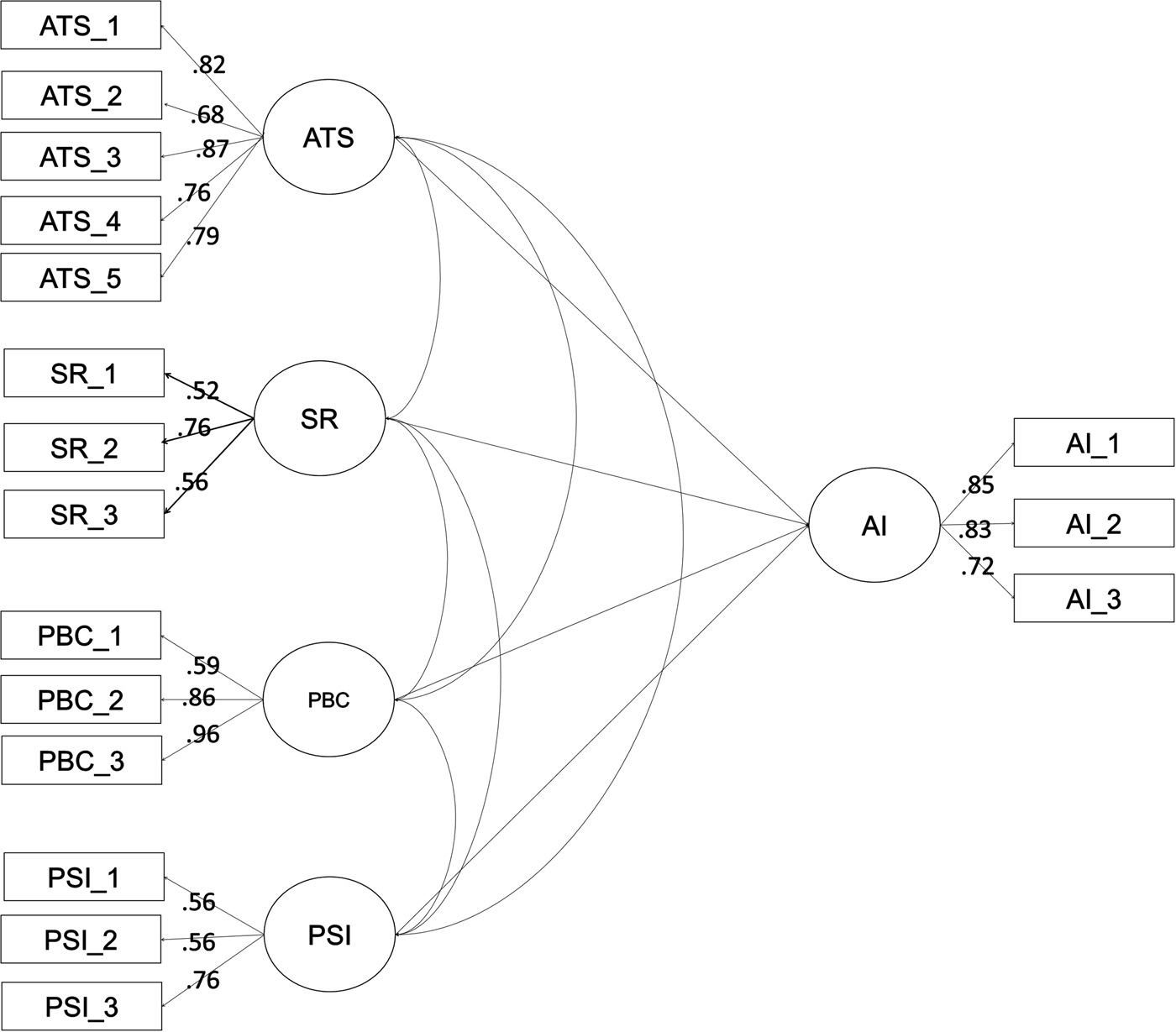
Fig. 2 Path diagram.
Table 5. Correlation results

***P < 0.05.
Conclusions
The principle of SA comprises a crucial alteration in young farmers' behavioral components. SA seeks to minimize the total effect on the economy, human health and the ecosystem by utilizing renewable resources and preventing environmental damage. As stated in the results section, attitudes toward SA significantly quantify adoption intentions. Perception is usually derived from mind storming tendencies, whereas decisions have usually been made as per the like or dislike of any particular thing. The more optimistic or auspicious farmers are regarding SA, the more likely they are to adopt SA practices. The findings also reflected that favorable resources, tactical knowledge and training facilities also led farmers to adopt SA practices. However, Bangladesh is still facing hurdles for facilitating SA practices because the farmer's literacy rate is low, and they possessed limited knowledge regarding SA. The governmental authority could initiate long-term training facilities, promote innovative technologies and provide support via subsidies to increase the approachability of SA within agricultural sectors. Easy financing and risk-sharing opportunities could be introduced to improve young farmers' self-identity and PBC. In addition, agricultural extension services could act more responsibly and sensibly to support technical know-how and other guidance to enrich farmers' knowledge, which eventually improves the attitude level of young farmers. In measuring SA's adoption criterion, perceived self-identity has also emerged as the crucial factor.
Moreover, there is small involvement of social referents traced to the formulation of the decisional framework to availing these dynamic criteria of modern farming systems. Governments need to emphasize and promote the dimensions of a farmer's self-identity. Such activities help farmers create their self-identity and usually assist in shaping positive perceptions toward SA. Agricultural departments and extension services need to work closely with farmers and increase awareness-building campaigns to promote SA practices within rural farmers, as most of the rural farmers in Bangladesh are relatively uneducated and mostly not aware of the goodness of SA. Agriculture extension departments need to increase and expand demonstration facilities to transmit the social, economic and environmental benefits of sustainable agricultural practices. Legislators and policymakers should impose strict rules and a certain level of environmentally friendly farming practices, which eventually increase the adoption rate. These tactics are crucial for most developing countries, such as Bangladesh.
Moreover, the farmer needs to be more aware of the new technology of farming and environmentalism. In the context of our study, we found that a social referencing factor does not have significant impacts on triggering the adoption behavior of framers regarding SA. However, it has a positive and viable relationship related to resource obtainability opportunities and self-identity formation. This could be because most of the farmers within the surveyed area lack knowledge regarding sustainability and do not possess sufficient expertise. Therefore, the government should increase training facilities and promote new environmentally friendly technologies to minimize the gap between the theory and practices of SA.
The study comprised its findings by evaluating a relatively small sample size from the prospects of a developing country's agricultural sector. Therefore, there is a chance of bias in the responses. If the data can be traced from a wide region covered with different geographic circumstances, it will be more interesting. However, the study used the notion of SA with broad aspects. It will be more influential if the adoption intention of some specific sustainable practices could have been examined. Future studies could use several distinctive SA practices in the form of a model and test the affectivity of those practices (check supplementary material for more details regarding several sustainable practices). Moreover, the study largely depended on statistical software; it would be more useful to give SEM in the form of equations to portray the findings more concisely.
Supplementary material
The supplementary material for this article can be found at https://doi.org/10.1017/S1742170521000429.
Data
Data and material are available upon request.
Acknowledgements
We cordially thank Professor Su Zhong Feng, Professor, Management School, Xian Jiaotong University, for assistance with the SEM technique guidelines, and Dr. Saleh Shahriar for his comments that significantly help us to improve the manuscript. We would also like to show our gratitude to the Experts group for sharing their pearls of wisdom within the questionnaire parts, which was a great help during this research, and we thank two ‘anonymous’ reviewers for their so-called insights.
Conflict of interest
There are no conflicts of interest/competing interests associated with the study.
Code availability
Not applicable to the study.
Authors’ statement
All the authors read and approve the submission into the current form. All authors declare there is no conflict of interest associated with this article.