Introduction
Inflammation has been implicated in the pathophysiology of severe mental disorders such as schizophrenia (SCZ) and affective (AFF) disorders (Khandaker et al., Reference Khandaker, Cousins, Deakin, Lennox, Yolken and Jones2015; Goldsmith et al., Reference Goldsmith, Rapaport and Miller2016). Many studies have shown aberrant levels of a range of inflammatory markers in these patients (Khandaker et al., Reference Khandaker, Cousins, Deakin, Lennox, Yolken and Jones2015; Goldsmith et al., Reference Goldsmith, Rapaport and Miller2016; Leboyer et al., Reference Leboyer, Oliveira, Tamouza and Groc2016). Further support for the involvement of the immune system in severe mental disorders comes from recent genetic findings of immune-related genes associated with SCZ (Schizophrenia Working Group of the Psychiatric Genomics Consortium, 2014; Sekar et al., Reference Sekar, Bialas, De Rivera, Davis, Hammond, Kamitaki, Tooley, Presumey, Baum, Van Doren, Genovese, Rose, Handsaker, Daly, Carroll, Stevens and Mccarroll2016), and findings from epidemiological studies suggesting that infections and auto-immune diseases increase the risk for SCZ and bipolar disorder including AFF disorders with psychosis (Eaton et al., Reference Eaton, Byrne, Ewald, Mors, Chen, Agerbo and Mortensen2006; Brown and Derkits, Reference Brown and Derkits2010; Eaton et al., Reference Eaton, Pedersen, Nielsen and Mortensen2010). However, the role of inflammation and immune activation in severe mental disorders is still poorly understood.
Many studies have shown increased levels of cardiometabolic risk factors like dyslipidemia and obesity in severe mental disorders (Laursen et al., Reference Laursen, Munk-Olsen and Vestergaard2012; Ringen et al., Reference Ringen, Engh, Birkenaes, Dieset and Andreassen2014), and there is overwhelming evidence for excess mortality related to cardiovascular disease (CVD) in these disorders (Crump et al., Reference Crump, Sundquist, Winkleby and Sundquist2013; Dieset et al., Reference Dieset, Andreassen and Haukvik2016). Inflammation is an important part of the pathophysiology of CVD, for instance atherosclerosis is classified as an inflammatory disorder (Libby, Reference Libby2002; Cox et al., Reference Cox, West and Cripps2015), and new immune-modulating treatments targeting atherosclerosis are emerging (Sage and Mallat, Reference Sage and Mallat2017). Thus, the immune system may play a role in both severe mental disorders and CVD, supported by genetic studies which report pleiotropy between severe mental disorders and CVD risk factors (Andreassen et al., Reference Andreassen, Djurovic, Thompson, Schork, Kendler, O'donovan, Rujescu, Werge, Van De Bunt, Morris, Mccarthy, Roddey, Mcevoy, Desikan and Dale2013; Andreassen et al., Reference Andreassen, Harbo, Wang, Thompson, Schork, Mattingsdal, Zuber, Bettella, Ripke, Kelsoe, Kendler, O'donovan, Sklar, Mcevoy, Desikan, Lie, Djurovic and Dale2015). Although cardiometabolic risk factors and SCZ show genetic pleiotropy, the direction of effect may be divergent, meaning that although the same locus may be associated with both disorders, the direction of effect are opposite, suggesting somewhat different underlying mechanisms despite statistical signal in the same gene loci in the two disorders. Further, cardiometabolic risk factors have traditionally been attributed to lifestyle factors and antipsychotic medication in this patient group (Dieset et al., Reference Dieset, Hope, Ueland, Bjella, Agartz, Melle, Aukrust, Rossberg and Andreassen2012; Laursen et al., Reference Laursen, Munk-Olsen and Vestergaard2012; Ringen et al., Reference Ringen, Engh, Birkenaes, Dieset and Andreassen2014). Immune abnormalities could represent a causal link between severe mental disorders and cardiometabolic risk factors explaining part of the CVD comorbidity, or could only be associated with the comorbid cardiometabolic risk factors and thus a secondary phenomenon in severe mental disorders.
The inflammatory markers were chosen based on certain criteria. First, all of the markers reflect pathways that are activated during CVD. Second, they reflect distinct but also overlapping pathways during inflammation and immune activation (e.g. T cell and monocyte activation, endothelial cell activation, activation of the tumor necrosis factor pathway). Third, based on experimental studies and some in vivo studies these pathways could also be activated in severe mental disorders, but data on several of these markers on large population studies are scarce. Lastly, these markers are regarded as stable and can be reliably measured. Markers of T-lymphocyte activation and regulation include the chemokine CXCL16, soluble interleukin-2 receptor (sIL-2R) and activated leukocyte cell adhesion molecule (ALCAM) representing adaptive immunity, while soluble CD14 (sCD14) and macrophage inhibitory factor (MIF) are markers of monocyte/macrophage activation representing innate immunity; two major parts of the immune system that also show considerable cross-talk. CXCL16 has been implicated in SCZ in genetic studies (Lee et al., Reference Lee, Kim and Song2013; Ueland et al., Reference Ueland, Gullestad, Nymo, Yndestad, Aukrust and Askevold2015), sCD14 has been shown to be upregulated in activated microglia (McKibben et al., Reference Mckibben, Margolick, Grinspoon, Li, Palella, Kingsley, Witt, George, Jacobson, Budoff, Tracy, Brown and Post2015; Johansson et al., Reference Johansson, Jakobsson, Fortgang, Zetterberg, Blennow, Cannon, Hultman, Wetterberg and Landen2016) and MIF has been related to depression (Bloom and Al-Abed, Reference Bloom and Al-Abed2014). Aberrant levels of sIL-2R has been demonstrated in SCZ and AFF disorders (Khandaker et al., Reference Khandaker, Cousins, Deakin, Lennox, Yolken and Jones2015; Goldsmith et al., Reference Goldsmith, Rapaport and Miller2016) while ALCAM may facilitate migration of lymphocytes across the blood–brain barrier (BBB) (Wagner et al., Reference Wagner, Bilinska, Pokryszko-Dragan, Sobczynski, Cyrul, Kusnierczyk and Jasek2014), and these mediators could also be regulated systemically in these disorders. However, many studies of systemic inflammation in severe mental disorders have not taken cardiometabolic risk factors like body mass index (BMI) and blood lipid levels into account, and it is largely unknown if alterations in inflammatory markers are secondary to comorbid cardiometabolic risk factors.
In the current study we aim to disentangle immune activation related to cardiometabolic risk factors from severe mental disorders in a well described and large cohort. We hypothesized that assessment of robust circulating markers reflecting different inflammatory pathways relevant for the progression of atherogenesis in patients with SCZ and AFF and subsequent adjustment for established comorbid cardiometabolic risk factors would reveal if the enhanced systemic inflammation in these disorders is an epiphenomena of increased risk for CVD. Thus, we first investigated selected inflammatory markers known to be involved in CVD, i.e. CXCL16, sIL-2R, sCD14, MIF and ALCAM in patients compared to healthy controls (HC) in a large cohort (n = 1639), including differences between SCZ and AFF. Second, we assessed if the potential differences in levels of inflammatory markers are explained by comorbid cardiometabolic risk factors including BMI, glucose and blood lipid levels, and finally if level of inflammatory markers are associated with treatment with psychotropic medication and symptom severity. In addition, we repeated the analyses in a smaller 1 year follow-up sample (n = 216) to confirm the results.
Materials and methods
Setting and participants
The current study is part of the ongoing Thematically Organized Psychosis (TOP) Study which includes patients from hospitals in the Oslo region, Stavanger, Trondheim and South-East regional hospitals in Norway (Hope et al., Reference Hope, Dieset, Agartz, Steen, Ueland, Melle, Aukrust and Andreassen2011, Reference Hope, Hoseth, Dieset, Morch, Aas, Aukrust, Djurovic, Melle, Ueland, Agartz, Ueland, Westlye and Andreassen2015; Simonsen et al., Reference Simonsen, Sundet, Vaskinn, Birkenaes, Engh, Faerden, Jonsdottir, Ringen, Opjordsmoen, Melle, Friis and Andreassen2011). The main criterion of inclusion in the TOP Study is a diagnosis of SCZ spectrum disorder, bipolar spectrum disorder or major depressive disorder with psychotic features according to Diagnostic and Statistical Manual of Mental Disorders (DSM)-IV. All participants were between 18 and 65 years and able to give written informed consent. The patients were recruited during early phase after an acute episode (post-acute episode) mostly from outpatient clinics and only included when they were clinically stable enough to provide informed consent. Participants were excluded if they did not speak Scandinavian and/or presence of pronounced cognitive deficit (IQ < 70) and/or severe brain damage/illness in order to make sure that all participants were able to fully understand the meaning of the study. The participants were able to withdraw from the study at any point without explanation (Morch et al., Reference Morch, Dieset, Faerden, Hope, Aas, Nerhus, Gardsjord, Joa, Morken, Agartz, Aukrust, Djurovic, Melle, Ueland and Andreassen2016). They all underwent the same investigation protocol, which includes assessment of psychiatric symptoms, diagnosis, physical examination, neuropsychological testing and collection of blood samples according to a pre-defined blood sampling protocol. The HC were randomly selected from statistical records (http://www.ssb.no) from the same catchment area as the patients in the Oslo region. HC were between 18 and 60 years old, apparently healthy individuals based on clinical examination and disease history, and none had any history of severe mental disorders, head injury, neurological disorders, illicit drug use, close relatives with severe mental disorder or medical problems that somehow could interfere with brain function (e.g. hypothyroidism, uncontrolled hypertension and diabetes) (Hope et al., Reference Hope, Melle, Aukrust, Steen, Birkenaes, Lorentzen, Agartz, Ueland and Andreassen2009, Reference Hope, Dieset, Agartz, Steen, Ueland, Melle, Aukrust and Andreassen2011, Reference Hope, Ueland, Steen, Dieset, Lorentzen, Berg, Agartz, Aukrust and Andreassen2013, Reference Hope, Hoseth, Dieset, Morch, Aas, Aukrust, Djurovic, Melle, Ueland, Agartz, Ueland, Westlye and Andreassen2015). All participants have given written consent and the study was approved by the Norwegian Scientific Ethical Committees and the Norwegian Data Protection Agency. The authors assert that all procedures contributing to this work comply with the ethical standards of the relevant national and institutional committees on human experimentation and with the Helsinki Declaration of 1975, as revised in 2008.
Sample
A total of 992 patients with severe mental disorders and 647 HC were included from 2002 until 2013 in the current study. In the sample, a total of 675 patients had a SCZ spectrum disorder [SCZ, schizoaffective disorder, schizophreniform disorder and psychosis not otherwise specified (NOS)] and were included in the diagnostic group ‘Schizophrenia’ (SCZ), while 317 patients had an AFF disorder (bipolar I, bipolar II, bipolar NOS and major depressive disorder with psychotic symptoms) and were included in the diagnostic group ‘Affective’ (AFF). SCZ and AFF groups combined were included in the ‘all patients’ group.
Participants with cancer, autoimmune or inflammatory diseases, on-going infections, C-reactive protein (CRP) >20 mg/L of any reason, or patients receiving treatment with immune modulating or suppressant drugs were excluded from the study.
A total of 216 participants (HC n = 92 and patients n = 124; including SCZ n = 67, AFF n = 57) underwent repeated assessments after 1 year. This follow-up sample was not significantly different from the main cohort on majority of clinical and sociodemographic variables. For details see online Supplementary information.
Clinical assessments
The patients were interviewed and sociodemographic history, medical history, substance use, psychiatric symptoms, medication and potential side effects were recorded. They all underwent diagnostic interviews based on Structured Clinical Interview in DSM-IV axis I Disorders (SCID-1), and symptom assessments with Positive and Negative Syndrome Scale (PANSS), Young Mania Rating scale (YMRS), and Calgary Depression Scale for Schizophrenia (CDSS). Function was assessed with the functioning score of the split version of the Global Assessment of the Functioning Scale (GAF). Diagnostic evaluation was performed by trained psychologists and psychiatrists, all of whom participated regularly in diagnostic meetings supervised by professors in psychiatry. The reliability of diagnostic and symptom assessments was very good. The overall agreement for the DSM-IV diagnostic categories tested was 82% and the overall κ 0.77 (95% confidence interval 0.60–0.94) (Simonsen et al., Reference Simonsen, Sundet, Vaskinn, Birkenaes, Engh, Faerden, Jonsdottir, Ringen, Opjordsmoen, Melle, Friis and Andreassen2011).
All participants including HC were screened with history taking, physical examination and routine blood tests, smoking habits, alcohol consumption, and use of illicit drugs in the two weeks period prior to blood sampling. The HC were interviewed for current or previous history of severe mental disorder themselves or in their family, and assessed with Primary Care Evaluation of Mental Disorders.
Cardiometabolic risk factors
Plasma levels of total cholesterol, high density lipoprotein (HDL) cholesterol (HDL), low density lipoprotein (LDL) cholesterol (LDL), triglycerides (TG) and glucose were measured at the Department of Clinical Chemistry, Oslo University Hospital, using an Integra 800 instrument from Roche Diagnostics, according to standard methods. All participants were weighed on calibrated digital weights under equal conditions, height was measured with standard methods and BMI (kg/m2) was calculated.
Inflammatory markers
Plasma levels of CXCL16, sIL-2R, sCD14, MIF and ALCAM were measured in duplicate by enzyme immunoassays (EIA) in using commercially available antibodies (R&D Systems, Minneapolis, MN, USA) in a 384 format using a combination of a SELMA (Jena, Germany) pipetting robot and a BioTek (Winooski, VT, USA) dispenser/washer. Absorption was read at 450 nm with wavelength correction set to 540 nm using an ELISA plate reader (Bio-Rad, Hercules, CA, USA). Intra- and inter-assay coefficients of variation were <10% for all EIAs. For immunoassays blood was taken using EDTA vials and the plasma was isolated the next working day and stored at −80 °C. Blood sampling was performed between 8:00 and 17:00 hours with some variations between patients and HC. Most participants were fasting during blood collection.
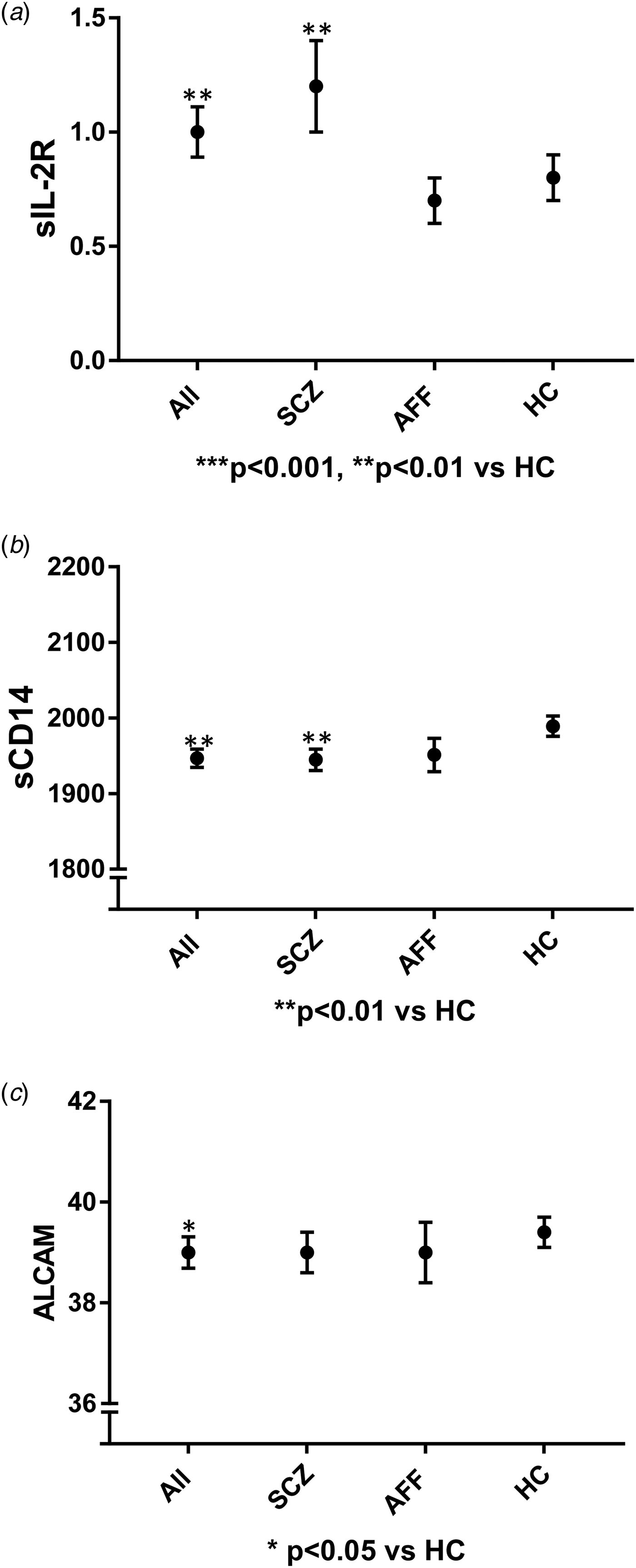
Fig. 1. Figures presenting main findings with inflammatory marker levels in diagnostic group using mean and standard error of mean. sIL-2R: soluble interleukin-2 receptor; sCD14: soluble CD14; ALCAM: activated leukocyte cell adhesion molecule; p-values obtained after correction for age, gender, total cholesterol and Bonferroni for sIL-2R and sCD14, ALCAM corrected for age, gender, total cholesterol, glucose, BMI, and Bonferroni, ALL: all patients; SCZ: schizophrenia; AFF: affective; HC: healthy controls.
Medication
The information regarding prescribed medications including antipsychotics, antidepressants, mood stabilizers and lithium used by patients were obtained by clinical interview and hospital records. We calculated ‘defined daily dosages’ (DDD) according to the World Health Organization (WHO) principles, as described previously (Morch et al., Reference Morch, Dieset, Faerden, Hope, Aas, Nerhus, Gardsjord, Joa, Morken, Agartz, Aukrust, Djurovic, Melle, Ueland and Andreassen2016). The DDD is the assumed average maintenance dose per day for a drug used for its main indication in adults and provide a fixed unit of measurement independent of dosage form (http://www.whocc.no/atc_ddd_index/).
Statistical analyses
All statistical analyses were performed using the SPSS software package for Windows, version 22.0 (SPSS Chicago, USA). All analyses were two-tailed with a level of significance set at p < 0.05. All skewed data were log-transformed (CXCL16, sIL-2R, MIF) prior to further analyses. We used analysis of variance (ANOVA) and χ2 tests to investigate differences in demographic variables between patients and HC.
In order to identify possible confounders we performed bivariate correlations using Pearson or Spearman coefficients for continuous variables and ANOVA for categorical variables. Age, gender, ethnicity, smoking status, alcohol consumption, use of illicit drugs, time of blood sampling, and use of psychotropic medication (including cumulative DDD of antipsychotics, antidepressants, mood stabilizers and lithium; online Supplementary Table 1, Bonferroni corrected p-value set at 0.01; 0.05/4 medication groups) were included as possible confounders. We also investigated correlations between the inflammatory markers and core symptoms in the total patient group, and Bonferroni corrected p-value for these correlation analyses were set at 0.01.
Overall, we identified age and gender as potential confounders and they were included in the subsequent analyses for all inflammatory markers.
Analysis of covariance (ANCOVA) was used to investigate differences between all patients (including the separate diagnostic groups SCZ and AFF) and HC and included Bonferroni correction to adjust for multiple testing. The ANCOVA analyses were Bonferroni corrected for multiple testing based on the number of group comparisons. The inflammatory markers were selected based on a clear hypothesis in which they have a known role in CVD in addition to independent roles within the immune system and thus we did not correct for the number of markers. In order to investigate the effect of cardiometabolic risk factors like glucose, BMI, total cholesterol, HDL, LDL and TG we performed partial bivariate correlations between the cardiometabolic risk factors and inflammatory markers controlling for diagnosis. We then included these cardiometabolic risk factors which correlated with the inflammatory markers as covariates in the subsequent ANCOVA model to investigate differences between patients and HC. We included only the covariates that correlated with the different inflammatory markers in the bivariate analyses. BMI (in addition to total cholesterol and glucose) correlated only with ALCAM in the preliminary bivariate correlation analysis and was thus included as a covariate in the main ANCOVA analysis for ALCAM, but not for the other inflammatory markers. There was collinearity between total cholesterol, TG, HDL and LDL levels, so we included only total cholesterol levels in the ANCOVA model as it is a sensitive marker of cardiometabolic risk and less sensitive to fasting status (Rahman et al., Reference Rahman, Blumenthal, Jones, Martin, Gluckman and Whelton2018).
We also did follow-up analyses of the influence of HDL, LDL and TG, and the main result remained the same. We repeated the same ANCOVA analyses in the follow-up subsample and included the same covariates as described above. We also repeated the analysis in which we excluded those with CRP above 10 (n = 44) and the main results remained the same (online Supplementary Table 8).
Results
Sample characteristics
The clinical characteristics of the study population are shown in Table 1. The mean age and gender distribution in patients and HC were mainly the same. Significantly more patients smoked cigarettes, used illicit substances and were of non-European origin compared to the HC. In addition, the majority (78%) of patients received psychotropic drug treatment. The patients had significantly higher BMI and LDL levels, while HDL levels were lower compared to HC reflecting an unfavorable metabolic profile in the patients groups.
Table 1. Clinical characteristics and cardiometabolic risk factors

Analyzed with ANOVA for continuous variables and χ2 test for categorical variables. IU: international units; n = number; s.d.: standard deviation; SCZ: schizophrenia; AFF: affective; HC: healthy controls; ALL: all patients; N/A: not applicable; n.s.: not significant; PANSS: Positive and Negative Syndrome Scale; CDSS: Calgary Depression Scale for Schizophrenia; YMRS: Young Mania Rating Scale; GAF-F: Global Assessment of Functioning-functions; GAF-S: Global Assessment of Functioning-symptoms; HDL: high density lipoprotein cholesterol; LDL: low density lipoprotein cholesterol; BMI: body mass index; mmol: millimoles per liter.
Inflammatory markers before adjustment for cardiometabolic risk factors
Levels of CXCL16 (p = 0.03) and sIL-2R (p = 7.8 × 10−5) were higher, while sCD14 tended to be lower (p = 0.05) in patients compared to HC after adjustment for other potential confounders. There was no significant difference between patients and HC for MIF (p = 0.34) or ALCAM (p = 0.60). For details, see Table 2.
Table 2. Level of inflammatory markers (ANCOVA) before adjustment for cardiometabolic risk factors

*p < 0.05, **p < 0.01, ***p < 0.001 compared to HC after adjustment for age, gender and Bonferroni correction. sIL-2R: soluble interleukin-2 receptor; sCD14: soluble CD14; MIF: macrophage inhibitory factor; ALCAM: activated leukocyte cell adhesion molecule; n: number; s.d.: standard deviation; n = number; n.s.: not significant; ALL: all patients; SCZ: schizophrenia; AFF: affective; HC: healthy controls; ANCOVA: analysis of covariance.
Analysis of the two diagnostic groups (SCZ and AFF) compared to HC separately showed that CXCL16 (p = 0.04) and sIL-2R (p = 1.1 × 10−5) were significantly higher in the SCZ group while non-significant differences were found in the AFF group after correction for multiple testing. sCD14, MIF and ALCAM were not significantly different between any of the separate diagnostic groups and HC.
The correlation analyses of cumulative DDDs of different medication groups and inflammatory markers did not reveal any significant associations (online Supplementary Table 1).
The correlation analyses of core symptoms and inflammatory markers did not reveal any strong associations. There was a weak correlation between sIL-2R and GAF-S (r = −0.07), and between ALCAM and CDSS (r = −0.08), which did not remain significant after correction of multiple testing (online Supplementary Table 2).
Inflammatory markers after adjustment for cardiometabolic risk factors
The correlation analyses of cardiometabolic risk factors and inflammatory markers are presented in Table 4. Total cholesterol was included as covariate for all of the inflammatory markers in addition to BMI and glucose for ALCAM. After adjustment for cardiometabolic risk factors and confounders, levels of sIL-2R (p = 0.001) were higher, sCD14 (p = 0.002) and ALCAM (p = 0.03) were lower in patients compared to HC (Fig. 1). For the other inflammatory markers there were no significant differences between patients and HC after adjustment for cardiometabolic risk factors (Tables 3 and 5).
Table 3. Difference in inflammatory markers in patients and HC after adjustment for cardiometabolic risk factors (ANCOVA)
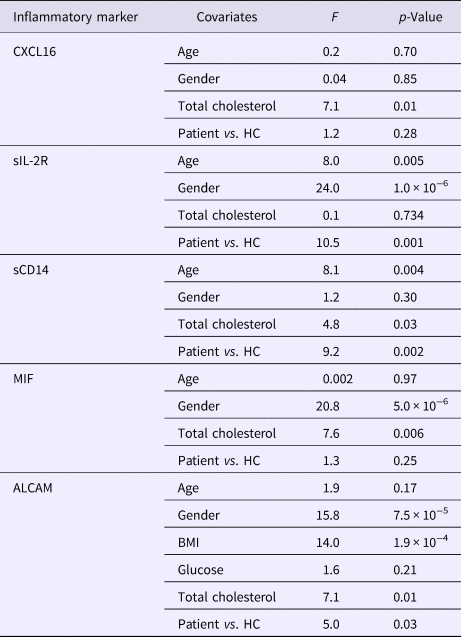
sIL-2R: soluble interleukin-2 receptor; sCD14: soluble CD14; MIF: macrophage inhibitory factor; ALCAM: activated leukocyte cell adhesion molecule; BMI: body mass index; HDL: high density lipoprotein cholesterol; LDL: low density lipoprotein cholesterol; HC: healthy controls; df: degrees of freedom; F: F-test; ANCOVA: analysis of covariance.
Table 4. Partial bivariate correlations with Pearson coefficients between inflammatory markers and cardiometabolic risk factors controlling for diagnosis

*p < 0.05, **p < 0.01, ***p < 0.001, sIL-2R: soluble interleukin-2 receptor; sCD14: soluble CD14; MIF: macrophage inhibitory factor; ALCAM: activated leukocyte cell adhesion molecule; CVD: cardiovascular disease; BMI: body mass index; HDL: high density lipoprotein cholesterol; LDL: low density lipoprotein cholesterol; mmol: millimoles per liter; kg: kilograms; m: meter.
Table 5. Level of inflammatory markers after adjustment for cardiometabolic risk factors (ANCOVA)

*p < 0.05, **p < 0.01, ***p < 0.001 compared to HC (ANCOVA), sIL-2R: soluble interleukin-2 receptor; sCD14: soluble CD14; MIF: macrophage inhibitory factor; ALCAM: activated leukocyte cell adhesion molecule; ALL: all patients; SCZ: schizophrenia; AFF: affective; HC: healthy controls.
a Adjusted for age, gender, total cholesterol.
b Adjusted for age, gender, BMI, glucose, total cholesterol.
Analysis of separate diagnostic groups revealed that sIL-2R was significantly higher (p = 3.0 × 10−4) and sCD14 lower (p = 0.01) in the SCZ group while non-significant differences were observed in the AFF group, after correction for multiple testing. No significant differences in ALCAM, CXCL16, and MIF between separate diagnostic groups and HC were observed. Total cholesterol was used as the lipid parameter in all these analyses, but similar pattern was shown also when including TG, LDL or HDL cholesterol (data not shown).
To confirm that our findings were stable, we repeated the analyses in a subsample assessed after 1 year (online Supplementary Tables 3 and 4). After controlling for cardiometabolic factors, a significantly lower level of sCD14 (p = 0.01) and a trend level increase of sIL-2R (p = 0.07) was found in the patient group. The reduction in sCD14 was also significant in the SCZ subgroup (p = 0.04).
Receiver operating characteristic (ROC) curve analysis revealed that all of the inflammatory markers had low predictive power (area under the curve < 0.6) with low sensitivity and specificity (online Supplementary Table 9).
Discussion
The main findings of the current study were increased levels of sIL-2R and reduced levels of sCD14 and ALCAM in severe mental disorders with the most prominent alterations in the SCZ group. The most marked changes were observed for sIL-2R, suggesting a marked T cell activation in these patients accompanied by monocyte activation as suggested by decreased levels of sCD14. The differences remained significant after controlling for comorbid cardiometabolic risk factors and multiple testing suggesting that this phenotype is a characteristic of severe mental disorders per se.
The current results of an independent association of immune abnormalities in severe mental disorders after controlling for cardiometabolic risk factors may support the concept of an independent causative effect of the immune system in mental disorders. This is in line with recent studies indicating immune-related genes in severe mental illness, particularly SCZ pathophysiology (Schizophrenia Working Group of the Psychiatric Genomics Consortium, 2014; Sekar et al., Reference Sekar, Bialas, De Rivera, Davis, Hammond, Kamitaki, Tooley, Presumey, Baum, Van Doren, Genovese, Rose, Handsaker, Daly, Carroll, Stevens and Mccarroll2016). Some of the literature on inflammatory abnormalities in SCZ and AFF groups could be biased by comorbid cardiometabolic risk factors particularly in higher age groups where such risk factors are more prevalent. There is great heterogeneity between previous studies in respect to potential confounders included (Goldsmith et al., Reference Goldsmith, Rapaport and Miller2016). Several studies exploring different aspects of inflammation in this patient group have included BMI as a confounder (Hope et al., Reference Hope, Dieset, Agartz, Steen, Ueland, Melle, Aukrust and Andreassen2011; Russell et al., Reference Russell, Ciufolini, Gardner-Sood, Bonaccorso, Gaughran, Dazzan, Pariante and Mondelli2015; Morch et al., Reference Morch, Dieset, Faerden, Hope, Aas, Nerhus, Gardsjord, Joa, Morken, Agartz, Aukrust, Djurovic, Melle, Ueland and Andreassen2016). Cholesterol levels have been controlled for in few studies (Dieset et al., Reference Dieset, Hope, Ueland, Bjella, Agartz, Melle, Aukrust, Rossberg and Andreassen2012), and these studies reported altered cytokine levels in line with our own findings.
The most prominent finding in the current study was the increased levels of sIL-2R in severe mental disorders and in particular in SCZ. sIL-2R is produced by activated T cells, and it has been reported to be increased in severe mental disorders in many studies both during acute and chronic illness phases (Goldsmith et al., Reference Goldsmith, Rapaport and Miller2016). IL-2 is the main growth factor for T cells (i.e. the inflammatory Th1 subtype), and sIL-2R, reflecting shedding of IL-2 receptor alpha from activated T cells, is a stable and robust marker of IL-2 signaling (Witkowska, Reference Witkowska2005). In contrast to IL-2, it circulates at readily detectable levels, and is a stable and reliable marker of T cell and in particular Th1 cell action in clinical samples. However, it has also been found to be involved in CVD (Durda et al., Reference Durda, Sabourin, Lange, Nalls, Mychaleckyj, Jenny, Li, Walston, Harris, Psaty, Valdar, Liu, Cushman, Reiner, Tracy and Lange2015). Here we show that sIL-2R is significantly elevated also after controlling for cardiometabolic risk factors. Thus, these findings suggest that T cell activation could have an independent pathogenic role in severe mental disorders. There are some studies that show that patients with SCZ have a blunted T cell response and in particular in the Th1 cell subsets which may seem in contrast to present findings (Bergink et al., Reference Bergink, Gibney and Drexhage2014). However, our findings suggest a marked T cell activation in vivo in these disorders which in fact may predispose to impaired T cell response when further activated in vitro. From a mechanistic point of view, T cell activation and in particular the balance between Th1 and Th2 cells have been shown to influence of the tryptophan/kynurenine metabolism-mediated serotonergic and glutamatergic abnormalities in major depressive disorder and SCZ (Kim et al., Reference Kim, Myint, Lee, Han, Lee, Kim and Leonard2004; Irwin and Miller, Reference Irwin and Miller2007; Muller et al., Reference Muller, Krause, Weidinger and Schwarz2014). Our findings herein support a persistent T cell activation, most probably reflecting Th1 cell activation in these patients also in vivo supporting the concept of T cells as a potential major player in these disorders (Khandaker et al., Reference Khandaker, Cousins, Deakin, Lennox, Yolken and Jones2015). Although different inflammatory markers may have overlapping functions, there are distinct differences in patterns of activation and levels which may explain why inflammatory markers representing T cell activities like sIL-2R and CXCL16 show different results in this patient group.
In contrast to the consistent finding of increased levels of sIL-2R in severe mental disorders, we somewhat surprisingly found decreased levels of sCD14 and ALCAM in these disorders and in particular in SCZ after correcting for cardiometabolic risk factors, which also may seem in contrast with some previous studies (Johansson et al., Reference Johansson, Jakobsson, Fortgang, Zetterberg, Blennow, Cannon, Hultman, Wetterberg and Landen2016). The reason for these findings is at present not clear, but several, not mutually exclusive explanations could exist. First, these markers and in particular sCD14 have shown to be elevated in CVD and in patients with high risk for developing CVD (Idzkowska et al., Reference Idzkowska, Eljaszewicz, Miklasz, Musial, Tycinska and Moniuszko2015; McKibben et al., Reference Mckibben, Margolick, Grinspoon, Li, Palella, Kingsley, Witt, George, Jacobson, Budoff, Tracy, Brown and Post2015). Therefore, given the higher risk of CVD among patients compared to controls, it is not inconceivable that previous studies of patients with severe mental disorders that have not adjusted for these risk factors have overestimated the levels of these markers in severe mental disorders. Indeed, in our study the difference in these markers between patients and HC was more prominent (and decreased) when adjusting for cardiometabolic risk factors. Second, there are also some other studies reporting normal monocyte activation in SCZ as compared with increased monocyte activation in depressive disorders as assessed by neopterin levels (Muller, Reference Muller2014). Finally, while the innate immunity may play a role in the pathogenesis of severe mental disorders, it may not be enhanced but rather decreased activation that are involved. In animal models of multiple sclerosis (MS), CD14 deficiency is associated with increased disease severity and inflammatory infiltration of monocytes and neutrophils within the central nervous system (CNS) (Walter et al., Reference Walter, Doering, Letiembre, Liu, Hao, Diem, Bernreuther, Glatzel, Engelhardt and Fassbender2006; Halmer et al., Reference Halmer, Davies, Liu, Fassbender and Walter2015). It has also been suggested that T cells may suppress CD14 for impediment of damage recognition (Janova et al., Reference Janova, Bottcher, Holtman, Regen, Van Rossum, Gotz, Ernst, Fritsche, Gertig, Saiepour, Gronke, Wrzos, Ribes, Rolfes, Weinstein, Ehrenreich, Pukrop, Kopatz, Stadelmann, Salinas-Riester, Weber, Prinz, Bruck, Eggen, Boddeke, Priller and Hanisch2016), and it may in fact be a link between T cell activation and impaired monocyte activation as suggested in the current study. Although our findings may challenge previous knowledge in this field, the role of innate immunity and monocyte/macrophages in severe mental disorders is still unclear and our findings in the current study will have to be confirmed in even larger studies that also include longitudinal testing. These studies should also include markers of the myriad of different monocyte subsets which may have different or even opposite roles in immunity.
We and others have previously reported raised levels of the cell adhesion molecule ALCAM in CVD (Smedbakken et al., Reference Smedbakken, Jensen, Hallen, Atar, Januzzi, Halvorsen, Aukrust and Ueland2011), and as for sCD14, ALCAM levels was found to be decreased in severe mental disorders when adjusting for cardiometabolic risk factors. ALCAM is found on endothelial cells including the BBB which has been shown to facilitate migration of lymphocytes across the BBB (Wagner et al., Reference Wagner, Bilinska, Pokryszko-Dragan, Sobczynski, Cyrul, Kusnierczyk and Jasek2014). Further, genetic variants of ALCAM have been implicated in development and progression of MS (Wagner et al., Reference Wagner, Bilinska, Pokryszko-Dragan, Sobczynski, Cyrul, Kusnierczyk and Jasek2014) and white matter brain pathology has also been implicated in SCZ (Najjar and Pearlman, Reference Najjar and Pearlman2015). ALCAM blockade has been shown to restrict transmigration of T cells and monocytes across the BBB indicating that ALCAM has an important function in maintaining BBB integrity (Cayrol et al., Reference Cayrol, Wosik, Berard, Dodelet-Devillers, Ifergan, Kebir, Haqqani, Kreymborg, Krug, Moumdjian, Bouthillier, Becher, Arbour, David, Stanimirovic and Prat2008). The reduced levels of ALCAM may indicate disruption of the BBB, but at present the implication of our finding of reduced ALCAM levels is unclear.
The current study has some limitations. First, it is still uncertain to what degree peripheral levels of circulating inflammatory markers reflect levels in the brain (Girgis et al., Reference Girgis, Kumar and Brown2014; Leboyer et al., Reference Leboyer, Oliveira, Tamouza and Groc2016). There are, however, increasing reports of communication across the BBB and the brain has also been shown to have lymphatic drainage (Louveau et al., Reference Louveau, Smirnov, Keyes, Eccles, Rouhani, Peske, Derecki, Castle, Mandell, Lee, Harris and Kipnis2015). Furthermore, peripheral blood levels of some inflammatory markers correlate with cerebrospinal fluid levels suggesting that circulating levels to some degree mirror changes in the CNS (Tomasik et al., Reference Tomasik, Rahmoune, Guest and Bahn2016). Second, we did not identify any correlations between symptom severity and the inflammatory markers in the current study; however, patients included in this study were usually in a more stable phase in their illness and we cannot rule out that the levels of inflammatory markers could be higher in the more acute phases. Third, for adjustment of cardiometabolic risk, we did not have data on blood pressure and arterial hypertension. Another limitation may be that previous infections may also influence inflammatory markers and finally, as plasma was not isolated immediately after blood collection, we cannot exclude ex vivo activation of inflammatory pathways or protein degradation. However, since samples were processed similarly in patients and HC, these effects would be random and more likely attenuate any differences between the study groups. Moreover, the combined sample of patients with severe mental disorders represents a heterogeneous group in terms of symptoms, and the diagnostic groups are also heterogeneous (Cuthbert, Reference Cuthbert2015). However, this effect would more likely attenuate the main results and lead to type-II errors. Interestingly, many argue that there might be a subgroup of patients who have immune alterations – ‘the immune phenotype’ – across the boundaries of the DSM diagnostic categories (Leboyer et al., Reference Leboyer, Oliveira, Tamouza and Groc2016). The low predictive power of this panel of markers as shown in the ROC analysis in terms of diagnostic groups may be due to heterogeneity within the groups and/or influenced by clinical phase of illness. To understand more of the role of immune activation in severe mental disorders other comorbidities like autoimmunity, malignancies and chronic obstructive pulmonary disease should be considered in future studies. Nevertheless, it is important to keep in mind that there may be other distinct differences between the patient group and HC that we did not control for like instance childhood maltreatment which may perhaps be a risk factor for increased inflammatory markers.
Our findings suggest that that patients with severe mental disorders and in particular those with SCZ are characterized by a marked T cell activation accompanied by indices of decreased monocyte activation that is seen even after correcting for cardiometabolic risk factors.
Our findings emphasize the importance of adjusting for co-morbidities and in particular CVD related markers and risk factors, when analyzing inflammatory markers in patients with severe mental disorders, a population with a high prevalence of these risk markers. Our findings also challenge previous knowledge in this field and suggest that the combination of enhanced T cell activation and impaired monocyte/macrophage activation may be a characteristic of these patients. The present findings and in particular their potential implication for the pathogenesis of severe mental disorders will have to be further investigated in forthcoming studies.
Supplementary material
The supplementary material for this article can be found at https://doi.org/10.1017/S0033291718004142
Author ORCIDs
Ragni H. Mørch, 0000-0001-7449-1269.
Acknowledgements
The authors would like to thank the participants of the study for their contribution, and the clinicians who were involved in patient recruitment and clinical assessments.
Author contributions
OAA, ID and RHM designed the study. The other co-authors (AF, ER, SH, EH, EG, MA, TI, IJ, GM, IA, IM, PA, SD, and TU) participated in data collection and quality control. RHM, ID and OAA were responsible for data analysis and interpretation. RHM, ID and OAA wrote the first draft of the manuscript. All authors contributed to and have approved the final manuscript.
Financial support
The Research Council of Norway (#213837, #223273, #217776), the South-Eastern (#2017-112, #2016-064), Western (#91141) Norway Regional Health Authority and KG Jebsen Stiftelsen (SKGJ-2011-36) are acknowledged for financial support.
Conflict of interest
OAA and RHM have received speaker's honorarium from Lundbeck. All other authors report no conflicts of interest.