Introduction
The Interbrand Report 2020 showed that most of the world's top brands (Apple, Amazon, Microsoft, and Google) are based on platform business models (Interbrand, 2020). A (digital) platform business enables value-creating interactions between external producers and consumers (Parker et al., Reference Parker, van Alstyne and Choudary2016). These types of business models are radically changing the understanding of how demand (consumers) and supply (producers) interact, especially in comparison to traditional pipeline businesses. A pipeline business, also known as a linear business, takes in components, combines these components to products or services, and sells the outputs to customers (Johnson, Reference Johnson2017). In contrast, a digital platform business serves as a virtual marketplace, where producers and consumers meet and exchange goods or services. Logically, the platform business has to have the means to serve two sides of the market sufficiently. Thus, the success of a digital platform business depends on its ability to attract producers and consumers to the platform, offer a suitable environment for an exchange between the participating entities, and guarantee their return for future exchanges (Parker et al., Reference Parker, van Alstyne and Choudary2016). To ensure this success, digital platforms rely heavily on Artificial Intelligence (AI) to optimize the targeting of customers, the delivery of information, matching the right participants (Buxmann and Schmidt, Reference Buxmann and Schmidt2019), and assessing the demand for products (Goli et al., Reference Goli, Zare, Tavakkoli-Moghaddam and Sadeghieh2019). Thus, digital platforms make use of intelligent decision-making. Intelligent decision-making lies in the heart of AI, is fully automated, and occurs in real-time (Buxmann and Schmidt, Reference Buxmann and Schmidt2019).
In the age of digital transformation, not only corporates but also startups are increasingly creating digital platform business models instead of linear ones. According to Blank and Dorf (Reference Blank and Dorf2012, p. 20), a startup is “a temporary organization in search of a scalable, repeatable, profitable business model”. Next to the temporal characteristic, startups are also characterized by their operations on low budgets. The characteristics alleviate the factors time and money to crucial elements for startups in the development of their business model (Ries, Reference Ries2011). To save time and money, it is important to know what prospective customers want and are willing to pay for. Traditionally, corporates applied the common method of business experiments to establish how well a customer received a new product or service. In doing so, managers relied heavily on past data, their intuition, and experience to launch new products or services. Taking a look into practice, however, has revealed that using data that captures the past was not a reliable measure to predict consumer behavior regarding innovations. Therefore, current research suggests that startupsand corporates apply observation- or survey-based business experiments in their creation of innovations and start with it in the early development stages of these products or services (Ries, Reference Ries2011; Thomke and Manzi, Reference Thomke and Manzi2014). The application of business experiments is a way of validating whether the business model is repeatable and scalable and, thus, helps startups and incumbents to gain insights into the execution of their strategy. Current validation processes for business models are generic for all kinds of enterprises. However, they are primarily designed for pipeline business models and disregard the logic of digital platforms (Ries, Reference Ries2011). If startups and corporates validate their digital platform business ideas with validation processes for pipeline business models, it is likely that the validation does not fulfill its purpose or might provide wrong insights due to the differences between pipeline and digital platform business models. As of the authors’ knowledge, no validation process exists which focuses on digital platform business models and incorporates the consumers’ and the producers’ sides in their validation.
Therefore, the purpose of this paper was to create a validation process for digital platform business models. The new validation process aims to increase the success rate of a digital platform business and limit the risk of building a product or service potential customers do not desire or are not willing to pay for. To create the validation process, the authors followed the Design Science Research methodology. This methodology served as a mental model and guided participants in designing solutions to identified problems by assessing current knowledge. In this particular research setting, prior research on validation processes was used and enriched with knowledge of digital platform business models to design a validation process that can be applied to digital platform business models of corporates or startups. Therefore, this paper answers the following research questions:
RQI: How can startups and corporates validate their digital platform business model using business experiments?
RQ2: How can the validation process be executed with limited investments available, precisely time and money?
The answers to these research questions were found by analyzing current validation processes for business models. The analysis revealed the existence of similarities between pipeline business models and digital platform business models, allowing a transfer of certain elements of the current validation process to the validation process of digital platforms. Based on these findings, the Smart Platform Experiment Cycle (SPEC) was designed and demonstrated by applying it in a startup with a digital platform business model.
This paper proceeds as follows. The next section elaborates on the theoretical framework, including relevant validation processes and the logic of the digital platform business models. Next, the Design Science Research methodology is explained and showed how it was used to design the SPEC, which is also presented in this section. Section “Demonstration in a real startup case” shows how SPEC was applied and externally validated by a startup, proving that it fulfills its purpose as a validation tool for digital platform business models. Finally, this research concludes with a discussion and future implications for researchers, practitioners, and corporates/startups dealing with innovations in a digital platform environment.
Theoretical framework
Definition of validation
Validation evaluates whether “the right product [was] built” and shows if “the product does what it is supposed to do in the intended operational environment” (Engel, Reference Engel2010, p. 17). In other words, validation shows if the product, service, or system fulfills the stakeholder's needs and to what extent a potential customer accepts the product, service, or system and deems it suitable. It is done “either during or at the end of the development process” (Engel, Reference Engel2010, pp. 14–15). Hence, it can be seen as a “continuous and systematic comparison” of the present state with the defined objectives (Albers et al., Reference Albers, Behrendt and Ott2010, p. 5). According to Albers et al. (Reference Albers, Behrendt, Klingler, Reiß and Bursac2017), validation activities reveal if customer needs are met, and technical requirements are followed. In this regard, the term verification is often used as well since they are correlated. Generally, verification indicates an internal process evaluating if a product, service, or system meets the agreed requirements and specifications or complies with regulations or defined conditions set at the start of product development. Essentially, verifying a product or service intends to answer the questions “was the product built (written, built, coded, assembled, and integrated) correctly”. Consequently, these two aspects point out the internal and external perspectives in developing products, services, or systems needing consideration (Blank and Dorf, Reference Blank and Dorf2012, p. 27).
Linear validation methods
Business experiments
Experiments are a specific form of survey or observation under controlled conditions (environmental factors are excluded). Experiments aim to “measure the effect that an action has on a situation by demonstrating a causal relationship or determining conclusively that one thing is the result of another” (Martin and Hanington, Reference Martin and Hanington2012, p. 20). More precisely, in “an ideal experiment, the tester separates an independent variable (the presumed cause) from a dependent variable (the observed effect) while holding all other potential cause constant, and then manipulates the former to study changes in the latter” (Thomke and Manzi, Reference Thomke and Manzi2014, p. 4). Depending on the way the results are determined, a distinction is made between a survey experiment (e.g., price of a product and purchase intent) and an observation experiment (e.g., in the case of comparable customers, prices are lowered in a company, and the changes in turnover are observed) (Weis and Steinmetz, Reference Weis and Steinmetz2008).
Through experiments, businesses can gain a new understanding of strategy execution as it reveals the “potential impact and the value of tactical changes” (Davenport, Reference Davenport2009). Blank and Dorf (Reference Blank and Dorf2012) integrated business experiments in their work and developed the Customer Development Process; a process that utilizes the traditional cycle of experimenting as a foundation to empirically validate each component of the Business Model Canvas (BMC), which is formulated as testable hypotheses (Blank and Dorf, Reference Blank and Dorf2012). Ultimately, a business model is created with the help of customers. Ries (Reference Ries2011) called this method Lean Startup since it keeps all processes as lean as possible, including all experiments, to find out how to build a viable business model. Unlike in traditional strategic planning, the Lean Startup method uses experiments from the first day on and includes real products, tested on real customers, instead of developing them in Research and Development departments (Ries, Reference Ries2011). Despite early experiments, a certain degree of uncertainty still surrounds the results of experiments. To diminish the uncertainty, business experimentation undergoes a Four-Step Iterative Cycle that tests an array of solution concepts repetitively (Thomke, Reference Thomke2003).
Four-Step Iterative Cycle
Business experimentation is a useful identifier for doing business better (Thomke and Manzi, Reference Thomke and Manzi2014). According to Thomke (Reference Thomke2003), it is common that the direction of results is not obvious at first. Therefore, business experimentation is seen as a Four-Step Iterative Cycle, in which several solution concepts are generated and consecutively tested “against an array of requirements and constraints” (Thomke, Reference Thomke2003, p. 92). The Four-Step Iterative Cycle consists of the steps (1) design, (2) build, (3) run, and (4) analyze. It is repeatable and might involve “multiple individuals, groups, or departments” (Thomke, Reference Thomke2003, p. 94).
Figure 1 shows the Four-Step Iterative Cycle. In step (1) design, a tester defines learning goals for the experiments by reviewing “existing data, observations, and prior experiments” (Thomke, Reference Thomke2003, p. 95). Furthermore, concepts and hypotheses are formulated through creative methods such as brainstorming (Thomke, Reference Thomke2003, p. 95; Ries, Reference Ries2011). Afterward, different experiments are chosen, which run in parallel and are analyzed simultaneously. The tester usually brainstorms different designs, which show radical changes compared with the existing design (Thomke, Reference Thomke2003). Interestingly, practice has shown that radical changes are not necessary, and minor changes would be sufficient to reach the learning goals (Thomke and Manzi, Reference Thomke and Manzi2014). In step (2) build, the needed prototypes to conduct the experiments are built either virtually or physically. To execute step (3), run the experiments, either a real setting or an artificial environment at the laboratory is chosen. When choosing the laboratory setting, the tester should be aware that potential errors might remain undetected since real circumstances cannot be replicated fully. In step (4) analyze, the results of the experiments are analyzed by comparing them to predefined expectations. The findings are used to adjust the understanding of the experimented object. As a result, the analyze step is seen as the step which generates the greatest learning about the cause-and-effect relationship. By using the Four-Step Iterative Cycle, the tester can gain insights and learn about possible solutions and errors, which have not been considered before. The findings guide the tester to “revise and refine the solutions” until “an acceptable result” is found by repeating the cycle (Thomke, Reference Thomke2003, p. 93). As soon as the hypotheses can be validated sufficiently, the experiments can be stopped. Otherwise, the findings are used as a basis for adjusting the experiment and starting the cycle anew (Thomke, Reference Thomke2003).

Fig. 1. Four-Step Iterative Cycle (own presentation based on Thomke (Reference Thomke2003)).
Lean Startup
According to the Lean Startup methodology, finding the right business model for a startup is the outcome of experiments, while finding the right product is the steps of business experimenting (Ries, Reference Ries2011). Similar to traditional business experimentation, the Lean Startup methodology uses an iterative cycle to learn about the product that fits the customer most. Additionally, customer feedback is used to identify the product that provides the highest benefit. Based on business experiments, Ries created the Build-Measure-Learn (BML) feedback loop that serves as the core of the Lean Startup method. Here, customers generate feedback and data after interacting with products. Qualitative feedback reflects how satisfied customers are with the performance or the service, while quantitative feedback shows how many customers have used the product. Regarding the BML feedback loop, entrepreneurs are aiming to reduce the overall lead-time of the feedback loop. The goal is to obtain the greatest possible feedback with minimal effort by building a product for a particular target group, such as investors or early adopters with certain characteristics in the shortest possible time and minimizing waste (Ries, Reference Ries2011). Figure 2 shows the BML feedback Loop. Only the interplay of all activities ensures entrepreneurial thinking. The BML feedback loop consists of three phases: (1) build, (2) measure, and (3) learn. Unlike in business experiments, where hypotheses are built based on observations, data, and previous experiments, startups build their hypotheses based on assumptions. Additionally, startups distinguish between a value hypothesis and a growth hypothesis.

Fig. 2. Build-Measure-Learn feedback loop (own presentation based on Ries (Reference Ries2011)).
In the step (1) build phase, it is essential to build the Minimum Viable Product (MVP) fast. The MVP represents a product that features the smallest possible set of functions desired by the customers (Ries, Reference Ries2011; Blank and Dorf, Reference Blank and Dorf2012). As startups have limited resources available, it would be a waste of time and money to build a detailed product based on an idea since it is not clear which functions the customer would use most or would be willing to pay for later (Ries, Reference Ries2011). The MVP aims to find out whether the proposed product offers a solution for the customer and if the customer is willing to pay for this solution, essentially revealing customers’ wishes. The MVP is considered the fastest way to go through the BML feedback loop (Ries, Reference Ries2011). The measure phase (2) aims to test the hypotheses made about the quality, price, and costs of the product. In this state, a quantitative approach called innovation accounting should be applied, which reveals if the “engine-tuning efforts” come to fruition and is desired by the customer (Ries, Reference Ries2011, p. 77). Every step in the experiment is considered a learning milestone that shows the progress the startup makes accurately and objectively. The third phase, learning, is similar to step four of the Four-Step Iterative Cycle and reveals whether the formulated hypotheses can be verified or not. It also indicates if the strategy of the startup realized by the MVP fulfills the customer's needs or if a change of the strategy is necessary (Ries, Reference Ries2011). Since the likelihood exists that a reformulation of the strategy is in order, it is important to go through the BML feedback loop fast and invest in the MVP.
Customer Development Process
The Customer Development Process is a tool that organizes the search for a business model (phase one) and the execution of the business model (phase two) with a scalable product. The process consists of four steps: step (1) customer discovery and step (2) customer validation, both representing the search of the business model. Step (3) customer creation and step (4) company building represent the execution of the business model that has been developed, tested, and verified in the previous steps (Blank and Dorf, Reference Blank and Dorf2012).
Customer discovery and customer validation are significant for startups because both phases deal with the MVP and early adopters (Ries, Reference Ries2011). The combination of the two steps “refine[s], corroborate[s], and test[s] a startup's business model”. Upon their completion, the verification of “the product's core features, [and] the market's existence” is finalized. Additionally, customers are located, “the product's perceived value and demand” tested, “the economic buyer” identified, “pricing and channel strategies” established, and “the proposed sales cycle and process” checked. The Business Model Execution phase is only entered when a respectable sized customer group with repeated sales and a profitable business model is validated (Blank and Dorf, Reference Blank and Dorf2012, pp. 27–28). The customer creation step stimulates demand by end-users and thereby scales the business. The last step, company building, turns the startup into a company (Blank and Dorf, Reference Blank and Dorf2012). Figure 3 shows the Customer Development Process.

Fig. 3. Customer Development Process (own presentation based on Blank and Dorf (Reference Blank and Dorf2012)).
The Customer Development Process is illustrated “as a circular track with recursive arrows”, signaling the iterative nature of startups since several adjustments are made until the right business model is found (Blank and Dorf, Reference Blank and Dorf2012, p. 23). The iterative process results in the extraction of a factual business model for the startup and ensures its success as a profitable and growing business. The customer discovery is the first step and turns each section of the BMC into testable hypotheses. Experiments are developed for each hypothesis to validate or falsify the existing business model.
Customer discovery and customer validation are steps that test the business model of the startup and determine if there is a market for the product and if the customers are willing to pay for it. It happens, however, quite often in the customer discovery and customer validation step that some hypotheses turn out to pivot. It is important to note that a pivot is not considered a failure (Blank and Dorf, Reference Blank and Dorf2012). Pivots are major changes to the components of the BMC due to customer feedback (Ries, Reference Ries2011; Blank and Dorf, Reference Blank and Dorf2012). Adapting the BMC and changing course throughout the development of the most valued product is a significant part of a startup. It is, in fact, better to pivot along the way instead of designing and producing a detailed product and afterward being faced with the fact that the customer does not need the product nor is willing to pay for it. If so, it would have been a waste of resources (money and time) that startups have to avoid (Ries, Reference Ries2011; Bank, Reference Bank2014; Vogels, Reference Vogels2016). Thus, startups go through these two steps several times until the educated guesses are proven facts and are scalable. After these two phases, there is no possibility to pivot.
Digital platform definition and canvas
Digital platform definition
There is a major distinction between pipeline and platform business models. Most of the currently existing business models are pipeline and product-oriented business models. The business logic of these models apply a sequenced and linear combination of input-throughput-output logic. Value is produced upstream and consumed downstream. It can be symbolized by a linear flow, much like water running through a pipe. In contrast, a “platform is a business based on enabling value-creating interactions between external producers and consumers” (Parker et al., Reference Parker, van Alstyne and Choudary2016, p. 5). It is not only a mobile app, website, or as often falsely believed a technology. A platform is considered a business model (Goettlinger, Reference Goettlinger2016; Moazed and Johnson, Reference Moazed and Johnson2016) that facilitates “efficient social and business interactions conciliated by software” (Choudary, Reference Choudary2015, p. 18). According to Moazed and Johnson (Reference Moazed and Johnson2016), a platform has four core functions. It must be able to (1) attract and (2) match an interdependent group of users, (3) offer an “open, participative, plug-and-play infrastructure”, and (4) should manage “social and economic interactions” (Choudary, Reference Choudary2015, p. 24) that emerge between users. Established rules manage the interactions within the network, which also promote trust and quality. These four core functions are supported by intelligent decision-making that promotes, for instance, the success of the matching activities of a digital platform. To illustrate, Buxmann and Schmidt (Reference Buxmann and Schmidt2019) have created an algorithm that supports the task of recruitment agencies in finding and matching job candidates based on abilities and skills stated in CVs with job openings in their database. As such, digital platform business models use AI to create value more efficiently, for example, by matching the right producers and consumers (Buxmann and Schmidt, Reference Buxmann and Schmidt2019) or by identifying the behavior of participants and adjusting their offerings accordingly (Sánchez et al., Reference Sánchez, Sánchez-Medina and Pellejero2020). Knowing the participants’ behavior is especially important as the value creation of a digital platform is based on the exchange of information, goods, or services and a type of currency between the participants on the platform (Parker et al., Reference Parker, van Alstyne and Choudary2016, p. 36; Fig. 4).

Fig. 4. Structure of a digital platform.
The exchange of information is considered the starting point of every platform since information is transferred first, even if the platform's main purpose is to exchange goods or services. As a result, the platform must initially be designed for collecting and transferring information to enable eventual exchanges of goods or services (Parker et al., Reference Parker, van Alstyne and Choudary2016). The initial exchange of information results in the exchange of goods or services (the value unit) and can be exchanged either through the platform or outside of it (Parker et al., Reference Parker, van Alstyne and Choudary2016). Lastly, the exchange of a type of currency occurs since the customer pays in some way for the good or service received; it can be either monetary or social. The customer pays either money via “credit card data, a PayPal transaction, a Bitcoin transfer, or (rarely) physical cash” (Parker et al., Reference Parker, van Alstyne and Choudary2016, p. 37). As an alternative, customers also pay the producer with social value. By attracting attention to the producer or by writing positive reviews about their experience, customers can increase the producer's fame and influence on the platform and beyond. Furthermore, the platform itself wants to receive a monetary reward for the services it offers. Platforms that exchange monetary currency as payment ensure that this transaction occurs internally and charges a fee for facilitating the interaction. Platforms with intangible forms of payment allow third parties to advertise their products on their sites and charge them for these services (Parker et al., Reference Parker, van Alstyne and Choudary2016). Platform businesses offer and govern the environment for an exchange of resources the participants bring into the business. Hence, platforms grow much faster and are revolutionary compared with the traditional linear pipe businesses (Choudary, Reference Choudary2015; Moazed and Johnson, Reference Moazed and Johnson2016; Parker et al., Reference Parker, van Alstyne and Choudary2016). According to Choudary (Reference Choudary2015) and Moazed and Johnson (Reference Moazed and Johnson2016), linear pipe businesses produce their own goods or services and sell them downstream through the supply chain. It is why pipeline businesses, unlike platforms, own and control their resources, generate growth of the business by mergers and acquisitions, and create value by efficient processes throughout the entire supply chain. In contrast, a platform neither owns nor controls resources, such as inventory or stock. It is done by the participants (Choudary, Reference Choudary2015; Parker et al., Reference Parker, van Alstyne and Choudary2016). Platforms offer the participants the infrastructure to facilitate the exchange of resources among the users, thereby making it mutually rewarding, also for the platform itself since it benefits from the network effect (Choudary, Reference Choudary2015; Parker et al., Reference Parker, van Alstyne and Choudary2016). The network effect is considered the impact the number of users have on the value created for the users on a platform (Shapiro and Varian, Reference Shapiro and Varian1998; Farrell and Klemperer, Reference Farrell, Klemperer, Armstrong and Porter2007; Parker et al., Reference Parker, van Alstyne and Choudary2016).
Although many companies make use of the platform business model, platform businesses can take on various functions. They can be categorized into nine different types and organized by the core value that is exchanged via the platform. As seen in Figure 5, the identified nine different types can be categorized into exchange platforms and maker platforms. The development platform can be further subcategorized into a closed development platform, a controlled development platform, and an open development platform. Therefore, 11 different platform types are shown instead of 9 (Moazed and Johnson, Reference Moazed and Johnson2016). The key attribute indicates that the difference between a maker and an exchange platform is the matching intention. It means the maximum number of units of an item a producer can exchange at a given time. In this regard, the matching intention of exchange platforms has a limited, discrete value (Moazed and Johnson, Reference Moazed and Johnson2016). To illustrate, an eBay seller has the choice to sell five identical T-shirts, but the matching intention for each unit of inventory is 1:1. It is because, after the closure of the auction, the T-shirts cannot be bought by another buyer. Furthermore, the interactions by Uber is 1:1 or 1:many connections, mostly because an Uber driver is looking for a passenger in a certain period, so s(he) is looking for one passenger or a group of passengers. In comparison, maker platforms do not have a limited matching intention; the interactions are 1:many connections. In theory, it is considered infinite (Moazed and Johnson, Reference Moazed and Johnson2016) because demographically seen many people are born each day, and everybody can watch the same YouTube video.
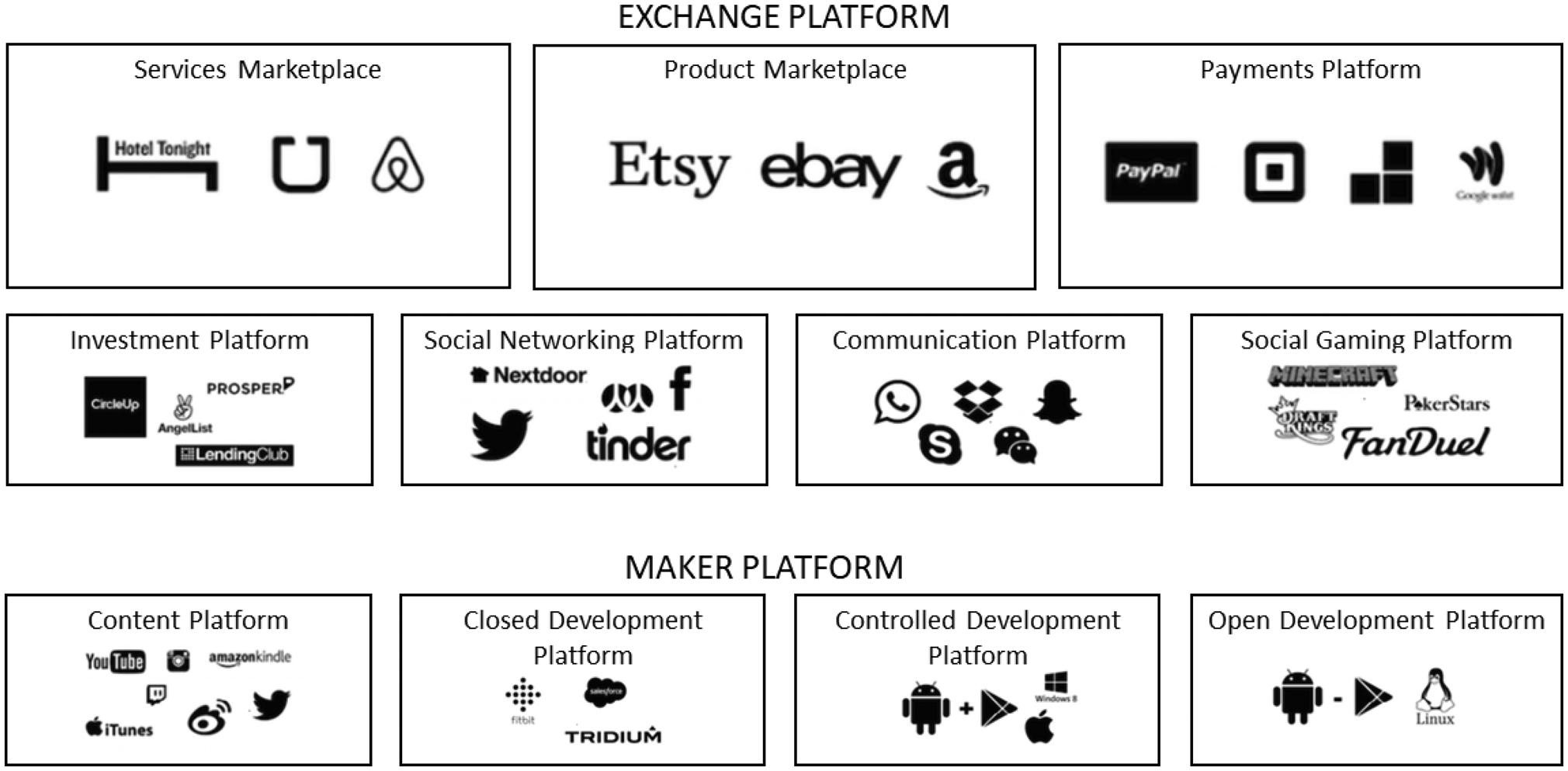
Fig. 5. Digital platform types (own presentation based on Moazed and Johnson (Reference Moazed and Johnson2016)).
This paper focuses on exchange platforms, more precisely, on the type of Service Marketplace, such as Uber and Airbnb. Service marketplaces are markets that operate between demand and supply and make use of the platform business model to serve its customers. Often, these types of markets are called two-sided markets. According to Drake (2015), a market is two-sided as soon as two parties and an intermediary are involved. The two parties are generally a buyer and a seller, while the intermediary is either a real or a virtual meeting place. Therefore, economic lectures often use the term two-sided platform (2SP) as a synonym for the two-sided market. The term 2SP was first identified in the paper Platform Competition in Two-sided Markets written by professors Jean Charles Roche and Jean Tirole in 2000 (Evans and Schmalensee, Reference Evans and Schmalensee2016). Besides, the professors proposed a formal definition for 2SP in 2006. They consider a “market [as] two-sided, if the platform can affect the volume of transactions by charging more to one side of the market and reducing the price paid by the other side by an equal amount”. In other words, the pricing strategy is of importance, and platforms have to design it attractively for both parties to participate (Rochet and Tirole, Reference Rochet and Tirole2006). In this light, a single nightclub business model, where men and women can meet and interact with each other, could be considered as a two-sided market (Evans and Schmalensee, Reference Evans and Schmalensee2016). The 2SP needs two different groups of customers: men and women. Often in nightclubs, one group proportion is higher than the other one, and attending the nightclub will become unattractive for the one group. To influence the volume of the two groups, the two-sided market allows the club owner to charge different prices to the groups,thereby influencing the proportions of participants and the attractiveness to attend the nightclub in general (Evans, Reference Evans2011). In conclusion, the term 2SP shows a certain way of organizing one's business by balancing and influencing two sides of the market by pricing strategies and benefiting from its effect on the number of participants. In addition to 2SP, the term multi-sided platform (MSP) appears in relation to platform business models. Similar to the 2SP, an MSP brings together a group of customers, which benefit from interacting with each other. On MSPs, the group of customers often consists of two or more interdependent entities and can only create value when all entities are present and interact with each other (Osterwalder and Pigneur, Reference Osterwalder and Pigneur2010). Therefore, the key function of an MSP is “to attract and serve all groups simultaneously”; however, the MSP thereby often faces the chicken- and egg-dilemma (Osterwalder and Pigneur, Reference Osterwalder and Pigneur2010, p. 78). The dilemma describes the issue arising when launching a platform. Both sides are needed for the success of the platform, but one side of the market is only attracted to the platform when the other side is present (Parker et al., Reference Parker, van Alstyne and Choudary2016). To solve this problem, MSPs “subsidiz[e] a customer segment” by attracting users to the side and offering free services while attracting another group of customers to the platform, for instance, advertisers, and charging them fully for their services (Osterwalder and Pigneur, Reference Osterwalder and Pigneur2010, p. 78). An important success factor for MSPs is to know which customer segment to subsidize to (1) increase the number of users and (2) to benefit from the network effect (Eisenmann et al., Reference Eisenmann, Parker and van Alstyne2006; Osterwalder and Pigneur, Reference Osterwalder and Pigneur2010).
Looking more closely at the definition of 2SP and MSP, the pricing strategy depicts the key difference between these two platform types. While a 2SP gains profit through both groups of customers by lowering the price for one group by an equal amount, it raises the price for the other, an MSP charges nothing for its service to one group but charges the other fully (Osterwalder and Pigneur, Reference Osterwalder and Pigneur2010; Parker et al., Reference Parker, van Alstyne and Choudary2016). However, nowadays, in the Internet era, economists do not distinguish between 2SP and MSP since it is very likely that platforms facilitate interactions between more than only two groups of customers. As a result, the distinction between a 2SP and an MSP became blurry, and the terms are used interchangeably by economists (Evans and Schmalensee, Reference Evans and Schmalensee2016).
The platform canvas
The platform canvas is a “central planning framework” that summarizes all platform elements “as a set of building blocks” (Choudary, Reference Choudary2015, p. 137). It is a tool to build an interaction-based platform business (Choudary, Reference Choudary2015). Figure 6 illustrates the platform canvas with each building block. As shown, producers are connected to the platform's tools and services regarding creation via channels and access control, whereas consumers are linked to the platform's consumption tools and services via channels and filters. Currency and capture are most important for the platform since it should generate profit for offering an environment for interaction.

Fig. 6. Platform canvas (Choudary, Reference Choudary2015).
To recall, a platform business offers producers and consumers an infrastructure to interact with each other (Choudary, Reference Choudary2015). Therefore, the starting point of a platform canvas is the interaction itself. The first four building blocks of the platform canvas are producer, consumer, platform, and value. While the producers create value, the platform facilitates the transfer of value to the consumer (Choudary, Reference Choudary2015). Producers and consumers are rolesdefined during platform design to get a better understanding of their motivation to participate. Value represents the supply or inventory on the platform and can adopt any form (Choudary, Reference Choudary2015). It is why platform design should define the value unit first.
The next phase of the platform canvas regards the “plug-and-play nature of a platform business” (Choudary, Reference Choudary2015, p. 24). The platform needs to be open enough to attract users to participate and simultaneously ensure that the system has “quality [ … ] and relevance” (Choudary, Reference Choudary2015, p. 141). It is done by the building blocks channels, access control, and filters. To encourage “open participation”, platforms can use different channels such as “websites, apps, widgets, or plug-ins” or channel partners that pull producers and consumers to the platform (Choudary, Reference Choudary2015, p. 140). By controlling the access to the platform, the platform can determine the type of producers and the value units, thereby ensuring quality for consumers. Access is also regulated by setting “editorial, algorithmic or social mechanism” for producers (Choudary, Reference Choudary2015, p. 141). Filters regulate the content delivered to the consumers and display only relevant value units to them, ensuring that they do not leave due to information overload (Parker et al., Reference Parker, van Alstyne and Choudary2016).
Tools and services connect the building blocks of the platform canvas that facilitate the interaction. It includes tools and services regarding creation, curation, and customization, as well as consumption (Choudary, Reference Choudary2015). Creation tools and services link producers to the platform by supporting the creation of value units. Tools and services of curation and customization enhance “features, functionalities, and services” on the platform, while consumption tools and services connect the consumer via channels and filters to the platform; it involves, among others, the “set-up of consumption interfaces, newsfeeds, [and] external widgets” (Choudary, Reference Choudary2015, p. 143).
The last two building blocks summarize the value captured by the platform, which is currency and capture. Currency is the payment made by the consumer to the producer as an exchange for the received value unit. The currency can be either monetary or social (Choudary, Reference Choudary2015; Parker et al., Reference Parker, van Alstyne and Choudary2016). Capture ensures that the platform generates profit through the business, either by charging the users “when actual money is exchanged” orE allowing third parties to access the platform and charging them for their service (Choudary, Reference Choudary2015, p. 144).
Monetization and launch of digital platforms
Monetization
Parker et al. (Reference Parker, van Alstyne and Choudary2016, p. 107) point out that the choice of the right pricing strategy “is one of the most difficult and fascinating issues” for a platform business. Failing might cause friction and counteracts the benefits of a potential network effect. It is recommended to use a monetization strategy only when the platform knows what value it creates (Parker et al., Reference Parker, van Alstyne and Choudary2016). The created value can either be (1) “value for consumers: access to value created on the platform” (e.g., videos on YouTube for the viewer), (2) “value for producers or third-party providers: access to a community or market” (video creators have viewers), (3) “for both consumers and producers: access to tools and services that facilitates interactions” (YouTube facilitates infrastructure to upload videos), or (4) “for both consumers and producers: access to curation mechanism that enhance the quality of interactions” (Parker et al., Reference Parker, van Alstyne and Choudary2016, p. 111). These value forms are the source of excess value created by the platform and should be exploited correctly (Parker et al., Reference Parker, van Alstyne and Choudary2016). Thus, literature identified four ways to monetize a platform, which are in line with the value forms (Parker et al., Reference Parker, van Alstyne and Choudary2016). Value Form (1): Charging a Transaction Fee. Charging a transaction fee is a monetization of value after a match was made and a transaction has occurred. Thus, the barrier for entry and participation is bypassed, and the growth of the network is not inhibited. Platforms have to find the right fee to be charged without discouraging the transaction (Parker et al., Reference Parker, van Alstyne and Choudary2016). Value Form (2): Charging for Access is done by charging producers for access to the platform who indirectly interact with the consumer. These are, for instance, producers of advertisements or producers that are interested in a large number of users (Parker et al., Reference Parker, van Alstyne and Choudary2016). Value Form (3): Charging for Enhanced Access provides producers with enhanced tools that give them competitive advantages. It enables them to stand out among the producers to attract consumers. Fees can be charged for “targeted messages, more attractive presentation, or interaction with particularly valuable users” (Parker et al., Reference Parker, van Alstyne and Choudary2016, p. 119). It is important to maintain the user's trust by distinguishing that content is part of enhanced access. Furthermore, it should be ensured that the content is embedded in the usual curation principles of the producer to maintain the user base (Parker et al., Reference Parker, van Alstyne and Choudary2016, p. 121). Value Form (4): Charging for Enhanced Curation. The larger a platform's content gets, the more likely the consumers will have difficulties in finding high-quality content, which ultimately reduces its value for the consumer. To avoid the loss of value, the platform can “ensure quality and choice” by “rigorous curation and screening” of the producers of the platform in exchange for a fee upon access to the platform (Parker et al., Reference Parker, van Alstyne and Choudary2016, p. 122). The question of whom to charge in a platform business might be difficult as well since a platform facilitates interaction between at least two or more different groups of users. The decision to charge one group might even cause unpredictable effects on the other groups involved (Parker et al., Reference Parker, van Alstyne and Choudary2016, p. 122). Traditional pricing logic, in contrast, disregards the network effect and tries to reach the highest revenue possible by charging both sides of the market (Yglesias, Reference Yglesias2014). Applying this pricing strategy in a platform environment would likely discourage participation and decrease the network effect.
Network effect
The network effect is a market-/growth-building tool that supports the creation of value and competitive advantage of a platform business. The network effect shows how the value of a product for one user depends on the number of other users (Shapiro and Varian, Reference Shapiro and Varian1998; Farrell and Klemperer, Reference Farrell, Klemperer, Armstrong and Porter2007; Parker et al., Reference Parker, van Alstyne and Choudary2016). To derive the definition of the network effect, two decisive factors need to be understood first: early adopters and positive feedback. Early adopters have “a major impact on the diffusion of an innovation [ … ] within a system” and are customers who like to use new innovative products (Conway and Steward, Reference Conway and Steward2009, p. 156). They are most likely to oversee mistakes and provide feedback on their user experience (Ries, Reference Ries2011, p. 61). Through positive feedback, early adopters encourage other users to join a network (Farrell and Klemperer, Reference Farrell, Klemperer, Armstrong and Porter2007). If the number of adopters reaches a certain threshold, the network inevitably attracts more and more users, consequently creating the network effect (Farrell and Klemperer, Reference Farrell, Klemperer, Armstrong and Porter2007). The threshold for the network effect is also known as the critical mass; the critical mass is important for the platform to overcome the chicken- and egg-dilemma (Shapiro and Varian, Reference Shapiro and Varian1998; Farrell and Klemperer, Reference Farrell, Klemperer, Armstrong and Porter2007). Other terms used for the network effect are network externalities or demand economies of scale (Shapiro and Varian, Reference Shapiro and Varian1998). The Oxford-Dictionary defines economies of scale as “a proportionate saving in costs gained by an increased level of production” (Oxford-Dictionary, 2020). In economics, externalities arise when the production or consumption of a product or service generates costs or benefits to individuals, which are not involved in producing the product or service (Begg and Ward, Reference Begg and Ward2013). Due to the rise in costs or benefits, the network effect can be either positive or negative (Shapiro and Varian, Reference Shapiro and Varian1998; Parker et al., Reference Parker, van Alstyne and Choudary2016). Positive network effects increase the value for each user and occur when a platform community is well-managed (Begg and Ward, Reference Begg and Ward2013; Parker et al., Reference Parker, van Alstyne and Choudary2016). In contrast, negative network effects occur when platforms are not well-managed and decrease the value for users (Begg and Ward, Reference Begg and Ward2013; Parker et al., Reference Parker, van Alstyne and Choudary2016). Since a network benefits from any additional user, the negative network effect is not a common occurrence (Shapiro and Varian, Reference Shapiro and Varian1998).
Nowadays, the positive network effect is also pushed to existing platform businesses through (demand) economies of scale. Significant for demand economies of scale is gaining competitive advantage by “technological improvements on the demand side”. Thus, it is a “fundamental source of [a] positive network effect” (Parker et al., Reference Parker, van Alstyne and Choudary2016, p. 19).
Figure 7 illustrates how the network effect influences market side one and market side two of the platform. As shown, the network effect can be distinguished into two types, the direct network effect, also known as the same-side effect, and the indirect network effect, also known as the cross-side effect.

Fig. 7. Network effect (own presentation based on Westhead (Reference Westhead2016)).
The chicken- and egg-dilemma
The chicken- and egg dilemma is the conflict that a platform faces during the launch stage. Both sides of the market – producers and consumers – are essential for the platform business, and both sides depend on the other's entry to make the platform attractive (Osterwalder and Pigneur, Reference Osterwalder and Pigneur2010; Moazed, Reference Moazed2015; Parker et al., Reference Parker, van Alstyne and Choudary2016). To illustrate, gamers (entity one) will only buy a certain game console (platform) if there are enough games available to use the console. Game developers (entity two) will only develop games when the number of gamers buying the game for that particular gaming console is high enough to make the development profitable (Osterwalder and Pigneur, Reference Osterwalder and Pigneur2010). In this example, it appears that user acquisition is more important to make the platform successful. However, practice has shown that user commitment creates long-run success (Parker et al., Reference Parker, van Alstyne and Choudary2016). To facilitate user commitment, platform businesses should focus on pull strategies (traditional pipeline businesses engage in push strategies) and should not only rely on creating awareness to accelerate adoption and usage of their goods and services (Parker et al., Reference Parker, van Alstyne and Choudary2016). In the digital era, the key factor of success is to design their goods and services attractively to pull consumers “naturally ( … ) into their orbit” (Parker et al., Reference Parker, van Alstyne and Choudary2016, p. 85). Furthermore, platform businesses can benefit from user commitment and the active usage of the platform as “true indicators [for] customer adoption” instead of “sign-ups [or] acquisitions” (Parker et al., Reference Parker, van Alstyne and Choudary2016, p. 85). Therefore, it is important to reward participation by offering incentives that are “organically connected” to the interaction facilitated on the platform (Osterwalder and Pigneur, Reference Osterwalder and Pigneur2010, p. 79). Consequently, a platform business has to ensure that the applied launch strategy pulls users to the platform and incentivizes user commitment to guarantee long-term success (Parker et al., Reference Parker, van Alstyne and Choudary2016). Often this is done by subsidizing value to the early adopters in monetary terms through product features or user sequencing ( Osterwalder and Pigneur, Reference Osterwalder and Pigneur2010; Moazed, Reference Moazed2015). To solve or avoid the chicken- and egg dilemma, the literature has identified eight launch strategies that platform businesses can utilize independently or in combination (Osterwalder and Pigneur, Reference Osterwalder and Pigneur2010; Moazed, Reference Moazed2015; Parker et al., Reference Parker, van Alstyne and Choudary2016).
Design and development
Design Science Research methodology
The authors employed the Design Science Research methodology by Peffers et al. (Reference Peffers, Tuunanen, Rothenberger and Chatterjee2007) and applied the first four activities of this process model. Compared with traditional, description-oriented research, which discovers and justifies unexplained phenomena, design-oriented research designs and evaluates solutions for relevant problems (Peffers et al., Reference Peffers, Tuunanen, Gengler, Rossi, Hui, Virtanen and Bragge2006).
Following this design-oriented research approach, researchers, external startups, and corporates who were already exploiting digital platform business models came together in workshops to design and evaluate a new validation process. Following the first activity of design science research, problem identification, and motivation, the researcher examined current state-of-the-art approaches to validate business models. It was found that current approaches are all designed for pipeline business models, which follow an input-throughput-output logic. Validation processes for the orchestration of markets (digital platforms) were not found. Entering the second activity, Objectives of a solution, the participants set the objective to design a validation process that is applied to digital platform business models. For the design and development activity, the participants first examined pipeline business models and digital platform business models and extracted similarities between the models. With the similarities between a pipeline and digital platform in mind, the researchers looked at the elements in the validation processes that validate the similarities in the business models and extracted these as the element for the new validation process. In doing so, the participants remained consistent with the current literature and were more likely to be complete and robust with their modeling (Peffers et al., Reference Peffers, Tuunanen, Gengler, Rossi, Hui, Virtanen and Bragge2006). Next, the participants identified differences and used current literature to point out what elements and factors needed to be considered and added to validate a digital platform business model. In the case of startups, for instance, the factors time and money are crucial. In the case of platform business models, the two sides of the market had to be included as well. Figure 8 summarizes the presented linear validation methods, shows components of a digital platform, and illustrates an initial comparison to the platform validation process, which was designed in the research.
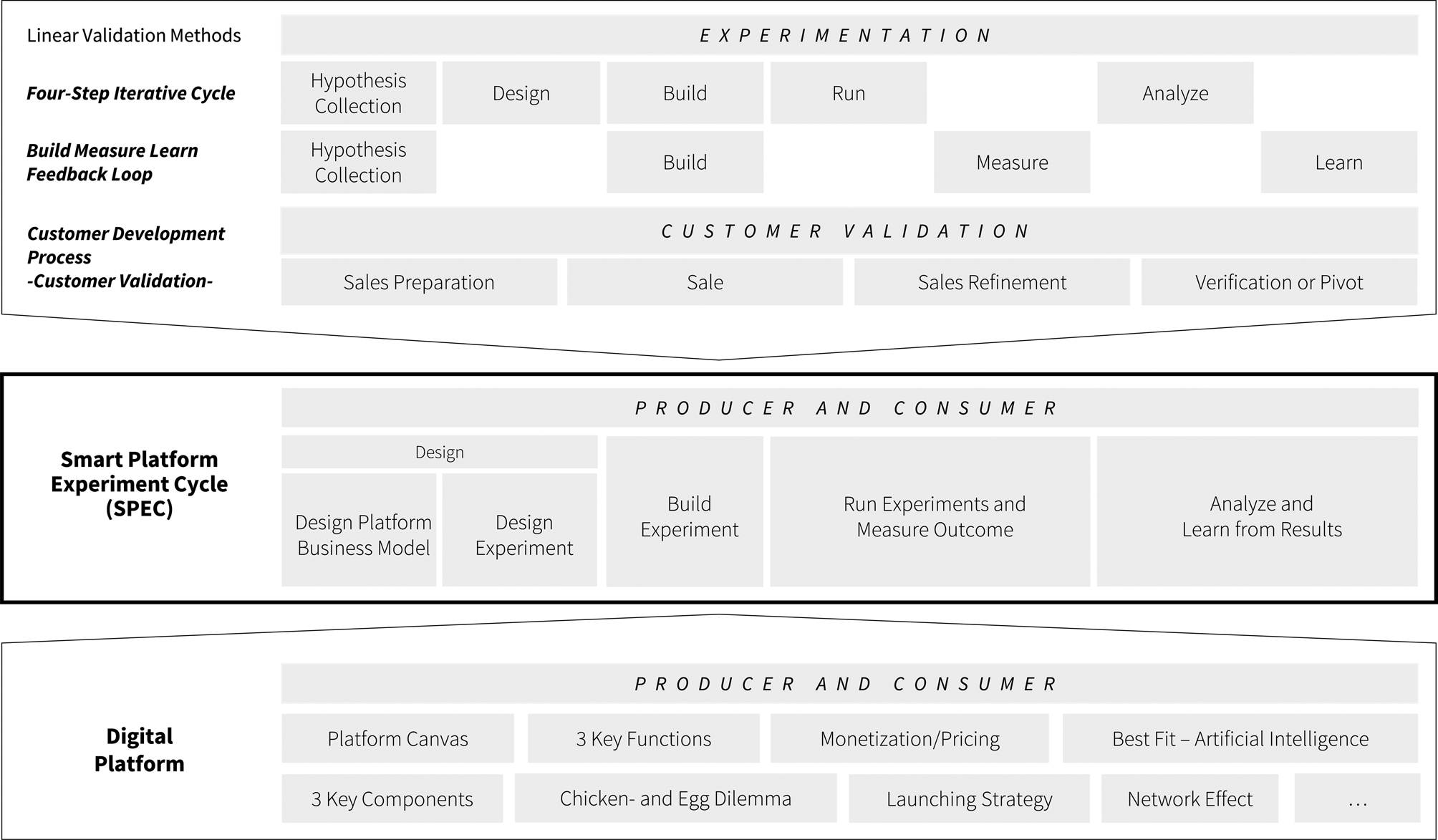
Fig. 8. Framework comparison.
To summarize, the researchers extracted the elements of existing validation processes and added important components based on the knowledge of digital platforms and startups. It resulted in the creation of an early market validation process for digital platform business models, a new process called the Smart Platform Experiment Cycle. Following the fourth step of the Design Science Research methodology, demonstration, SPEC was applied to test its efficacy in solving the identified shortcomings. Peffers et al. (Reference Peffers, Tuunanen, Gengler, Rossi, Hui, Virtanen and Bragge2006) suggest doing so by involving it “in experimentation, simulation, a case study, proof, or other appropriate activity” (p. 90). This research applied it to the startup GassiAlarm.
Smart Platform Experiment Cycle
The SPEC consists of five steps: (1) design the digital platform business model, (2) design experiments for consumers and producers, (3) build experiments including a MVP and implement a Measuring Metric, (4) run the experiments and measure the outcome, as well as (5) analyze and learn from the results. In more detail, in step (1) design the digital platform business model, the startup proves if the developed and verified business model, which might be structured in a BMC, includes the three key components of a platform business model: value unit, participants, and filter. Additionally, the three key functions pull, facilitate, and match are proven and (re)designed. Furthermore, pricing and launching strategies are defined. Finally, the Platform Business Model (PBM) is structured and visualized in a platform canvas of Choudary (Reference Choudary2015). It is worth mentioning that the designed digital platform business model in this step is only based on verified assumptions the startup has made.
In the second step (2) design experiments for consumers and producers, experiments regarding customer segments, sales channels, customer relationships, and pricing models are designed for both sides of the platform: producers and consumers. It also needs to be decided which building block of the designed platform business model is the most relevant to validate first and, therefore, to set up a strategic roadmap or timeline for the forthcoming experiments. For instance, designing the right pricing strategy for the platform is a very complex and difficult task. Charging the user at the wrong stage might cause friction upon entry and counteract the benefits of the network effect. A pricing strategy imposed at deal completion could avoid friction and encourage value creation. Additionally, the platform must decide whom to charge for the service since the users can take on many roles and ultimately impact the network effect. However, to design the pricing strategy, it needs to be clear who the customers of the digital platform are. To structure all experiments, the template “Hypothesis-MVP-Duration-Currency-Threshold-Experiment” based on MIT Global Entrepreneurship Bootcamp is used (Chen, Reference Chen2016). According to Thomke and Manzi (Reference Thomke and Manzi2014), several questions need to be answered to make the business experiment worth the time and effort: Does the experiment have a clear purpose? Thus, the learning goals, which should be achieved, should be defined first. If a hypothesis that needs to be tested is not well defined and stated, the test might be ineffective and cause additional costs with no helpful results. According to Yoskovitz (Reference Yoskovitz2011), the following template is used to define a well-structured hypothesis: “I believe [target market] will [do this action/ use this solution] for [this reason]”.
Once a hypothesis is defined, it needs to be clarified which method and environment are used to validate the MVP. Also, the duration of the experiment needs to be defined. Based on the hypothesis, the experimenting environment, the duration, a currency, and respective thresholds need to be determined. It is crucial to define how the hypothesis will be tested and how the outcome will be measured. Predefined thresholds help to decide whether an experiment was successful or not. Besides, it helps the experimenting team to remain impartial when it comes to the results (Fig. 9).
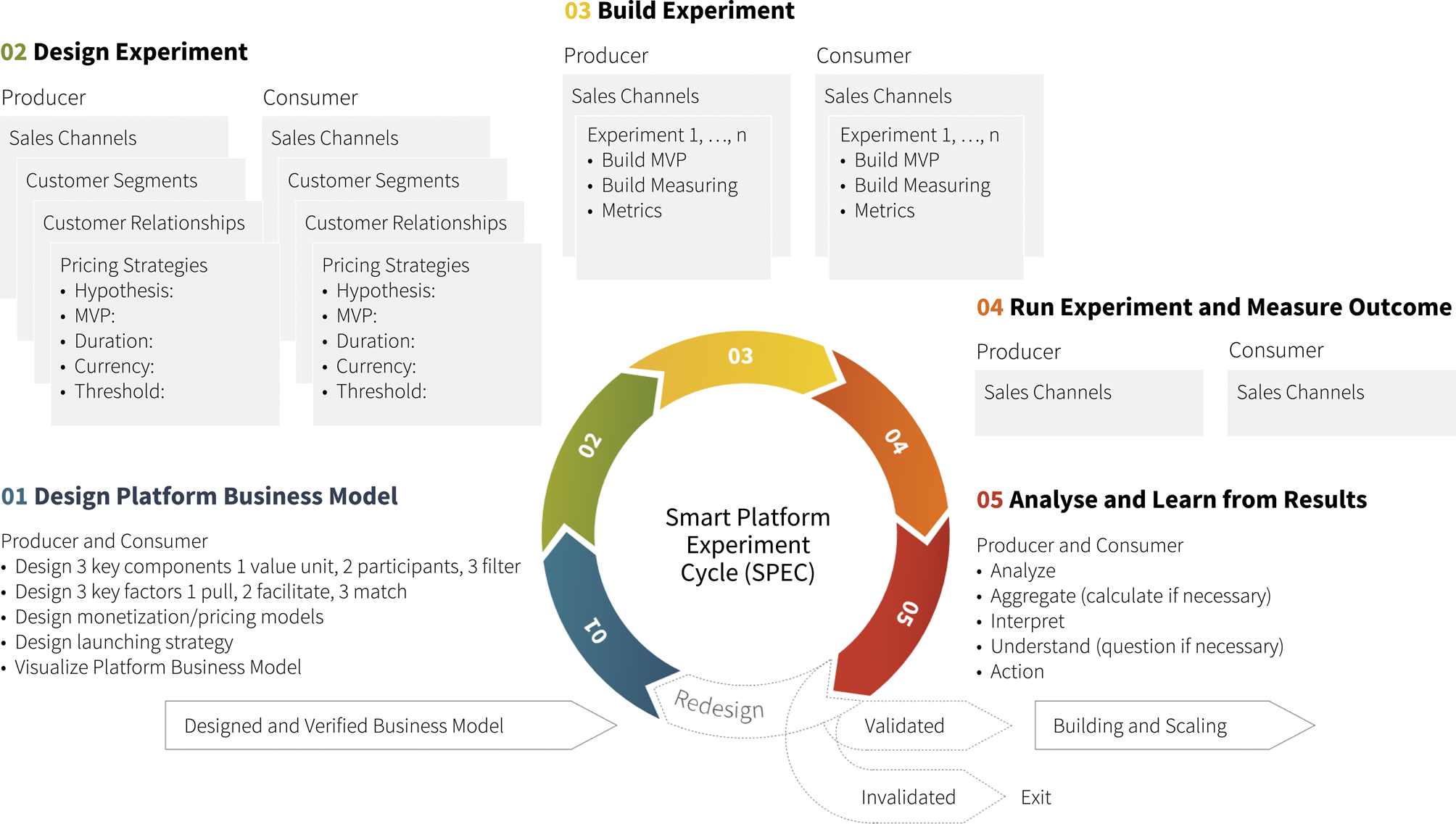
Fig. 9. Smart Platform Experiment Cycle (SPEC).
Step (3) is about building the experiments, including a MVP and implementing a Measuring Metric. For each element, for instance, customer segments, sales channels, customer relationships, and pricing models, different experiments must be built. A landing page or mockup (MVP) is designed and is presented to the customer, or more precisely, to the early adopters, as soon as possible. The early adopters are presented with a High-Fidelity MVP with more functions. The focus does not lie on developing a high-end product and spending a lot of time in the development, only to realize that the product will not be used or purchased by the customer. The MVP is developed fast and represents the solution to the perceived problem. By utilizing Call-To-Action (CTA), which is an invitation to the user, the user is motivated to be active on the website, while the implementation of buttons connects the user to the page. The two distinguished groups, the producers and consumers, are separated at registration on the webpage/mockup due to the different needs that need to be met. Moreover, a survey is implemented as an independent variable to control the answers at registration. A measuring metric tracks and monitors all steps of the experiment. The traffic on the webpage, for example, can be tracked and analyzed by Google Analytics.
In step (4) run experiments and measure the outcome, the startup should keep an eye on the test environment and the costs. If it is necessary, an adjustment of the experiments (1 … n) must be made.
In step (5) analyze and learn from the results, the startup must analyze the given data, calculate key performance indicators (KPIs), and learn from the qualitative and quantitative results. Experimentation means building, learning, and continuous improvement. SPEC is an iterative validation cycle since it indicates three possible steps, which can be taken, after concluding step (5) of the cycle. One result (5.1) might be a validated hypothesis based on real data, which leads either to the next iteration of SPEC or to the customer creation phase in the Customer Development Process (Blank and Dorf, Reference Blank and Dorf2012). Another possible result (5.2) might be an invalidated hypothesis, which signals a stop to all startup activities before more time and money is wasted. A third result (5.3) might be an invalidated hypothesis as well, but with data leading to new findings, which point out a possible redesign of the business model.
Demonstration in a real startup case
To validate the created SPEC, whichspecializes in digital platform business models, a real startup case was used. The startup GassiAlarm applied the new validation process in compliance with a given low budget of 1000 Euros. The objective was to validate if the considered digital platform business model was successful or not. In the case of GassiAlarm, two prototypes (websites) were developed and tested on 111 customers, who represent producers (dog owners) and consumers (dog sitters). Before using the SPEC, the founder-team developed a customer-oriented business model for dog care services according to Lean Startup and the Customer Development Process, focused on the Customer Discovery. A dog owner might not be able to take care of his or her dog for 365 days a year due to vacation or illnesses. In those cases, the dog owner needs a reliable person who can take care of the dog during his or her absence. In most German households, dogs are not merely seen as pets who protect the house; dogs are considered permanent family members. In contrast, some people love dogs but do not own a dog. Among these people, there is a subgroup (students), who like to go out with dogs and would like to have a part-time job with the fun factor “dog”. A digital platform business model might match these two distinct groups of users, the dog owners (producers) and the dog sitters (consumers). Hypothetically, the digital platform would pay itself through a service commission fee. GassiAlarm used SPEC to provide evidence that backs up the business idea and prevents it from failing without wasting money and time.
In step (1) design, the digital platform business model, GassiAlarm, implemented three key components: the value unit, the participants, and the filter. Dog owners produced the first value unit by asking for a dog care service for a certain period. In general, a dog owner can produce more value units when s(he) needs another dog sitter at another time. The second value unit, or more precisely, value units, were produced by the dog sitter when sharing activities and photos with the cared-for dog on the platform. This value unit showed the dog's well-being during dog care and was not only for the specific dog owner. Doing a good job could result in a recommendation of the sitter to other owners. As part of the participants, the producer is the dog owner who creates a value unit by asking for caretaking for a dog on the platform. The dog sitter represents the consumer side by looking for a part-time job and enjoys being with a dog. Their motivation for participating can either be through a monetary currency as they are being paid for their service or through social currency (e.g., attention) as they are, for instance, able to share the activities with the dog. The filter is an algorithm that sends dog owners a fitting profile of dog sitters for their inquiry and also dog sitters a fitting profile of a dog (dog owner). By setting the right main filter categories, for instance, walking for an hour or caring 24 h, the match will be created if the producer and the consumer confirm the inquiry.
The three key functions, pull, facilitate, and match, guarantee that the platform will be active and successful in the long term. Dog sitters and dog owners must be pulled to the platform. To solve the chicken- and egg dilemma, four of the eight elaborated strategies of Parker et al. (Reference Parker, van Alstyne and Choudary2016) were chosen. GassiAlarm implemented the big-bang adoption strategy (e.g.,Business Cards, Flyers, Facebook Advertisement), the micro-market strategy (targeting inhabitants in one city), the producer evangelism strategy (offer producers of goods and services the infrastructure to attract their consumers), and the marque strategy (incentives for active members which, for example, create content like newsfeed posts). The matching algorithm focused on different categories. Setting the main categories filtered the type of service, distance, location (ZIP Code), dates, price, and activity. Subfilter settings were experience, gender of the dog, and the size of the dog. Prices were displayed on a range according to the expectations of the dog owner and the dog sitter. Designing the monetization model and the launching strategy for digital platforms lead toward the network effect. The product benefit to a user depends on the total number of other users using it. For GassiAlarm, it meant that the cost of an additional user profile was growing linearly, but the value of all users was growing exponentially at the same time. The growing number of dog owners generated a positive network effect. The more dog owners participated in the platform, the more likely a dog sitter will find a suitable dog for dog sitting and thereby will earn money. The platform canvas summarizing and visualizing all aspects of the platform architecture is shown in Figure 10.

Fig. 10. Platform canvas for a dog care service (own presentation based on Choudary (Reference Choudary2015)).
In step (2) of the SPEC, design experiments for consumers and producers, different experiments in the customer segments, customer relationships, and sales channels were designed. Exemplary, the experiment regarding the sales channel is described in greater detail. Sales channels have to be proven for the special number of users in the micro-market. Depending on the performance, there was only the possibility of a pass or a failure for the channel. If the sales channel worked in one city, it probably would work in another city as well. Facebook and Google have been chosen as an example for a sales experiment.
Hypothesis: Facebook is a digital channel, which pulls potential customers to GassiAlarm.
MVP: Webpage GassiAlarm
Duration: 15 November–3 December 2017.
Currency: Email addresses (leads)
Threshold: Clicks on webpage: 40% of the people who visit the landing page will sign up as a potential user.
Hypothesis: Google is a digital channel, which pulls potential customers to GassiAlarm.
MVP: Webpage GassiAlarm
Duration: 18 November–17 December 2017
Currency: Email addresses (leads)
Threshold: Clicks on webpage: 40% of the people who visit the landing page will sign up as a potential user.
For step (3) build experiments including a MVP and implement a Measuring Metric, a first MVP (website) was designed as a frontend MVP. The two distinguished groups, the producers and consumers, were clearly separated by registration buttons. A dog owner chose between five different types of dog care, needed to indicate a maximum price for the dog care, and disclosed the dog's name, breed, age, size, and activity. If there was a direct inquiry for dog care, a calendar for check-in and check-out displayed it. Finally, a fixed commission fee of 6% for the platform was shown. Concerning the dog sitters, the registration asked several questions, for instance, the minimum price for hourly dog caring and the minimum price for a whole day. Registration did not automatically create a value unit but a lead. A value unit would be created when a dog owner sent a request for a special period, where s(he) needed someone to take care of his or her dog. A sale would be generated if there was a successful match. To measure all online activities, Google Analytics was implemented.
In step (4), run experiments and measure the outcome, different Facebook and Google campaigns were launched to determine how high the cost per lead and the cost per sales are. Traffic was generated on the GassiAlarm webpage by users who clicked on the Facebook or Google link and passed through to GassiAlarm. Exemplary, two Facebook campaigns had the goal to generate traffic, constrained by spending 35 Euros on each group. Only people who lived in Karlsruhe could see different Facebook campaigns. The demographics for dog owners were set to age 30 to 65+ and for dog sitters 17 to 30 years and student. However, using Facebook as a sales channel posting advertisements in Facebook groups, where only dog owners shared problems, was not possible. Of course, if a member were to be accepted, he/she could have posted a link in that group. Yet, the likelihood would increase that the member will be removed from the group as it might be against the rules to post something unrelated in the group. So, this guerrilla marketing method was not used. The Google advertisement was for dog owners and dog sitters. The demographic settings were set to people who lived in Karlsruhe at the age of 17 to 99 years and were looking for dog care. The slogan was “GassiAlarm – Die innovative Hundebetreuung”, which translates to GassiAlarm – the innovative dog care service. A limit of 45 Euros per month was set. While the campaign was active, 75% of the people used their smartphone to click on the advertisement, only eight persons used a tablet, and 17% of the users used their computer. The total cost spent on the advertisement was 31.59 Euros. The number of impressions was 3440. The traffic on GassiAlarm was tracked and analyzed by Google Analytics since the launch was on the first of November.
Step (5) analyze and learn from the results. It is interesting to note that the cost per click for dog owners laid at 0.35 Euro and for dog sitters at 0.53 Euro on Facebook. In this relation, the term conversion rate came into play, which indicates the ratio of people who visited the website and signed up as users. In total, 200 people clicked on the link and landed on the GassiAlarm webpage, of which only 15 people signed up. The conversion rate was 7.5%. Regarding owners, the conversion rate was 2.5%, and in the case of dog sitters, it was 5%. Resulting of the sales experiment on Facebook, the cost per lead amounted to 0.45 Euro (90 Euro/200 people). In total, 308 people who lived in Karlsruhe visited the landing page. Keeping that number in mind, it led to a ratio of 64.9% (200/308*100%) of people who have been directed to GassiAlarm by Facebook. The threshold set at 40% of people visiting the landing page would sign up as potential leads were reached. Facebook passed as a sales channel. According to Google, a total of 72 people clicked on the link and landed on GassiAlarm, of which only 8 signed up. The conversion rate was 11.1%. Concerning dog owners, the conversion rate was 8.3%, and in the case of dog sitters, it was 2.8%. Derived from the sales experiments Google, the cost per click amounted to 0.44 Euro (31.59 Euro/72 people), and the cost per lead amounted to 3.95 Euro (31.59 Euro/8 people). Recalling the results, 308 people, who live in Karlsruhe, visited the landing page. Keeping that number in mind, led to a ratio of 23.4% (72/308*100%) of people directed to GassiAlarm by Google. The threshold was set at 40% of people, who visited the landing page, would sign up as potential leads were not reached. Thus, Google failed as a sales channel.
The total number of signed-up participants was 157 dog owners and dog sitters. Due to incomplete profiles during registration, the final number of participants was 111, of which 57 were dog sitters and 54 dog owners. The experiments have been performed between 1 November 2017 and 31 January 2018. An independent survey confirmed that only people who are interested in dog care visited the website. Learnings from the sales experiments showed that 98.2% of dog owners require someone for dog walking approximately for 1 h; only 1.8% expressed no demand for that service. Only 3.5% of dog owners need someone to take care of the dog for a full day while caring for more than 1 day was indicated by 8.7%. 91.2% prefer dog care at the sitter's home for 1day, whereas 93.0% prefer dog care at the dog sitter's home for more than 1 day. In contrast, 100% of dog sitters are searching for the demand for dog walking. 68.5% of all dog sitters would like to do dog care at the dog owner's house for 1 day, while 50% would do dog caring at the sitter's house for more than 1 day. 51.8% are willing to do dog care at their own home. Furthermore, 38.6% indicated to prefer caring for more than 1 day (Table 1).
Table 1. Learnings from sales experiments

As a result, the hypothesis dog owners pay, on average, 27 Euro for 1 day of dog care and 12 Euro for an hour of dog care was validated. The highest price dog sitters set for 1 h of dog care were 20 and 150 Euro for 24 h. The lowest price was zero Euro per day. Seven percent of the dog sitters would take care of the dog without asking for payment because they enjoy the leisure activity with the fun factor dog. Dog owners were willing to pay 29.35 Euro per day for dog care, which was more than the average of 27 Euro. In the case of 24 h of dog care, the students' average price lay by 38.07 Euro. Dog sitters demand that supply was invalidated. For 1 h of dog care, the price depended highly on the activity itself. If someone did an extra with the dog, such as going for a swim, dog owners would be willing to pay more and dog sitters would ask for more money. The hypothesis dog owners pay on average 12 Euro for an hour of dog care was invalidated since dog owners were willing to pay a price of 11.33 Euro for 1 h of dog care. In the case of dog sitters, however, a match for 1 h could be made because the average price lay by 11.21 Euro. Table 2 shows an overview of the average prices.
Table 2. Learnings from pricing experiments

One learning from the pricing strategy was obtained at the beginning when the commission fee was set to a fixed 6%. For dog sitters, the service of GassiAlarm was free. In total, 43.2% of dog owners and dog sitters participated during the time of the fixed commission fee and accepted it. Due to the iterative approach of the SPEC, the pricing strategy of the platform business model was redesigned, and a new hypothesis was formulated: Dog owners and dog sitters will give a higher commission fee if they can decide on their own. In this experiment, the commission fee was set variable from 0% to 10% of the revenue. Now, dog owners and dog sitters determined a range. 17.1% of the dog owners set 0% as a commission fee, while 9.7% set the maximum of 10% as a commission fee. The average commission fee of dog owners was 4.0%. 19.0% of the dog sitters set 0% as a commission fee, while 9.5% set the maximum of 10% as a commission fee. The average commission fee was 5.1%. Ultimately, the commission fee can be added and totaled to 9.1%.
Discussion
This research was set out to create a validation process for startups and corporates with a digital platform business model by using business experiments. Unlike existing validation processes that are solely designed for pipeline business models, the new validation process needed to incorporate the logic of digital platforms (Parker et al., Reference Parker, van Alstyne and Choudary2016, p. 5). Furthermore, this research aimed to create a validation process that includes the attribute of saving time and money. This aspect was included since it is a decisive factor of whether or not to pursue a digital platform business model for startups, or in some instances, also for corporates (Blank and Dorf, Reference Blank and Dorf2012). The solution to this research was gained by extensively analyzing current validation processes, namely the Four-Step Iterative Cycle, the BML feedback loop, and the Customer Development Process. By following steps one to four of the Design Science Research methodology, The SPEC, a validation process for digital platform business models, was builtby following steps one to four of the Design Science Research methodology.
The SPEC takes the startup through the steps (1) design the digital platform business model, (2) design experiments for consumers and producers, (3) build experiments including a MVP and implement a Measuring Metric, (4) run the experiments and measure the outcome, as well as (5) analyze and learn from the results. The SPEC was created by regarding the differences and similarities between a pipeline and digital business model. In this regard, those elements were extracted that create a product or service that is repeatedly used or purchased by the customers. Therefore, the SPEC incorporates, for instance, the philosophy of involving the two customer groups early on by obtaining customer feedback repeatedly and using those insights to adjust the business models accordingly. Furthermore, the SPEC also points out how appropriate the hypothesis or assumptions are that were formulated for the digital platform business model and directs the user toward three possible outcomes after step 5 of the process is reached. Thus, the SPEC is considered an iterative validation cycle for early market validation.
The SPEC keeps all processes as lean as possible, including the experiments to validate a digital platform business model. In the case of GassiAlarm, experiments in the area of sales channels, customer segments, customer relations, and pricing strategies prevented the waste of time and money. Also, the digital platform business model was successfully validated. The application of SPEC within GassiAlarm showed that the business is not repeatable, which was confirmed by 85.5% of dog owners and dog sitters. It was revealed that GassiAlarm was not able to bind both sides of the market to the platform and generate repeatable transactions between them on the platform (Rochet and Tirole, Reference Rochet and Tirole2006). What is more, the SPEC helped the startup to structure and target their experiments correctly, which involved validating the digital platform business model with the customer. The application of SPEC limited the waste of time and money and made it possible that GassiAlarm stays within the set budget of 1000 Euros. Due to the low budget available, network effects could not be proven, which are essential as a market-/growth-building tool for digital platforms (Shapiro and Varian, Reference Shapiro and Varian1998; Farrell and Klemperer, Reference Farrell, Klemperer, Armstrong and Porter2007; Parker et al., Reference Parker, van Alstyne and Choudary2016). It requires a higher budget to develop a functioning backend with user profiles and integrate AI tools properly. Moreover, the iterative approach of SPEC revealed that users did not welcome the initial pricing strategy of GassiAlarm. Only after redesigning the pricing strategy, GassiAlarm was able to grasp the willingness of producers and consumers to pay for their service. As such, focusing on the average price was the wrong approach to generate matches. Setting the price too high or too low can destroy the results and also prevents gaining insights into information about matches. The matching intention of the digital platform is limited and proved to be a very sensitive matter. After the dog sitter is matched with the value unit, the value unit is not available anymore for other dog sitters. Creating the perfect match factors, such as the type of the dog care service or the time spent with dog caring, is of utmost importance. All in all, GassiAlarm received helpful revelations by applying SPEC. It could make improvements in their predefined rules and learned when to exit and redesign the digital platform business model.
The necessity of AI sheds an ambiguous light on the decision making of startups. A startup is constrained in terms of money and time, and thus, keeps all processes as lean as possible. On the one hand, a venture idea should get to the market fast, and on the other hand, the products should be composed as an MVP to avoid creating something that the market would not pay for. The integration of AI is essential as it supports the four core functions of attracting, matching, ensuring the interaction of producers and consumers by optimizing the infrastructure, and lastly, accomplishing the participants' return to the platform. Various authors have pointed out the integral role of AI and the relating intelligent decision-making (Buxmann and Schmidt, Reference Buxmann and Schmidt2019; Goli et al., Reference Goli, Zare, Tavakkoli-Moghaddam and Sadeghieh2019; Sánchez et al., Reference Sánchez, Sánchez-Medina and Pellejero2020). Integrating AI can enhance the network effect through superior matching algorithms or increases effectiveness and accuracy in the match itself by applying filter algorithms (Buxmann and Schmidt, Reference Buxmann and Schmidt2019). Furthermore, AI can forecast the participants' needs and reveals what adjustments should be made on the platform to meet those needs (Buxmann and Schmidt, Reference Buxmann and Schmidt2019; Goli et al., Reference Goli, Zare, Tavakkoli-Moghaddam and Sadeghieh2019). In more advanced stages, the startups could integrate AI-based customer analyses and make use of recommendation engines to promote up-and-cross-selling by sending the right notification at the right time (Buxmann and Schmidt, Reference Buxmann and Schmidt2019). Especially making use of cross-selling to both existing and potential customers increases customer equity. Customer equity is the aggregated expected lifetime value of existing and future customers and reflects the firm value held in customer assets, which eventually translates into firm value (Hogan et al., Reference Hogan, Lehmann, Merino, Srivastava, Thomas and Verhoef2002). However, all this comes at a price that startups do not have excessively, especially in the beginning. Startups must know which AI algorithms to integrate in their MVP to turn the business idea into a successful business. In the early stages of the business, the startup should focus on AI that supports attracting participants to the digital platform and matching the right users. In later stages, where the focus lies on scaling the business, AI is the only way to achieve sustainable success and calls for sophisticated filter algorithms and analyses. Therefore, it can be concluded in line with Hahn et al. (Reference Hahn, Traunecker, Niever and Basedow2020) that the investment and integration of AI in the digital platform business model creates a significant value. The SPEC is aware of the importance of AI and puts these elements at the forefront in step (1) of the process, where the emphasis is put on the correct realization of the three key components and the three key functions in digital platform business models.
Taking a closer look at SPEC, step (1) design the digital platform business model raises the question of where the verified business models are coming from, which are the starting point of SPEC. It is a prerequisite to generate and verify the business model ideas to start with SPEC. Various platform design tools help companies and startups to develop a digital platform business model fast and efficiently. In this context, the Google Sprint (Knapp et al., Reference Knapp, Zeratsky and Kowitz2016) is a method that quickly generates insights into how to solve identified problems and test new ideas in just 5 days. Although the validation of Google Sprint is yet not robust for digital platform business models, it could be possible to accelerate entering SPEC by applying Google Sprint verification on the digital platform business idea. In line with this indication, a further research study could revolve around the question of to what extent the Google Sprint method would accelerate the process to reach step (1) of the SPEC and how it needs to change to be adapted to serve the digital platform logic. Furthermore, in this research, the first step of SPEC was done by employing the Platform Canvas (Choudary, Reference Choudary2015). The Platform Canvas is simple and easy in its application. However, it is important to investigate if and how step (1) of SPEC can be expanded by other platform design tools such as the Platform Value Canvas, the Platform Business Model Canvas by Walter (Reference Walter2021), or the Platform Design Canvas by Cicero (Reference Cicero2021), or other tools and methods. The methods enable a more thorough assessment of the phases and require more time to apply correctly.
The steps (2) to (5) of SPEC guide a startup that is operating a digital platform business model on how to conduct business experiments. By following it, the startup gained insights into customer segments, customer relationships, and sales channels regarding producers and consumers. Business experiments are a disciplined approach demonstrating a causal relationship by measuring the effect an action has on a situation (Martin and Hanington, Reference Martin and Hanington2012). The challenge of business experiments lies in the steps (2) design, (3) build, (4) run and measure, and (5) analyze and learn as they need to ensure efficacy and the revelation of the underlying causalities. It might be very challenging for startups as most of their business and operations are not determined yet and are mostly based on hypotheses that need to be confirmed first. Startup also needs to operate quickly and are constrained by budgets which might limit the degree of reliability and validity of results. Therefore, it is important to create well-designed business experiments since they can reduce the need for time and money decisively.
The current relevance of digital platforms and their power to penetrate B2C markets, such as Airbnb and Uber, and bring business ideas into another dimension. In the age of digital transformation, digital platforms are not only created in B2C markets. The “German Mittelstand” is taking steps toward developing new digital B2B platforms. For instance, LaserHub (founded in 2017) is transforming the sheet metal industry. On the one hand, by providing the capacity of sheet metal machines and, on the other hand, by enabling customers to meet their demand for sheet metal parts. The question is, to what extent must SPEC (B2C) be transformed, to be applied to platforms that operate the B2B market. The growth of digital platforms in the B2C and B2B markets continues to generate room for further research. Therefore, research in early market validation is indispensable.
At this point, the authors would like to address the validity of the SPEC itself. Recalling the definition of validation, it reveals if the right product was built and shows if “the product does what it is supposed to do in the intended operational environment” (Engel, Reference Engel2010, p. 17). This research has shown preliminarily that SPEC can be used as a validation process for startups that follow a service marketplace digital platform business model. The empirical application of SPEC within a real startup created first insights and hints toward a positive external validation. However, further research is needed that scientifically validates the SPEC approach by applying it in other startups and corporate projects, and other types of digital platform business models to increase external validity and robustness. Furthermore, it should also be investigated to what extent SPEC holds when applied in a startup that builds a product or service for the B2B market. As platform-based ideas often bring a radical change in their respective markets, the authors are working on a more general framework of how to create, verify, validate (SPEC), and scale these business model innovations, also known as Radical Innovation Engineering (RIE). Additionally, the exploitation and scaling of validated business models should be the subject of further research.
Conclusion
The SPEC can be used as an early market validation process for digital platform business models to design, analyze, and validate the business models. The focus of the SPEC is on validating the building blocks channels, customer segments, value proposition, customer relationships, and the revenue streams of a digital platform business model. The new validation approach of SPEC, which follows the process of Lean Startup combined with the Four-Step Iterative Cycle and the Customer Development Process, prevents waste of money and time and creates a basis of whether or not to pursue a digital platform business model.
Patrick Brecht, MSc, works as a research associate at the Karlsruhe University of Applied Sciences after completing his study in industrial engineering with a focus on sales. In addition to his passion for sales, he has a growing fascination for science and entrepreneurship. In his role as a project manager at the xLab, Experimental Lab for Innovation and Entrepreneurship, he supports startups in the area of business model innovation. His focus lies in validating startup potential within the field of smart business experiments and platform business models.
Manuel Niever, MSc, successfully completed his studies of industrial engineering at the Karlsruhe University of Applied Sciences in 2016. Since then, he has been a research associate with a focus on innovation processes and methods as well as business model development. For his doctoral research at IPEK – Institute of Product Development at the KIT (Karlsruhe Institute of Technology), he works in the research group innovation management. Within his work, he develops a concept of innovation coaching for the process-oriented support of people in agile innovation projects.
Roman Kerres, MSc, successfully completed his studies at the University of Applied Sciences Karlsruhe in industrial engineering in 2016. In his work as a research associate, he focuses on new business models for small- and medium-sized companies to meet their challenges in the context of digitalization and exploration. This work forms the basis for his dissertation, in which he develops an innovation model for SMEs concerning radical innovations to rethink existing business models. His valuable experience in the development of digital business models in medium-sized companies led to a new lecture format for the platform economy.
Anja Ströbele, BSc, is a research associate of the xLab at the Karlsruhe University of Applied Sciences. Her main research interests are in the field of innovation, platform business models, and experimentation. During her studies, she developed a passion for research and an interest in innovation and entrepreneurship from analyzing the factors that turn a user innovator into a social entrepreneur with the help of the theory of planned behavior. Her current master's degree focus is on marketing finance that not only provides an understanding of the interface of these two disciplines but also gave insights into design process models to create financial innovations.
Prof. Dr. Carsten H. Hahn works as a director of research and innovation at SAP and additionally holds a professorship for Innovation and Entrepreneurship at the Karlsruhe University of Applied Sciences. After studying business informatics at the University of Mannheim and doing his doctorate in Marketing at University of Mainz, he began his career as an assistant to the executive board of SAP. In his academic work, he founded the xLab, which deals with entrepreneurship and innovation concepts in research, teaching, and practical application.