High-frequency heart rate variability, defined by cyclic oscillations in heart rate across successive respiratory cycles (see, e.g., Malik & Camm, Reference Malik and Camm1995; Zisner & Beauchaine, Reference Zisner, Beauchaine and Cicchetti2016), is of interest to developmental psychopathologists given well replicated associations with numerous aspects of psychological well-being and dysfunction (see, e.g., Beauchaine, Reference Beauchaine2001; Beauchaine & Thayer, Reference Beauchaine and Thayer2015; Berntson et al., Reference Berntson, Bigger, Eckberg, Grossman, Kaufmann, Malik and van der Molen1997; Obradovic & Boyce, Reference Obradović and Boyce2012). As outlined below, high-frequency heart rate variability is quantified by measuring respiratory sinus arrhythmia (RSA), the component of HRV associated with breathing.Footnote 1 Over the past two decades, consistent findings have emerged demonstrating positive associations between RSA and numerous psychological adjustment outcomes among children, adolescents, and adults. These outcomes include, effective behavior regulation during social challenges (e.g., Hastings, Nuselovici, et al., Reference Hastings, Nuselovici, Utendale, Coutya, McShane and Sullivan2008; Hastings, Sullivan, et al., Reference Hastings, Sullivan, McShane, Coplan, Utendale and Vyncke2008), empathic responding to others who are in distress (Fabes, Eisenberg, & Eisenbud, Reference Fabes, Eisenberg and Eisenbud1993; Liew et al., Reference Liew, Eisenberg, Spinrad, Eggum, Haugen, Kupfer and Baham2011), positive social interactions with partners (Diamond, Fagundes, & Cribbet, Reference Diamond, Fagundes and Cribbet2012), proficient executive function (Thayer, Hansen, Saus-Rose, & Johnsen, Reference Thayer, Hansen, Saus-Rose and Johnsen2009), social competence (Eisenberg et al., Reference Eisenberg, Fabes, Murphy, Maszk, Smith and Karbon2008), sustained attention capabilities (Suess, Porges, & Plude, Reference Suess, Porges and Plude1994), temperamental complaisance (Huffman et al., Reference Huffman, Bryan, del Carmen, Pedersen, Doussard-Roosevelt and Porges1998), and protection against psychopathology in adverse rearing conditions (e.g., El-Sheikh, Harger, & Whitson, Reference El-Sheikh, Harger and Whitson2001; Shannon, Beauchaine, Brenner, Neuhaus, & Gatzke-Kopp, Reference Shannon, Beauchaine, Brenner, Neuhaus and GatzkeKopp2007). Thus, RSA is associated positively with a broad array of behavioral competencies.
RSA and Psychopathology
In contrast to positive associations between RSA and both psychological adjustment and resilience, abnormally low resting RSA, and to a lesser extent excessive RSA reactivity (i.e., withdrawal) to behavioral challenges, especially emotion evocation tasks, are observed in many psychiatric disorders that are characterized by emotion dysregulation, and correlate with several psychopathological traits (see Beauchaine, Reference Beauchaine2015a, Reference Beauchaine2015b; Beauchaine & Thayer, Reference Beauchaine and Thayer2015; Chambers & Allen, Reference Chambers and Allen2007). These include anxiety disorders (e.g., Hastings, Nuselovici, et al., Reference Hastings, Nuselovici, Utendale, Coutya, McShane and Sullivan2008; Hastings, Sullivan, et al., Reference Hastings, Sullivan, McShane, Coplan, Utendale and Vyncke2008; Kemp et al., Reference Kemp, Brunoni, Santos, Nunes, Dantas, de Figueiredo and Lotufo2014; Thayer, Friedman, & Borkovec, Reference Thayer, Friedman and Borkovec1996), attention problems (see Rash & Aguirre-Camacho, Reference Rash and Aguirre-Camacho2012), autism (Neuhaus, Bernier, & Beauchaine, Reference Neuhaus, Bernier and Beauchaine2014; Patriquin, Scarpa, Friedman, & Porges, Reference Patriquin, Scarpa, Friedman and Porges2013), bipolar disorder (Moon, Lee, Kim, & Hwang, Reference Moon, Lee, Kim and Hwang2013; Quintana et al., Reference Quintana, Westlye, Kaufmann, Rustan, Brandt, Haatveit and Andreassen2015), borderline personality disorder (Austin, Riniolo, & Porges, Reference Austin, Riniolo and Porges2007), callousness (de Wied, van Boxtel, Matthys, & Meeus, Reference de Wied, van Boxtel, Matthys and Meeus2012), conduct disorder (e.g., Beauchaine, Gatzke-Kopp, & Mead, Reference Beauchaine, Gatzke-Kopp and Mead2007; Beauchaine, Katkin, Strassberg, & Snarr, Reference Beauchaine, Katkin, Strassberg and Snarr2001), depression (e.g., Koenig, Kemp, Beauchaine, Thayer, & Kaess, Reference Koenig, Kemp, Beauchaine, Thayer and Kaess2016; Rottenberg, Reference Rottenberg2007; Rottenberg, Salomon, Gross, & Gotlib, Reference Rottenberg, Salomon, Gross and Gotlib2005; Rottenberg, Wilhelm, Gross, & Gotlib, Reference Rottenberg, Wilhelm, Gross and Gotlib2002),Footnote 2 nonsuicidal self-injury (e.g., Crowell et al., Reference Crowell, Beauchaine, McCauley, Smith, Stevens and Sylvers2005), panic disorder (e.g., Asmundson & Stein, Reference Asmundson and Stein1994), phobias (e.g., Ahs, Sollers, Furmark, Fredrikson, & Thayer, Reference Ahs, Sollers., Furmark, Fredrikson and Thayer2009), psychopathy (Hansen, Johnsen, Thornton, Waage, & Thayer, Reference Hansen, Johnsen, Thornton, Waage and Thayer2007), schizophrenia (see Clamor, Lincoln, Thayer, & Koenig, Reference Clamor, Lincoln, Thayer and Koenig2015; Quintana et al., Reference Quintana, Westlye, Kaufmann, Rustan, Brandt, Haatveit and Andreassen2015), and trait hostility (Sloan et al., Reference Sloan, Shapiro, Bigger, Bagiella, Steinman and Gorman1994), among others. Moreover, heterotypic comorbidity is associated with greater reductions in RSA during emotion evocation than either internalizing or externalizing symptoms alone (Calkins, Graziano, & Keane, Reference Calkins, Graziano and Keane2007; Pang & Beauchaine, Reference Pang and Beauchaine2013).
This lengthy, transdiagnostic list indicates that low resting RSA and excessive RSA reactivity to emotion evocation mark one or more fundamental self-regulatory functions that are disrupted across various manifestations of psychopathology (see Beauchaine, Reference Beauchaine2001, Reference Beauchaine2015a, Reference Beauchaine2015b; Beauchaine & Thayer, Reference Beauchaine and Thayer2015). Reduced tonic RSA is nearly always found among adolescent and adult samples with clinical levels of psychopathology, and excessive RSA reactivity is often observed among clinical samples during emotion evocation tasks.Footnote 3 Several important questions emerge from these findings. First, what specific trait does RSA mark that captures vulnerability to such a broad range of psychopathological conditions, and second, what are the neural substrates of this trait? Addressing these questions is important given that improved understanding of etiology often leads to more effective interventions (see, e.g., Beauchaine & Cicchetti, Reference Beauchaine and Cicchetti2016; Beauchaine, Neuhaus, Brenner, & Gatzke-Kopp, Reference Beauchaine, Neuhaus, Brenner and Gatzke-Kopp2008). In addition, how does this trait develop, and do its neural substrates exhibit plasticity, which may also have implications for treatment? These questions have been at the forefront of our research for almost two decades, but especially in recent years (see, e.g., Beauchaine, Reference Beauchaine2001, Reference Beauchaine2015a, Reference Beauchaine2015b; Beauchaine, Gartner, & Hagen, Reference Beauchaine, Gartner and Hagen2000; Beauchaine & McNulty, Reference Beauchaine and McNulty2013; Beauchaine & Thayer, Reference Beauchaine and Thayer2015; Beauchaine et al., Reference Beauchaine, Katkin, Strassberg and Snarr2001, Reference Beauchaine, Gatzke-Kopp and Mead2007, Reference Beauchaine, Gatzke-Kopp, Neuhaus, Chipman, Reid and Webster-Stratton2013; Conradt et al., Reference Conradt, Beauchaine, Lester, Lagasse, Shankaran, Bada and Hammond2016; Crowell et al., Reference Crowell, Beauchaine, McCauley, Smith, Stevens and Sylvers2005, Reference Crowell, Baucom, Yaptangco, Bride, Hsiao, McCauley and Beauchaine2014; Neuhaus, Bernier, & Beauchaine, Reference Neuhaus, Bernier and Beauchaine2016).
RSA, Emotion Dysregulation, and Prefrontal Cortex Function
It now well recognized that, like deficiencies in RSA, emotion dysregulation is observed across a wide range of psychiatric conditions (e.g., Beauchaine, Reference Beauchaine2001, Reference Beauchaine2015b; Beauchaine & Thayer, Reference Beauchaine and Thayer2015; Cole, Hall, & Hajal, Reference Cole, Hall, Hajal, Beauchaine and Hinshawin press). An ever-expanding literature indicates that difficulties with emotion regulation (ER) predispose to, and are observed in, most forms of psychopathology (e.g., Beauchaine, Reference Beauchaine2015a; Cole, Dennis, Hall, & Martin, Reference Cole, Dennis, Hall, Martin, Vandekerckhove, von Scheve, Ismer, Jung and Kronast2008; Kaufman et al., Reference Kaufman, Puzia, Mead, Crowell, McEachern and Beauchaine2016). Emotion regulation is often defined as the set of processes through which individuals shape their emotional experience and expression in support of adaptive behavior (see, e.g., Aldao, Reference Aldao2013; Thompson, Reference Thompson and Thompson1990). Shaping of emotions is effected through various interrelated mechanisms, some automated, some effortful, and some social, including attention allocation and other forms of cognitive control, dyadic interactions in core social relationships, operant reinforcement of physiological responding, and self-directed behaviors such as avoidance, reappraisal, and problem solving (e.g., Aldao, Nolen-Hoeksema, & Schweizer, Reference Aldao, Nolen-Hoeksema and Schweizer2010; Beauchaine, & Zalewski, Reference Beauchaine, Zalewski, Dishion and Snyder2016; Butler & Randall, Reference Butler and Randall2013; Crowell et al., Reference Crowell, Butner, Wiltshire, Munion, Yaptangco and Beauchaine2016).
In contrast to ER, emotion dysregulation is a pattern of emotional experience and/or expression that interferes with/obstructs effective goal-directed behavior (e.g., Beauchaine, Reference Beauchaine2015a; Beauchaine & Gatzke-Kopp, Reference Beauchaine and Gatzke-Kopp2012). Across nearly all forms of psychopathology, at least one negative emotion (e.g., anger, anxiety, panic, rage, sadness) is experienced either so intensely or so persistently that it interferes with adaptive function (see Beauchaine, Reference Beauchaine2015a). As we have reviewed elsewhere, and as outlined briefly above, RSA is a reliable autonomic nervous system marker of emotion dysregulation (see, e.g., Austin et al., Reference Austin, Riniolo and Porges2007; Beauchaine, Reference Beauchaine2001, Reference Beauchaine2015a, Reference Beauchaine2015b; Porges, Reference Porges1995, Reference Porges2007).
Most first-generation theories of emotion regulation and dysregulation considered the parasympathetic nervous system (PNS) to be a self-regulatory system in its own right (see, e.g., Fox, Reference Fox1994). According to these theories, both RSA and self-regulatory capacity emanate from the PNS, with central nervous system source nuclei in the brainstem (see Porges, Reference Porges1995, Reference Porges1996). Although these brainstem structures are clearly implicated in cardiorespiratory coupling, as reflected in RSA (see below; e.g., Garcia, Koschnitzky, Dashevskiy, & Ramirez, Reference Garcia, Koschnitzky, Dashevskiy and Ramirez2013), more recent models propose that associations between ER and RSA are effected through top-down, prefrontal control over subcortical neural networks (see Beauchaine, Reference Beauchaine2015a; Beauchaine & Thayer, Reference Beauchaine and Thayer2015; Thayer et al., Reference Thayer, Hansen, Saus-Rose and Johnsen2009). According to these newer models, brainstem nuclei specified in earlier theories of ER serve as final common pathways from prefrontal regions to the PNS, but are not responsible for ER itself.
Although full articulation of the cortical substrates of ER and RSA is beyond the scope of this article, several findings, many of which derive from extensive and accumulating neuroimaging research that was unavailable when first-generation models were formulated, provide strong support for prefrontal influences over both ER and RSA. For example, an increasing literature base indicates that neural regulation of fear and anxiety occurs through lateral prefrontal inhibition of amygdalar activity and reactivity, and that neural regulation of impulsivity occurs through orbitofrontal and dorsolateral prefrontal inhibition of striatal activity and reactivity (see, e.g., Davidson, Reference Davidson2002; Heatherton, Reference Heatherton2011; Heatherton & Wagner, Reference Heatherton and Wagner2011; Macdonald, Goines, Novacek, & Walker, Reference Macdonald, Goines, Novacek and Walker2016). Furthermore, RSA has been linked to prefontal cortex function through several research paradigms and methods, including cerebral blood flow as assessed using both positron emission tomography and fMRI (see Thayer, Ahs, Fredrikson, Sollers, & Wager, Reference Thayer, Åhs, Fredrikson, Sollers and Wager2012), lesion studies (Buchanan et al., Reference Buchanan, Driscoll, Mowrer, Sollers, Thayer, Kirschbaum and Tranel2010), pharmacologic blockade studies (Ahern et al., Reference Ahern, Sollers, Lane, Labiner, Herring, Weinand and Thayer2001), pulsed arterial spin labeling (Allen, Jennings, Gianaros, Thayer, & Manuck, Reference Allen, Jennings, Gianaros, Thayer and Manuck2015), and retinal vessel analysis (Schuster, Jarczok, Fischer, Thayer, & Vossmerbaeumer, Reference Schuster, Jarczok, Fischer, Thayer and Vossmerbaeumer2014). Neuroimaging research also indicates that successful treatment of disorders that are characterized by emotion dysregulation, such as depression, normalizes previously disrupted associations between prefrontal cortex function and RSA (e.g., Smith, Allen, Thayer, Fort, & Lane, Reference Smith, Allen, Thayer, Fort and Lane2014). Finally, inhibitory efferent pathways from the medial prefrontal cortex to the PNS have been described in the literature for some time (e.g., Barbas, Saha, Rempel-Clower, & Ghashghaei, Reference Barbas, Saha, Rempel-Clower and Ghashghaei2003; Ter Horst & Postema, Reference Ter Horst and Postema1997; Thayer et al., Reference Thayer, Hansen, Saus-Rose and Johnsen2009; Wong, Masse, Kimmerly, Menon, & Shoemaker, Reference Wong, Masse, Kimmerly, Menon and Shoemaker2007). Thus, considerable evidence now suggests that both RSA and emotion regulation are influenced strongly by top-down, prefrontal control over subcortical neural networks, and that RSA serves as a peripheral index of emotion regulation under appropriate stimulus conditions.
Quantifying RSA
Heart rate variability is distributed across a wide range of frequencies, which are often divided into very low, low, and high frequency bands in efforts to isolate both neural and nonneural sources of cardiac activity and reactivity (see, e.g., Beauchaine, Reference Beauchaine2001; Berntson et al., Reference Berntson, Bigger, Eckberg, Grossman, Kaufmann, Malik and van der Molen1997; Task Force of the European Society for Cardiology and the North American Society of Pacing and Electrophysiology, 1996). Diurnal influences, baroreceptor activation, tonic and phasic influences of the SNS and PNS, biomechanical factors, other nonneural effects, and pathophysiological processes all affect HRV (see Beauchaine & Zisner, Reference Zisner, Beauchaine and Cicchetti2016; Berntson et al., Reference Berntson, Bigger, Eckberg, Grossman, Kaufmann, Malik and van der Molen1997; Porges, Reference Porges1995; Ritz, Reference Ritz2009). By definition, RSA is the component of HRV associated with respiration (see, e.g., Berntson et al., Reference Berntson, Bigger, Eckberg, Grossman, Kaufmann, Malik and van der Molen1997; Zisner & Beauchaine, Reference Zisner, Beauchaine and Cicchetti2016). Inhibitory PNS efference from the brainstem decelerates HR during exhalation, whereas PNS withdrawal accelerates HR during inhalation. Given appropriate control for respiration (statistical or paced breathing), RSA is a noninvasive measure of parasympathetic efference to the heart, as determined by pharmacologic blockade (see Allen, Chambers, & Towers, Reference Allen, Chambers and Towers2007; Grossman & Taylor, Reference Grossman and Taylor2007; Grossman, van Beek, & Wientjes, Reference Grossman, van Beek and Wientjes1990; Hayano et al., Reference Hayano, Sakakibara, Yamada, Yamada, Mukai, Fujinami and Takata1991; Ritz, Reference Ritz2009).Footnote 4 RSA can be measured using either time domain or frequency domain methods (see Allen et al., Reference Allen, Chambers and Towers2007; Katona & Jih, Reference Katona and Jih1975; McCabe, Yongue, Ackles, & Porges, Reference McCabe, Youngue, Ackles and Porges1985; Santucci et al., Reference Santucci, Silk, Shaw, Gentzler, Fox and Kovacs2008; Thayer, Hansen, & Johnsen, Reference Thayer, Hansen, Johnsen and Steptoe2010).
Time versus frequency domain assessment
The simplest approach to computing HRV is to use time domain measures. These include the standard deviation of R-R intervals, and the mean successive difference in R-R intervals, among other metrics. Time domain measures calculate HRV directly from the interbeat interval time series. These measures are rarely used in the child and adolescent literatures. For this reason, and because they are not on the same metric as frequency domain measures (described below) and therefore cannot be compared directly, we do not discuss them further.
Other commonly used measures of HRV are computed in the frequency domain. Spectral analysis, including fast Fourier transform (FFT) and autoregressive (AR) techniques, is the most commonly used method (Poliakova et al., Reference Poliakova, Dionne, Dubreuil, Ditto, Pihl, Pérusse and Boivin2014). Frequency domain methods quantify the amount, or “power,” of HRV within specified frequency bands, which are sometimes referred to as “windows” (see, e.g., Grossman, Reference Grossman1992; Parati, Saul, Di Rienzo, & Mancia, Reference Parati, Saul, Di Rienzo and Mancia1995). Fast-Fourier analysis converts time-related phenomena into a spectral density function across all observed frequencies. As depicted in Figure 1, FFT can isolate and quantify spectral power within specific frequency bands, which may have important implications for identifying peripheral and central nervous system sources of health and disease (see above). By convention, adult spectral density functions are typically divided into low-frequency HRV (<0.04 Hz), mid-frequency HRV (0.04–0.15 Hz), and high-frequency HRV (>0.15 Hz). At normal adult breathing rates, high-frequency HRV yields an estimate of RSA.
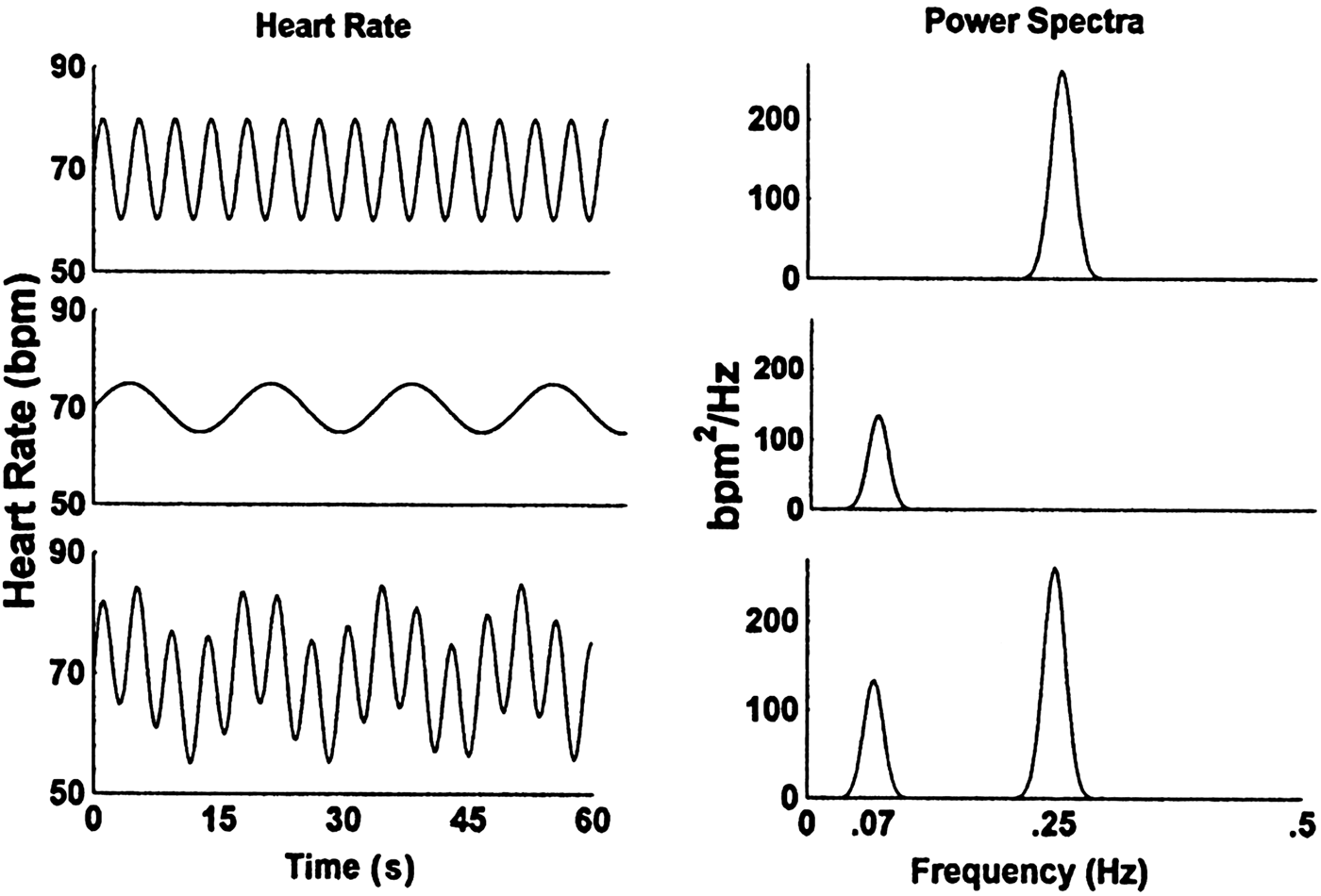
Figure 1. (Left) Fictitious heart rate signal and (right) associated power spectra. The top two panels represent pure high-frequency variability (0.25 Hz), the middle two panels represent low-frequency variability (0.07 Hz), and the bottom two panels represent the combined (i.e., high- and low-frequency) signal. Actual heart rate signals include spectral power at additional frequencies. Adapted from “Vagal Tone, Development, and Gray's Motivational Theory: Toward an Integrated Model of Autonomic Nervous System Functioning in Psychopathology,” by T. Beauchaine, Reference Beauchaine2001, Development and Psychopathology, 13, 183–214. Copyright 2001 by Cambridge University Press. Adapted with permission. Adapted also from “The Utility of Spectral Analytic Techniques in the Study of Autonomic Regulation of Beat-to-Beat Heart Rate Variability,” by E. Mezzacappa, D. Kindlon, F. Earls, and J. P. Saul, Reference Mezzacappa, Kindlon, Earls and Saul1994, International Journal of Methods in Psychiatric Research, 4, 29–44. Copyright 1994 by John Wiley & Sons, Inc. Adapted with permission.
Provided that the RSA band is defined to match respiratory rates of participants, high-frequency spectral power quantifies vagal efference to the heart (see Grossman, Reference Grossman1992; Grossman, Karemaker, & Wieling, Reference Grossman, Karemaker and Wieling1991; Ritz, Reference Ritz2009). However, breathing rates vary substantially both within and between individuals and samples. This is especially problematic when ages of participants are dispersed widely, since children breath at much higher frequencies than adults (see below). To avoid misspecifying respiratory frequencies, some researchers who work with adults have turned to AR spectral analysis to bypass population-based selection of appropriate respiratory windows (see Thayer, Reference Thayer2002; Thayer et al., Reference Thayer, Hansen, Johnsen and Steptoe2010; Thayer, Peasley, & Muth, Reference Thayer, Peasley and Muth1996). AR methods apply true components analysis to the interbeat interval time series to empirically derive the appropriate respiratory frequency for each participant (see Kay & Marple, Reference Kay and Marple1981). Autoregressive spectral analysis should therefore be more accurate than spectral analysis conducted without correction for respiration.
Developmental Shifts in RSA and Links to Psychopathology
To date, AR spectral analysis has not been used in child or adolescent samples. Furthermore, a large proportion of studies in the child development and developmental psychopathology literatures have used RSA frequency bands that do not match breathing rates of participants. Although our intent is not to criticize individual researchers or labs (our own work suffers from the same limitation), a brief literature search yields dozens of examples of respiratory frequencies being misspecified in research with both normal and psychopathological samples of children and adolescents. These misspecifications include (a) use of adolescent and adult frequency bands to assess RSA among infant and child samples (the most common misspecification), (b) use of child frequency bands to evaluate RSA among adolescents and adults, (c) use of a single frequency band to evaluate RSA among cross sectional samples with broad age ranges, and (d) use of a single frequency band to evaluate development of RSA in longitudinal samples. Such misspecifications may yield artifactual RSA–behavior relations (Type I errors), and/or obscure real RSA behavior relations (Type II errors). Given that 4-year-olds breath at over twice the respiratory frequency of adults, such effects could be substantial.
With these caveats in mind, evidence collected to date suggests that among individuals without psychopathology, resting RSA increases linearly with age from preschool to adolescence (see Beauchaine et al., Reference Beauchaine, Katkin, Strassberg and Snarr2001; Crowell et al., Reference Crowell, Beauchaine, Gatzke-Kopp, Sylvers, Mead and Chipman-Chacon2006; Zisner & Beauchaine, Reference Zisner, Beauchaine and Cicchetti2016). After young adulthood, RSA decreases gradually across the life span, although such decreases are offset somewhat by physical fitness (e.g., Byrne, Fleg, Vaitkevicius, Wright, & Porges, Reference Byrne, Fleg, Vaitkevicius, Wright and Porges1996). As discussed above, however, accurate assessment of RSA depends on appropriate specification of respiration rates, which decrease substantially from childhood to adulthood (Fleming et al., Reference Fleming, Thompson, Stevens, Heneghan, Plüddemann, Maconochie and Mant2011; Wallis, Healy, Undy, & Maconochie, Reference Wallis, Healy, Undy and Maconochie2005). Table 1 summarizes observed respiration rates (at the 2.5 percentile, which captures 97.5% of individuals) for children and adolescents, ages 4–16 years. Most evidence for increases in RSA across development derives from assessments that have not used age-corrected respiratory frequencies. Thus, the magnitude (and existence) of developmental shifts in RSA may be uncertain. As shown in Figure 2, using an adult respiratory band with children is likely to overestimate RSA, since doing so adds spectral power that is not respiratory related. Accordingly, one objective of this article is to evaluate age effects on RSA by reanalyzing existing data from our labs that were not analyzed originally with proper respiratory frequencies.

Figure 2. (Color online) Effects of using an adult respiration frequency band to estimate respiratory sinus arrhythmia for a typical 4-year-old. The appropriate respiratory band, derived from population values (Table 1), captures spectral power between 0.33 and 0.50 Hz. Above 0.50 Hz, there is negligible if any spectral power. When an adult respiratory frequency of 0.15 Hz (also from Table 1) is used, considerable nonrespiratory-related spectral power is added, as indicated by the double-headed arrow. This adds noise to the child's respiratory sinus arrhythmia estimate. Frequency (Hz) is plotted along the x-axis, and power spectral density is plotted along the y-axis.
Table 1. Respiration rates (2.5 percentile; breaths/minute), and corresponding cycles per second (Hz) by age

Note: Respiration rates were evaluated among 1,109 children, adolescents, and adults, as reported by Wallis et al. (Reference Wallis, Healy, Undy and Maconochie2005). The 2.5 percentile captures 97.5% of individuals.
Evidence also suggests that age moderates links between RSA both internalizing and externalizing psychopathology. Group differences between externalizing samples and controls in resting RSA are often not observed until middle school (e.g., Beauchaine et al., Reference Beauchaine, Katkin, Strassberg and Snarr2001, Reference Beauchaine, Gatzke-Kopp and Mead2007; Crowell et al., Reference Crowell, Beauchaine, Gatzke-Kopp, Sylvers, Mead and Chipman-Chacon2006). Similar findings have recently been reported for depression (Koenig et al., Reference Koenig, Kemp, Beauchaine, Thayer and Kaess2016). According to developmental theories of emotion dysregulation, links between RSA and psychopathology emerge in part through protracted operant conditioning of emotional lability within families. From this perspective, deficiencies in RSA develop over time through repeated aversive interaction patterns that slowly canalize abnormal patterns of physiological activity and reactivity (see Beauchaine, Reference Beauchaine2015a, Beauchaine & McNulty, Reference Beauchaine and McNulty2013; Beauchaine & Zalewski, Reference Beauchaine, Zalewski, Dishion and Snyder2016; Crowell et al., Reference Crowell, Baucom, Yaptangco, Bride, Hsiao, McCauley and Beauchaine2014). Given the above discussion, it is important to eliminate the possibility that apparent age effects on RSA–psychopathology relations are artifactual. Thus, we evaluate whether RSA–externalizing relations differ as a function of age when age-appropriate frequency bands and AR spectral analysis are used to compute RSA. Addressing this question may help to determine whether existing findings are valid despite widespread misspecification of respiratory frequencies.
Finally, developmental changes in RSA reactivity (i.e., withdrawal) to emotion evocation are also observed, becoming less pronounced among healthy controls from preschool to adolescence (see Beauchaine et al., Reference Beauchaine, Katkin, Strassberg and Snarr2001; Crowell et al., Reference Crowell, Beauchaine, Gatzke-Kopp, Sylvers, Mead and Chipman-Chacon2006; Pang & Beauchaine, Reference Pang and Beauchaine2013). This suggests that healthy control children become better able to regulate their physiological reactions to emotion evocation as they mature. In contrast, children with externalizing behavior problems become more reactive as they mature (see Pang & Beauchaine, Reference Pang and Beauchaine2013). Accordingly, we also evaluate age effects on RSA reactivity using developmentally appropriate respiratory frequencies and AR spectral analysis.
Method
Data sets
All analyses were conducted using data from a sample that combined participants from five separate studies (total N = 559). The age range of participants was 4 to 17 years. All data sets included assessments of baseline RSA, RSA reactivity, and externalizing psychopathology. Although each study included additional measures, these are not the focus of this article, so they are not discussed. Interested readers are referred to original sources. Methods of recruitment and specific tasks used in each study, which are presented in order of increasing age, are described below. As is customary in psychophysiological research, RSA reactivity was computed as task minus baseline, and RSA data were log transformed to normalize their distributions (see Zisner & Beauchaine, Reference Zisner, Beauchaine and Cicchetti2016).
In Crowell et al.’s (Reference Crowell, Beauchaine, Gatzke-Kopp, Sylvers, Mead and Chipman-Chacon2006) study, participants between ages 4 and 6 years were recruited to investigate autonomic correlates of attention-deficit/hyperactivity disorder (ADHD) and oppositional defiant disorder (ODD) in early childhood. Patterns of RSA and RSA reactivity were compared between 18 participants with ADHD and ODD versus 20 controls (n = 38). The current analysis includes 35 of these participants (17 ADHD/ODD: 8 female, 9 male; 18 control: 9 female, 9 male). Three participants were dropped (1 ADHD/ODD, 2 control) due to file corruption. The sample was 65.7% Caucasian.
The ADHD/ODD group was ascertained via interview using the Child Symptom Inventory (CSI; Gadow & Sprafkin, Reference Gadow and Sprafkin1997a), which yields diagnostic cutoffs for most DSM-IV (American Psychiatric Association, 1994) syndromes. Parent reports on the ADHD and ODD scales of the CSI were used. Parents also completed the aggression, attention problems, and anxious/depressed subscales of the Child Behavior Checklist (CBCL; Achenbach & Edelbrock, Reference Achenbach and Edelbrock1991). Children were included in the externalizing group if they met DSM-IV criteria for both ADHD (hyperactive/impulsive or combined subtype) and ODD on the CSI, and scored at or above the 98th percentile on the attention problems and aggression subscales of the CBCL. Control group children were required to score below the 60th percentile on the CBCL scales, and to exhibit no more than two symptoms of ADHD or ODD.
All participants underwent a stimulus-free, 5-min resting baseline in a sound-attenuated room. Baseline RSA was extracted from the last minute of this rest period, which was followed by participation in a stressful, 1-min game that participants could not win. RSA was defined as spectral power above 0.15 Hz.
The Beauchaine et al. (Reference Beauchaine, Gatzke-Kopp, Neuhaus, Chipman, Reid and Webster-Stratton2013) study evaluated the efficacy of the Incredible Years® intervention (Webster-Stratton & Reid, Reference Webster-Stratton, Reid, Weisz and Kazdin2010) for 99 children, ages 4–6 years, with the hyperactive/impulsive or combined subtype of ADHD. A typically developing control group (n = 41) also participated (see Beauchaine et al., Reference Beauchaine, Neuhaus, Gatzke-Kopp, Reid, Brekke, Olliges and Webster-Stratton2015). All data reported herein were collected prior to treatment. Children who were later treated were required to (a) meet DSM-IV criteria for the hyperactive/impulsive or combined subtype of ADHD on the Diagnostic Interview Schedule for Children—Parent Report (Shaffer, Fisher, Lucas, Mina, & Schwab-Stone, Reference Shaffer, Fisher, Lucas, Mina and Schwab-Stone2000), (b) score at or above the 95th percentile on the CBCL attention problems scale, and (c) remain medication free throughout the intervention. Among the 99 treated participants, 48 also met criteria for ODD, which was not an exclusion criterion. The current analysis included 121 participants (86 ADHD: 21 female, 65 male; 35 control: 11 female, 24 male). Nineteen participants were dropped (13 ADHD, 6 control) due to problems with either data acquisition or data corruption that resulted during transfer of files to a new server. The sample was 76.0% Caucasian.
As in the Crowell et al. (Reference Crowell, Beauchaine, Gatzke-Kopp, Sylvers, Mead and Chipman-Chacon2006) study, all children underwent a stimulus-free, 5-min resting baseline in a sound-attenuated room. Baseline RSA was extracted from the last minute of this rest period. Children also participated in a challenging 7-min block building task that is quite difficult for 4- to 6-year-olds to complete, and pulls for both parental and child frustration, as assessed by dyadic conflict and expressions of negative affect (Beauchaine, Strassberg, Kees, & Drabick, Reference Beauchaine, Strassberg, Kees and Drabick2002). RSA was defined as spectral power above 0.15 Hz.
In the Fortunato, Gatzke-Kopp, and Ram (Reference Fortunato, Gatzke-Kopp and Ram2013) study, participants were drawn from a clinical trial of a preventive intervention for high-risk children (see Gatzke-Kopp, Greenberg, & Bierman, Reference Gatzke-Kopp, Greenberg and Bierman2015, for details). Briefly, all children who were enrolled in kindergarten among nine schools in a single school district were screened using teacher reports of aggressive/oppositional behavior from the Teacher Observation of Child Adaptation—Revised (Werthamer-Larsson, Kellam, & Wheeler, Reference Werthamer-Larsson, Kellam and Wheeler1991). Children who scored in the upper quartile of externalizing behavior within each classroom (n = 207) were recruited as a part of a high-risk externalizing group (diagnostic procedures were not used), and children who scored in the bottom quartile in matched classrooms (n = 132) were recruited into a low-risk comparison group. Among high-risk children, 100 were assigned randomly to a social skills training program that took place during the second half of kindergarten and the first half of first grade. The remaining 107 were assigned to a no-treatment control group. Unlike the Fortunato et al. manuscript, data included in the present analyses were taken from a 2-year follow-up when children were in second grade. Given evidence that interventions for externalizing behavior can affect autonomic function (e.g., Beauchaine et al., Reference Beauchaine, Neuhaus, Gatzke-Kopp, Reid, Brekke, Olliges and Webster-Stratton2015), children in the intervention condition were excluded from analyses.
At the time of physiological assessments, second-grade teachers completed the Strengths and Difficulties Questionnaire (SDQ; Goodman, Reference Goodman1997) to assess current levels of externalizing and internalizing symptoms. Of the original sample, 180 children remained in the school district and had teacher-supplied symptom ratings. Physiological data were missing for 3 participants, resulting in a total of n = 177 for the present analyses (100 high risk: 37 female, 63 male; 77 low risk: 24 female, 53 male). The sample was 30.5% Caucasian.
Resting RSA was computed while participants were asked to sit still for 2-min while they “traveled through space” to play a game. A moving star field video was presented on a computer screen during the recording. Participants first completed a cognitive game (these data are not evaluated here), before “traveling through space” again for another 2 min, after which they viewed four emotion-induction film clips extracted from the movie The Lion King (see Fortunato et al., Reference Fortunato, Gatzke-Kopp and Ram2013; Gatzke-Kopp, Jetha, & Segalowitz, Reference Gatzke-Kopp, Jetha and Segalowitz2014, for details). In the present analyses, baseline RSA was extracted from the final minute of the first 2-min baseline (before the child participated in any tasks), and RSA reactivity was assessed only during the sad film clip, to maintain consistency with the other studies.
The P ang and Beauchaine (Reference Beauchaine, Gatzke-Kopp, Neuhaus, Chipman, Reid and Webster-Stratton2013) study included 207 children, ages 8–12 years, who participated in a longitudinal evaluation of depression and conduct problems across middle childhood (also see Beauchaine, Hong, & Marsh, Reference Beauchaine, Hong and Marsh2008; Kopp & Beauchaine, Reference Kopp and Beauchaine2007; Shannon et al., Reference Shannon, Beauchaine, Brenner, Neuhaus and GatzkeKopp2007; Vasilev, Crowell, Beauchaine, Mead, & Gatzke-Kopp, Reference Vasilev, Crowell, Beauchaine, Mead and Gatzke-Kopp2009). Based on results of interviews with parents using the CSI, children were placed into one of the following four groups: control (n = 69) could not meet CSI criteria for ODD, conduct disorder (CD), major depressive disorders (MDD), or dysthymia (DYS); conduct problems (n = 30) met CSI criteria for CD and/or ODD, did not meet CSI criteria for DYS or MDD; depression/dysthymia (n = 28) met CSI criteria for MDD or DYS and failed to meet CSI criteria for CD and ODD; comorbid (n = 80) met inclusion criteria for both the depressed and conduct problems groups. Parents also completed the aggression, attention problems, and anxious/depressed subscales of the CBCL. Scores at or above the 95th percentile on CBCL scales that were relevant to each group (e.g., aggression for the conduct problems group, anxious/depressed for the depression/dysthymia group) were also required for admission. The current analysis included 162 participants (83 conduct problems/comorbid, 23 depression/dysthymia: 31 female, 75 male; 56 control: 30 female, 26 male). Of the original 207 participants, 45 participants were dropped due to file corruption when data were transferred to a new server (27 conduct problems/comorbid, 5 depression/dysthymia, 13 control). The sample was 61.7% Caucasian.
All participants underwent a stimulus-free, 5-min resting baseline in a sound-attenuated room. Baseline RSA was extracted from the last minute of this rest period. Next, participants watched a 3-min scene from The Champ, a film that depicts a boy with his dying father. This film is effective in eliciting strong emotional reactions (see Marsh, Beauchaine, & Williams, Reference Marsh, Beauchaine and Williams2008). RSA was defined as spectral power above 0.15 Hz.
The Beauchaine et al. (Reference Beauchaine, Katkin, Strassberg and Snarr2001) study compared patterns of psychophysiological responding among boys, ages 12–17 years, with either (a) ADHD (n = 17), (b) ADHD and CD (n = 20), or (c) no psychiatric disorder (n = 22). Diagnoses were ascertained via parent interviews using the Adolescent Symptom Inventory (Gadow & Sprafkin, Reference Gadow and Sprafkin1997b). Adolescents were included if they met DSM-IV diagnostic criteria for their respective group. Adolescents in the ADHD group were also required to score at or above the 95th percentile on the CBCL attention problems scale, below the 70th percentile on the CBCL delinquency scale, and endorse no CD criteria. Adolescents in the ADHD/CD group were required to score at or above the 95th percentile on the delinquency and aggressive behavior subscales of the CBCL. Control group participants could not meet DSM-IV criteria for any disorder, or score above the 60th percentile on any CBCL scale. The current analysis includes 64 participants (18 ADHD, 22 ADHD/CD: 0 female, 40 male; 24 control: 0 female, 24 male). Although the original analysis included 59 participants, data from 5 additional participants were collected following publication. The sample was 79.7% Caucasian.
Resting RSA was measured during the last minute of a 5-min, stimulus free baseline in a sound-attenuated room. RSA reactivity was measured while participants watched a 2-min video of an escalating conflict between age- and race-matched peers. RSA was defined as spectral power above 0.15 Hz.
Original analyses
As noted above, RSA was computed in a similar fashion across data sets. Although the second-grade data from the Fortunato et al. (Reference Fortunato, Gatzke-Kopp and Ram2013) paper have not been reported elsewhere, all other studies used FFT, and defined RSA as spectral power above 0.15 Hz. These windows are all appropriate for older adolescents and adults, not for young children or early adolescents. As described by Zisner and Beauchaine (Reference Zisner, Beauchaine and Cicchetti2016), windowing should differ depending on participant age, given age changes in respiration (see above; Table 1).
Current analysis
Kubios HRV software (version 2.2) was used to analyze all data. Kubios HRV includes options for analysis in which (a) FFT frequency bands are specified manually, and (b) AR spectral analysis is used (Tarvainen, Niskanen, Lipponen, Ranta-aho, & Karjalainen, Reference Tarvainen, Niskanen, Lipponen, Ranta-Aho and Karjalainen2014). Prior to analysis, a medium artifact correction was applied, and smoothing priors were used both to reduce distortion of data end points and to remove the lowest frequency trend components (λ = 300; Tarvainen, Ranta-aho, & Karjalainen, Reference Tarvainen, Ranta-aho and Karjalainen2002). All data were analyzed using both age-corrected FFT (FFTAC), based on respiratory frequencies specified by Wallis et al. (Reference Wallis, Healy, Undy and Maconochie2005), and AR. For the FFTAC analyses, the upper end for all frequency windows was set at 0.50 Hz, regardless of age. For example, 6-year-old children's frequency windows were set at 0.30–0.50 Hz, whereas 16-year-old children's frequency windows were set at 0.18–0.50 Hz. As noted in Table 1, these age corrections capture respiratory frequencies for 97.5% of individuals. All spectral density functions were inspected visually to ensure that respiratory peaks and their surrounding distributional dispersion were captured using an upper bound of 0.50 Hz. In all but two cases, the 0.50-Hz upper boundary was effective. For these two exceptions, the upper bound was moved manually. For AR spectral analysis, respiratory spectral power distributions were identified for each participant by Kubios. As outlined above, all baseline estimates of RSA were computed during the last minute of each individual study baseline. Task estimates of RSA were computed across the entirety of each individual study task.
In some cases, we had baseline but not task data for a participant, and in other cases we had task data but no baseline data. In these circumstances, we imputed missing data following recommendations outlined by Graham (Reference Graham2009). Baseline data were imputed for 8 participants (0.01% of total data), and task data were imputed for 19 participants (0.03% of total data).
Our index of externalizing behavior was restricted by available measures. The only measure of externalizing that was available for at least four of the five studies was the CBCL aggression subscale (Beauchaine et al., Reference Beauchaine, Katkin, Strassberg and Snarr2001, Reference Beauchaine, Gatzke-Kopp, Neuhaus, Chipman, Reid and Webster-Stratton2013, Crowell et al., Reference Crowell, Beauchaine, Gatzke-Kopp, Sylvers, Mead and Chipman-Chacon2006; Pang & Beauchaine, Reference Pang and Beauchaine2013). The conceptually closest available measure for the Fortunato et al. (Reference Fortunato, Gatzke-Kopp and Ram2013) study was SDQ conduct problems. Accordingly, SDQ conduct problem scores were converted to T scores to place them on the same metric as CBCL aggression scores (see Youth in Mind, 2013).
In order to test relations between RSA and externalizing scores, correlations were first calculated between RSA and both CBCL and SDQ scales. Analysis of variance (ANOVA) was used to evaluate potential differences between methods for both baseline and reactivity scores. Next, we compared those with clinical levels of externalizing behavior (T ≥ 70; 98th percentile) with the remainder of the sample on both baseline RSA and RSA reactivity in one-way ANOVAs. This ensured that any effects that were restricted to participants with clinical levels of psychopathology would be captured rather than swamped by correlations computed across the entire sample (see Footnote 3).
Results
Before computing inferential statistics, distributions of age and externalizing were assessed for normality. Skew and kurtosis values, respectively, were 0.49 and 0.01 for age, and 0.57 and –0.45 for externalizing. All of these values fall within acceptable ranges. Assessing normality was important given that each data set included separate clinical/high risk and control groups, and therefore did not represent the entire range of externalizing behavior in the population. Sex is not included in any of the analyses presented below, since it did not alter directions of association, change significance levels of correlations, or affect results in the group-based analyses.
Comparisons of analysis method across data sets
Correlational analyses
The total sample included 559 children and adolescents (171 female, 388 male) between ages 4 and 17 years (M = 8.19, SD = 2.94). There were significant positive correlations of large effect size among RSA analysis methods (FFT, FFTAC, and AR) for both baseline scores (all rs ≥ .72; see Table 2), and reactivity scores (all rs ≥ .58; see Table 3). At baseline, there were also significant positive correlations between age and RSA for both FFTAC and AR (both rs ≥ .13; see Table 2). For reactivity scores, age and RSA were correlated positively across all scoring methods (all rs ≥ .20; see Table 3). Thus, children became less reactive with age. At baseline, the correlation between age and RSA using FFTAC was significantly larger than the correlations between both age and RSA using AR (z = 2.54, p = .01) and FFT (z = 4.20, p < .01). Correlations between age and RSA using AR, and between age and RSA using FFT, did not differ (z = 1.66, p = .09). For reactivity, the correlation between age and RSA using AR was larger than the correlations between age and RSA using both FFTAC (z = 1.90, p = .06) and FFT (z = 2.06, p < .05). Correlations between age and RSA using FFTAC and FFT did not differ (z = 0.16, p = .87).
Table 2. Correlations among baseline RSA values and age

Note: N = 559. RSA, respiratory sinus arrhythmia; FFT, fast Fourier transform; FFTAC, FFT with age correction; AR, autoregressive.
**p ≤ .01.
Table 3. Correlations among and RSA reactivity values and age

Note: N = 559. RSA, respiratory sinus arrhythmia; FFT, fast Fourier transform; FFTAC, FFT with age correction; AR, autoregressive.
**p ≤ .01.
Resting RSA comparisons
Next, original FFT scores, which did not use age-corrected respiratory frequencies, were compared with FFTAC and AR scores at rest. There were significant main effects for all three contrasts, including the original analysis versus FFTAC comparison, F (1, 554) = 306.11, p < .001, ηp 2 = 0.36, the original analysis versus AR comparison, F (1, 554) = 69.92, p < .001, ηp 2 = 0.11, and the FFTAC versus AR comparison, F (1, 554) = 161.20, p < .001, ηp 2 = 0.23. As shown in Figure 3, these main effects indicate that baseline RSA scores were highest for the original FFT analyses and lowest for FFTAC.

Figure 3. Relations between data set and spectral power, by analysis method. Data sets are plotted along the x-axis by increasing age. Significant main effects and interactions were observed for all comparisons. Thus, original analyses, which were conducted without age-corrected respiratory frequencies, overestimated baseline respiratory sinus arrhythmia compared with both age-corrected fast Fourier transform and autoregressive techniques, especially among younger samples. See text for additional details.
These main effects must be interpreted in the context of significant Analysis Method × Data Set interactions for both the original analysis versus FFTAC comparison, F (4, 554) = 14.35, p < .001, ηp 2 = 0.09, and the FFTAC versus AR comparison, F (4, 554) = 12.14, p < .01, ηp 2 = 0.08. In addition, the Analysis Method × Data Set interaction for the original analysis versus AR comparison approached significance, F (4, 554) = 2.15, p = .07, ηp 2 = 0.02. These interactions indicate that (a) original FFT overestimated RSA compared with both FFTAC and AR among younger participants, and (b) differences between FFTAC and AR were larger among younger participants (see Figure 3).
RSA reactivity comparisons
For the reactivity analyses, there were significant main effects for the original FFT versus AR comparison, F (1, 554) = 66.39, p < .001, ηp 2 = 0.11, and the FFTAC versus AR comparison, F (1, 554) = 65.82, p < .01, ηp 2 = 0.11, but not for the original FFT versus FFTAC comparison, F (1, 554) = 0.94, p = .33, ηp 2 < 0.01. As shown in Figure 4, estimates of RSA withdrawal were smaller for both original FFT and FFTAC than for AR.

Figure 4. Relations between data set and respiratory sinus arrhythmia reactivity by analysis method. Data sets are plotted along the x-axis by increasing age. The original analyses, which were conducted without age-corrected respiratory frequencies, underestimated respiratory sinus arrhythmia reactivity among younger samples. See text for additional details.
Significant Analyses Method × Data Set interactions were also observed for all three contrasts, including the original analysis versus FFTAC comparison, F (4, 554) = 8.78, p < .001, ηp 2 = 0.06, the original analysis versus AR comparison, F (4, 554) = 17.47, p < .001, ηp 2 = 0.11, and the FFTAC versus AR comparison, F (4, 554) = 5.50, p < .01, ηp 2 = 0.04. These interactions indicate that (a) original FFT underestimated RSA reactivity among younger participants, and (b) differences between FFTAC and AR were larger among younger participants (see Figure 4).
Relations among RSA, RSA reactivity, and externalizing behavior
RSA externalizing correlations
As shown in Table 4, in the combined data set there were significant negative correlations at baseline between RSA and externalizing scores for both the original analysis method (r = –.13) and AR (r = –.12). Thus, lower baseline RSA was associated with higher externalizing scores using these methods. These associations were carried primarily by the oldest sample, for whom correlations between RSA and externalizing scores ranged from r = –.17 (FFT) to r = –.23 (FFTAC). In the other data sets, no correlations between baseline RSA and externalizing scores were significant at any age (see Table 4). No significant correlations were observed between RSA reactivity and externalizing scores at any age, regardless of measure.
Table 4. Correlations between externalizing scores and RSA values by analysis method across data sets

Note: RSA, respiratory sinus arrhythmia; FFT, fast Fourier transform; FFTAC, FFT with age correction; AR, autoregressive.
a Reactivity assessed during frustration.
b Reactivity assessed during sad mood induction.
c Reactivity assessed while viewing peer conflict.
†p < .10. **p ≤ .01.
Group differences
As noted in Footnote 3, low RSA and/or excessive RSA reactivity among participants with clinical levels of psychopathology could be swamped in correlational analyses across the larger sample, thereby going undetected. Thus, we also evaluated group differences between those whose externalizing T scores in the clinical range (T ≥ 70; 98th percentile) based on national norms (n = 178) versus the rest of the sample (n = 381). For baseline RSA, significant group differences were observed for both FFT, F (1, 557) = 6.53, p = .01, and AR, F (1, 557) = 7.08, p = .008. For FFTAC, the group effect was not significant, F (1, 557) = 2.20, p = .14. No group differences were found for RSA reactivity, all Fs (1, 557) ≤ 0.91, all ps ≥ .34. Nearly identical findings were observed when the clinical cutoff was placed at the 95th as opposed to the 98th percentile.
Discussion
Our objectives in conducting this study were twofold. First, we sought to characterize effects of misspecifying respiratory frequencies on estimates of RSA across development. Second, we sought to evaluate whether RSA–externalizing relations are observed when FFTAC and/or AR spectral analysis are used, in order to determine whether existing evidence for such relations is credible, or a possible artifact of inappropriate scoring methods. Below we discuss these objectives, and consider how our findings apply to the existing literature on the development of RSA, and its links to externalizing behavior and emotion dysregulation. We conclude with recommendations for future research.
Effects of misspecifying respiratory frequencies on estimates of RSA across development
Findings from this study indicate clearly that FFTAC or AR spectral analysis should be used when computing RSA among developmental samples. Even though original, uncorrected FFT baseline RSA values correlated highly with both FFTAC and AR estimates, uncorrected FFT values did not correlate with age, and therefore obscured developmental increases in resting RSA. No such effect was observed for RSA reactivity, for which all computational methods correlated positively with age, indicating less PNS withdrawal to eliciting stimuli across development. Smaller reductions in RSA to emotion evocation across development are consistent with the notion that emotion regulation improves as children mature.
There were also significant interactions between analysis method and data set for both baseline and reactivity measures of RSA. Without age-corrected respiratory frequencies, FFT overestimated baseline RSA, especially among the younger samples. In contrast, FFT without age-corrected respiratory frequencies underestimated RSA reactivity (withdrawal) to emotion evocation among younger samples. Finally, FFT without age-corrected respiratory frequencies underestimated developmental changes in both RSA and RSA reactivity from early childhood to adolescence. These findings suggest that literature-wide overestimates of resting RSA, underestimates of RSA reactivity, and underestimates of developmental shifts in RSA and RSA reactivity may exist. Accordingly, either age-corrected respiratory frequencies should be used when estimating RSA from FFT analysis or AR spectral analysis should be used. As reviewed above, FFTAC involves selecting appropriate respiratory frequencies for each participant based on tabulated values from large samples (Wallis et al., Reference Wallis, Healy, Undy and Maconochie2005), whereas AR spectral analysis identifies observed respiratory frequencies for each participant empirically (see Kay & Marple, Reference Kay and Marple1981).
There were also significant differences between FFTAC and AR. Specifically, FFTAC produced lower estimates of resting RSA, and higher estimates of RSA reactivity, especially among younger participants. Since AR identifies respiratory frequencies for each individual empirically rather than extracting an age-appropriate respiration rate from tabulated values, it may be more accurate than FFTAC. If this is the case, AR spectral analysis might be considered the best option in future research.
A potential concern is that the respiratory frequencies we used for FFT and FFTAC truncated spectral power distributions for some individuals, resulting in underestimates of baseline RSA and RSA reactivity, especially among younger participants. Even though we used respiration frequencies that capture 97.5% of the population (see Table 1), these values define respiratory peaks. Spectral power is disbursed around those peaks. To ensure that we captured this dispersion, we inspected all spectral density plots visually. These inspections revealed only two cases for which the high-frequency cutoff had to be shifted to capture the entire high-frequency spectral power distribution. Thus, truncating spectral power distributions was not a significant issue in this sample. The homogeneity of variance assumption was not violated in any of the ANOVAs, all Fs (4, 554) ≤ 1.52, all ps ≥ .19. However, visual inspection is quite time-consuming, which provides another argument in favor of AR.
Links between RSA and externalizing behavior
In the combined sample, there were significant negative correlations, albeit of small effect size, between baseline RSA and externalizing scores for both original FFT and AR spectral analysis, but not for FFTAC. Consistent with existing literature (e.g., Beauchaine et al., Reference Beauchaine, Katkin, Strassberg and Snarr2001; Mezzacappa et al., Reference Mezzacappa, Tremblay, Kindlon, Saul, Arseneault, Seguin and Earls1997; Pang & Beauchaine, Reference Pang and Beauchaine2013), lower resting RSA was associated with greater externalizing behavior. These findings were carried by RSA–externalizing correlations observed in the oldest sample, which were generally larger than those observed in the younger samples, particularly for FFTAC and AR.
As noted elsewhere, sympathetic nervous system deficiencies, which reliably characterize externalizing samples, are evident as early as preschool (see Beauchaine, Reference Beauchaine2015a; Crowell et al., Reference Crowell, Beauchaine, Gatzke-Kopp, Sylvers, Mead and Chipman-Chacon2006). In contrast, PNS deficiencies tend to emerge later in development (Beauchaine & Gatzke-Kopp, Reference Beauchaine and Gatzke-Kopp2012; Beauchaine et al., Reference Beauchaine, Gatzke-Kopp and Mead2007; Fortunato et al., Reference Fortunato, Gatzke-Kopp and Ram2013). Similar findings have since been reported for depressed youth, whose deficiencies in RSA emerge in adolescence, not childhood (Koenig et al., Reference Koenig, Kemp, Beauchaine, Thayer and Kaess2016). According to operant reinforcement accounts of emotion dysregulation, altered PNS activity and reactivity develop over time in families with both externalizing and internalizing children through social reinforcement mechanisms. In many of these families, escalation of dyadic conflict/invalidation and associated physiological arousal are negatively reinforced because conflict and invalidation terminate aversive interactions (see Beauchaine & Zalewski, Reference Beauchaine, Zalewski, Dishion and Snyder2016; Crowell et al., Reference Crowell, Baucom, Potapova, McCauley, Fittleson, Barth and Beauchaine2013, Reference Crowell, Baucom, Yaptangco, Bride, Hsiao, McCauley and Beauchaine2014). Through these negative reinforcement mechanisms, emotion dysregulation and associated deficiencies in RSA emerge over time among affected children (Beauchaine, Reference Beauchaine2015a). Our findings indicate that age-related changes in associations between externalizing behavior and deficiencies in resting RSA are not an artifact of using age-inappropriate respiratory frequencies when calculating RSA.
Despite very small effects in the correlational analyses, baseline RSA among those with externalizing T scores in the clinical range was lower than in the rest of the sample. This finding applied to both FFT and AR, but not to FFTAC. Although we do not know why FFTAC was less sensitive to group differences than the other methods, these findings are potentially important, as they suggest a nonlinearity in RSA–externalizing relations. As outlined in Footnote 3, normative variation on externalizing measures may be best construed as temperamentally driven rather than a marker of psychopathology. Thus, RSA may mark emotion deregulatory processes primarily among those with significant psychopathology. Similar nonlinearities apply to certain neural substrates of ADHD (Plichta & Scheres, Reference Plichta and Scheres2014).
There were no significant associations between RSA reactivity and externalizing scores, which is inconsistent with several studies of RSA reactivity among clinical samples (see above). At first glance, these findings also appear to be inconsistent with original results from three of the five constituent studies (Beauchaine et al., Reference Beauchaine, Katkin, Strassberg and Snarr2001, Reference Beauchaine, Gatzke-Kopp, Neuhaus, Chipman, Reid and Webster-Stratton2013; Pang & Beauchaine, Reference Pang and Beauchaine2013). However, the original analyses evaluated more inclusive measures of externalizing behavior, not solely aggression. In contrast, we were forced to use a measure that was available for four of the five studies.
Implications for emotion dysregulation research
Effects of misspecifying respiratory frequencies in estimating RSA may have important implications for the emotion dysregulation literature. Perhaps most important, developmental changes in RSA and RSA reactivity have likely been underestimated in previous research. Developmental increases in baseline RSA and developmental decreases in RSA reactivity to emotion evocation both suggest improved emotion regulation as children mature. According to our analyses, these developmental changes may be steeper than previously thought (cf. Zisner & Beauchaine, Reference Zisner, Beauchaine and Cicchetti2016). Without proper age corrections for respiratory frequencies, baseline RSA is overestimated, and RSA reactivity is underestimated in younger samples. Our findings indicate that variance in RSA reactivity may have been truncated in many young samples. Since RSA reactivity to emotion evocation may mark emotion regulatory capacity (see Beauchaine Reference Beauchaine2015a, Reference Beauchaine2015b; Beauchaine & Thayer, Reference Beauchaine and Thayer2015), these findings suggest that effect sizes in studies of RSA reactivity–emotion regulation associations may have been underestimated, and should therefore be revisited.
Limitations and future directions
As alluded to above, seeming discrepancies between these findings and those from previous analyses could be related to differences in externalizing measures. Unfortunately, we could not use DSM diagnoses, continuous DSM symptom counts, or broadband CBCL externalizing scores, since they were not consistently available across studies. Thus, CBCL aggression scores were used. Broadband CBCL externalizing scores include both aggression and delinquency items, and therefore reflect a broader array of problem behaviors, as do DSM conduct disorder scales. Thus, smaller effect sizes than those reported in the original analyses, those reported in the literature more broadly, may reflect use of a more circumscribed measure of externalizing conduct.
In addition to limitations already discussed, we were missing data for 70 participants, largely as a result of file corruption that occurred when transferring data to a new server. This reduced sample size and affected statistic power, and rendered data slightly different from those in the original analyses. Furthermore, different baseline and task measures were used across samples. Fortunato et al. (Reference Fortunato, Gatzke-Kopp and Ram2013) used a “vanilla” baseline (watching a neutral video), whereas all other studies used true baselines. As noted above, vanilla baselines can result in underestimates of RSA reactivity. In addition, Crowell et al. (Reference Crowell, Beauchaine, Gatzke-Kopp, Sylvers, Mead and Chipman-Chacon2006) and Beauchaine et al. (Reference Beauchaine, Gatzke-Kopp, Neuhaus, Chipman, Reid and Webster-Stratton2013) used frustration tasks, whereas Fortunato et al. (Reference Fortunato, Gatzke-Kopp and Ram2013) and Pang and Beauchaine (Reference Pang and Beauchaine2013) used sad emotion inductions, and Beauchaine et al. (Reference Beauchaine, Katkin, Strassberg and Snarr2001) used an escalating peer conflict. Frustration tasks are more demanding metabolically, which can affect RSA reactivity. However, this cannot explain null outcomes. As we have described elsewhere (e.g., Beauchaine, Reference Beauchaine2012; Zisner & Beauchaine, Reference Zisner, Beauchaine and Cicchetti2016), and as alluded to in Footnote 3, stimulus conditions should be considered carefully when interpreting psychophysiology–behavior relations, and different tasks cannot be considered equivalent. In developmental research, this presents a difficult problem, since the same stimuli cannot be used to evoke similar psychological states and reactions at all ages. Thus, even though all tasks used in the constituent studies evoked emotion, differences in tasks are unavoidably confounded with the ages of participants, and may have contributed to apparent developmental shifts in RSA reactivity from preschool to adolescence.
Finally, heterogeneity of clinical attributes across samples likely limits generalizability of our findings, particularly with respect to externalizing outcomes. Nevertheless, the finding that misspecifying respiratory frequencies among younger participants has especially large effects on accuracy of both RSA assessment and estimates of developmental shifts in RSA and RSA reactivity is likely valid.
Caveats aside, age-appropriate respiratory frequencies should be used when estimating RSA, whether applied manually via FFTAC or automatically using AR spectral analysis. Doing so will eliminate overestimates of resting RSA and underestimates of RSA reactivity among young children. Our findings apply only to situations in which adult or adolescent respiratory frequencies are used with children. Our literature search also yielded studies in which toddler (and in some cases infant) respiratory frequencies were used for child and adolescent samples. Although one might surmise that such studies would yield an opposite pattern of results, this is an empirical question that should be examined in future research. Misspecifying respiratory frequencies across development may also affect estimates of inter- and intraindividual stability in longitudinal studies. Reliabilities in developmental samples may be underestimated if incorrect respiratory frequencies are used. It is our hope that this article stimulates additional research on these and other questions.