Introduction
Most proteins are made of more than one polypeptide chain, and thus they have a quaternary structure (QS) in the classical nomenclature of Linderström-Lang & Schellman (Reference Linderström-Lang, Schellman, Boyer, Lardy and Myrback1959), who named primary structure the amino acid sequence, secondary structure, the α helices and β sheets, and tertiary structure, the chain fold. Moreover, many, if not all, proteins interact with others to form binary complexes or higher-order assemblies that carry out all types of cellular processes. Indeed, the biological function of a protein can be seen as defined by the context of its interactions in the cell, and inappropriate interactions can lead to diseases (Alberts, Reference Alberts1998; Eisenberg et al. Reference Eisenberg, Marcotte, Xenarios and Yeates2000). Thus, the unraveling of the underlying principles that govern protein–protein recognition is both central to the construction of the networks that define cell biology (Robinson et al. Reference Robinson, Sali and Baumeister2007) and instrumental in new drug development (Wells & McClendon, Reference Wells and McClendon2007).
The quaternary structure is a very early discovery in comparison with other levels of macromolecular assembly in biology. It was first identified in the mid 1920s by Svedberg (Reference Svedberg1927), when he determined the molecular weight of hemoglobin by sedimentation in the ultracentrifuge. The value he obtained, almost 68 000 Da, implied the presence of four subunits in the molecule. Sedimentation also showed that hemocyanin, a copper-containing protein, had a molecular weight of millions, and presumably many subunits. Svedberg's discovery predates by decades that of the α helix and the β sheet by Pauling & Corey (Reference Pauling and Corey1951), the first amino acid sequence of Sanger & Thompson (Reference Sanger and Thompson1953) and the first X-ray structure of Perutz (Reference Perutz1960). Perutz' crystallographic studies of hemoglobin revealed the secondary and tertiary structures of the subunits, fully confirmed Svedberg's description of its QS and showed that the QS changes when oxygen binds. They inspired Monod and his collaborators, who introduced the concept of allostery. The allosteric model of regulatory mechanisms gives a central role to the QS and the way it changes when ligands bind (Monod et al. Reference Monod, Changeux and Jacob1963, Reference Monod, Wyman and Changeux1965). In those years, only a few scores of proteins had their sequence or X-ray structure determined, but many had their QS established, mostly in the ultracentrifuge, so that Darnall & Klotz (Reference Darnall and Klotz1975) could tabulate the QS of more than 500 proteins. The advent of DNA sequencing changed the setting of protein studies altogether. Obtaining an amino acid sequence suddenly became easy and fast, and a wide gap opened between our knowledge of the primary structure and that of the other levels of protein structure. Structural genomics initiatives, launched worldwide in 1998–2000 to close that gap, initially targeted single-gene products (Sali, Reference Sali1998), a choice that reflects the views of that time. Since then, genome-wide genetic and biochemical studies have demonstrated that most gene products are part of multi-molecular assemblies in all cells and organisms (Giot et al. Reference Giot, Bader, Brouwer, Chaudhuri and Kuang2003; Li et al. Reference Li, Armstrong, Bertin, Ge, Milstein, Boxem, Vidalain, Han, Chesneau, Hao, Goldberg, Li, Martinez, Rual, Lamesch, Xu, Tewari, Wong, Zhang, Berriz, Jacotot, Vaglio, Reboul, Hirozane-Kishikawa, Li, Gabel, Elewa, Baumgartner, Rose, Yu, Bosak, Sequerra, Fraser, Mango, Saxton, Strome, Van Den Heuvel, Piano, Vandenhaute, Sardet, Gerstein, Doucette-Stamm, Gunsalus, Harper, Cusick, Roth, Hill and Vidal2004; Gavin et al. Reference Gavin, Aloy, Grandi and Krause2006; Krogan et al. Reference Krogan, Cagney, Yu, Zhong, Guo, Ignatchenko, Li, Pu, Datta, Tikuisis, Punna, Peregrín-Alvarez, Shales, Zhang, Davey, Robinson, Paccanaro, Bray, Sheung, Beattie, Richards, Canadien, Lalev, Mena, Wong, Starostine, Canete, Vlasblom, Wu, Orsi, Collins, Chandran, Haw, Rilstone, Gandi, Thompson, Musso, St Onge, Ghanny, Lam, Butland, Altaf-Ul, Kanaya, Shilatifard, O'Shea, Weissman, Ingles, Hughes, Parkinson, Gerstein, Wodak, Emili and Greenblatt2006). Protein–protein interaction and QS have now returned to the front of the stage, and protein assemblies are the targets of several recent structural genomics initiatives (Russell et al. Reference Russell, Alber, Aloy, Davis, Korkin, Pichaud, Topf and Sali2004; Janin, Reference Janin2007), and structural biologists make major efforts to study them by crystallography, nuclear magnetic resonance (NMR) and electron microscopy.
The QS of a protein or a protein assembly is almost invariably essential to its function, and it must be established along with the sequence and fold of its components. This implies determining first the subunit composition, then the geometry of the assembly, and especially its symmetry, and lastly, the details of the interactions made by chemical groups and amino acid residues at the interfaces between the subunits. This review is devoted to the analysis of such interactions in different types of assemblies for which high-resolution structural data are available from X-ray studies. Protein–protein complexes are non-obligate, and mostly transient, assemblies that form when two preformed proteins meet. Oligomeric proteins assemble as the constituent polypeptide chains fold, and are mostly permanent; as their name (coined by Monod) implies, they comprise a few subunits. Icosahedral virus capsids are also permanent, but they comprise tens to hundreds of subunits. Whereas X-ray studies usually leave the nucleic acid component of icosahedral viruses undefined, a comparison of protein–protein interaction with protein–DNA and protein–RNA interaction is of general interest, and we include here data on all three processes of macromolecular recognition.
Since Svedberg, hemoglobin has been the paradigm oligomeric protein. Mammalian hemoglobins are heterotetramers, ‘hetero’ referring to the different amino acid sequences of the α and β chains. Their QS can be noted as α2β2 or (αβ)2 to show that they comprise two αβ pairs related by twofold symmetry. The pairs are oriented differently in deoxy and in oxy-hemoglobin, which affects their interface and leads to the change in heme affinity for oxygen that makes oxygen binding cooperative (Perutz, Reference Perutz1970; Baldwin & Chothia, Reference Baldwin and Chothia1979). Most animal species have hemoglobins. Their sequences are related and they have the same characteristic fold, but not necessarily the same QS: some are homodimers (the two chains have the same sequence), others are monomers, or form larger assemblies. Their function is to bind oxygen in all cases, but the diversity of the QS allows oxygen binding to be regulated in different manners adapted to the physiology of each organism. A number of oligomeric proteins and protein–protein complexes have regulatory properties like hemoglobin. In these proteins and many others, the function directly implicates the subunit interactions. Thus, the antigen binding site of an immunoglobulin is shared between the heavy and light chains, the active site of an oligomeric enzyme can be at a subunit interface, and molecular machines work by changing subunit–subunit contacts in a cyclic manner; ATP synthase (Stock et al. Reference Stock, Leslie and Walker1999, Reference Stock, Gibbons, Arechaga, Leslie and Walker2000) is a well-known example. An understanding of their biological function depends on analyzing their structure and the interactions between their subunits.
The renewed interest in protein–protein interaction has led to the publication in recent years of several major reviews (Noreen & Thornton, Reference Noreen and Thornton2003a; Ponstingl et al. Reference Ponstingl, Kabir, Gorse and Thornton2005; Janin et al. Reference Janin, Rodier, Chakrabarti and Bahadur2007) and collective books (Kleanthous, Reference Kleanthous2000; Janin & Wodak, Reference Janin and Wodak2003; Fu, Reference Fu2004). New tools have been developed for its study in domains that range from genetics, cell biology and biochemistry to analytical chemistry, biophysics and structural biology. The emphasis in this review is on structure-based bioinformatics and computational tools, and especially the tools that are publicly available as Web servers (URLs are cited in Table 1). We review here results of their application to sets of complexes, oligomeric proteins and viral capsids, which illustrate the role of protein–protein interaction in a wide variety of biological processes. We also introduce for comparison data on two systems of a different nature: protein–nucleic acid complexes and protein crystals. The first illustrates how the chemical nature of the partners modulates macromolecular interactions, and the second sheds light on the structural basis of specificity, an essential feature of biological assemblies that crystal packing lacks. The general rules that can be drawn from that analysis are relevant to the nature, the stability and the specificity of the interactions and shed light on protein evolution and the manner in which biological macromolecules self-assemble.
Table 1. Databases and Web servers for structure-based protein–protein interactions

Tools to study quaternary structure
Experimental determination of the subunit composition
To determine the QS of a protein, one needs first to know its subunit composition. This may be established by introducing chemical cross-links between the polypeptide chains, or, more commonly, by comparing the molecular weights of the native protein and the constituent chains. The subunit molecular weights are obtained by gel electrophoresis under denaturing conditions, or calculated from the amino acid sequence taking into account post-translational modifications, if any. They can also be accurately measured by mass spectrometry, a powerful method not easily applicable to the native protein at present, because non-covalent bonds tend to break during sample desorption. Appropriate procedures are actively developed, and mass spectrometry will certainly be in a near future the choice method to determine the QS of proteins (Benesch & Robinson, Reference Benesch and Robinson2006).
At present, native molecular weights are commonly measured directly by equilibrium analytical centrifugation, static light scattering or small-angle X-ray scattering (SAXS), methods that require purified protein in milligram quantity, or indirectly by methods less demanding in terms of equipment and the protein sample. Dynamic light scattering (DLS) measurements of the translational diffusion coefficient, and NMR or fluorescence polarization measurements of the rotational diffusion coefficient, yield data from which a molecular weight can be derived if the protein is known to be globular. Gel filtration on a molecular sieve (also called size exclusion chromatography), the most common method of all, yields the Stokes radius of the protein. Since the diffusion coefficients and the Stokes radius depend on the shape of the protein as well as its size, a QS based on a gel filtration pattern or a DLS measurement may not be correct and it should be verified by other methods.
Most non-obligate complexes, and a few oligomeric proteins, dissociate at low concentration. This can be seen in the ultracentrifuge, or by gel filtration or DLS, when the dissociation constant of the same order as the protein concentration, or typically 10−6–10−4 m in such studies. However, a heterogeneous sample may yield a similar pattern to a monomer–oligomer equilibrium, and the measurement has to be made at several concentrations in order to demonstrate that the system is at thermodynamic equilibrium. With non-obligate complexes, the equilibrium can also be analyzed after mixing the components, but this is not generally feasible with oligomeric proteins, very few of which are available in a monomeric form.
Molecular symmetry of oligomeric proteins
A protein with n identical subunits usually has internal symmetry. The symmetry operations that superimpose an object onto itself form a point group. Mirror symmetries being excluded for proteins, the point group can be of one of three types: cyclic, dihedral or cubic (Fig. 1). Oligomers that display the symmetries of a cyclic C n group have an n-fold axis: their subunits are related by 360°/n rotations. The dihedral D m groups require an even number of subunits, n=2m; they possess an m2-fold axis and m2-fold axes orthogonal to it. The T (tetrahedral) cubic point group has non-orthogonal twofold and threefold axes; in addition, the O (octahedral) point group has fourfold axes, and the I (icosahedral) point group, fivefold axes.

Fig. 1. Symmetry of oligomeric proteins. An oligomeric protein with n identical subunits may have the symmetries of the cyclic C n point group (top row), one with 2n subunits, the symmetries of the dihedral D n point group (middle row); cubic symmetries (bottom row) require the protein to have 12, 24 or 60 identical subunits. Symmetry axes of different types are marked as dotted lines. Courtesy of E. Lévy (Cambridge, UK).
Symmetry is a general property of oligomeric proteins (Goodsell & Olson, Reference Goodsell and Olson2000). The most common is C 2 in homodimers, but in larger oligomers, dihedral symmetry is much more frequent than cyclic symmetry, for soluble proteins at least. Thus, D 2 tetramers are more common than C 4, and D 3 hexamers are more common than C 6. In contrast to soluble proteins, membrane proteins do not normally display dihedral symmetry, incompatible with the polarity of biological membranes, but they often have cyclic symmetry. Examples are the C 3 trimer of bacteriorhodopsin, the C 4 tetramer of the potassium channel and the C 5 pentameric acetylcholine receptor. Cubic symmetry requires n to be a multiple of 12 in the T point group, 24 in O and 60 in I. As a consequence, it is present only in large oligomers, and the best-documented example is the icosahedral symmetry of the viral capsids discussed below.
Quaternary structure and the Protein Data Bank
The Protein Data Bank (PDB; Berman et al. Reference Berman, Westbrook, Feng, Gilliland, Bhat, Weissig, Shindyalov and Bourne2000) stores atomic coordinates issued from X-ray and NMR studies. In April 2008, the PDB contained more than 50 000 entries describing the atomic structure of some 20 000 different proteins. It should be the natural place to look for their QS, yet deriving a QS from the information in a PDB entry is cumbersome and sometimes misleading. The reason is intrinsic to crystallography: in a protein crystal, inter-molecular contacts coexist with the subunit contacts that define the QS, and distinguishing one from the other is sometimes not straightforward. Algorithms specifically developed for this purpose have been reviewed by Poupon & Janin (in press). The problem does not arise for NMR structures, which are determined in solution, but few NMR studies address oligomeric proteins or protein–protein complexes, due to their larger size and symmetry that creates ambiguities when assigning resonances.
By convention, a crystallographic PDB entry reports atomic coordinates for the crystal asymmetric unit (ASU), rather than the molecular assembly in solution, which the PDB defines as the biomolecule. There is no simple relation between the ASU and the biomolecule: a monomeric protein can yield crystals with two or more chains in the ASU, an oligomeric protein, crystals with only one chain, in which case its symmetry must be a crystal symmetry. The QS is often not mentioned as such in a PDB entry, and when the word ‘dimer’ appears, the protein needs not be a dimer in solution. Since 1999, most PDB entries contain two records that define the biomolecule. REMARK 300 relates its subunit composition to the content of the ASU; REMARK 350 cites the matrices needed to build it from the ASU. Thus, if a homodimeric protein crystallizes with a monomer in the ASU, REMARK 300 will mention one chain and REMARK 350 two matrices. But if there is a dimer in the ASU, REMARK 300 will cite two chains, and REMARK 350, only the identity matrix.
Converting this information into a QS requires some effort, but several databases offer that service and give access to the atomic coordinates of the biomolecule: Biounit, ProtBuD and 3 D-Complex (described in Section 2.4). Biounit, accessible through the PDB interface at the Research Collaboratory for Structural Bioinformatics (RCSB; Rutgers University, New Jersey), relies on REMARK 300/350 or on supporting information from the authors if the records are absent. ProtBuD (Protein Biological Unit Database; Xu et al. Reference Xu, Canutescu, Obradovic and Dunbrack2006) reports the QS of the biomolecule in both the PDB and the PQS database. Probable Quaternary Structure (PQS; Henrick & Thornton, Reference Henrick and Thornton1998), PITA (Protein InTerfaces and Assemblies; Ponstingl et al. Reference Ponstingl, Kabir and Thornton2003) and PISA (Protein Interfaces, Surfaces and Assemblies; Krissinel & Henrick, Reference Krissinel and Henrick2007), implement the approach of the problem developed at the European Bioinformatics Institute (EBI-EMBL, Hinxton, UK). It is based on the geometric and physical chemical properties of the interfaces between molecules, and ignores the information in the header of a PDB entry, although the two agree in 82% of the cases (Xu et al. Reference Xu, Canutescu, Obradovic and Dunbrack2006). PQS and PITA apply crystal symmetries to the molecules in the ASU, generate neighbors and score each pairwise interface on the basis of the buried area, plus a solvation energy term in PQS or a statistical potential in PITA. The QS is then iteratively built by retaining the interfaces that achieve high scores. In PISA, the iterative construction is replaced by a graph exploration that surveys all the assemblies that can be formed in the crystal. PISA handles non-protein components, and it may detect assemblies missed by PQS or PITA. Given a PDB code or a set of atomic coordinates, the user interfaces of all three servers return information on the pairwise interfaces and the assemblies that pass their respective criteria, and they allow downloading their atomic coordinates.
Mining the biochemical literature
The QS information in the PDB is not documented and may not be updated when new data become available. It should therefore be completed, and possibly corrected, by surveying the biochemical literature and identifying data that concern the protein assembly in solution. The analysis of the interfaces in protein–protein complexes and oligomeric proteins described in Section 4 below has been performed on curated sets that were assembled by manual surveys, and represent only a small fraction of the PDB. Recently, Lévy (Reference Lévy2007) carried out a large-scale literature search with keywords related to the QS and to methods for molecular weight determination. He was able to assign the QS of more than 3000 proteins, and cover about one-quarter of the PDB by extending the assignment to close homologs. The agreement with the curated datasets is nearly perfect, but the annotated QS disagrees with the PDB biomolecule in about 15% of the entries, and in up to 27% of the proteins with non-redundant sequences.
The results of the search are accessible through the PiQSi (Protein Quaternary Structure Investigation; Lévy, Reference Lévy2007) database, which is derived from the 3D Complex database (Lévy et al. Reference Lévy, Pereira-Leal, Chothia and Teichmann2006), and interlinked with it. Like Biounit, 3D Complex relies on the information in the PDB entries, but its graph description of the QS and its hierarchic structure are original. The QS hierarchy of 3D Complex, shared with PiQSi and inspired of the domain hierarchy in SCOP (Murzin et al. Reference Murzin, Brenner, Hubbard and Chothia1995), has a top level of ‘topologies’ that depend on the number of subunits, the symmetry and the pattern of contacts in the molecular assembly. Below, it has ‘families’ that represent evolutionary relationships, and QSx ‘classes’ in which x is the sequence identity between equivalent chains in related assemblies. PiQSi, which initially contained about 10 000 entries, is being updated to cover the whole PDB. When a PDB code or a protein sequence is entered, the interface displays the protein QS as a graph, and cites the MedLine ID code of the references used to annotate it (Fig. 2). A tag indicates whether the biomolecule in the PDB is thought to be correct, incorrect or uncertain, and points to a comment that supports the annotator's opinion. PiQSi has another very valuable feature: users can submit new annotations that will be processed by the curators and eventually propagated to the database.

Fig. 2. Graph representation of the protein QS. The PiQSi database (Lévy, Reference Lévy2007) reports the QS of proteins in the PDB, checked against the literature. 4hhb (top): Human hemoglobin is shown as a graph where the nodes are the α and β subunits (in two different colors), and the edges are the subunit contacts in the tetramer; the label next to each edge is the number of residues implicated; the tetramer has (approximate) D 2 symmetry. 1b4s (bottom): The nucleoside diphosphate kinase of Dictyostelium discoideum is a homohexamer with D 3 symmetry; ‘NO’ in the ‘Error?’ column indicates that the literature agrees with the QS described in the PDB entry.
Tools to study macromolecular interfaces
Geometry and the definition of interfaces
The buried surface model
Given the atomic structure of a macromolecular assembly, defining the interface between two components A and B may be viewed as a problem of geometry in space. The simplest definition is based on distance: the AB interface is the set of atoms or chemical groups i of A and j of B, which satisfy the condition d ij<d 0. In most implementations, d 0 depends on the atomic or group radii r i and r j, and on a cutoff value r 0 in the range 0·5–2 Å:

The interface can also be defined as the surface buried between the two components (Chothia & Janin, Reference Chothia and Janin1975). Given the solvent accessible surfaces of A, B and the AB pair, any point of the accessible surface of A or B that does not belong to the accessible surface of AB is part of the interface. The solvent accessible surface is at one probe radius (r W=1·4–1·5 Å for a water probe) of the molecular surface, and its construction may implement the rolling sphere algorithm of Lee & Richards (Reference Lee and Richards1971), or an analytical algorithm. The buried surface area, or interface area, that represents the interface size, is computed as:

where ASAA, ASAB and ASAAB are the accessible surface areas of A, B and the AB pair. In that model, the atoms and residues that lose ASA in AB are part of the interface. The BSA is essentially proportional to their number, and also to the number of atom pairs that satisfy Eq. (1) with r 0=2r W.
Several servers, including PP at University College London, and PISA at the European Bioinformatics Institute (see Section 2.3), return the areas of the solvent accessible (ASA) and of the buried surfaces (BSA) of individual polypeptide chains, residues and atoms, when atomic coordinates are entered. These servers, and many publications, quote a BSA ‘per subunit’, that is BSA/2 in Eq. (2). This assumes implicitly that A and B bury the same surface area, which is exact only for interfaces with C 2 symmetry. The difference between the contributions of the two partners to the BSA depends on the shape of the interface. In most cases, it is only a few percents, but it may exceed 10% when a convex surface is in contact with a concave surface; examples are protease inhibitors binding to proteases (Lo Conte et al. Reference Lo Conte, Chothia and Janin1999) and RNA binding to proteins (Bahadur et al. Reference Bahadur, Zacharias and Janin2008).
The Voronoi model
An alternative definition of interfaces is based on the Voronoi diagram and a related construction, the alpha-complex. The Voronoi diagram associates to each atom its Voronoi cell, the convex polyhedron that contains all points of space closer to that atom than to any other atom. Its first application to proteins was developed by Richards (Reference Richards1974) to evaluate the volume occupied by individual atoms or chemical groups. Because it does not account for the different sizes of the chemical groups, the Voronoi diagram is at present replaced in most applications by the closely related power diagram (Gellatly & Finney, Reference Gellatly and Finney1982; Aurenhammer, Reference Aurenhammer1987). Instead of the Euclidean distance |ax| between a point x and an atom centered in a, this diagram uses the power distance p(x) of x with respect to the ball of radius r that represents the atom or chemical group:

The Voronoi cell of an atom then comprises all points of space that have a power distance to that atom less than to any other atom. Its facets belong to the radical plane, which contains the intersection of the spheres if they do intersect.
The Voronoi (or power) diagram offers a natural definition of contacts: two atoms are in contact if and only if their Voronoi cells share a facet. Labeling molecules A and B with different colors, the set of ‘bicolor’ facets shared by atoms of A and B forms the AB Voronoi interface (Fig. 3). However, the atoms on the molecular surface all have unbounded or poorly defined Voronoi cells, a problem that may be circumvented by placing solvent molecules on the surface (Soyer et al. Reference Soyer, Chomilier, Mornon, Jullien and Sadoc2000; Dupuis et al. Reference Dupuis, Sadoc, Jullien, Angelov and Mornon2005), or solved in a mathematically more rigorous manner by using an extension of the power diagram. This extension, called the alpha complex (Edelsbrunner & Mucke, Reference Edelsbrunner and Mucke1994), makes use of a remarkable property of the power diagram: it is invariant when a constant α is added to all squared radii, r 2, in Eq. (3). For a given value of α, the alpha complex is built like a power diagram, except that one restricts the Voronoi cell of each atom to its associated ball and seeks intersections between these restricted regions. Thus, a facet between two atoms is not part of the alpha complex if the associated balls do not intersect, or if the facet lies outside the intersection.

Fig. 3. The Voronoi model of a protein–protein interface. The interface between hen egg white lysozyme (gray ribbon) and the Fv fragment of antibody D1.3 (heavy chain in red, light chain in blue) is drawn as a set of Voronoi facets that belong to the alpha-complex for α=0. Unbounded facets have been removed by the procedure of Cazals et al. (Reference Cazals, Proust, Bahadur and Janin2006). The interface is heavily hydrated with the water molecules drawn as gray spheres. PDB entry 1vfb (Bhat et al. Reference Bhat, Bentley, Boulot, Greene, Tello, Dall'Acqua, Souchon, Schwarz, Mariuzza and Poljak1994). Courtesy of F. Cazals (Sophia-Antipolis, France).
Applications of the alpha complex to macromolecules have been reviewed by Poupon (Reference Poupon2004). The interfaces defined in this manner may still contain a few unbounded facets that Edelbrunner and collaborators (Ban et al. Reference Ban, Edelsbrunner and Rudolph2004; Headd et al. Reference Headd, Ban, Brown, Edelsbrunner, Vaidya and Rudolph2007) removed through an elaborate iterative retraction procedure. The algorithm of Cazals et al. (Reference Cazals, Proust, Bahadur and Janin2006), implemented on the Intervor server, does it on purely geometric criteria. Both procedures have been applied to a set of protein–protein interfaces that had been previously analyzed with the buried surface approach by Chakrabarti & Janin (Reference Chakrabarti and Janin2002). The Voronoi interface area, calculated as the sum of the areas of the bicolor facets, correlates linearly with the BSA in both cases, but the correlation is much better with the procedure of Cazals et al. (Reference Cazals, Proust, Bahadur and Janin2006) than that of Ban et al. (Reference Ban, Edelsbrunner and Rudolph2004) (R 2=0·98 vs. 0·85). A remarkable result is that about 13% of the atoms that share bicolor facets do not contribute to the BSA, mostly because they are not solvent accessible in the free molecules. Thus, the Voronoi interface comprises significantly more atoms, and especially more main chain atoms, than the buried surface.
Topology: modules, patches, core, rim and segments
The topology of an interface represents the various ways in which it can be split. The contacts between macromolecules often implicate several regions on the surface of each partner, and these regions have been given different names by different authors: interaction sites (Jones & Thornton, Reference Jones, Thornton and Kleanthous2000), recognition patches (Chakrabarti & Janin, Reference Chakrabarti and Janin2002), interface modules (Reichmann et al. Reference Reichmann, Rahat, Albeck, Meged, Dym and Schreiber2005, Reference Reichmann, Rahat, Cohen, Neuvirth and Schreiber2007). They often belong to distinct structural domains that may constitute autonomous units in the proteins, each with a specific interaction pattern (Copley et al. Reference Copley, Ponting, Schultz and Bork2002). Classical examples are the immunoglobulin domains or the Sarc homology SH2 and SH3 domains, but databases such as InterPro (Apweiler et al. Reference Apweiler, Attwood, Bairoch and Bateman2001), PFAM and its derivative iPFAM (Finn et al. Reference Finn, Marshall and Bateman2005) list thousands more. The topology of an interface may also be defined biochemically. The interface modules of Reichmann et al. (Reference Reichmann, Rahat, Albeck, Meged, Dym and Schreiber2005) are obtained by grouping residues on the basis of the cooperative interactions observed in double mutant cycle experiments: the interactions are high within a module, and negligible between modules. In the systems studied by Reichmann et al. (Reference Reichmann, Rahat, Albeck, Meged, Dym and Schreiber2005), clustering the residues by distance yields the same grouping as the cooperative interactions. This suggests that the modules should resemble the ‘hot regions’ of Keskin et al. (Reference Keskin, Ma and Nussinov2005), which group adjacent conserved hotspots from alanine-scanning experiments (see Section 4.1.3).
The recognition patches of Chakrabarti & Janin (Reference Chakrabarti and Janin2002) are identified by applying to the interface atoms the average linkage method, a geometric clustering algorithm that relies on a threshold distance d m. The algorithm is run separately on each component protein, and therefore one component may contain more clusters than the other. However, in the tests performed by Chakrabarti & Janin (Reference Chakrabarti and Janin2002) on a set of 70 protein–protein complexes, the difference was never more than one with the default value d m=15 Å, half the maximum distance between atoms in the set, and it could be brought to zero by adjusting d m to values in the range 15–20 Å. Thus, the recognition patches generally occur in pairs, one on each component protein. The 70 complexes contain 46 single-patch interfaces (with one patch on each component), and 24 that are multi-patch.
Jones & Thornton (Reference Jones and Thornton1997) used a related procedure in a study aiming to predict interaction sites from amino acid sequences. n being the number of residues in an observed interface, they defined surface patches as sets of n solvent-accessible residues surrounding a central accessible residue, and compared the values of six different parameters in the patches that overlapped with the actual interface and those that did not. Three of the parameters were physical–chemical: a solvation potential, a residue interface propensity and hydrophobicity. The other three were geometric: the mean ASA of the residues in the patch, a planarity and a protrusion index. Planarity was measured by the root mean squared deviation of all the patch atoms from the least squares plane through the atoms, protrusion, by fitting an ellipsoid to the protein or domain (Thornton et al. Reference Thornton, Edwards, Taylor and Barlow1986).
The Voronoi model offers an alternative definition of the topology. The set of facets that form the Voronoi interface may be split into subsets of facets that have edges in common (Fig. 4). The subsets, named connected components by Cazals et al. (Reference Cazals, Proust, Bahadur and Janin2006), correlate well with the recognition patches identified by the clustering algorithm in the 70 interfaces of Chakrabarti & Janin (Reference Chakrabarti and Janin2002), and because their definition does not depend on a distance cutoff, they may represent a more robust description of the interface topology.

Fig. 4. Two views of the topology of a protein–protein interface. The heterotrimeric G-protein transducin (1got; Lambright et al. Reference Lambright, Sondek, Bohm, Skiba, Hamm and Sigler1996) is drawn with main chain tubes in cyan for the Gβ and Gγ subunits, and a gray surface for the Gα subunit. The geometric clustering procedure of Charkrabarti & Janin (Reference Chakrabarti and Janin2002) splits the region of the Gα surface in contact with Gβγ into two recognition patches painted blue and red. The red surface belongs to the N-terminal helix of Gα, which is disordered in free Gα. On the right, the Voronoi model of the interface (Cazals et al. Reference Cazals, Proust, Bahadur and Janin2006) is seen to give the same description of its topology: the two connected components correspond to the two patches, each surrounded by hydration water.
Another approach distinguishes between the regions of an interface that are fully buried and those that remain partly accessible to solvent. In the macromolecular assemblies considered here, only a minority (20–45%) of the interface atoms have zero ASA, and they tend to belong to hydrophobic residues. Chakrabarti & Janin (Reference Chakrabarti and Janin2002) define the interface core as the set of residues that contain atoms buried at the interface; the remaining interface residues form the rim. Section 4.1.3 relates the core/rim topology to the ‘O ring’ model of protein–protein interfaces put forward by Bogan & Thorn (Reference Bogan and Thorn1998) based on site-directed mutagenesis data. These data, collected in the ASEdb database (Thorn & Bogan, Reference Thorn and Bogan2001), suggest that the ‘hotspot’ residues, those that have a large effect on the stability of a complex when mutated, tend to be surrounded by energetically less important residues that occlude bulk solvent from them. The former should therefore belong to the core of the interface, and the latter, to its rim. Reichmann et al. (Reference Reichmann, Rahat, Albeck, Meged, Dym and Schreiber2005) and Keskin et al. (Reference Keskin, Ma and Nussinov2005) have reached similar conclusions from different starting points.
Interfaces can also be split into segments along the polypeptide chain. Jones & Thornton (Reference Jones and Thornton1996) define interface segments as stretches of residues that start and end with an interface residue. A segment may contain intervening non-interface residues, but only in stretches of n residues or less; n=5 in their implementation, n=4 in that of Pal et al. (Reference Pal, Chakrabarti, Bahadur, Rodier and Janin2007) and in the data presented in Section 4. The number of segments, typically 1–8 in protein–protein complexes, and that of interface residues per segment, typically 1–10, describe how these residues are distributed along the polypeptide chain. Another point of interest is their distribution into secondary structure elements (Guharoy & Chakrabarti, Reference Guharoy and Chakrabarti2007).
Atomic packing, cavities and shape complementarity
The Voronoi diagram allows making a precise estimation of the atomic packing. The Voronoi cell of an atom buried inside a protein represents the space it occupies, and its volume is essentially determined by its chemical nature (Richards, Reference Richards1974). The volumes occupied by atoms buried inside proteins are the same, or possibly slightly smaller, as in crystals of small molecules or peptides (Harpaz et al. Reference Harpaz, Gerstein and Chothia1994; Pontius et al. Reference Pontius, Richelle and Wodak1996; Tsai et al. Reference Tsai, Taylor, Chothia and Gerstein1999; Tsai & Gerstein, Reference Tsai and Gerstein2002). Therefore, the interior of proteins must be tightly packed in spite of the occasional cavities. The Voronoi volumes of surface atoms, obtained by modelling the solvent around the protein, are about 7% larger (Gerstein et al. Reference Gerstein, Tsai and Levitt1995; Gerstein & Chothia, Reference Gerstein and Chothia1996), which suggests that the packing is less tight on the protein surface.
Voronoi cells can also be constructed for atoms buried at interfaces. Those are relatively few, but the construction can make use of the crystallographic solvent molecules reported in high-resolution X-ray structures, in which case the volume measurement can be made on a majority of the interface atoms. Atoms at the interfaces of protein–protein complexes (Lo Conte et al. Reference Lo Conte, Chothia and Janin1999) and of oligomeric proteins (Ponstingl et al. Reference Ponstingl, Kabir, Gorse and Thornton2005) have been shown in this way to occupy the same volume as inside proteins to within 1–2%. This implies that they are close-packed, and therefore, that the molecular surfaces buried in the contact have complementary shapes. However, the fraction of the interface atoms that are buried is itself a parameter that depends on the quality of the packing. A poor fit of the molecular surfaces creates cavities (Hubbard & Argos, Reference Hubbard and Argos1994), and results in an interface that buries comparatively few atoms, yet these atoms may still be close-packed.
Several other estimates of the atomic packing at interfaces have been proposed over the years. The first was the shape complementarity index S c of Lawrence & Colman (Reference Lawrence and Colman1993). Given the coordinates of the AB complex, S c is evaluated by first using the program MS of Connolly (Reference Connolly1983) to define grid points of the molecular surface of A and B, and calculating a local index S(x) at all grid points x:

where d is the distance of x on A to the closest grid point on B, u is the angle of the normal vectors to the surfaces at these two points, w is a weight factor adjusted to 0·5 Å−2. S c is the median value of S(x) after removing grid points on the edge of the contact region that yield abnormal low values. Protein–protein complexes and oligomeric proteins typically have S c values near 0·70 (Lawrence & Colman, Reference Lawrence and Colman1993; Bahadur et al. Reference Bahadur, Chakrabarti, Rodier and Janin2004).
Another approach to atomic packing is to detect cavities and measure their volume. Program SURFNET (Laskowski, Reference Laskowski1995) does that by fitting spheres in between the two molecular surfaces; spheres in contact with atoms at the edge of the interface are removed if their radius exceeds 10 Å. The volume of the union of the spheres is the gap volume V gap, and the ratio to the buried surface area, the gap volume index:

Typical values of I gap in complexes and oligomeric proteins are in the range 2–3 Å (Jones & Thornton, Reference Jones and Thornton1996). The PP server at University College London allows calculating V gap and I gap from input atomic coordinates. Because the larger spheres tend to be on the edge, the gap volume index may take inconsistent values when the interface is small, or split into many patches that have a high proportion of edge atoms. This remark also applies to the edge removal step in the S c index calculation.
The ‘local’ L D and ‘global’ G D indexes of Bahadur et al. (Reference Bahadur, Chakrabarti, Rodier and Janin2004) are less sensitive to edge effects. L D is the mean value over i of the local density of the interface atoms, estimated as the number n i of those that are within a given distance D of interface atom i. With D=12 Å, L D is in the range 40–45 for most complexes and oligomeric proteins. G D, which also represents a surface density, is estimated as the ratio N/A ellips, where N is the number of the interface atoms and A ellips is the area of the equatorial section of their ellipsoid of inertia. Typical values of G D are near 1·3 atom Å−2 (Bahadur et al. Reference Bahadur, Chakrabarti, Rodier and Janin2004). The ProFace server of the Bose Institute, Calcutta (Saha et al. Reference Saha, Bahadur, Pal, Mandal and Chakrabarti2006), returns values of several interface parameters including L D when coordinates are uploaded.
Chemical and physical–chemical properties
Chemical and amino acid compositions
The chemical composition of an interface is commonly characterized by the relative abundance of the different types of atoms or amino acid residues. Atom types are often defined as non-polar/polar/charged, or main chain/side chains. The latter distinction is useful because the protein main chain contributes significantly to most macromolecular interfaces, and biochemical approaches based on site directed mutagenesis tend to ignore that contribution. In addition to protein atoms, the interface may comprise water molecules (see Section 3.4.3).
A composition may be evaluated by counting interface atoms or residues, or by measuring their contribution to the BSA. The two definitions are nearly equivalent for atoms, but with residues, the area-based composition reflects their size as well as their abundance. In either case, the fractions may be converted into propensities:

where f i is the number or area fraction of type i at the interface, f i°, the corresponding number in a reference set that can be the whole protein, its interior or its surface. If the reference is the protein surface, f i° may be either a fraction of the number of solvent-accessible atoms or residues, or a fraction of their contribution to the ASA. Then, p i>0 implies that interfaces are enriched in atoms or residues of type i relative to the protein surface in contact with the solvent; p i<0 implies that the interfaces are depleted in type i. An interface commonly comprises only a few tens of residues, and propensities calculated on individual interfaces or on small sets with 20 residue types have poor statistics. Grouping types with similar properties may improve the statistics, but this will mask at least one interesting feature: Arg and Lys are usually counted as similar, yet protein–protein interfaces are depleted in Lys, but not Arg.
Hydrophobicity
Hydrophilicity/hydrophobicity is an important physical chemical property of the protein surface, and possibly the one most relevant to its propensity to interact with a ligand. It can be estimated at the atomic level: aliphatic and aromatic groups in proteins are non-polar, and therefore hydrophobic; O- and N-containing groups are polar and hydrophilic. When they interact with a ligand, all these groups become partly or fully dehydrated, and groups of the ligand also, allowing solvent molecules to gain degrees of freedom. Their entropy increases, causing the hydrophobic effect to favor association. The system enthalpy also changes, and it can do so in either direction, because van der Waals and polar interactions made by surface groups with the solvent are replaced by protein–ligand interactions. The whole process can be viewed as a transfer of the ligand from water to the protein environment. With hydrocarbons, which make similar van der Waals interactions with water and other solvents, and no polar interaction, the free energy of transfer scales linearly with the BSA. Calibrations based on their relative solubility in water and organic solvents yield a slope of 20–30 cal mol−1 Å−2 (Chothia, Reference Chothia1974; Vajda et al. Reference Vajda, Weng and DeLisi1995). Site-directed mutagenesis experiments discussed in Section 4.1.3 suggest that this correctly represents the contribution of the hydrophobic effect to protein–protein interaction, although the contribution of individual hydrophobic groups may depend on the structural context.
Polar interactions and hydration
Electrostatic interactions implicate polar groups containing oxygen or nitrogen atoms. These atoms bear a partial or a full charge, and they form H bonds, much stronger than van der Waals interactions. Electrostatics plays an essential role in all processes involving proteins, DNA or RNA, but its evaluation requires elaborate calculations that are highly sensitive to the solvent model (Sheinerman et al. Reference Sheinerman, Norel and Honig2000). The H bonds between protein groups, or between the protein and a ligand, have an energy similar to those made with water, and the balance depends on the details of their geometry and their environment. Program HBPLUS (McDonald & Thornton, Reference McDonald and Thornton1994) is commonly used to detect H bonds in protein structures; with a donor–acceptor distance cutoff of 3·9 Å, the default value in its implementation by the PP server of University College London, it retains H bonds that may be much weaker than that those between water molecules in the liquid, where the oxygen–oxygen distance is 2·8 Å. H bonding is mostly a dipole–dipole interaction, but electrostatics also involves long-range interactions between charged groups, and weaker interactions involving aromatic groups or water bridges. Although efficiently screened by salts under most conditions, long-range electrostatic interactions may play an active part in the kinetics of association (Schreiber et al. Reference Schreiber, Shaul and Gottschalk2006).
The representation of electrostatics in force fields is not accurate enough to give reliable values of the energy balance of these interactions in a water environment. In practice, all-atom force fields perform best if they are calibrated on actual protein structures. An example is the Rosetta force field, which has yielded excellent models of several proteins and protein–protein complexes (Bradley et al. Reference Bradley, Misura and Baker2005; Schueler-Furman et al. Reference Schueler-Furman, Wang, Bradley, Misura and Baker2005). Energy refinement with an all-atom force field is computationally expensive, albeit well suited for distributed computing (Das et al. Reference Das, Qian, Raman, Vernon, Thompson, Bradley, Khare, Tyka, Bhat, Chivian, Kim, Sheffler, Malmström, Wollacott, Wang, Andre and Baker2007). It is usually left for late steps of structure predictions, and earlier steps rely instead on knowledge-based statistical potentials, of which TASSER (Zhang et al. Reference Zhang, Arakaki and Skolnick2005) is an example. Knowledge-based potentials, originally developed to model protein folding, are now commonly used for protein design (Poole & Ranganathan, Reference Poole and Ranganathan2006). They can also be applied to protein interactions (Keskin et al. Reference Keskin, Bahar, Badretdinov, Ptitsyn and Jernigan1998), but the tendency in recent years has been to develop potentials that are specifically designed for that purpose (Mintseris et al. Reference Mintseris, Pierce, Wiehe, Anderson, Chen and Weng2007), and to complement the statistical approach with machine-learning procedures that can handle parameters not easily converted into potentials (Block et al. Reference Block, Paern, Hüllermeier, Sanschagrin, Sotriffer and Klebe2006; Zhu et al. Reference Zhu, Domingues, Sommer and Lengauer2006; Ofran & Rost, Reference Ofran and Rost2007; Bernauer et al. Reference Bernauer, Bahadur, Rodier, Janin and Poupon2008).
The interface between two proteins, or between a protein and a nucleic acid in a complex, is never completely dehydrated. This can be seen in high-resolution X-ray structures that report water molecules: many are located at molecular interfaces. Rodier et al. (Reference Rodier, Bahadur, Chakrabarti and Janin2005) consider as interface waters all crystallographic waters located within 4·5 Å of atoms of both subunits; nearly all are within H-bond distance of at least one protein polar atom. Their number increases with the interface size, but also with the resolution of the X-ray structure, which strongly suggests that solvent is under-reported in most studies.
Conformation changes
Conformation changes, which occur frequently when a protein interacts with a ligand, can be assessed in cases where X-ray or NMR structures are available for both the free and the liganded protein. The ligand can be a small molecule, a nucleic acid or another protein, and the change may affect just a few atoms in the region of contact, or the whole protein. Its amplitude can be estimated by performing a least-square superposition of the free and liganded protein, and measuring the root-mean-square displacement (RMSD) of the Cα atoms. As the RMSD tends to increase with the protein size, it may be useful to normalize it (Carugo & Pongor, Reference Carugo and Pongor2001), or use instead a comparison of the Cα–Cα distances in the free and liganded structures.
The RMSD ranges between 0·2 and 8 Å in a set of 124 protein–protein complexes for which the free component structures are available (Hwang et al. Reference Hwang, Pierce, Mintseris, Janin and Weng2008). Half of the complexes in that set have an RMSD <1 Å, comparable to what is obtained when two different X-ray structures of the same protein are superimposed. They display only side chain rotations and localized main chain movements, and can be assumed to form by rigid-body association. By contrast, the complexes with a large RMSD display movements of all sorts: of surface loops, of secondary structure elements and, in some cases, of whole domains. The conformation observed in complexes may preexist the interaction, or reflect an induced fit that follows the encounter of the two molecules (Grünberg et al. Reference Grünberg, Nilges and Leckner2006), but in either case, the conformation change is an essential element of the recognition process.
Conservation in evolution
Proteins with closely related sequences usually have the same QS, but this breaks down at a certain level of divergence. Hemoglobin is a tetramer in mammals, but not invertebrates, and sea lamprey, a vertebrate, has a monomeric hemoglobin with 31% sequence identity to human. This example shows that QS is much less conserved than the secondary or tertiary structure, which is the same in all globins. Lévy et al. (Reference Lévy, Erba, Robinson and Teichmann2008) find that, at a 30–40% sequence identity level, 30% of the proteins in PiQSi have a different QS, and that the QS changes in half of the homologs with less than 30% identity. Thus, homology does not reliably predict the QS at levels of identity where the fold is certainly maintained, and this may be why standard tools for homology modelling generally ignore the QS.
An earlier report by Aloy et al. (Reference Aloy, Ceulemans, Stark and Russell2003) finds that the interaction between domains is almost invariably conserved above 30% sequence identity. This finding is at the origin of the InterPreTS (Interaction Prediction through Tertiary Structure; Aloy & Russell, Reference Aloy and Russell2003) procedure. It uses a library of Pfam domains known to interact, called 3DID (Stein et al. Reference Stein, Russell and Aloy2005). If two proteins contain sequences related to a domain pair in 3DID, the sequences are aligned with the closest homologs, and the interaction modelled on that in the pair. InterPreTS was used to carry out structural predictions on 102 protein complexes in yeast, yielding at least partial models of half of the complexes (Aloy et al. Reference Aloy, Böttcher, Ceulemans, Leutwein, Mellwig, Fischer, Gavin, Bork, Superti-Furga, Serrano and Russell2004). At low levels of sequence identity, threading methods may complement homology in QS predictions. The M-TASSER procedure (Grimm et al. Reference Grimm, Zhang and Skolnick2006) was designed for predicting homodimers and, like InterPreTS, it uses a library of templates. A query sequence is threaded with TASSER (Zhang et al. Reference Zhang, Arakaki and Skolnick2005) to generate models of the monomers that are structurally aligned on the dimers in the library, and then refined. The structural alignment identified a correct template in about half of the sequences of a test set, and the refined models had an average RMSD of 5·9 Å relative to the native structures (Chen & Skolnick, Reference Chen and Skolnick2008).
The divergence of the QS during protein evolution may also be evaluated at the residue level. A conserved QS implies conserved interfaces, and therefore, a selection pressure on the interface residues. Given the aligned sequences of N homologous proteins, the variability at sequence position i is commonly measured by the Shannon entropy (Sander & Schneider, Reference Sander and Schneider1993; Elcock & McCammon, Reference Elcock and McCammon2001; Caffrey et al. Reference Caffrey, Somaroo, Hughes, Mintseris and Huang2004; Guharoy & Chakrabarti, Reference Guharoy and Chakrabarti2005):

p k is the fraction of the sequences that have in i a residue of type k; s(i)=0 at fully conserved positions, s(i)=lnT at totally divergent positions. The number T of types can be 20, or less if types with similar properties (e.g. hydrophobic) are merged (Elcock & McCammon, Reference Elcock and McCammon2001). The entropy depends on the choice of the aligned sequences, their number and their diversity, but it can be normalized to the average value in the polypeptide chain in order to limit that dependence.
Valdar & Thornton (Reference Valdar and Thornton2001) and Armon et al. (Reference Armon, Graur and Ben-Tal2001) use a similar approach with more elaborate measures of the evolutionary divergence that take into account observed mutation rates, in order to predict binding sites. The procedure of Valdar & Thornton (Reference Valdar and Thornton2001) is implemented in the Scorecons server of the European Bioinformatics Institute, and that of Armon et al. (Reference Armon, Graur and Ben-Tal2001) in the ConSurf server of Tel Aviv University. The Evolutionary Trace procedure (Lichtarge et al. Reference Lichtarge, Bourne and Cohen1996; Res & Lichtarge, Reference Res and Lichtarge2005; Mihalek et al. Reference Mihalek, Res and Lichtarge2006), also designed to predict binding sites, builds phylogenetic trees from sets of homologous sequences. In addition to residue conservation, pairwise interactions between proteins may be identified by searching for the co-evolution of their amino acid sequences, but the accuracy is poor unless the structure of a homologous complex is available (Pazos & Valencia, Reference Pazos and Valencia2002; Valencia & Pazos, Reference Valencia and Pazos2002; Mintseris & Weng, Reference Mintseris and Weng2005; Halperin et al. Reference Halperin, Wolfson and Nussinov2006; Juan et al. Reference Juan, Pazos and Valencia2008).
Docking predictions
Docking methods aim to model the structure of a complex from that of its components. Protein–protein docking procedures have often been reviewed (Halperin et al. Reference Halperin, Ma, Wolfson and Nussinov2002; Smith & Sternberg, Reference Smith and Sternberg2002; Marshall & Vakser, Reference Marshall, Vakser and Waksman2005; Gray, Reference Gray2006; Wiehe et al. Reference Wiehe, Peterson, Pierce, Mintseris and Weng2007). Several are implemented as Web servers (Table 1). Their performance can be tested on benchmark sets of protein–protein complexes (Gao et al. Reference Gao, Douguet, Tovchigrechko and Vakser2007; Hwang et al. Reference Hwang, Pierce, Mintseris, Janin and Weng2008). Since 2001, protein–protein docking is assessed by blind predictions in the CAPRI experiment (Critical Assessment of PRedicted Interactions; Janin et al. Reference Janin, Henrick, Moult, Eyck, Sternberg, Vajda, Vakser and Wodak2003; Janin, Reference Janin2005; Janin & Wodak, Reference Janin and Wodak2007). A CAPRI target is a protein–protein complex that has a known, but still unpublished, experimental structure, and known component structures. CAPRI predictors dock the components and submit models that are assessed against the experimental structure. In six years, 28 target complexes have been submitted to the prediction, which yielded good-quality models of a majority of them. Details of the methods and the results can be found in the evaluation papers (Mendez et al. Reference Méndez, Leplae, De Maria and Wodak2003, Reference Méndez, Leplae, Lensink and Wodak2005; Lensink et al. Reference Lensink, Méndez and Wodak2007), and the special issues of Proteins: Structure, Function and Bioinformatics in which they appear.
Most docking procedures perform an exploration step followed by a refinement step. The first step moves one component as a rigid body relative to the other. In the fast Fourier transform (FFT) correlation algorithm, the two protein structures are mapped onto a cubic grid and each grid point weighted to mark its position relative to the molecule. The correlation function of the weights associated to the two proteins is calculated by FFT for all translations of one protein relative to the other, and the calculation is repeated for all orientations. Procedures based on that algorithm are implemented in a number of servers: ClusPro (Comeau et al. Reference Comeau, Gatchell, Vajda and Camacho2004), SmoothDock, MultiDock (Gabb et al. Reference Gabb, Jackson and Sternberg1997), Gramm-X (Tovchigrechko & Vakser, Reference Tovchigrechko and Vakser2006). Other docking algorithms randomly generate starting positions, and apply heuristic methods to optimize them (May & Zacharias, Reference May and Zacharias2007). In RosettaDock (Gray et al. Reference Gray, Moughon, Wang, Schueler-Furman, Kuhlman, Rohl and Baker2003; Wang et al. Reference Wang, Schueler-Furman, Andre, London, Fleishman, Bradley, Qian and Baker2007), this is done in two successive steps of Monte Carlo optimization: one that uses a simplified protein model and force field, and the other, explicit atoms and the very successful Rosetta force field originally developed for protein folding. PatchDock (Schneidman-Duhovny et al. Reference Schneidman-Duhovny, Inbar, Nussinov and Wolfson2005a) implements an efficient computer vision algorithm in which the protein surfaces are divided into concave, convex and flat patches, and complementary patches are brought together.
The second step of a docking procedure aims to evaluate and rank the models issued from the exploration step; if near-native solutions are identified, they are subjected to further refinement. The scoring functions used for ranking models may include energy, geometric complementarity, propensities and other terms specific to each approach. When external information is available on the complex, for instance from an interface prediction, sequence conservation, or biological data on mutants, it can be used as a constraint during the exploration step, or be incorporated in the scoring function. The HADDOCK program (Dominguez et al. Reference Dominguez, Boelens and Bonvin2003; van Dijk et al. Reference van Dijk, de Vries, Dominguez, Chen, Zhou and Bonvin2005) handles external data, including NMR data if they exist, as restraints during energy minimization. Conformation changes can also be modelled at that stage. HADDOCK and RosettaDock do it by leaving free some main chain dihedral angles (Chaudhury et al. Reference Chaudhury, Sircar, Sivasubramanian, Berrondo and Gray2007; de Vries et al. Reference de Vries, van Dijk, Krzeminski, van Dijk, Thureau, Hsu, Wassenaar and Bonvin2007). Instead, other procedures generate a number of alternative conformations and perform ‘cross-docking’ on the conformers (Schneidman-Duhovny et al. Reference Schneidman-Duhovny, Inbar, Nussinov and Wolfson2005a; Grünberg et al. Reference Grünberg, Nilges and Leckner2006; Krol et al. Reference Krol, Chaleil, Tournier and Bates2007). In either case, flexibility greatly increases the size of the calculation and the number of false positives.
Docking known structures is an appropriate procedure for predicting non-obligate complexes, but this is usually not applicable to oligomeric proteins, so that very few have been CAPRI targets. A remarkable exception has been the envelope glycoprotein of a flavivirus, known to exist both as a dimer in the viral particle, and as a trimer in the form that promotes membrane fusion when the virus infects a cell (Bressanelli et al. Reference Bressanelli, Stiasny, Allison, Stura, Duquerroy, Lescar, Heinz and Rey2004). In CAPRI, the structure of the trimer had to be predicted from that of the dimer, a difficult task in which only one predictor succeeded. In other systems where an experimental structure of the components is lacking, docking may be performed on models generated in silico by homology or threading (Tovchigrechko et al. Reference Tovchigrechko, Wells and Vakser2002; Aloy et al. Reference Aloy, Böttcher, Ceulemans, Leutwein, Mellwig, Fischer, Gavin, Bork, Superti-Furga, Serrano and Russell2004, Reference Aloy, Pichaud and Russell2005; Zhang & Skolnick, Reference Zhang and Skolnick2004).
Assemblies with more than two components can be generated by adding one component at a time, in which case the constraints due to the occupied space are seen to severely constrain the search (Inbar et al. Reference Inbar, Benyamini, Nussinov and Wolfson2005). Thus, a structural model of the nuclear pore (Alber et al. Reference Alber, Dokudovskaya, Veenhoff, Zhang, Kipper, Devos, Suprapto, Karni-Schmidt, Williams, Chait, Sali and Rout2007) could be built by assigning a fold type to domains in the constituent proteins, modelling the domains and assembling them by optimizing a score function under a large number of restraints derived from experiment. The nuclear pore has a C 16 symmetry that played a major role in that operation. Symmetry has now been incorporated in several of the docking procedures originally designed to model binary complexes (Berchanski et al. Reference Berchanski, Segal and Eisenstein2005; Pierce et al. Reference Pierce, Tong and Weng2005; Schneidman-Duhovny et al. Reference Schneidman-Duhovny, Inbar, Nussinov and Wolfson2005a, Reference Schneidman-Duhovny, Inbar, Nussinov and Wolfsonb).
The structural basis of macromolecular recognition
A broad classification of the different modes of macromolecular recognition can be based on the time scale on which they occur. The QS of oligomeric proteins mostly represents a permanent association. Protein–protein complexes, which involve partners that have independent existence, are mostly transient, yet they cover a wide range of affinities and lifetimes. Thus, the complex between barnase, a bacterial ribonuclease, and its intracellular inhibitor barstar, has a K d≈10−14 m and a half-life of days (Schreiber & Fersht, Reference Schreiber and Fersht1993). In contrast, redox proteins form complexes with K d≈10−6 m and half-lives shorter than 1 s, well adapted to their role in electron transfer (Crowley & Carrondo, Reference Crowley and Carrondo2004). The protein–DNA and protein–RNA complexes considered in Section 4.5 cover a range at least as wide. Irrespective of their stability, all these interactions are biologically significant; they play major roles in essential processes of life, and therefore, they are subject to evolutionary selection. In the cell, they compete with all sorts of interactions that result from the random collision of cellular components. Crystal contacts may serve as a model of those: they have the same physical basis as other protein–protein interactions, but they are non-specific and do not undergo evolutionary selection.
Protein–protein complexes
Size and topology of the interfaces
Sets of non-obligate protein–protein complexes of known X-ray structure have been assembled by Janin & Chothia (Reference Janin and Chothia1990), Jones & Thornton (Reference Jones and Thornton1996), Lo Conte et al. (Reference Lo Conte, Chothia and Janin1999) and recently by Hwang et al. (Reference Hwang, Pierce, Mintseris, Janin and Weng2008). Table 2 reports average properties of the protein–protein interfaces in a set of 70 complexes adapted from Lo Conte et al. (Reference Lo Conte, Chothia and Janin1999) by Chakrabarti & Janin (Reference Chakrabarti and Janin2002). The table compares their interfaces to those of homodimers and to crystal contacts. The interface size measured by the BSA has a mean value of 1910 Å2. An average of 204 atoms contribute to that BSA; they belong to 57 amino acid residues, that is about 28 residues per component protein. When site-directed mutagenesis experiments are performed on some of these complexes, many of the residues that lose ASA can be changed to Ala without much effect on the dissociation constant; only a fraction, termed ‘hotspots’, yield large changes in K d. In the human growth hormone (HGH)/HGH receptor system for instance, 31 interface residues were mutated on the receptor, but only 11 mutants showed a significant loss of affinity for the hormone (Clackson & Wells, Reference Clackson and Wells1995). Similar results have been obtained in other systems (Bogan & Thorn, Reference Bogan and Thorn1998; DeLano, Reference DeLano2002). The discrepancy with the geometric definition of the interfaces is due in part to the limits of the alanine-scanning method: it does not test all the residues (Ala and Gly are almost always omitted), and ignores the interactions made by the main chain, which contributes 20% of the BSA and a majority of the interface H bonds (Lo Conte et al. Reference Lo Conte, Chothia and Janin1999). Nevertheless, the discrepancy is real, and relevant to the topology of the interfaces and the core/rim model discussed below.
Table 2. Properties of protein–protein interfaces

a Data from Chakrabarti & Janin (Reference Chakrabarti and Janin2002) on a subset of the complexes of Lo Conte et al. (Reference Lo Conte, Chothia and Janin1999).
b Data from Bahadur et al. (Reference Bahadur, Chakrabarti, Rodier and Janin2003) ; the set of Dey et al. (unpublished data) was derived from the PiQSi database (Lévy, Reference Lévy2007) as described in the text.
c Homodimers described in PiQSi (Lévy, Reference Lévy2007) as being in equilibrium with the monomer, based on the literature.
d Pairwise interfaces in crystals of monomeric proteins. The first mean BSA value and the S.D. are for the 1320 interfaces in the 152 crystal forms analyzed by Janin & Rodier (Reference Janin and Rodier1995). All other numbers are for 188 interfaces with BSA >800 Å2 that were selected among those 1320 interfaces by Bahadur et al. (Reference Bahadur, Chakrabarti, Rodier and Janin2004).
e Score obtained by summing over the whole interface the propensity of individual residues to occur at the interface of homodimers (Bahadur et al. Reference Bahadur, Chakrabarti, Rodier and Janin2004).
f Number of polypeptide segments per 1000 Å2 of BSA. A separate dataset of 204 structures has been used for protein–protein complexes (Pal et al. Reference Pal, Chakrabarti, Bahadur, Rodier and Janin2007).
g Data from Rodier et al. (Reference Rodier, Bahadur, Chakrabarti and Janin2005).
h Mean values in subsets that comprise 52 protein components of the complexes (excluding antigen–antibody complexes), 121 homodimers and 102 monomeric proteins in crystal contacts. s is the mean Shannon entropy in aligned sequences. Data from Guharoy & Chakrabarti (Reference Guharoy and Chakrabarti2005).
Lo Conte et al. (Reference Lo Conte, Chothia and Janin1999) noted that all the protein–protein complexes in their set bury more than 1100 Å2, and that a majority has a BSA in the range of 1200–2000 Å2. They retained that range as defining ‘standard-size’ protein–protein interfaces, as opposed to the ‘small’ interfaces with BSA <1200 Å2, and the ‘large’ interfaces with BSA >2000 Å2. An interface with a BSA of more than 1200 Å2 implicating about 60 atoms and 16 residues per component, allows the stable, specific association of two proteins. Nearly all antibody–antigen and many enzyme–inhibitor complexes have standard-size interfaces. The barnase–barstar complex mentioned above does, and also several protease–inhibitor complexes with K d well below 10−12m. Antigen–antibody complexes are not quite as stable: they typically have a nanomolar K d and half-lives of a few hours (Braden & Poljak, Reference Braden, Poljak and Kleanthous2000; Sundberg & Mariuzza, Reference Sundberg and Mariuzza2002).
The recent set of complexes assembled by Hwang et al. (Reference Hwang, Pierce, Mintseris, Janin and Weng2008) confirms and extends these results. The interface size distribution in that set is shown in Fig. 5. The mean BSA and its standard deviation are essentially the same as in Table 2; 7% of the interfaces are small, 55% are standard size and 38% are large. Three complexes in the new set have a BSA near 1000 Å2, and one buries only 810 Å2. It occurs in a complex of the Cbl-b ubiquitin ligase with ubiquitin (PDB entry 2oob). Ubiquitin binding promotes the dimerization of the Cbl-b ligase (Peschard et al. Reference Peschard, Kozlov, Lin, Mirza, Berghuis, Lipkowitz, Park and Gehring2007), which buries additional surface, but several other complexes involving ubiquitin also have a small BSA. They are short-lived like the redox complexes involved in electron transfer, also listed in Fig. 5. Their interfaces are of standard size or smaller (Crowley & Carrondo, Reference Crowley and Carrondo2004); the smallest, with a BSA of 830 Å2, occurs in a ternary assembly (PDB entry 2 mta). Taken together, the BSA data suggest that the minimum protein surface that must be buried to form a functional complex is of the order of 900 Å2 and implicates no more than 12 residues on each partner. The ubiquitin ligase/ubiquitin and the redox complexes illustrate biological processes that depend on short-lived interactions associated with a minimal size interface. Such processes are still poorly represented in the PDB in spite of their importance (Noreen & Thornton, Reference Noreen and Thornton2003b).

Fig. 5. Interface size in transient protein–protein complexes. Histogram of the BSA in the set of 124 protein–protein complexes assembled by Hwang et al. (Reference Hwang, Pierce, Mintseris, Janin and Weng2008), and the set of 11 redox protein complexes of Crowley & Carrondo (Reference Crowley and Carrondo2004). The Hwang set comprises 25 antigen–antibody complexes, 35 enzyme/inhibitor or substrate complexes and 64 complexes of other types. The mean value of the BSA is 1910 Å2 in that set, and 1290 Å2 in the redox set.
With few exceptions, the small and standard-size interfaces have a simple topology: they comprise a single pair of recognition patches as defined by the clustering algorithm of Chakrabarti & Janin (Reference Chakrabarti and Janin2002), and a single connected component in the Voronoi model of Cazals et al. (Reference Cazals, Proust, Bahadur and Janin2006). The antibody–antigen interface shown in Fig. 3 above is an example of those. Standard-size interfaces are also mostly planar, except in protease–inhibitor complexes where the inhibitor offers a convex surface to the concave active site of the protease (Lo Conte et al. Reference Lo Conte, Chothia and Janin1999). In contrast, the large interfaces usually consist of multiple pairs of recognition patches, at least one of them of standard size (Chakrabarti & Janin, Reference Chakrabarti and Janin2002). An example is the Gα/Gβγ interface of transducin (Fig. 4): the blue patch is standard size, and the red patch is small. Individual patches may be planar, but that is unlikely for the whole interface.
The proteins that carry more than one recognition patch on their surface often have the capacity to undergo conformational changes of large amplitude. In transducin, the red patch implicates the N-terminal segment of Gα. This segment is disordered in the free subunit, and forms an α helix in the complex (Lambright et al. Reference Lambright, Sondek, Bohm, Skiba, Hamm and Sigler1996). Thus, it undergoes a disorder-to-order transition during association. In other systems, the proteins may have domains, each bearing a recognition patch, that move one relative to the other when the complex forms. In the set assembled by Hwang et al. (Reference Hwang, Pierce, Mintseris, Janin and Weng2008), the structure of the free components is known along with that of the complex, and the amplitude of the conformation changes can be estimated by least-square superposition. Figure 6 indicates that the RMSD of the C-alpha atoms tends to increase with the BSA. It is smaller than 1·8 Å in all the complexes with small interfaces, and in 90% of those with standard-size interfaces. This confirms that rigid-body recognition is the preferred mechanism for assembling complexes with standard-size, single-patch interfaces. On the other hand, the RMSD is more than 1·8 Å for 40% of the complexes with large interfaces. Large multi-patch interfaces allow flexible recognition to occur, and we may assume that the additional buried surface and atomic interactions pay for the cost of the conformational change (Lo Conte et al. Reference Lo Conte, Chothia and Janin1999; Janin et al. Reference Janin, Rodier, Chakrabarti and Bahadur2007).

Fig. 6. Interface size and conformation changes. In the set of Hwang et al. (Reference Hwang, Pierce, Mintseris, Janin and Weng2008), the free components of the complexes have a known structure that can be compared to their structure in the complex by rigid-body least-square superposition. The residual RMSD of the C-alpha atoms is plotted against the BSA of each complex. The dotted lines are drawn at RMSD=1·8 Å and BSA=2000 Å2. The categories are the same as in Fig. 5.
Composition, packing and hydration
The interfaces of non-obligate complexes have been examined from the viewpoint of their chemical composition. Table 2 shows that the fractional contribution of non-polar (carbon-containing) groups to the BSA is f np=58% on average. This value is almost the same as for the ASA of the average monomeric protein (f np=57%; Miller et al. Reference Miller, Janin, Lesk and Chothia1987), which reflects the fact that the protein surface involved in an interface remains solvent accessible until the components of the complex meet. Thus, it cannot have very different physical–chemical properties from the remainder of the protein surface. The interfaces of antigen–antibody complexes (f np=51%) tend to be more polar than the average accessible surface, and those of protease–inhibitor complexes (f np=61%) are less polar (Lo Conte et al. Reference Lo Conte, Chothia and Janin1999). The interfaces comprise fewer charged groups, and therefore, more neutral polar groups, than the protein surface; the charged groups contribute 14% of the BSA in Table 2 instead of 19% to the ASA (Miller et al. Reference Miller, Janin, Lesk and Chothia1987). The polar fraction also governs the capacity of interface atoms to form H bonds. On average, transient complex interfaces contain 10 H bonds, about 1 per 190 Å2 of BSA (Table 2), defined with the geometric criteria of HBPLUS (McDonald & Thornton, Reference McDonald and Thornton1994). The number of H bonds, n HB, in individual interfaces increases with the BSA, but the correlation is mediocre (R 2=0·84). Moreover, high-resolution X-ray structures tend to display more H bonds, which suggests that the surface density calculated on the whole sample is underestimated.
A large majority of the atoms at protein–protein interfaces is still accessible to the solvent in the complex. The fraction of fully buried interface atoms (zero ASA), f bu, is only 34% on average (Table 2), and it never exceeds 50%. Measurements of the Voronoi volume indicate that the buried interface atoms pack as densely as the atoms of the protein core. This implies that the interfaces are close-packed, a statement that can be extended to 60% of the interface atoms by including the crystallographic water molecules in the construction of the Voronoi diagram. In most cases, the volumes are within 1% of those of the protein core (Lo Conte et al. Reference Lo Conte, Chothia and Janin1999).
Residual water plays an important role in the packing. It is abundant at the interfaces: they contain an average of 20 water molecules, that is 10 water molecules per 1000 Å2 of BSA (Table 2). This amounts to about 10% of the hydration water present before association, a water molecule covering about 10 Å2 of the protein surface on average. This estimate of the residual hydration of the interfaces is certainly below the reality, because X-ray structures do not report solvent in a consistent way, and the surface density of interface water increases with the resolution even faster than for H bonds (Rodier et al. Reference Rodier, Bahadur, Chakrabarti and Janin2005). Water molecules make many polar interactions and H bond bridges across the interface. The balls representing water in Figs 3 and 4 illustrate two different patterns of interface hydration that can be described as ‘wet’ and ‘dry’ (Janin, Reference Janin1999). The antigen–antibody interface (Fig. 3) is wet, that is it is hydrated all through its surface. The Gα/Gβγ transducin interface (Fig. 4) is split into two distinct patches, both dry: many water molecules line their edges, but few if any penetrate inside.
Although the interface residues of a non-obligate complex are surface residues in the free components, there are some noticeable features in their amino acid composition. Relative to the accessible protein surface, the interfaces are depleted in Glu, Asp and Lys, and enriched in Met, Tyr and Trp. The propensities, shown in Fig. 7 c together with those of protein–nucleic acid complexes discussed in Section 4.5, are generally weak, but they become more apparent once the interfaces are dissected into a core and a rim as in Fig. 7 a. The rim, made of residues in which none of the interface atoms are fully buried, has a composition close to the protein accessible surface. The core comprises all the buried interface atoms, and 55% of all interface residues on average. It is enriched in aromatic residues, and to a lesser extent, in aliphatic residues, but not in Val, Ala and Pro. Val is indifferent, whereas Ala and Pro have a negative propensity for the interface core. Albeit aliphatic, the side chains of these residues are more constrained than in Ile, Leu, Met or the aromatic residues, and it may be that the flexibility of the longer side chains makes them more amenable to the needs of the interface formation. Arginine, far from being disallowed like the other charged residues, contributes 10% of the BSA in both the core and the rim, and also 10% of the ASA (Chakrabarti & Janin, Reference Chakrabarti and Janin2002).

Fig. 7. Residue propensities for interfaces vs. the protein surface. The propensities [Eq. (6)] are derived from the relative contributions of the 20 amino acid types to the BSA of the interfaces and the ASA of the proteins in the same set. (a) Core and rim of the interfaces of protein–protein complexes. Data from Chakrabarti & Janin (Reference Chakrabarti and Janin2002). (b) The core of the homodimers interfaces is compared to crystal packing interfaces. Data from Bahadur et al. (Reference Bahadur, Chakrabarti, Rodier and Janin2004). (c) The interfaces of protein–DNA (Nadassy et al. Reference Nadassy, Wodak and Janin1999) and protein–RNA complexes (Bahadur et al. Reference Bahadur, Zacharias and Janin2008) are compared to those of protein–protein complexes (Chakrabarti & Janin, Reference Chakrabarti and Janin2002).
The core/rim model of protein–protein recognition
Interface residues make inter-molecular interactions. They are structurally and possibly functionally important, and therefore subject to a selection pressure that should be detected in homologous sequences. Several methods for predicting protein–protein contacts rely on identifying patches of surface residues conserved in evolution (Lichtarge et al. Reference Lichtarge, Bourne and Cohen1996; Armon et al. Reference Armon, Graur and Ben-Tal2001; Valdar & Thornton, Reference Valdar and Thornton2001; Lichtarge & Sowa, Reference Lichtarge and Sowa2002; Ma et al. Reference Ma, Elkayam, Wolfson and Nussinov2003). The conservation signal is usually weak, but like the amino acid composition signal, it can be enhanced by splitting the interfaces into core and rim regions. Guharoy & Chakrabarti (Reference Guharoy and Chakrabarti2005) estimated the effect of evolutionary selection by measuring Shannon entropies in sets of aligned sequences. In protein–protein complexes, the mean value of the normalized entropy of the interface residues was s=0·65 in the core and s=0·80 in the rim (Table 2). Figure 8 shows how the core and the rim of an interface are distributed on the surface of two proteins, and compares that distribution with the sequence entropy. The proteins are the inhibitor component of an enzyme–inhibitor complex, and the subunit of a homodimer.
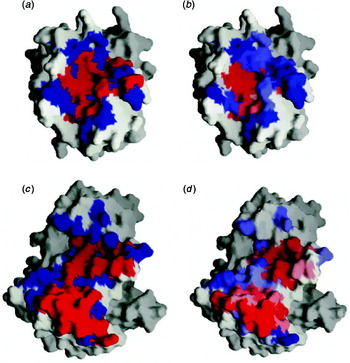
Fig. 8. The core/rim model of protein–protein interfaces. Top row: The protease component of the elastase–ovomucoid inhibitor complex (1ppf; Bode et al. Reference Bode, Wei, Huber, Meyer, Travis and Neumann1986). Bottom row: The subunit of the malate dehydrogenase homodimer (1bmd; Kelly et al. Reference Kelly, Nishiyama, Ohnishi, Beppu and Birktoft1993). (a, c) The core (red) comprises the residues that contain interface atoms with zero ASA, the rim (blue), the residues where all the interface atoms are accessible (Chakrabarti & Janin, Reference Chakrabarti and Janin2002). (b, d) Shannon entropies of interface residues in aligned sequences [Eq. (7)]; red stands for low entropies (maximum conservation); blue denotes high entropies (maximum divergence). Figure made with GRASP (Nicholls et al. Reference Nicholls, Sharp and Honig1991), courtesy of M. Guharoy (Calcutta, India).
The picture of the interface conservation derived from the Shannon entropy is coherent with the results of site-directed mutagenesis. Clackson & Wells (Reference Clackson and Wells1995) noted that, in the HGH/HGH receptor system, the residues of the receptor that show large changes of affinity upon mutation to Ala are grouped at the center of the interface. In general, hotspot residues tend to cluster and be sheltered from the solvent (Bogan & Thorn, Reference Bogan and Thorn1998; Halperin et al. Reference Halperin, Wolfson and Nussinov2004). Guharoy & Chakrabarti (Reference Guharoy and Chakrabarti2005) observed that in 14 complexes, there is a correlation between the contribution of the interface residues to the BSA and ΔΔG d, the change in the free energy of dissociation of the complex upon their mutation to Ala. The correlation is significant for the interface core, but not the rim, and it yields a slope of 26–38 cal mol−1 Å−2, consistent with the estimates of Chothia (Reference Chothia1974) and Vajda et al. (Reference Vajda, Weng and DeLisi1995) based on the solubility of hydrocarbons. Sundberg et al. (Reference Sundberg, Urrutia, Braden, Isern, Tsuchiya, Fields, Malchiodi, Tormo, Schwarz and Mariuzza2000) and Li et al. (Reference Li, Huang, Swaminathan, Smith-Gill and Mariuzza2005) introduced a series of different size side chains at two sites of the interface of antibody–lysozyme complexes: a site at the center of the interface, the other at its periphery. In both series, ΔΔG d is proportional to the change in the BSA, but the slope is twice as large at the central site than at the peripheral site (46 vs. 21 cal mol−1 Å−2).
Other features of the interfaces support the core/rim distinction, for instance the ‘residue depth’ of Chakravarty & Varadarajan (Reference Chakravarty and Varadarajan1999), who find it to be correlated to ΔΔG d values, or the distribution of the conserved ‘dry residues’ of Mihalek et al. (Reference Mihalek, Res and Lichtarge2007). Taken together, the site-directed mutagenesis and the sequence conservation data suggest that, in a complex, protein–protein recognition exerts most of its selection pressure on the interface core. The data fit an ‘O ring’ model in which the interface has a core of buried atoms and (partly) buried residues, surrounded by a rim of residues whose atoms remain solvent accessible (Bogan & Thorn, Reference Bogan and Thorn1998; Lo Conte et al. Reference Lo Conte, Chothia and Janin1999). The model applies as such to a single patch standard-size interface, for instance the antigen–antibody interface of Fig. 3, which is the one that was mutated by Sundberg et al. (Reference Sundberg, Urrutia, Braden, Isern, Tsuchiya, Fields, Malchiodi, Tormo, Schwarz and Mariuzza2000), or the enzyme–inhibitor interface of Fig. 8 a. In a multi-patch interface like that of transducin (Fig. 4), or the HGH/HGH receptor complex where the receptor is a homodimer and bears one recognition patch per subunit, each patch makes its own O ring.
To conclude, we wish to stress that the core/rim model, and the mutant data themselves, should not be interpreted to say that the rim plays no part in the interaction. The model and the data only state that the rim residues contribute less to the free energy of dissociation than the core residues, or that their contribution does not depend heavily on the nature of their side chain.
Oligomeric proteins
Interface size and stability in homodimers
Subunit interfaces have been analyzed in several sets of oligomeric proteins that comprise tetramers, hexamers and larger assemblies (Argos, Reference Argos1988; Janin et al. Reference Janin, Miller and Chothia1988; Jones & Thornton, Reference Jones and Thornton1995, Reference Jones, Thornton and Kleanthous2000; Ponstingl et al. Reference Ponstingl, Kabir, Gorse and Thornton2005), but most of the available data concern homodimers, and we will limit our analysis to that category. Table 2 lists the mean values of the interface parameters in three separate sets of homodimers. That of Bahadur et al. (Reference Bahadur, Chakrabarti, Rodier and Janin2003) was manually assembled and comprises 122 proteins. A second set more than twice that size, and a third set of 19 ‘weak’ dimers were recently compiled by our group (Dey et al. unpublished data) with the help of an early version of the PiQSi database (Lévy, Reference Lévy2007); they are non-redundant at the 35% sequence identity level, and the QS of the proteins in them was confirmed by checking the literature. The weak dimers are homodimers reported to be in equilibrium with the monomers in the publications cited in PiQSi. Some of the homodimers in the first two sets may also be weak in that sense, but the scanned publications did not say. Noreen & Thornton (Reference Noreen and Thornton2003a, Reference Noreen and Thorntonb) also assembled a set of weak dimers, but due to different criteria of choice, the present list shares only two entries with theirs.
The most obvious feature that distinguishes the homodimer interfaces in Table 2 is their large BSA, which on average is twice that of the non-obligate complexes. As the number of interface atoms and interface residues increases linearly with the BSA, the factor of 2 also applies to atoms and residues. However, the average BSA is of little significance given the very large standard deviation. Figure 9 shows that individual values spread from less than 1000 Å2 to more than 6000 Å2; the largest in the set is 14 300 Å2. The BSA distribution is essentially the same in the Bahadur set and the larger new set, but it is very different in the weak dimer set. The mean BSA of the weak dimers is 1620 Å2, consistent with the data of Noreen & Thornton (Reference Noreen and Thornton2003b), who report an average of 740 Å2 for the buried surface area per subunit (that is, BSA/2) in their set of homodimers in equilibrium with monomers. In Fig. 9, most of the interfaces with BSA <1000 Å2 belong to the weak dimers. The smallest, with a BSA near 750 Å2, occur in cytochrome c6 (1c6o) and ferredoxin (1sj1), two proteins that may not be actual homodimers in the cell. The functional state of cytochrome c6 is likely to be a heterodimer (Schnackenberg et al. Reference Schnackenberg, Than, Mann, Wiegand, Huber and Reuter1999); that of ferredoxin is unknown.

Fig. 9. Interface size in homodimers. Histogram of the BSA in the three sets of homodimers reported in Table 2; the weak dimers are in equilibrium with the monomers.
Chemical and amino acid composition
Non-polar (carbon-containing) groups contribute f np=65% of the BSA of homodimer interfaces, significantly more than to the accessible protein surface (f np=57%), or to the interfaces in complexes; the weak dimer interfaces (f np=62%) are in between. The hydrophobic character of the homodimer interfaces is associated in Table 2 with a lesser contribution of the neutral polar groups, but not of the charged groups, to the BSA. Nevertheless, homodimer interfaces do not, in general, form large hydrophobic patches (Larsen et al. Reference Larsen, Olson and Goodsell1998), and they contain many H bonds and water molecules. Table 2 reports an average of 18–19 H bonds and 44 water molecules per interface, which makes the surface density of the H bonds and the water molecules in homodimer interfaces similar to that of the complexes. The data also suggest that the H bond density is less in the weak dimers, but the statistics are poor because of the small size of that set.
The amino acid composition of the homodimer interfaces has often been analyzed (Jones & Thornton, Reference Jones and Thornton1995, Reference Jones and Thornton1996; Tsai et al. Reference Tsai, Lin, Wolfson and Nussinov1997; Bahadur et al. Reference Bahadur, Chakrabarti, Rodier and Janin2003, Reference Bahadur, Chakrabarti, Rodier and Janin2004). It follows the same trends as the atomic composition: the interfaces are enriched in aliphatic and aromatic residues, on average by a factor of about 2, and depleted by the same factor in charged residues other than Arg. When the relative contributions of the residue types to the BSA and the ASA are expressed as propensities, the observation made above for the complexes remains valid: the propensities are much more marked for the residues of the interface core, which contain the buried interface atoms, than the interface rim. Figure 7 b reports the propensities to be at the interface core in the Bahadur set. A comparison with Fig. 7 a shows that they generally resemble those in complexes, but the aliphatic residues Ala, Val and Leu are more abundant. Leu and Arg are the largest contributors to the cores of homodimer interfaces, each with 10–11% of the BSA. In the complexes, Tyr and Arg both contribute 10% of the core BSA; Leu is third, but with only 6% (Chakrabarti & Janin, Reference Chakrabarti and Janin2002; Bahadur et al. Reference Bahadur, Chakrabarti, Rodier and Janin2003).
The propensity of an atom or a residue to be at an interface vs. the protein surface may be viewed as one-body statistical potential. The R p score of Bahadur et al. (Reference Bahadur, Chakrabarti, Rodier and Janin2004), derived simply by summing the propensities over all the residues of an interface, has a positive and large mean value in homodimers (Table 2). It is also positive, but much lower, in the complexes and the weak dimers, in line with their relative stabilities. Two-body potentials can be derived statistically by counting pairwise contacts across the interfaces (Moont et al. Reference Moont, Gabb and Sternberg1999; Glaser et al. Reference Glaser, Steinberg, Vakser and Ben-Tal2001; Ofran & Rost, Reference Ofran and Rost2003; Saha et al. Reference Saha, Bahadur and Chakrabarti2005). When residue–residue contacts are analyzed in different types of protein–protein interfaces, the homodimers show a peculiar feature: pairs containing identical residues are much more frequent than expected on a random basis. This results from contacts made by twofold-related residues along the symmetry axis, which make up 12% of the BSA. After correcting for the relative abundance of each residue type, Leu comes to be by far the largest contributor to the pairwise contacts (13%), followed by Phe, Val and Arg (Saha et al. Reference Saha, Bahadur and Chakrabarti2005). The leucine zippers are a well-known example of how Leu/Leu contacts may contribute to the stability of a homodimer.
Atomic packing and sequence conservation
The oligomeric proteins are generally known to assemble while the subunits fold, and their interfaces can be expected to be close-packed like the subunits' interior. Ponstingl et al. (Reference Ponstingl, Kabir, Gorse and Thornton2005) observe that the Voronoi volumes of the interface atoms of oligomeric proteins are within a few percent of the reference volumes of Tsai et al. (Reference Tsai, Taylor, Chothia and Gerstein1999), but this applies only to the buried atoms, which are a minority (f bu=35–36% in Table 2). Buried atoms are even fewer at the interfaces of weak dimers (f bu=28%). Table 2 lists two other parameters related to the atomic packing: the L D packing index of Bahadur et al. (Reference Bahadur, Chakrabarti, Rodier and Janin2004) and the S c shape complementarity score of Lawrence & Colman (Reference Lawrence and Colman1993). Both take very similar mean values in homodimers and in complexes, which supports the idea that all these interfaces are close-packed. On the other hand, the weak dimer interfaces have low values of f bu and of L D, and they may be poorly packed.
Guharoy & Chakrabarti (Reference Guharoy and Chakrabarti2005) analyzed the conservation of the interface residues in homodimers, and obtained a result very similar to what they had seen in complexes (Table 2): the mean Shannon entropy of interface residues is significantly less than the average in the whole polypeptide chain, and it is lower for the residues of the interface core than the rim. In that study, the mean number of residues in the core was the same in both types of interfaces, and the core represented a majority of the residues. This suggests that the core/rim model of interfaces is also valid for homodimers, but the correlation with hotspots is difficult to establish in their cases. Most are permanent assemblies much less amenable to a quantitative study than a non-obligate complex. The monomers are in general not observed, and K d values cannot be measured. The measurement can, in principle, be done on the weak dimers, but mutagenesis data are comparatively scarce on those.
Non-specific interactions in crystals
Size and composition of crystal packing interfaces
Protein crystals are held by the same forces that stabilize other macromolecular assemblies, and one may compare the interfaces between pairs of molecules in crystals to those of the complexes and oligomeric proteins (Janin & Rodier, Reference Janin and Rodier1995; Carugo & Argos, Reference Carugo and Argos1997; Dasgupta et al. Reference Dasgupta, Iyer, Bryant, Lawrence and Bell1997). A feature then strikes the eye: their small size. In the set of 1320 pairwise interfaces assembled by Janin & Rodier (Reference Janin and Rodier1995), the mean BSA is 570 Å2. Crystal packing nevertheless buries much protein surface, because each molecule has 8–10 neighbors, and it makes pairwise interfaces with all. The average crystal packing interface represents only one-third of that of a complex, and one-sixth of that of a homodimer. The great majority buries less than 800 Å2 and implicates fewer than 13 residues per protein molecule. It is often assumed that such interfaces cannot be biologically meaningful, but there are some larger ones in crystals. The BSA values approximate an extreme value distribution, in which large values are much more frequent than in a Gaussian distribution. Moreover, the interfaces with twofold symmetry tend to be larger than those created by other types of crystal symmetry operations (Janin, Reference Janin1997). About 10% of the crystal packing interfaces are similar in size to those of the complexes, and a majority of those have twofold symmetry. They form ‘crystal dimers’, which are artifacts of crystallization and do not exist in solution, yet they may be difficult to distinguish from biological homodimers on the basis of the crystal structure alone.
Bahadur et al. (Reference Bahadur, Chakrabarti, Rodier and Janin2004) selected 188 large crystal packing interfaces by keeping only those with a BSA >800 Å2 in the set of Janin & Rodier (Reference Janin and Rodier1995). They have an average BSA of 1510 Å2, comparable to standard-size interfaces in complexes, and they comprise about the same average number of atoms and of residues (Table 2). Their chemical composition is also similar to that of the biologically significant interfaces, except for a slight excess of charged groups. Their amino acid composition is reported in Fig. 7 b in the form of propensities that are all small (<0·5) in absolute value, but follow the same pattern as homodimers and complexes: there is a mild depletion in Lys, and a mild excess of aliphatic and aromatic residues. The R p score, which is the sum of the propensities of individual interface residues to be at a homodimer interface and has a positive mean value in complexes, is negative on average at crystal contacts (Table 2).
Crystal packing interfaces have a comparatively low average density of H bonds: 1 per 280 Å2 of BSA, instead of 1 per≈200 Å2 in complexes and homodimers (Table 2). This may be related to their low fraction of buried atoms: f bu=21% vs. 34–36%; and their high level of hydration: 15 waters per 1000 Å2 of interface area vs. 10–11 in homodimers and complexes. Their topology is another cause for these differences. A standard-size interface in a complex is in general single-patch and compact, and a crystal packing interface of the same size is almost always fragmented. This can be seen in Fig. 10, which compares the interfaces of a biological homodimer (κ-bungarotoxin, PDB entry 1kba) and a crystal dimer (pokeweed antiviral protein, PDB entry 1qci). The two are of similar size, but the second is poorly packed; it buries very few atoms and its L D index is low. In Table 2, the fragmentation of crystal packing interfaces shows in the low mean value of L D and in the number of polypeptide segments, which is greater on average at a crystal packing interface than at an interface of the same size in a complex; homodimer interfaces implicate an even smaller number of segments in proportion of their size.

Fig. 10. The atomic packing of a specific and a non-specific interface. The κ-bungarotoxin homodimer (1kba) and the pokeweed antiviral protein crystal dimer (1qci) form interfaces of a similar size, with a BSA of almost 1000 Å2, but only the first is biologically significant. Each subunit of either dimer contains about 50 interface atoms, drawn here as spheres in the plane of the twofold axis. Their packing is very different, as are the values of the fraction of buried atoms (f bu=28% vs. 7%) and the L D index (31 vs. 13).
Biological assemblies vs. crystal artifacts
These differences may be used to identify crystal and biological dimers in PDB entries. The interface size alone correctly distinguishes homodimers from monomers in 85% of the cases (Ponstingl et al. Reference Ponstingl, Henrick and Thornton2000). Additional information can be drawn from the literature, residue conservation or physical–chemical properties of the interfaces as discussed above (Section 2.3). In the sample of Bahadur et al. (Reference Bahadur, Chakrabarti, Rodier and Janin2004), where all the crystal packing interfaces have a BSA >800 Å2, size is not a powerful discriminator, but it can be combined with three parameters that tend to take higher values in biological homodimers than crystal dimers: the fractions of non-polar groups (f np) and buried atoms (f bu), and the number of H bonds (n HB). Thus, an interface has an 88% probability of belonging to a biological homodimer if it satisfies one of the two conditions:


and it has the same probability to be due to the crystal packing if neither condition is met (Janin et al. Reference Janin, Rodier, Chakrabarti and Bahadur2007).
The molecular contacts in crystals are unspecific and not biologically meaningful, with a few interesting exceptions (Janin, Reference Janin1997). Unlike specific interfaces, they should exert no evolutionary pressure on the regions of the protein surface implicated, an assumption supported by a comparison of aligned sequences. Table 2 reports the normalized Shannon entropy of the residues in the large crystal packing interfaces. Its mean value is s≈1, meaning that they evolve at the same rate as the average residue in the polypeptide chain to which they belong. Moreover, the core and the rim have nearly identical s, the core and rim residues being defined here by the buried atoms as in the other types of interfaces even though many crystal packing interfaces do not have a proper core, because they bury few atoms and are highly fragmented. In addition, proteins often come in several crystal forms that use different contacts. Pancreatic ribonuclease, for instance, crystallizes in at least six forms. Several contain the same large interface that forms a dimer in solution under the conditions that lead to crystallization, but the other crystal packing contacts essentially implicate the whole protein surface (Crosio et al. Reference Crosio, Janin and Jullien1992).
Icosahedral virus capsids
Symmetry
Icosahedral virus capsids are multi-subunit assemblies, a category that constitutes most of the cellular machines, but is poorly represented in the PDB at present (Dutta & Berman, Reference Dutta and Berman2005). The capsids encapsulate and protect the viral genome, and they are frequently implicated in the recognition and infection of target cells. They are very large objects with molecular weights of millions, and a symmetry that greatly helps in their study, so that atomic structures could be solved three decades ago for tomato bushy stunt virus (Harrison et al. Reference Harrison, Olson, Schutt, Winkler and Bricogne1978) and satellite tobacco necrosis virus (Liljas et al. Reference Liljas, Unge, Jones, Fridborg, Lövgren, Skoglund and Strandberg1982). Here, we can compare the subunit interfaces in capsids to those found in binary assemblies in terms of their structural and physical chemical properties, and discuss the process of capsid self-assembly.
The virus capsids were considered spherical until Crick & Watson (Reference Crick and Watson1956) proposed that they have the symmetry of the cubic I (icosahedral) point group. This was demonstrated by the electron microscopy studies of Caspar & Klug (Reference Caspar and Klug1962), who introduced the rule of quasi equivalence and the lattice triangulation number T. Quasi equivalence allows the icosahedral asymmetric unit (IAU) to contain T subunits, and the whole capsid, 60T subunits instead of the 60 implied by the point group. The subunits in the IAU are related by inexact or ‘quasi’ symmetries. They have identical sequences, but different conformations, and they make different contacts with their neighbors (Rossmann & Johnson, Reference Rossmann and Johnson1989). X-ray structures are available for a number of capsids with the T=1 lattice exemplified by satellite tobacco necrosis virus, the T=3 lattice exemplified by tomato bushy stunt virus, and also the pT3 (pseudo T=3) lattice exemplified by rhinovirus (Rossmann et al. Reference Rossmann, Arnold, Erickson, Frankenberger, Griffith, Hecht, Johnson, Kamer, Luo, Mosser, Rueckert, Sherry and Vriend1985). The pT3 capsids resemble the T=3 capsids, but the subunits in their IAU have different sequences. The PDB also reports the X-ray structures of a few capsids with larger T numbers, or with lattices that do not follow the rule of quasi equivalence, for instance the very large and complex capsids of Bluetongue virus (Grimes et al. Reference Grimes, Burroughs, Gouet, Diprose, Malby, Ziéntara, Mertens and Stuart1998) and phage PhiX174 (Dokland et al. Reference Dokland, Bernal, Burch, Pletnev, Fane and Rossmann1999). The VIPER database of the Scripps Research Institute (Shepherd et al. Reference Shepherd, Borelli, Lander, Natarajan, Siddavanahalli, Bajaj, Johnson, Brooks and Reddy2006) offers a particularly convenient access to some 250 virus structures obtained by X-ray and electron microscopy.
Subunit interfaces in capsids
Bahadur et al. (Reference Bahadur, Rodier and Janin2007) have assembled a non-redundant dataset of 49 viral capsids that includes 11 with lattice T=1, 17 with lattice T=3, 10 with lattice pT3 and 11 others. In each capsid, a unique set of interfaces between pairs of polypeptide chains can be identified. Icosahedral symmetries repeat these unique interfaces 60 times, except those with twofold (I 2) symmetry, which are repeated 30 times. There is an average of 16 unique interfaces per capsid; one-third occurs within the IAU, another third has I 2, I 3 or I 5 symmetry, the remainder, a quasi-symmetry.
The average pairwise interface in that set has a BSA of 1750 Å2, similar to transient complexes, but the range of sizes is large. Bahadur et al. (Reference Bahadur, Rodier and Janin2007) split the pairwise interfaces into three size categories. The small interfaces (BSA <800 Å2), which make up 40% of the sample, contribute only 7% of the BSA. The remainder belongs to medium-size (800–2000 Å2) and large interfaces (more than 2000 Å2). The T=1 capsids contain in general three unique interfaces with a BSA >800 Å2, one of each of the I 2, I 3 and I 5 symmetry types; T=3 capsids contain 5–9, including 3 between the chains in the IAU; pT3 capsids contain 6–16.
In a virus capsid, each subunit has many neighbors and buries a large fraction of its surface in contacts with them. The average number of neighbors is 7 in T=1 and T=3 capsids. In these capsids, the subunit contacts implicate about 60% of all residues and bury about 45% of the subunit ASA. In pT3 capsids, the number of neighbors increases to 12; the fraction of the residues that are at interfaces, to 73%; and the fraction of the ASA that is lost, to 60%. The complexity of the capsid assembly and the multiplicity of interfaces of all sizes are illustrated in Fig. 11 for rhinovirus (PDB entry 1 aym; Hadfield et al. Reference Hadfield, Lee, Zhao, Oliveira, Minor, Rueckert and Rossmann1997). Its pT3 capsid contains four different polypeptide chains, one of which (chain A in gray) forms homopentamers about the icosahedral fivefold axes, whereas chains B (blue) and C (cyan) form heterohexamers about the threefold axes. Chain A covers much of the outer surface of the capsid, yet the bottom part of Fig. 11 shows that in two opposite orientations, most of its surface is involved in one or several of the 13 different interfaces it makes with its neighbors.

Fig. 11. Capsid assembly and subunit interfaces in rhinovirus. Rhinovirus (1aym; Hadfield et al. Reference Hadfield, Lee, Zhao, Oliveira, Minor, Rueckert and Rossmann1997) has a pT3 capsid with four polypeptide chains in the IAU. Top: The capsid and its icosahedral lattice; chain A in gray forms pentamers about the fivefold axes; chain B in blue and chain C in cyan form heterohexamers about the threefold axes. Bottom: The molecular surface of chain A is drawn in two orientations 180° apart in the plane of the I 5 axis (dashed line), and colored according the subunit contacts. Chain B is drawn as a gray tube, chain C as a black tube. In the capsid, chain A has 13 neighbors with which it makes five large, four medium-size and four small interfaces. The large interfaces are with chain B (blue surface) and chain C (green), two A chains in the A pentamer (pink and brown) and chain C′, threefold related to C (cyan). The medium size interfaces involve the small chain D (yellow) and symmetry-related chains B′, B″ and C″. Chain A5″, related to A by a 144° rotation about I 5, makes a small interface (red) that implicates only one residue of A. Figure adapted from Bahadur et al. (Reference Bahadur, Rodier and Janin2007).
An important feature of the capsid assembly cannot occur in binary complexes and homodimers: the pairwise interfaces between subunits overlap, and many atoms or residues are part of more than one. On average, 15% of the interface atoms and 24% of the interface residues are in contact with two neighboring subunits, 2% of the atoms and 5% of the residues with three, and there are extreme cases where a residue is in contact with seven subunits (Bahadur et al. Reference Bahadur, Rodier and Janin2007).
Composition and topology
Capsid interfaces resemble homodimer interfaces in their non-polar character (f np=63%), irrespective of their size (Bahadur et al. Reference Bahadur, Rodier and Janin2007). The fraction of buried atoms (f bu) is only 29% in the pairwise interfaces, but it increases to 36% in the whole assembly. The difference comes from atoms in contact with more than one neighboring subunit; they can have a non-zero ASA in each of the pairwise interfaces, and a zero ASA in the capsid. The large capsid interfaces have f bu and L D packing indexes similar to those of homodimers, which implies that they are well packed. In contrast, the interfaces with BSA <2000 Å2 bury very few atoms (f bu=11–23%), and their L D index is low. Like crystal packing contacts, they may be loosely packed.
The interface core, which contains the buried atoms, represents half of the residues in pairwise interfaces, and two-thirds in the whole capsid. The high proportion of core residues affects the amino acid composition of the capsid interfaces. As in homodimers and complexes, the core is enriched in aliphatic residues and depleted in Asp, Glu and Lys, but not Arg. On the other hand, the capsid interfaces contain fewer aromatic and more neutral polar residues: Ser, Thr, Asn, Gln and Pro contribute 32% of the BSA in capsids vs. only 21% in homodimers.
Capsid interfaces contain an average of 7 H bonds. The surface density of 1 H bond per 250 Å2 of BSA is less than for the homodimers in Table 2, but the difference is probably an artifact of the comparatively low resolution of many of the viral X-ray structures: the interface H bond density is 1 per 200 Å2 in the 17 capsids structures with 2·8-Å or better resolution.
The topology of capsid interfaces was analyzed with the clustering method of Chakrabarti & Janin (Reference Chakrabarti and Janin2002). Most of the small interfaces are single-patch, medium size interfaces form one to three pairs of patches and large interfaces three or more. Interfaces of equivalent size in complexes have a similar number of patches. When the interface residues are distributed into segments of the polypeptide chain following Jones & Thornton (Reference Jones and Thornton1997) and Pal et al. (Reference Pal, Chakrabarti, Bahadur, Rodier and Janin2007), the mean number of segments is 3·9 per chain, and that of interface residues per segment is 6·4, again like in the complexes in Table 2. However, the averaging hides a great disparity between the small interfaces, often fragmented into very short segments, and larger ones that tend to contain a small number of long segments. In T=3 and pT3 capsids, some interfaces contain segments of up to 47 residues located at the N or C terminus of the polypeptide chain. These tail segments adopt extended conformations and are heavily involved in subunit contacts. In T=3 capsids, the tails are preferentially involved in the twofold and quasi-sixfold interfaces; in pT3 capsids, they contribute primarily to the interfaces in the IAU (Bahadur et al. Reference Bahadur, Rodier and Janin2007).
Residue conservation
The subunit contacts hold the capsid together and play a major role in the process by which it assembles itself. They should therefore impose stringent evolutionary constraints on the protein sequence. Bahadur & Janin (Reference Bahadur and Janin2008) used the Shannon entropy to estimate residue conservation in sets of aligned capsid protein sequences. The mean value of s normalized to the average value in each polypeptide chain, is close to 1 for the interface residues, which reflects the fact that they constitute two-thirds of the polypeptide chain. Nevertheless, there are very significant differences within a chain. As in other systems, the residues of the protein interior are much better conserved than surface residues (s=0·7 vs. 1·6), and the residues of the interface core, much better conserved than the rim (s=0·8 vs. 1·2). Moreover, the capsids contain residues involved in several interfaces, with no equivalent in homodimers or binary complexes. They are abundant (34% of all interface residues), and their mean entropy s=0·8 shows that they are better conserved than the residues involved in only one interface.
The sequence conservation needs not be homogeneous within a given interface. An example is the interface between chains A and C of the pT3 rhinovirus (the green surface in Fig. 11). It is well conserved as a whole (s=0·9), but chain A has a highly divergent C tail with s=2·0 and a highly conserved N tail with s=0·6, and both tails contribute to the interface. The C tails are more divergent than the N tails in most pT3 capsids, but in general, the tails have the same rate of evolution as the parts of the polypeptide chains that form the globular core of the subunits (Bahadur & Janin, Reference Bahadur and Janin2008).
A plausible mechanism for capsid assembly
The self-assembly of a virus capsid comprises the folding of its subunits, their association and maturation steps that often involve conformation changes and covalent modifications. This elaborate process may implicate nucleic acids, accessory viral proteins and host chaperones, in addition to the protein subunits (Steven et al. Reference Steven, Trus, Booy, Cheng, Zlotnick, Caston and Conway1997, Reference Steven, Heymann, Cheng, Trus and Conway2005; Liljas, Reference Liljas1999; Caspar, Reference Caspar1980). Nonetheless, it can be amazingly fast: bacteriophage T4 goes through a complete cycle of infection, replication and lysis in 15 min; the capsid assembles in minutes in spite of the large number of polypeptide chains that constitute it (Leiman et al. Reference Leiman, Kanamaru, Mesyanzhinov, Arisaka and Rossmann2003). This implies that capsid self-assembly must go through a series of low-order steps and intermediate species. These species, called capsomers, should resemble small oligomeric proteins.
Prevelige et al. (Reference Prevelige, Thomas and King1993) have analyzed the in vitro self-assembly of bacteriophage P22, and Xie & Hendrix (Reference Xie and Hendrix1995), that of bacteriophage HK97. The T=7 lattice of these two capsids can be viewed as comprising pentamers with I 5 symmetry and hexamers with Q 6 quasi-symmetry, in a 1:5 ratio. In solution, the HK97 subunits form pentamers and hexamers that interconvert slowly and reassemble most efficiently when they are in the same 1:5 ratio as in the capsid. All models of the capsid self-assembly assume that the capsomers keep their structure during the process, and thus, they can be identified in the capsid itself (Johnson & Speir, Reference Johnson and Speir1997; Reddy et al. Reference Reddy, Giesing, Morton, Kumar, Post, Brooks and Johnson1998; Dokland, Reference Dokland2000; Zlotnick, Reference Zlotnick2005). Bahadur et al. (Reference Bahadur, Rodier and Janin2007) postulate in addition that the capsomers contain the largest interfaces, and that capsomer–capsomer association makes use of the medium-size interfaces in priority. The many small interfaces that are seen in the capsids may contribute to the stability of the final product, but they are unlikely to play a role in its formation.
In the case of HK97, this postulate suffices to determine an assembly pathway compatible with the data of Xie & Hendrix (Reference Xie and Hendrix1995). The largest interfaces are the I 5 and Q 6 interfaces, and they build the hexamers and pentamers seen in solution. The next largest are of medium size; they occur between hexamers or between hexamers and pentamers in the capsid, and in solution, they should allow hexamers to associate in pairs or with a pentamer (Bahadur et al. Reference Bahadur, Rodier and Janin2007). Recently, Stockley et al. (Reference Stockley, Rolfsson, Thompson, Basnak, Francese, Stonehouse, Homans and Ashcroft2007) have analyzed the assembly of bacteriophage MS2 by electrospray ionization-mass spectrometry. They find that the T=3 capsid of MS2 dissociates into symmetric dimers, some of which become asymmetric upon addition of an RNA stem–loop fragment. Symmetric and asymmetric dimers then associate into dimer hexamers, whereas no pentamer is formed. These observations are compatible with the model of Bahadur et al. (Reference Bahadur, Rodier and Janin2007) : MS2 has large I 2 and Q 2 interfaces that build a symmetric and an asymmetric dimer, respectively. The next largest are the Q 3 interfaces building the dimer hexamers; they are of medium size, and larger than the I 5 interface needed to build pentamers.
Protein–nucleic acid recognition
Nucleic acid recognition by proteins is a process of major interest to biology, actively studied by biophysicists, structural biologists and bioinformaticians. In this review, we consider it only in relation to protein–protein recognition, and use results of the transverse studies of protein–DNA complexes by Jones et al. (Reference Jones, van Heyningen, Berman and Thornton1999), Nadassy et al. (Reference Nadassy, Wodak and Janin1999) and Sarai & Kono (Reference Sarai and Kono2005), and of protein–RNA complexes by Jones et al. (Reference Jones, Daley, Luscombe, Berman and Thornton2001), Treger & Westhof (Reference Treger and Westhof2001), Ellis et al. (Reference Ellis, Broom and Jones2007) and Bahadur et al. (Reference Bahadur, Zacharias and Janin2008). Each study relies on sets of PDB entries that comprise 26–81 complexes, similar in size to the sets of protein–protein interfaces discussed in previous sections. The DNA is double stranded in most of the complexes; the RNA is single stranded with few exceptions, but when its sequence and length allow, it folds into stem–loops and a variety of other structures that include double helical segments. The complexes are mostly non-obligate, forming only when the protein encounters the nucleic acid, but like the protein–protein complexes of Section 4.1, they cover a wide range of stability, lifetimes and functions.
The data in Table 3 show that protein–DNA and protein–RNA complexes tend to bury less molecular surface than homodimers, which are obligate assemblies, but more than the average protein–protein complex. Here, again, the presence of large interfaces is associated with conformation changes that affect the protein and the nucleic acid components of many of the complexes (Jones et al. Reference Jones, van Heyningen, Berman and Thornton1999; Nadassy et al. Reference Nadassy, Wodak and Janin1999; Ellis & Jones, Reference Ellis and Jones2008). A peculiar feature of protein–RNA complexes is that more surface area is lost on the RNA than the protein side: 8% on average, which is like the distribution of the BSA at the interfaces of protease–inhibitor complexes, and unlike most other types of protein–protein interfaces (Bahadur et al. Reference Bahadur, Zacharias and Janin2008). The asymmetry, attributable to a convex RNA surface fitting into a concave protein surface, is low in protein–DNA complexes, and most pronounced when the RNA is short and does not form secondary structure.
Table 3. Properties of protein–nucleic acid interfaces

a Data from Bahadur et al. (Reference Bahadur, Zacharias and Janin2008). Numbers in the left column are for the protein component; those in the right column are for the RNA component.
b Data from Nadassy et al. (Reference Nadassy, Wodak and Janin1999). Numbers in the left column are for the protein component; those in the right column are for the DNA component.
c Data reported from Table 2 for comparison.
The BSA histograms of Fig. 12 show that the protein–nucleic acid interfaces have a wide range of size. Both DNA and RNA can form very large interfaces with proteins. The peak of the distribution is near 3000 Å2 for DNA and 2000 Å2 for RNA. Many of the proteins that bind DNA are homodimers or tandem repeats that bear two or more sites of interaction: 60% in the set of Nadassy et al. (Reference Nadassy, Wodak and Janin1999). This is less common with RNA binding proteins and may explain the larger average BSA of the protein–DNA interfaces. Moreover, while very few protein–DNA interfaces bury less than 1500 Å2, 10% of the protein–RNA interfaces have a BSA <1200 Å2. The smallest, with a BSA near 900 Å2, occur in crystals with a very short RNA component that may reproduce only part of the protein–RNA contact in the cell. Omitting those, the distributions in Fig. 12 suggest that there is a minimum size for a functional protein–nucleic acid interface, and that it has the same BSA as the smallest functional protein–protein interfaces. Thus, protein–nucleic and protein–protein recognition have a similar size rule, which extends from the BSA to the number of amino acid residues implicated in recognition, since the BSA per interface residue is nearly the same (Table 3). On the DNA and RNA side of the interfaces, the nucleotides engaged in secondary structure tend to contribute less to the BSA than those in extended segments, probably because they are less solvent accessible to start with.

Fig. 12. Interface size in complexes with DNA and RNA. Histogram of the BSA in the sets of protein–DNA and protein–RNA complexes reported in Table 3. The BSA is from both the protein and the nucleic acid components.
Beyond these similarities, major differences between the interfaces of protein–DNA or RNA complexes on one hand, and protein–protein complexes on the other hand, reflect the different chemical nature of proteins and nucleic acids. The bases, the sugars and the phosphate groups contribute about one-third each to the ASA of the nucleic acids, but their respective contributions to the BSA are different in DNA and RNA. The phosphates contribute 43% of the DNA surface buried in contacts with proteins, and the deoxyribose, 29%. With RNA, the phosphate contribution is less (26%), and that of the ribose reaches 39% because of the heavy implication of the 2′-OH in interactions with protein groups (Treger & Westhof, Reference Treger and Westhof2001; Bahadur et al. Reference Bahadur, Zacharias and Janin2008). The DNA or RNA side of the interfaces is highly polar and negatively charged, and in counterpart, the protein side is more polar than a protein–protein interface or than the solvent accessible surface: f np is 52–55% instead of 57%. Moreover, it is positively charged over 20–23% of its BSA, which implies a peculiar amino acid composition and marked propensities. The propensities to be in contact with DNA are more marked than with RNA, and they differ from the propensities to be in contact with another protein, plotted for comparison in the same Fig. 7 c. Asp and Glu are nearly excluded from contacts with both DNA and RNA; Arg is favored, whereas Lys is indifferent, but abundant. Aromatic residues are mildly favored at protein–RNA, but not protein–DNA interfaces; aliphatic residues are either indifferent (Val, Ile) or disfavored (Leu, Met, Pro).
Protein–nucleic acid interfaces bury an even lower fraction of their interface atoms than protein–protein interfaces do (f bu=24–29% vs. 34–36%; Tables 2 and 3) and their L D packing index is lower (37–39 vs. 42–45). This suggests that on average, the atomic packing is less tight than at protein–protein interfaces, yet the buried atoms on the protein side of protein–DNA interfaces have Voronoi volumes that are within 5% of the volumes in the protein interior (Nadassy et al. Reference Nadassy, Wodak and Janin1999). On the DNA side, the buried atoms have volumes greater by 0–8% than reference volumes derived from crystals of pure DNA (Nadassy et al. Reference Nadassy, Tomas-Oliveira, Alberts, Janin and Wodak2001). The packing quality expressed by the f bu and L D parameters is particularly poor in the complexes that involve transfer RNA, even though their interfaces are large (Bahadur et al. Reference Bahadur, Zacharias and Janin2008).
The polar character of protein–nucleic acid interfaces correlates with their large number of H bonds and high residual hydration (Table 3). The surface density of the H bonds is significantly greater than in protein–protein complexes (1 per 125–145 Å2vs. 190 Å2), in line with the contribution of polar atoms to the BSA of each system; and also that of the interface waters, but the values in Table 3 suffer from the unequal resolution of the X-ray structures and the inconsistent way they report solvent positions.
Conclusion: folding and recognition
Protein–protein recognition has much in common with protein folding. Both are self-assembly processes during which solvent is removed from the surface of polypeptide chains, whereas new van der Waals and polar interactions are formed between protein atoms. Features that stress that similarity are the atomic packing of the interfaces, their amino acid composition and their conservation in evolution. All three distinguish between the core and the rim of the interfaces. The packing density judged from the Voronoi volumes is the same for the atoms buried inside proteins and at the interfaces, where the buried atoms define the interface core. We have seen that the residue conservation in homologous sequences estimated by the Shannon entropy is a property of the interface core as opposed to the rim. In oligomeric proteins, the amino acid composition of the interface core resembles that of the protein interior, remote from that of the solvent accessible surface. This is presented in Table 4 in the form of Euclidean distances. The greatest distances are between the protein surface and either the interface core or the protein interior. The interface core of protein–protein complexes is equally distant in composition from the protein surface and the interior, in line with the fact that the residues concerned are surface residues in the free components, and interior residues in the complexes. The rim of both types of interfaces, and the crystal packing interfaces, which bury few atoms and do not have a well-defined core, are all close in composition to the protein surface. The protein surface in contact with DNA and RNA has a very different composition from all the other surfaces, but that can be safely attributed to the different chemical nature of its partner.
Table 4. Euclidean distances between amino acid compositions

The Euclidean distance Δf between the percent composition f of the protein surface or interior and that, f′, of an interface, is defined by Δf 2=1/19∑i=1−20(f i−f i′)2. The compositions are expressed as percent contributions to the ASA or the BSA.
Data from aBahadur et al. (Reference Bahadur, Chakrabarti, Rodier and Janin2003), bChakrabarti and Janin (Reference Chakrabarti and Janin2002), cBahadur et al. (Reference Bahadur, Chakrabarti, Rodier and Janin2004), dNadassy et al. (Reference Nadassy, Wodak and Janin1999) and eBahadur et al. (Reference Bahadur, Zacharias and Janin2008) .
Several other features do, however, distinguish protein–protein interfaces from the protein interior. One is the presence of immobilized water. Its abundance, which is certainly underestimated in Table 2, suggests that the dehydration of protein–protein interfaces is only 80–90% complete, whereas that of the protein interior is almost 100% (Hubbard & Argos, Reference Hubbard and Argos1994). Another concerns arginine residues. Their highly polar side chain is essentially excluded from the protein interior, but not of protein–protein interfaces. Arginine is abundant in all types of protein–protein interfaces, even in their core. Some interface arginines are essential to the biological function, for instance the P1 arginine of trypsin inhibitors (Janin & Chothia, Reference Janin and Chothia1976), or the ‘arginine finger’ of Ras-GAP that activates the hydrolysis of GTP by the Ras protein (Scheffzek et al. Reference Scheffzek, Ahmadian, Kabsch, Wiesmüller, Lautwein, Schmitz and Wittinghofer1997); others just contribute to stability. Birtalan et al. (Reference Birtalan, Zhang, Fellouse, Shao, Schaefer and Sidhu2008) have recently analyzed the role of arginines and tyrosines at the antigen-combining site of antibodies, where they are the most abundant residue types. Residues of both types make stabilizing interactions, but arginine discriminates poorly between the cognate antigen and other proteins. This reminds us of the dual role of arginine in protein–DNA recognition: its side chain gives H bonds both to the phosphate backbone and to guanine bases in the major groove. The first H bonds are non-specific, yet important for stability; the second are major elements of the sequence specificity of many transcription factors.
The analogy between subunit assembly and protein folding is particularly significant when the assembly undergoes large conformation changes, that is in flexible recognition. We observe that flexible recognition often leads to the formation of a large interface, whereas rigid-body recognition yields a small or a standard-size interface. The changes that accompany association in that case resemble late stages of protein folding: preformed elements, secondary structure or domains, move one relative to the others. The movements affect the shape of the protein surface, which raises the question of how specificity can be achieved, and at what stage of the assembly it appears. Disorder-to-order transitions, which represent early steps in folding, are the rule in homodimers, where folding and assembly occur simultaneously. They are a common event in proteins that bind DNA, and also in protein–protein complexes that have a natively denatured component. In our sample, such transitions are observed only locally, for instance at the N terminus of Gα in transducin.
The viral capsids display some remarkable features that they probably share with other multi-component assemblies, but not binary complexes or homodimers. In a capsid, the subunit contacts bury half or more of the protein surface; in complexes or homodimers, that fraction rarely exceeds 20%. The contacts implicate a majority of the protein sequence, and they affect its composition and its conservation in evolution much more than in a smaller assembly. Many interfaces of very different sizes coexist, and they frequently overlap, with one-third of the residues part of two or more interfaces; these residues are much better conserved than those involved in only one interface. The size distribution of the interfaces has led us to propose a simple model of the capsid assembly, in which the largest interfaces form first. This model, compatible with experimental data on two bacteriophages, may be extended to other multi-component systems: recent data from mass spectrometry indicate that the assembly of some large oligomeric proteins also proceeds by forming the largest interfaces first (Lévy et al. Reference Lévy, Erba, Robinson and Teichmann2008). The self-assembly of binary complexes has been extensively studied, and it is well understood in cases when rigid-body recognition is a valid approximation (e.g. barnase–barstar and antigen–antibody complexes). In comparison, flexible recognition and the self-assembly of multi-component systems are still enigmatic. Both processes are of great interest to biologists; they are undoubtedly complex, but they deserve that physicists and biophysicists give them the same attention as they have to protein folding over the past 20 years.
Acknowledgements
We thank E. Lévy (Cambridge, UK) for Fig. 1 and F. Cazals (Sophia-Antipolis, France) for Fig. 3. We acknowledge a Research Associateship to R.P.B. of the Deutsche Forschungsgemeinschaft, support to J.J. of the 3D-Repertoire and SPINE2-Complexes programs of the European Union, and financial support from DBT, India, to P.C.