1. IntroductionFootnote 1
Second language acquisition (SLA) and translation studies (TS) share a common interest in investigating language use where several linguistic systems are at play simultaneously in some way. Whilst the two disciplines have operated somewhat independently in the past, growing interest in similarities between them has recently led to the emergence of a new line of research described as constrained language use (Lanstyák & Heltai Reference Lanstyák and Heltai2012; Kolehmainen, Meriläinen & Riionheimo Reference Kolehmainen, Meriläinen, Riionheimo, Paulasto, Meriläinen, Riionheimo and Kok2014; Kruger & van Rooy Reference Kruger and van Rooy2016, Reference Kruger and van Rooy2018; Rabinovich et al. Reference Rabinovich, Nisioi, Ordan and Wintner2016),Footnote 2 which explores whether and to what degree different types of constrained language use have common characteristics.
Research designs in both SLA and TS tend to follow two seemingly opposed approaches. On the one hand, the study of crosslinguistic influence (CLI), traces of one language in another, is a central research focus in both disciplines (Jarvis Reference Jarvis2000 for SLA, Toury Reference Toury2012:310–315 for TS). On the other hand, both disciplines are interested in phenomena that function similarly irrespective of the languages or language-pairs involved, such as explicitation or simplification (Granger Reference Granger2015 for SLA, Mauranen & Kujamäki Reference Mauranen and Kujamäki2004 for TS). These approaches operate on different levels of abstraction – the potential general features will inevitably have language-specific realizations, which make a fully comparable operationalization between languages challenging (House Reference House2008, Becher Reference Becher2010). The problem is amplified by the fact that in the case of both SLA and TS, the body of research and the subsequent theorizing have been based on few languages and language-pairs, predominantly including English. To our knowledge, this is the case also in studies that explicitly explore constrained language use, although the meta-analysis by Kolehmainen et al. (Reference Kolehmainen, Meriläinen, Riionheimo, Paulasto, Meriläinen, Riionheimo and Kok2014) includes studies on various languages.
The focus on English limits the generalizability of the results gained thus far (for a discussion, see Volansky, Ordan & Wintner Reference Volansky, Ordan and Wintner2013). Given the extraordinary situation of English as a de facto lingua franca and the typological diversity of the languages of the world, further evidence on constrained language use is needed from languages of different typological and geopolitical status. The present paper addresses this research gap by exploring the typicalities of constrained language use in Finnish – a language that diverges from English considerably both typologically and geopolitically. We report a quantitative bottom–up study on similarities between Finnish as a second language (F2) and translated Finnish (FT). Specifically, we contrast F2 and FT with their non-constrained counterpart – non-translated Finnish as a first language (F1) – to see whether and to what extent the features that distinguish F2 and FT from F1 are shared by the constrained varieties. Our research questions are:
1. Which linguistic phenomena consistently distinguish both F2 and FT from F1?
2. Are there variety-, language-pair-, or register-specific differences?
3. How do the detected features of constrained Finnish line up with earlier suggestions of general phenomena?
Our data come from various corpora (listed towards the end of the present article), complemented with data collected ad hoc to increase data comparability. The data represent two registers – academic and narrative texts – and two first/source languages – German and Russian. We implement a two-phase methodological procedure to detect and analyze differences across the compared varieties. First, we conduct a keyness analysis (for the concept, see Gabrielatos Reference Gabrielatos, Taylor and Marchi2018), to detect syntactically defined part-of-speech bigrams whose frequency consistently distinguishes constrained and non-constrained Finnish across the majority of data subsets. Then, we carry out a Multi-dimensional Analysis (e.g. Biber Reference Biber1988, Reference Biber2014) based on these key features, to interpret their linguistic and textual distributional patternings in the light of constrained language use in Finnish.
The paper is structured as follows: Section 2 discusses the core theoretical constructs and earlier research and Section 3 introduces the data and method used. Section 4 reports on our results, linking them to earlier findings and Section 5 discusses their theoretical impact, offering concluding remarks on future work.
2. Variation in constrained language use
2.1 Varieties, crosslinguistic influences and registers
In order to reliably relate any given linguistic phenomenon to constrained language use, there are a minimum of three factors to take into account. First, two or more constrained varieties must diverge from the non-constrained variety (e.g. differing relative frequencies of a linguistic phenomenon). Second, this divergence should be observable across two or more language-pairs, so as to tease apart the general tendencies from CLI. Third, the study should be conducted across multiple registers, to control for the potential interaction between the observed divergence and register-specific particularities (see also Kruger & van Rooy Reference Kruger and van Rooy2018).
Lanstyák & Heltai (Reference Lanstyák and Heltai2012) point out that all communication is somehow constrained but that the research on constrained communication refers to varieties where constraining factors ‘play a greater than average role’ (e.g. learner language or translated language) (Lanstyák & Heltai Reference Lanstyák and Heltai2012:100). The underlying reason for these similarities has been suggested to stem from similar cognitive and social environments between such varieties (Kruger & van Rooy Reference Kruger and van Rooy2016:27). The situation resembles bilingual language activation in bilingualism studies (Grosjean Reference Grosjean and Nicol2001). Constrained language use can be seen to increase the cognitive load which can lead to increasing explicitness (Rohdenburg Reference Rohdenburg1996). In SLA, this mechanism has been suggested to take place in terms of interacting learning principles (Filipović & Hawkins Reference Filipović and Hawkins2013). Finally, differences between constrained and unconstrained varieties may also stem from strategic choices: as Lefer & Vogeleer (Reference Lefer and Vogeleer2013:10) point out, ‘it can be hypothesized that translators stop searching for a better solution when they believe their current solution to be sufficiently relevant’.
In our view, the link between the observed linguistic phenomena of constrained language use and their underlying explanation often boils down to complexity, or ‘a property or quality of a phenomenon or entity in terms of (1) the number and the nature of the discrete components that the entity consists of, and (2) the number and the nature of the relationships between the constituent components’ (Bulté & Housen Reference Bulté, Housen, Housen, Kuiken and Vedder2012:22). The notion of complexity can be approached from a relative or an absolute point of view (Miestamo Reference Miestamo, Kerge and Sepper2006), where the former adresses it in relation to language users and the latter in relation to linguistic features (for a detailed discussion in SLA context, see Bulté & Housen Reference Bulté, Housen, Housen, Kuiken and Vedder2012). Extending Bulté & Housen’s (Reference Bulté, Housen, Housen, Kuiken and Vedder2012) taxonomy of learner-/second-language (L2) complexity to constrained language use, we hypothesize that while the absolute complexity feeds into the objective determinants of relative complexity, the effect of such phenomena differs between constrained and non-constrained language users, which is consequently visible in their use of linguistic features defined complex in absolute terms.
In subsequent studies, the focus has mostly been on communication constrained by (at least) two languages. In a meta-analysis of constrained language use (in their terms, interlingualreduction), Kolehmainen et al. (Reference Kolehmainen, Meriläinen, Riionheimo, Paulasto, Meriläinen, Riionheimo and Kok2014) compare studies on translated, contact, and second language. Kruger & van Rooy (Reference Kruger and van Rooy2016) contrast translated English with indigenized varieties, whereas Rabinovich et al. (Reference Rabinovich, Nisioi, Ordan and Wintner2016) look at similarities between translated and second languages. To our knowledge, the most fine-grained take on the constrained varieties can be found in Kruger & van Rooy (Reference Kruger and van Rooy2018), as they distinguish not only native, L2 and translated varieties but also the amount of language contact in both native and L2 varieties. In the present study, the constrained varieties are Finnish written by L2 speakers and texts translated into Finnish from another language by professional translators (typically speakers of Finnish as the first language (L1)). The reference variety consists of non-translated texts written by L1 speakers of Finnish.
CLI is among the most widely studied phenomena in both L2 and TS contexts. At the core of the inquiry is the identification of linguistic phenomena that can be attributed to specific first/source languages. Following Jarvis (Reference Jarvis2000, Reference Jarvis2010), reliable support for CLI should be based on the following aspects: (i) congruent linguistic behavior in L2 by speakers of the same L1; (ii) diverging linguistic behavior in L2 by speakers of different L1s; (iii) congruence between the L2 behavior of speakers of the same L1 and the linguistic system of that language; and (iv) divergence between the linguistic systems of the L1s represented.
Despite the centrality of CLI within L2 and TS research, it has received only limited attention in research on constrained language use, and often the data used do not even allow for distinguishing general and language-pair-specific phenomena. Perhaps the most notable exception is Rabinovich et al. (Reference Rabinovich, Nisioi, Ordan and Wintner2016), who approach CLI from a computational perspective: they use texts translated into English from Germanic and Romance language families and build separate language models for each family. They then use these models to predict whether L2-English texts were written by L1 speakers of a Germanic or a Romance language. The results show that the model based on data from the same language family as the author’s L1 indeed outperforms the model based on the other language family – effectively corroborating the similarity of CLI in L2- and translated English.
Different registers of one language may vary drastically both qualitatively and quantitatively in their grammatical properties (for the concept, see Biber & Conrad Reference Biber and Conrad2009; for examples from a variety of languages’, see Biber Reference Biber2014). The notion of register-specific linguistic systems is theorized also to apply to the level of individual language users in Iwasaki (Reference Iwasaki2015), suggesting that the grammars of different registers are learned partially in isolation – in a usage-based fashion – and inter-connected only at a later stage. This suggestion is supported by Ivaska (Reference Ivaska2015) in the observation that academic F2 users adjust their tense use to that of F1 academic texts over the course of 16 months – due to their their extensive exposure to that register. In addition, Szymor’s (Reference Szymor2018) results support the usage-based nature of register-specific individual grammars, showing that the use of aspect in modal contexts in Polish translations of legal texts reflect distributions found in non-translated Polish in general rather than those of non-translated Polish legal texts. This can be related to the translators’ lesser exposure to that particular register and the ensuing reliance on their knowledge of other registers.
Finally, registers and CLI do not work in isolation. Although CLI is typically approached from a structural perspective – lexical, morphological or syntactic – registers are to some degree also specific to different linguacultures. As Lefer & Vogeleer (Reference Lefer and Vogeleer2013:15) put it, ‘some genre conventions are common to different cultures, while some others are culture-specific … As a result, in translation, localization … and non-native writing, interference and normalization can be traced not only at the micro-level of linguistic features but also at the macro-level of genre conventions’.
2.2 Contrastive quantitative research on constrained varieties in Finnish
We are not aware of any studies that explicitly address constrained language use in Finnish (although Jantunen Reference Jantunen and Eslon2008 draws from TS when hypothesizing possible L2 universal tendencies). Furthermore, systematic studies of register variation in either F2 or FT are rare. In what follows, we review earlier research on F2 and FT relevant for the present study.Footnote 3 Given the methodological foundations of our work, we limit ourselves to quantitative approaches. Furthermore, as our study is corpus-driven, we focus on bottom–up observations of potentially interesting phenomena, and then relate our findings to earlier studies. Finally, with a view to attributing any given observed linguistic phenomenon to a certain variety, we compare the said variety and other varieties (Szmrecsanyi Reference Szmrecsanyi2017). As a consequence, we only consider studies with a contrastive component (although earlier results discussed in Section 4 are not necessarily contrastive in nature).
Most quantitative contrastive work on F2 has been conducted on two corpora: the International Corpus of Learner Finnish (ICLFI, Jantunen Reference Jantunen2011a) or the Corpus of Advanced Learner Finnish (Ivaska Reference Ivaska2014a). Using an automatically annotated version of ICLFI, Jantunen (Reference Jantunen, Lehtinen, Aaltonen, Koskela, Nevasaari and Skog-Södersved2011b) detected grammatical key features that distinguish F2 from F1 at different proficiency levels. While groundbreaking as a research design, the results are very preliminary in nature with a number of methodological concerns related to CLI and register effects, which makes it difficult to attribute the findings to the F2 variable. Ivaska (Reference Ivaska2014b) detected four types of differences between F2 and F1: (i) F2 texts make less use of morphosyntactically complex constructions (see also Ivaska, Reunanen & Siitonen Reference Ivaska, Reunanen and Siitonen2016); (ii) F2 texts express modal possibility less frequently (see also Ivaska Reference Ivaska2014c); (iii) F2 texts are more narrative, as reflected in their higher frequency of the simple past tense (see also Ivaska Reference Ivaska2015); and (iv) certain register-specific, lexically limited constructions are less frequent in F2 texts. The results are methodologically reliable, in that all data belong to the same register, although this has the downside of limiting their generalizability. Potential CLI could not be evaluated, as the speakers’ L1 backgrounds were not controlled for.
As for possible CLI in F2, Spoelman (Reference Spoelman2013) studied the use of partitive case by Dutch, Estonian and German F2 users and showed clear differences between Estonian and the two other learner groups – likely due to typological similarities between Finnish and Estonian. Ivaska & Siitonen (Reference Ivaska and Siitonen2017a) detected morphological features that distinguished Chinese, Estonian, German, Polish, Russian and Swedish F2 users in a data-driven manner. They showed that Swedish F2 users express pronominal subjects overtly more often than the other learner groups and linked it to the fact that Swedish is the only L1 included with mandatory pronominal subject-marking and without conjugational subject marking. Similarly, Estonian F2 users use more numerals, possibly due to lexical and phrasal similarities between Finnish and Estonian.
Most quantitative contrastive approaches to FT use the Corpus of Translated Finnish (Mauranen Reference Mauranen and Olohan2000), including a number of studies on unique items – features that are typical for the target language but not for the source language. Tirkkonen-Condit (Reference Tirkkonen-Condit2004, Reference Tirkkonen-Condit, Mauranen and Jantunen2005) identified certain language-specific constructions of Finnish – verbs expressing sufficiency and the clitic pragmatic particles -kin and -hAn – to be less common in FT than in F1. Similarly, Kujamäki (Reference Kujamäki2004) reported on a Finnish–English/German–Finnish back-translation experiment and concluded that unique items are consistently used less in the back-translations than in the original F1. Jantunen & Eskola (Reference Jantunen and Eskola2002) (see also Eskola Reference Eskola2004, Jantunen Reference Jantunen2004) showed that unique items affect FT at various levels: the non-finite referative construction (e.g. Tiedän hänen tulleen ‘I know (that) (s)he has come’) is consistently more frequent in F1 than in FT from English or Russian. On the other hand, target language (here, Finnish) constructions with a formal equivalent in the source language (here, English and Russian) tend to be used more often in FT than in F1. In Jantunen & Eskola’s case, such is the case of a non-finite construction expressing motivation for a certain behavior (e.g. Kiirehdin ehtiäkseni junaan ‘I hurry (in order) to catch the train’), and the degree modifier hyvin ‘very’. Finally, Mauranen & Tiittula (Reference Mauranen, Tiittula, Mauranen and Jantunen2005) compared the use of subjectless impersonal constructions (e.g. Jos tupakoi … ‘if [one] smokes …’) in FT from English and German and F1 and concluded that the construction – which lacks a similar counterpart in Germanic languages – is more common in F1, while FT relies on solutions that are more aligned with those in the original English and German texts. According to Mauranen & Tiittula (ibid.), there is also a related explicitation tendency: FT contains more first person nominative pronouns (minä) than F1. This is probably due to constructional differences: both English and German have obligatorily overt subject marking, whereas in Finnish subject marking is obligatory only in the third person.
CLI in FT has also been studied outside the unique item hypothesis. Mauranen (Reference Mauranen2004) compared word frequency data to measure differences between FT and F1 and between SL-specific subcorpora and a subcorpus with a range of SLs. She concluded that ‘translations resemble each other more than original target language texts, but a clear source language effect is also discernible. This implies that transfer is one of the causes behind the special features of translated language’ (ibid.:78). Mauranen’s observation underlines the often-disregarded fact that general features of translated language and CLI are two facets of the same phenomenon, differing in their level of abstraction. L. Ivaska (Reference Ivaska, Jantunen, Brunni, Kunnas, Palviainen and Västi2019), in turn followed the line of research unearthed by Baroni & Bernardini (Reference Baroni and Bernardini2006) and Koppel & Ordan (Reference Koppel and Ordan2011) – with the goal to distinguish FT from F1 and to tease apart the different source languages of FT by means of core vocabulary frequencies and machine learning techniques. She was able to accurately distinguish FT and F1 texts; translations from different source languages, while diverging from each other in a systematic manner, were more difficult to categorize reliably. The results corroborate Mauranen’s point on the intertwined nature of CLI and the more general tendencies of translated language.
3. Data and methodFootnote 4
3.1 Data: Composite corpus of existing and ad hoc materials
The data in this study consist of 12 subcorpora, and include Finnish texts constrained by second language use and by translation in two registers (academic and narrative), together with comparable non-constrained texts. The first languages included are German and Russian. We attempted to maximize the replicability of the study results/design by choosing registers and first/source languages included in existing corpora. The existing corpora used are: Corpus of Translated Finnish (CTF, including both F1 and FT), Contrastive Corpus of Finnish and German (FinDe), International Corpus of Learner Finnish (ICLFI), InterCorp (including both F1 and FT), Corpus of Advanced Learner Finnish (LAS2, including both F1 and F2), Corpus of Academic Finnish (LAS1). In order to ensure the comparability of each constrained subcorpus and the respective unconstrained subcorpus, we use two unconstrained subcorpora for both registers – one of unpublished texts to match F2 data and another of published texts to match FT data (on the effects of editorial intervention, see Kruger Reference Kruger, De Sutter, Lefer and Delaere2017). To this end, we collected an additional, ad hoc corpus of unpublished F1 narrative texts. Finally, because in the context of F2, the proficiency level also plays a role, we included only texts representing advanced proficiency level. That is, all the texts from ICLFI have been evaluated to reflect level C (proficient user) in the Common European Framework of Reference. In LAS2, a minimum of 2 texts from each informant were evaluated, and only texts by informants where both evaluations reflect C level were included.
After stripping off all legacy annotation, we annotated the data according to the Universal Dependencies scheme (Bohnet et al. Reference Bohnet, Nivre, Boguslavsky, Farkas, Ginter and Hajič2013) using the Turku neural parser (Kanerva et al. Reference Kanerva, Ginter, Miekka, Leino and Salakoski2018). To minimize the effects of other artifacts (e.g. text length, topic, authorship), we then shuffled the sentences of each subcorpus and reconstructed text blocks of 50 sentences (Rabinovich et al. Reference Rabinovich, Nisioi, Ordan and Wintner2016). This step also ensures that the subcorpora are directly comparable while still maintaining the general variation inherent in them. Table 1 sums up the data provenance and size for the 12 subcorpora, 11 of which come from pre-existing resources and one is collected ad hoc.
Table 1. Data provenance and size information in terms of the number of text blocks (b) and words (w) in the corpora.

3.2 Keyness analysis
The first step in the analysis aims to detect consistent quantitative differences between the varieties that emerge from the data bottom up. First, we extracted the normalized frequencies of all syntactically defined part-of-speech (POS) bigrams from each text block. Each bigram provides information on POS, syntactic relationship, as well as the constituent order and hierarchy. Figure 1 exemplifies the feature set with the following POS bigrams: ADJNODE_amod_NOUNHEAD (nykyaikainen–tilanne ‘contemporary–situation’), NOUNNODE_nsubj:cop_ADJHEAD (tilanne–sellainen ‘situation–such’), AUXNODE_cop_ADJHEAD (on–sellainen ‘is–such’), ADJHEAD_ccomp_VERBNODE (sellainen–tietävät ‘such–know’), and so on. In this first phase, we included the 1000 most frequent bigrams.

Figure 1. Tree visualization of POS bigrams.
Next, we detected consistent differences between constrained and non-constrained texts. Each constrained data subset and its non-constrained counterpart were contrasted to find the bigrams whose frequency differences contribute to distinguishing the two. The resulting sets of bigrams were then compared to single out those bigrams that best correlate with the constrained vs. non-constrained distinction. This two-phase approach has two advantages related to the opposite pulls of comparability and generalizability (for a discussion, see Leech Reference Leech, Nesselhauf and Biewer2006): each pairwise comparison can be conducted with maximally comparable data, and each pairwise comparison is of equal importance in the overall comparison.
In the pairwise comparisons, we use Boruta feature selection (Kursa & Rudnicki Reference Kursa and Rudnicki2010) as a statistical technique to find the bigrams contributing to the difference. Boruta makes use of Random Forests (Breiman Reference Breiman2001), a machine learning algorithm for automatic data classification. Boruta adds randomness to the data by creating a duplicate variable for each actual variable (here, bigram) and randomly permutating its values (here, normalized frequencies). Then, it creates a decision-tree-based forest model to find the variables that are best at the classification task at hand (here, distinguishing between constrained and non-constrained texts). It then compares the actual variables’ performance to the duplicate variables and deems as important those variables that consistently outperform the permutated duplicates. The procedure is repeated iteratively – leaving out in each run variables that are clearly less significant than the duplicates – resulting in a list of bigrams that consistently contribute to the classification. After obtaining such lists from each pairwise comparison, we compare them to find the bigrams that are included in over half of the lists and can thus be considered key features.
Thirty-two of the 1,000 syntactic POS bigrams analyzed were considered as key in over half of the pairwise comparisons (see Appendix). While these comparisons make it possible to capture the bigrams that consistently contribute to distinguishing constrained from non-constrained language, they do not tell us anything about the underlying functional reasons. As pointed out by Gries (published online 16 April Reference Gries2019), applying tree-based methods without addressing the potential interactions between variables may indeed lead to suboptimal or even misleading interpretations. Thus, in order to analyze the variance and interactions between the detected key bigrams, we used them as variables in the subsequent analysis.
3.3 Multi-dimensional Analysis of consistent key features
To understand how the 32 bigrams resulting from the keyness analysis behave and interact, we conducted a Multi-dimensional Analysis (MDA, e.g. Biber Reference Biber1988, Berber Sardinha & Veirano Pinto Reference Berber Sardinha and Pinto2014) using them as variables: we conducted a factor analysis based on these key features, interpreted the resulting dimensions functionally, computed dimension scores and compared them across datasets to interpret the relationships between the dimensions and the type of constraint, register, and first/source language.
For the factor analysis, we used the functions found in the R package psych (Revelle Reference Revelle2018). Factor analysis provides a calculation of eigenvalues and a corresponding scree plot, which represents a standardized measure of the proportion of variance explained by a given factor. Having chosen the number of factors, we run the final analysis to obtain the factor loadings of each variable for each factor. Factor loadings are weights (ranging from −1 to 1) that reflect how much a given variable contributes to a given factor. As in many MDAs, we use a threshold level of 0.35 (absolute values) for factor loadings in order for a variable to be included in the functional interpretations and dimension score computations. For these computations, we followed the procedure described in Biber (Reference Biber1989:11) and standardized the variables’ normalized frequencies in terms of how many standard deviations the value is above or below the overall mean for that variable. We then computed the dimension scores for each dimension by adding (for each text block) the standarized values of the variables with positive loadings and subtracting those with negative loadings.
4. Results
4.1 Choosing the number of dimensions
The balanced, maximum-sized random sample used in the study consists of 64 text blocks of 12 subsets each, totaling 768 blocks. Before the actual factor analysis, we measured the factorability of the 32 included variables by means of the KMO measure of sampling adequacy (Kaiser Reference Kaiser1974). As this resulted in an overall value of 0.9 – considered by Kaiser (Reference Kaiser1974:35) as ‘marvelous’ – we felt confident proceeding with our analysis. The scree plot of the factor analysis (Figure 2) shows three factors with eigenvalues above 1, clearly distinct from the subsequent factors. Our final model is thus a rotated three-factor solution that allows for intercorrelated factors (with all factor correlations 0.37 or lower).

Figure 2. Scree plot of eigenvalues for dimensions.
4.2 Dimension 1: Clausal complexity and dialogue
The bigrams that load onto dimension 1 (see Table 2) portray various clause-level constructions that reflect two diverging phenomena: clausal elaboration/verbal complexity, and linguistic means of narration.Footnote 5 Complexity features include (potentially non-finite) adverbial clauses that act as modifiers, as seen in (1), and various non-finite clausal complements, like those in (2) and (3).
(1)
Table 2. POS bigrams loading onto dimension 1. The values in parentheses indicate that the bigram has a higher loading in another dimension.

(2)
(3)
The negatively loading bigrams can be seen to mirror this as they include different copular clauses, phrasal coordination and genitive modification, all of which reflect nominal complexity. In terms of the complexity taxonomy of Bulté & Housen (Reference Bulté, Housen, Housen, Kuiken and Vedder2012), these features can be said to reflect linguistic structure complexity – more specifically an interaction between formal and functional complexity. As examples (1)–(3) above show, these features portray structural complexity in terms of multiple morphological elements that interact with sentence, clause, and phrasal complexity (Bulté & Housen Reference Bulté, Housen, Housen, Kuiken and Vedder2012:27).
Paratactic coordination and proper noun subjects, as in (4), are used to switch between direct speech and narration, and pronominal subjects, in (5), and objects, in (6), are typical cohesive devices used in linear narration.
(4)
(5)
(6)
Cross-corpus comparison of the dimension scores reveals an interesting patterning. As seen in the text chunk groupings in Figures 3 (register), 4 (variety), and 5 (first/source language), the L1-narrative corpora have clearly positive mean scores (L1-narrative_pub: 5.7; L1-narrative_nonpub: 11.7), meaning that the features described above are typical for the said corpora. On the other hand, the F1-academic corpora (Figures 3 and 4) have clearly negative mean scores (L1-academic_pub: −5.3; L1-academic_nonpub: −3.6) meaning that the features are less typical. In other words, in L1-Finnish, narration elements and switches between direct and indirect speech are typical for narrative texts and atypical for academic texts. The mean score differences are clearly smaller in LT-corpora (LT-de_narrative: 4.8; LT-ru_narrative: 4.2 VS. LT-de_academic: −3.0; LT-ru_academic: 3.0) and missing from the L2-corpora (L2-de_narrative: −2.4; L2-ru_narrative: −2.8 VS. L2-de_academic: −6.0; L2-ru_academic: −6.3).

Figure 3. Scatterplot of dimensions 1 and 2 in relation to register. Each A represents a text block of academic text and each N a text block of narrative text.

Figure 4. Scatterplot of dimensions 1 and 2 in relation to variety. Each L1 represents a text block of non-translated Finnish as a first language, each L2 a text block of Finnish as a second language, and each LT a text block of translated Finnish.

Figure 5. Scatterplot of dimensions 1 and 2 in relation to first/source language. Each fi represents a text block of non-translated Finnish as a first language, each de a text block with German as the first/source language, and each ru a text block with Russian as the first/source language.
Our findings thus partly corroborate those of Kruger & van Rooy (Reference Kruger and van Rooy2018:235–236), who show that, in constrained English, reported speech often distinguishes non-native and native varieties. In a broader sense, this may in turn be related to the first dimension of most Multi-dimensional Analyses – involved versus informational production (e.g. Biber Reference Biber1988, Reference Biber2014). The difference is also related to non-finite verbal constructions, some of which are unique to Finnish, and have been studied under the unique item hypothesis (Eskola Reference Eskola2004). Besides their uniqueness, many of them are also morphosyntactically complex, and shown to distinguish even advanced L2-Finnish from L1-Finnish (Ivaska Reference Ivaska2014b, Ivaska & Siitonen Reference Ivaska and Siitonen2017b).
4.3 Dimension 2: Proper name explicitation
As seen in Table 3, most of the bigrams that load positively onto dimension 2 include proper nouns in various syntactic roles, such as pre- and post-verbal adjuncts in (7) below, phrasal coordination in (8), parts of compounds in (9), pre-nominal possessive modifiers in (10) and post-nominal modifiers in (11). This dimension is thus predominantly characterized by the use of proper nouns.
(7)
Table 3. POS bigrams loading onto dimension 2.

(8)
(9)
(10)
(11)
While there may be various factors influencing the use of proper nouns, it has been hypothesized (Baker Reference Baker, Baker, Francis and Tognini-Bonelli1993:243–244), and to a degree proved (Volansky et al. Reference Volansky, Ordan and Wintner2013), to distinguish translated and non-translated texts. Explicitation by means of proper nouns is in general supported by data patterning regarding dimension 2. As can be seen in the upper part of Figures 3, 4, and 5, positive mean scores for dimension 2 are almost exclusive to LT-corpora and L2-corpora. This could be due to the lack of gender distinctions in Finnish pronominal reference (where hän stands for both ‘he’ and ‘she’). However, earlier results do not support this hypothesis: according to Teitto (Reference Teitto2010:52), at least in narrative texts the differences are rather due to differences between dialogue and narration – and in narration proper names are actually more common in F1 than in FT. What is more, the patterning is not uniform, as the positive mean scores are dominated by three subcorpora (LT-ru_academic: 11.5; L2-ru_narrative: 6.3; L2-de_narrative: 3.6) while the rest of the subcorpora have mean scores close to 0 or even negative. In other words, while constrained Finnish may favour explicitation by means of proper nouns, and while this is probably not due to a single L1/SL or register, the observation cannot be generalized to all data subsets.
4.4 Dimension 3: Phrasal complexity
The bigrams that load onto dimension 3 (see Table 4) reflect two superficially diverging linguistic phenomena: non-prototypical clausal word order and noun phrase complexity. Pre-verbal non-core arguments like that illustrated in (12) below and direct objects like that in (13) contribute to the former, whereas pre- and post-nominal modification seen in (14) and (15), respectively – including non-finite verb constructions seen in (16) – as well as phrasal nominal coordination in (17) contribute to the latter.
Table 4. POS bigrams loading onto dimension 3. The values in parentheses indicate that the bigram has a higher loading in another dimension.

(12)
(13)
(14)
(15)
(16)
(17)
In terms the type of complexity seen here, examples (14)–(17) are all related to phrasal complexity, and in the case of (16) also to sentence complexity (Bulté & Housen Reference Bulté, Housen, Housen, Kuiken and Vedder2012:27). It is worth noting that many of the bigrams related to noun phrase complexity also load negatively on dimension 1 – which is in turn characterized by the interaction of morphological and syntactic complexity.
The mean score distribution for dimension 3 reveals a clear pattern. As seen in Figures 6, 7, and 8, the upper and lower extremes of the y-axis are dominated by L1- and LT-corpora. Positive values characterize the academic register and negative values characterize the narrative register, meaning that the constructions described above are typical for academic texts in these varieties and atypical for narrative texts. As with dimension 1, the mean scores reflect a stronger tendency in L1 (L1-academic_pub: 7.5; L1-academic_nonpub: 7.4 vs. L1-narrative_pub: −4.4; L1-narrative_nonpub: −6.9) than in LT (LT-de_academic: 5.0; LT-ru_academic: 4.5 vs. LT-de_narrative: −5.7; LT-ru_narrative: −5.6). The L2-corpora occupy the middle ground, indicating that the features do not follow any register-related patterning in these subcorpora (L2-de_academic: −0.9; L2-ru_academic: 1.8 vs. L2-de_narrative: −2.0; L2-ru_narrative: −0.7).
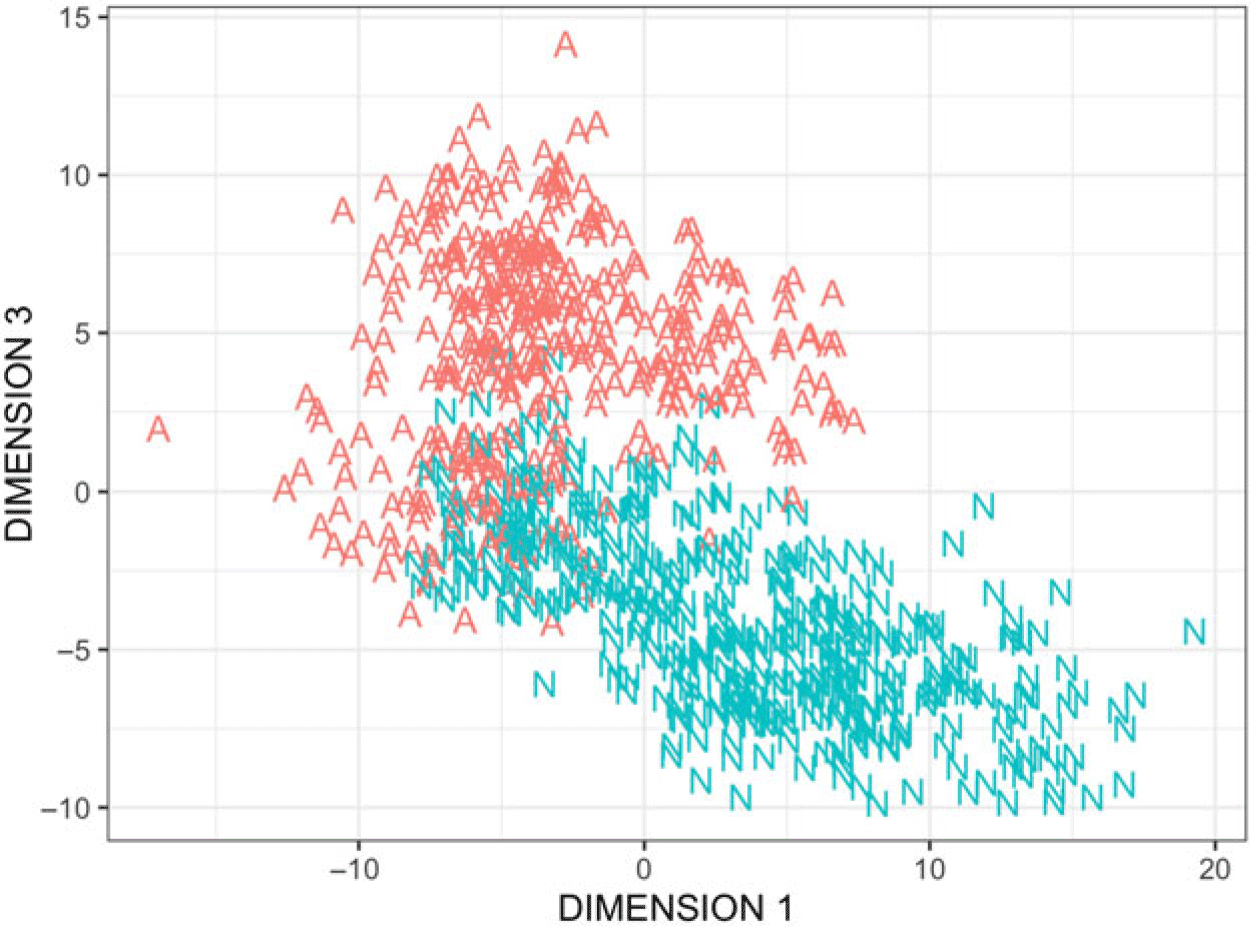
Figure 6. Scatterplot of dimensions 1 and 3 in relation to register. Each A represents a text block of academic text and each N a text block of narrative text.

Figure 7. Scatterplot of dimensions 1 and 3 in relation to variety. Each L1 represents a text block of non-translated Finnish as a first language, each L2 a text block of Finnish as a second language, and each LT a text block of translated Finnish.

Figure 8. Scatterplot of dimensions 1 and 3 in relation to first/source language. Each fi represents a text block of non-translated Finnish as a first language, each de a text block with German as the first/source language, and each ru a text block with Russian as the first/source language.
The pre-verbal nominal arguments are dominated by two constructions: active voice clauses where the non-prototypical word order is used as a cohesive device, as in (12) above; and passive voice constructions, where the pre-verbal positioning is prototypical, as in (13) above. Earlier research has identified the lesser use of non-prototypical word order as one of the complexity features that distinguish even advanced F2 from F1 (Ivaska Reference Ivaska2014b:177–179), and the relative frequency of the passive has also been shown to correlate with proficiency (Seilonen Reference Seilonen2013:58–59). Noun phrase complexity, then, may be related to another tendency found earlier – that post-verbal noun phrases in F2 are often less complex than those in F1 (Ivaska Reference Ivaska2014b:174–176). In other words, the two superficially diverging phenomena are probably intertwined, and both may ultimately be related to noun phrase complexity.
The present results corroborate earlier findings, showing that differences are closely related to register typicalities. Interestingly, greater use of nouns and noun phrase complexity are among the features distinguishing involved vs. informational production in many MDA-based studies of variation across registers (Biber Reference Biber2014), as well as a feature that differentiates constrained from non-constrained English (Kruger & van Rooy Reference Kruger and van Rooy2018:229–231)
5. Discussion and conclusion
We identified 32 syntactically defined POS bigrams as potentially relevant features in distinguishing constrained from non-constrained Finnish. In a Multi-dimensional Analysis, these bigrams formed three quantitatively distinct, functionally meaningful groups across datasets, indicating that verbal/clausal complexity, noun phrase complexity, and proper noun explicitation distinguish constrained from non-constrained Finnish across first/source languages and registers.
In the case of both verbal/clausal and noun phrase complexity, the direction of this relationship is not linear and uniform, but rather register-dependent. In non-constrained Finnish, the narrative register portrays higher verbal/clausal complexity than the academic register, whereas the academic register features more nominal complexity. The constrained varieties portray smaller differences: Finnish as a second language is generally characterized by lower verbal/clausal complexity, allowing one to group both registers together with non-constrained academic data. Translated Finnish, in turn, reflects the same distinction but to a lesser degree. As for noun phrase complexity, in non-constrained Finnish the academic register is more complex than the narrative register. Translated Finnish, again, reflects the same distinction but to a lesser degree, and Finnish as a second language positions itself in the middle of this continuum, with virtually no difference between the registers. Interestingly, while the distinction between these two dimensions is often related to the distinction between speaking and writing, some of the non-finite constructions included in this study – while used for reporting speech – are actually less common in spoken than in written Finnish (VISK: §538). Thus, the difference is due to the literary means for reporting spoken language rather than to spoken language as such, and the difference is not related to real-time production constraints but rather to the way they are syntactically mimicked in writing. The use of proper nouns does not show any clear register effects: it simply characterizes some of the constrained datasets where proper nouns are relatively more abundant than in the rest of the data.
Many of the constructions identified as verbally/clausally complex have been studied earlier in translated Finnish under the unique item hypothesis (Eskola Reference Eskola2004). Our results corroborate the hypothesis and suggest that it might apply to constrained language use in general. Similarly, noun phrase complexity has earlier been shown to distinguish the production of even advanced Finnish as a second language users from comparable first language users (Ivaska Reference Ivaska2014b). Our results confirm this observation and lend partial support to extending it to other forms of constrained language use.
Beyond Finnish, it is noteworthy that similar features have been identified as distinguishing constrained from non-constrained language use in English, too (Kruger & van Rooy Reference Kruger and van Rooy2016), and shown to correspond to proficiency in written informational registers in English (Biber, Gray & Staples Reference Biber, Gray and Staples2016). In both verbal/clausal and nominal complexity, the constrained varieties portray less inter-register variation than non-constrained data, which at the surface level supports the levelling out hypothesis (Baker Reference Baker and Somers1996). However, as suggested by Szymor (Reference Szymor2018), this may rather be a general usage-based mechanism, whereby constrained language use relies on models from registers with which the language users are more familiar. In terms of Bulté & Housen’s (Reference Bulté, Housen, Housen, Kuiken and Vedder2012) SLA complexity taxonomy, our results reflect a register-related interaction between morphological and syntactic complexity. Our results could thus be used both as a point of departure for a more focused complexity study regarding constrained Finnish, and as a potential direction for interpretation of constrainedness effects in other languages. Finally, the use of proper nouns has been suggested to reflect explicitation in translation (Baker Reference Baker, Baker, Francis and Tognini-Bonelli1993). As in Volansky et al. (Reference Volansky, Ordan and Wintner2013), our results lend partial support to the hypothesis, and extend it to constrained language in general: the difference is clear in some constrained datasets, but non-existent in others, suggesting that the use of proper nouns may be more sensitive to factors like topical variation rather than constrainedness.
Looking back to the three key theoretical constructs – varieties, CLI and registers – our results highlight their inherently intertwined nature. The most consistent quantitative differences between constrained and non-constrained varieties are indeed related to how they portray register typicalities. Both Finnish as a second language and translated Finnish show smaller differences between academic and narrative writing than non-translated first language Finnish. Still, the constrained varieties also diverge from each other, as the translated variety typically positions itself between the first and the second language varieties – corroborating the results of Kruger & van Rooy (Reference Kruger and van Rooy2016). Overall, our results clearly underline the centrality of register sensitivity in both language teaching and translator training and practice.
The present analysis only included two registers, and so the results are obviously shaped by their peculiarities. For example, switching between direct and indirect speech can be considered a peculiarity of narrative writing and, consequently, the inclusion of multiple registers could tease it apart from verbal complexity. However, with a topic as complex as constrained language use, one has to balance between what is desired and what is viable. Identifying and compiling the data needed even for the present design was highly challenging; including even one more register and keeping all the other variables unchanged would have required a minimum of five new data subsets.
The stepwise data-driven methodological procedure first contrasted each constrained data subset to a closely comparable non-constrained counterpart and then used these results to reveal the consistent differences. In other words, we could make full use of the existing resources, and narrow them down to a balanced subsample only for the second phase. This procedure was adopted to maximize data comparability without losing the generalizability of the results, and to control for the influence of each theoretically motivated construct, all the while doing justice to the inherent variation of naturally occurring linguistic data. We would suggest that studies conducted on a wider range of registers and typologically different languages, adopting sound quantitative methods supported by qualitative interpretations, are essential in the search for confirmation of the constrained language hypothesis.
Acknowledgements
We would like to thank the anonymous reviewers for their valuable comments and suggestions. We are obviously fully responsible for any remaining shortcomings or inadequacies. Ilmari Ivaska’s work was partially funded by the Alfred Kordelin Foundation, for which we are grateful.
Appendix. POS bigrams contributing the most to distinguishing constrained and non-constrained texts
Consistency indicates the number of pairwise comparisons in which the different bigrams were deemed as important.

Corpora used in the study
CTF = Corpus of Translated Finnish
Mauranen, Anna. 2000. Strange strings in translated language: A study on corpora. In Maeve Olohan (ed.), Intercultural Faultlines: Research Models in Translation Studies, 119–141. Manchester: St Jerome Publishing.
FinDe = Contrastive Corpus of Finnish and German. http://urn.fi/urn:nbn:fi:lb-20140730137.
ICLFI = International Corpus of Learner Finnish
Jantunen, Jarmo. 2011. Kansainvälinen oppijansuomen korpus (ICLFI): typologia, taustamuuttujat ja annotointi [International Corpus of Learner Finnish (ICLFI): Typology, variables and annotation]. Lähivõrdlusi. Lähivertailuja 21, 86–105.
InterCorp = InterCorp
Čermák, František & Alexandr Rosen. 2012. The case of InterCorp, a multilingual parallel corpus. International Journal of Corpus Linguistics 17(3), 411–427.
LAS1 = Corpus of Academic Finnish. https://www.utu.fi/en/university/faculty-of-humanities/finnish-and-finno-ugric-languages/syntax-archive.
LAS2 = The Corpus of Advanced Learner Finnish
Ivaska, Ilmari. 2014. The Corpus of Advanced Learner Finnish (LAS2): Database and toolkit to study academic learner Finnish. Apples: Journal of Applied Language Studies 8(3), 21–38.