History and Importance of Herbicide Resistance
As the most effective tool in the weed management toolbox, herbicides have been widely used in agriculture since their discovery in the 1940s. However, overreliance on herbicides resulted in selection of plant traits enabling weeds to survive herbicide treatments. A low number of resistant (R) biotypes preexist within a field population of weed species due to the natural variation caused by random mutations. This was confirmed in an old herbarium sample of blackgrass (Alopecurus myosuroides Huds.) collected in 1888 that possesses a single-nucleotide polymorphism at Ile-1781 of an acetyl-CoA carboxylase (ACCase) gene known to confer resistance to ACCase herbicides; that is, a resistance trait existed at least 100 yr before the introduction of the first ACCase herbicide (Délye et al. Reference Délye, Deulvot and Chauvel2013a). R biotypes are selected when exposed to a herbicide due to a positive fitness trait (Figure 1A). Resistance to herbicides was predicted more than six decades ago (Harper Reference Harper1956), but warnings and reports (e.g., Hilton Reference Hilton1957; Switzer Reference Switzer1957; Whitehead and Switzer Reference Whitehead and Switzer1963) were ignored until a mutation in the D1 protein of photosystem II leading to resistance of common groundsel (Senecio vulgaris L.) to simazine and atrazine was reported in 1970 (Rao Reference Rao2014; Ryan Reference Ryan1970). Now, more than 490 unique cases of herbicide-resistant plant populations have been detected in 70 countries (Heap Reference Heap2018), and herbicide resistance is a challenge to modern agricultural systems, because herbicide-resistant weed species can cause crop yield reduction, crop price reduction, reductions in the value of agricultural land, and additional costs for alternative control methods (Norsworthy et al. Reference Norsworthy, Ward, Shaw, Llewellyn, Nichols, Webster, Bradley, Frisvold, Powles, Burgos, Witt and Barrett2012).
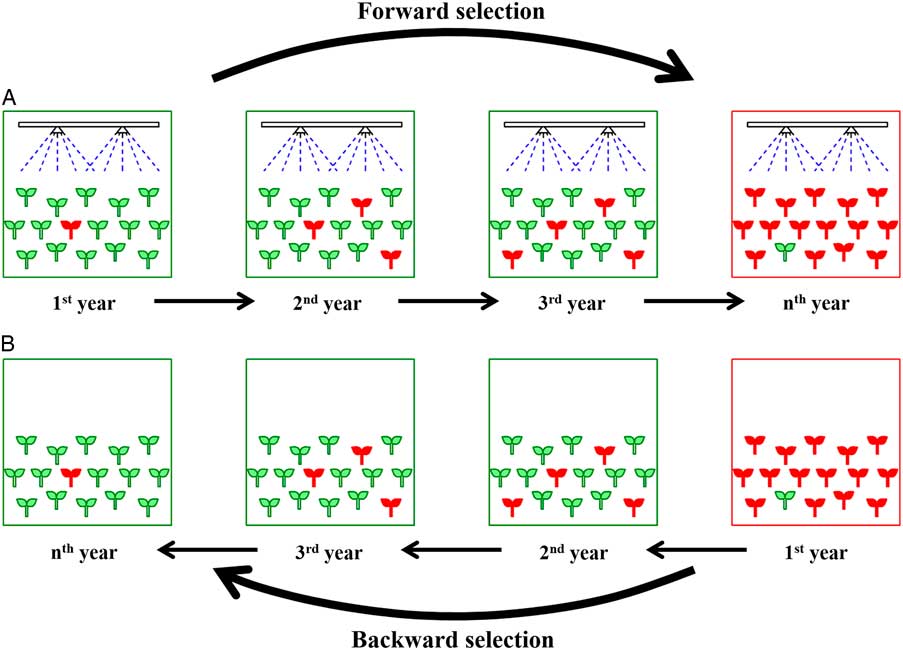
Figure 1 Balance between positive fitness (A) in the presence of selection pressure (herbicide) and negative fitness (B) in the absence of selection pressure. (A) Development of herbicide resistance occurs where resistant (R) biotypes (red symbols) are selected due to positive fitness in the presence of herbicide application for several years. (B) Susceptible biotypes (green symbols) might be selected due to negative fitness of R biotypes after cessation of herbicide application. According to classical population genetics, the forward selection may occur faster than the backward selection, as application of herbicide represents a severe selection pressure.
Proactive management of herbicide resistance by implementing additional agronomic measures (e.g., soil management, crop rotations) or chemical measures (e.g., mode-of-action rotation, using mixtures or sequential treatments), especially when cultivating herbicide-tolerant crops, is often not perceived as economical by farmers, at least in the short term. Long-term models are needed to illustrate the economic value of integrated weed management (IWM) (Powles and Gaines Reference Powles and Gaines2016).
Although herbicide resistance has attracted significant attention in recent years, the emergence of herbicide-resistant weed species has continued to increase (Figure 2) (Heap Reference Heap2018), and it has been argued that it is a wicked problem with no easy solution (Shaw Reference Shaw2016). Weed scientists have claimed that there are no clear reasons for the development of herbicide resistance (Barrett et al. Reference Barrett, Soteres and Shaw2016); however, overreliance on herbicides, especially herbicides belonging to the same chemical class or targeting the same site of action, is a major contributor (Powles and Gaines Reference Powles and Gaines2016). Additionally, the focus on confirmation of resistance and characterization of resistance mechanisms rather than on weed resistance management has failed to mitigate the problem (Neve Reference Neve2007).

Figure 2 Cumulative increase of herbicide-resistant weed species around the world. The first herbicide-resistant case was reported in 1957, and now more than 490 unique cases have been detected in 70 countries (Heap Reference Heap2018).
Best management practices (BMPs) were suggested by Norsworthy et al. (Reference Norsworthy, Ward, Shaw, Llewellyn, Nichols, Webster, Bradley, Frisvold, Powles, Burgos, Witt and Barrett2012) as a means to overcome herbicide resistance. One of the BMPs is knowledge about biology and ecology, including fitness, of the weed species. Fitness has been defined as the number of offspring an R genotype within a population produces relative to a nonresistant genotype. Fitness is not a fixed value; rather, it is determined within a particular environment or suite of ecological conditions and is influenced by the success of other phenotypes that exist in the same population (Radosevich et al. Reference Radosevich, Holt and Ghersa2007). Fitness is composed of many plant traits such as seed germination, dormancy, phenology, establishment, growth rate, pollination, seed size, seed yield per plant, and biomass production, all of which favor the prevalence of a given genotype.
As predicted by ecological and evolutionary theories, it is generally expected that some herbicide-resistant species will be less fit than the wild type in the absence of herbicide (Lehnhoff et al. Reference Lehnhoff, Keith, Dyer and Menalled2013a; Vila-Aiub et al. Reference Vila-Aiub, Neve and Powles2009b). On the other hand, herbicide resistance can be associated with a fitness cost, also called resistance cost. The fitness cost is caused by negative pleiotropic effects of resistance alleles (Vila-Aiub et al. Reference Vila-Aiub, Gundel and Preston2015). According to this evolutionary theory, in the absence of a herbicide, backward selection can occur (Cousens et al. Reference Cousens, Gill and Speijers1997). This means that without herbicide applications, the susceptible (S) individuals, as the fittest biotypes, may eventually dominate the field (Figure 1B), which will be in line with the aim of herbicide-resistance management strategies. It should be noticed that in practice, using small fitness differences to manage R plants may not be acceptable to farmers. However, the small fitness differences can be magnified by agronomic practices and can be used as one of multiple IWM components.
The hypothesis that herbicide resistance is often associated with a fitness cost has been clearly confirmed for triazine-resistant weed biotypes possessing the Gly-264 psbA gene (Gronwald Reference Gronwald1994). However, this idea was challenged recently (Darmency et al. Reference Darmency, Menchari, Le Corre and Délye2014), as a positive fitness was observed in a sethoxydim-resistant green foxtail millet [Setaria viridis (L.) P. Beauv×Setaria italica (L.) P. Beauv.] (Wang et al. Reference Wang, Picard, Tian and Darmency2010) and a glyphosate-resistant crop–wild hybrid (Oryza sativa L.×Oryza rufipogon Griff.) (Yang et al. Reference Yang, Li, Jiang, Wang, Cai, Su, Wang and Lu2017). Also, no resistance costs were detected with glyphosate-resistant Palmer amaranth (Amaranthus palmeri S. Watson) (Giacomini et al. Reference Giacomini, Westra and Ward2014; Vila-Aiub et al. Reference Vila-Aiub, Goh, Gaines, Han, Busi, Yu and Powles2014), so clearly, different resistance alleles and genes have different fitness implications. It is also believed that fitness costs associated with one physiological mechanism of resistance may be less than those resulting from two or more mechanisms in the same plant (Yu and Powles Reference Yu and Powles2014). Hence, resistance fitness costs are not general in all resistance cases, and it is essential to measure the fitness cost(s) of each herbicide-resistance case (Lehnhoff et al. Reference Lehnhoff, Keith, Dyer, Peterson and Menalled2013b).
A main reason for determining fitness cost is to develop proper tools for the management of herbicide-resistant weed species (Cousens and Fournier-Level Reference Cousens and Fournier-Level2018). Measuring and quantifying herbicide-resistance cost is also fundamental to predicting the frequency of R and S plants under various environmental conditions. Fitness cost has been studied for many years; however, improper experimental designs have led to misinterpretation of the results (Neve Reference Neve2007). Fortunately, in the last two decades, valuable knowledge has been provided (Bergelson and Purrington Reference Bergelson and Purrington1996; Cousens et al. Reference Cousens, Gill and Speijers1997; Vila-Aiub et al. Reference Vila-Aiub, Neve and Powles2009b), allowing scientists to study fitness of herbicide-resistant plants properly. However, we think the guidelines need to be reconsidered from a herbicide-resistance management point of view. In this review, we will highlight the inherent challenges associated with fitness cost studies and discuss previously published experimental designs (Vila-Aiub et al. Reference Vila-Aiub, Neve and Powles2009b, Reference Vila-Aiub, Neve and Roux2011, Reference Vila-Aiub, Gundel and Preston2015) in the context of the BMPs suggested by Norsworthy et al. (Reference Norsworthy, Ward, Shaw, Llewellyn, Nichols, Webster, Bradley, Frisvold, Powles, Burgos, Witt and Barrett2012). We postulate that fitness studies in weed science may require different approaches from those of other disciplines such as plant breeding, evolutionary ecology, and genetics.
Fitness Cost Studies Associated with Inherent Challenges
Due to the difficulties inherent in determining the expression and degree of fitness costs (Cousens and Fournier-Level Reference Cousens and Fournier-Level2018; Vila-Aiub et al. Reference Vila-Aiub, Gundel and Preston2015), it is often challenging to accurately estimate the fitness penalty endowed by pesticide-resistance alleles (Roux et al. Reference Roux, Giancola, Durand and Reboud2006). Consequently, many studies evaluating fitness were not properly designed, and the outcomes were vague and often meaningless (Bergelson and Purrington Reference Bergelson and Purrington1996; Neve Reference Neve2007; Vila-Aiub et al. Reference Vila-Aiub, Neve and Powles2009b, Reference Vila-Aiub, Gundel and Preston2015).The three main reasons for flawed fitness studies are: lack of homogeneity in the genetic background of plants, failure to study multigenerational fitness, and measuring fitness under noncompetitive conditions (Vila-Aiub et al. Reference Vila-Aiub, Gundel and Preston2015). Ways to overcome these weaknesses have been proposed elsewhere (Vila-Aiub et al. Reference Vila-Aiub, Neve and Powles2009b, Reference Vila-Aiub, Gundel and Preston2015). In a review, Vila-Aiub et al. (Reference Vila-Aiub, Neve and Powles2009b) listed five experimental factors to consider when measuring herbicide-resistance fitness costs: (1) ensuring the homogeneity of genetic background, (2) understanding the biochemical/molecular basis of herbicide resistance, (3) life-history traits, (4) competition for resources, and (5) environmental gradient. Incorporating these five factors can lead to accurate results and ease of interpretation. In practice, however, designing experiments that take into consideration all these factors may not be practical. Importantly, it may not be necessary to consider all experimental factors in all fitness studies, especially when the aim of the fitness study is to apply the results in herbicide-resistance management programs (Keshtkar et al. Reference Keshtkar, Mathiassen, Beffa and Kudsk2017a). Thus, before considering the the five factors, it is appropriate to clarify the aim of study.
In this context, it is noteworthy that there is no consensus among scientists such as plant breeders, geneticists/evolutionary ecologists, and weed scientists on how to study fitness. Plant breeders, dealing with herbicide-tolerant crops, often look for effects on fecundity (i.e., yield reduction) that might be associated with gene(s) inserted into crops, while evolutionary ecologists, dealing with dynamics of weed populations, are more concerned about the ratio of S and R alleles within a population or among populations over several generations. However, weed scientists, who deal with the management of herbicide-resistant weeds, want to find differences in traits between S and R plants collected within a field and exploit these differences in management strategies. We believe that to design effective herbicide-resistance management strategies, not only is negative fitness of R plants of relevance, as was stressed by Cousens et al. (Reference Cousens, Gill and Speijers1997), but positive fitness can also be exploited. For instance, a rigid ryegrass (Lolium rigidum Gaudin) population possessing the Ile-1781-Leu ACCase mutation showed seed dormancy in the absence of light, but this was not considered an intrinsic fitness cost (Vila-Aiub et al. Reference Vila-Aiub, Neve and Powles2009b). However, from an ecological point of view, seed dormancy is a positive fitness trait, because it is a common biological characteristic of many weeds that allows them to be successful and survive in nature. Seed dormancy in the aforementioned ACCase-target-site resistance (TSR) L. rigidum population can be broken when seeds receive enough light. Consequently, seed burial through cultivation was suggested as an agronomic practice to inhibit the seedling emergence of the R plants and favor the corresponding S phenotype (Vila-Aiub et al. Reference Vila-Aiub, Neve, Steadman and Powles2005). From a management point of view, it makes no difference whether the differences in plant traits are related to the resistance gene(s) or are due to a linkage between the resistance gene(s) and another non-resistance gene(s). In practice, the differences can be used to suppress R plants.
Approaches to Control the Genetic Background of Plant Materials
The genetic background can affect the cost of resistance (Bergelson Reference Bergelson1994; Bergelson and Purrington Reference Bergelson and Purrington1996; Giacomini et al. Reference Giacomini, Westra and Ward2014; Paris et al. Reference Paris, Roux, Berard and Reboud2008), but many fitness studies failed to ensure the homogeneity of the genetic background of their populations (Vila-Aiub et al. Reference Vila-Aiub, Neve and Powles2009b, Reference Vila-Aiub, Neve and Roux2011, Reference Vila-Aiub, Gundel and Preston2015). In most of the herbicide-resistance studies, R and S populations originated from different geographical locations (Keshtkar et al. Reference Keshtkar, Mathiassen, Beffa and Kudsk2017a). Because genetic differentiation is a common feature of weed populations adapted to different growing environments, such studies do not lead to scientifically meaningful results, because it is possible that R and S populations are dissimilar in other loci than the resistance locus/loci (Keshtkar et al. Reference Keshtkar, Mathiassen, Beffa and Kudsk2017a). Also, the results cannot be applied in resistance management programs, because the R and S populations originated in different geographical locations and very likely were adapted to different environmental conditions. Thus, improving the genetic background homogeneity of the plant material is vital in fitness studies. Fortunately, various experimental protocols such as single population, pedigreed lines, segregating population, multiple populations, multiple segregating crosses, near-isogenic lines (NILs), and transgenic lines can be used to improve the genetic backgrounds of the populations (Strauss et al. Reference Strauss, Rudgers, Lau and Irwin2002; Vila-Aiub et al. Reference Vila-Aiub, Neve and Roux2011). It should be stressed that it is possible to combine some of these methods to improve genetic background and produce more reliable results. For example, Martin et al. (Reference Martin, Benedict, Sauder, Wei, da Costa, Hall and Beckie2017) evaluated fitness costs in six populations of glyphosate-resistant kochia [Bassia scoparia (L.) A. J. Scott] (i.e., multiple populations approach), with R and S individuals selected within each population (i.e., single population approach) and then reciprocally crossed within each population (segregating population approach).
Notwithstanding the importance of this issue, it should be mentioned that some of the proposed methods for controlling genetic background are difficult to implement in herbicide-resistance studies, as discussed in the following sections. Accordingly, the method to control genetic background should be selected carefully based on the objectives of the planned study (Vila-Aiub et al. Reference Vila-Aiub, Neve and Roux2011).
NILs and Transgenic Lines
The NILs and transgenic lines approaches eliminate the effects of genetic background through introgression of a R allele into S plants; however, these methods are very time-consuming, and producing the NILs and transgenic lines can take up to 6 to 7 and 3 to 4 generations, respectively (Vila-Aiub et al. Reference Vila-Aiub, Neve and Roux2011, Reference Vila-Aiub, Gundel and Preston2015). Such a time expenditure may not be worthwhile if the objective is to develop resistance management programs. More importantly, the artificially obtained herbicide-resistant weeds (i.e., transgenic lines) have not been adapted to natural field conditions, so the results cannot be extrapolated to field-evolved R populations (Lehnhoff et al. Reference Lehnhoff, Keith, Dyer, Peterson and Menalled2013b; Vila-Aiub et al. Reference Vila-Aiub, Neve and Powles2009b). In vitro rescue required in the transgenic approach can lead to somaclonal variation in plant traits. The transgenic method is also an expensive approach with ecological risk of transgene escape and the generation of super-weed species. It is often difficult to employ the NIL method in weed species, as creation of NILs requires cross-pollination of individuals (Vila-Aiub et al. Reference Vila-Aiub, Gundel and Preston2015), and flower emasculation of weeds might not be an easy task. Like the NIL approach, the transgenic method is often difficult in weed species, as in vitro callus induction and plant regeneration from callus in weeds might not be as easy as in crop species due to lack of available techniques for wild species. Moreover, even if it were easy to create R and S isogenic lines (i.e., to have a fully controlled genetic background using these approaches), it cannot simply be claimed that the fitness costs are due to the resistance gene, because the expression of the genome close to the position of an inserted gene can be affected by insertion mutations, that is, there are positional effects of gene insertion in transgenic plants (Purrington and Bergelson Reference Purrington and Bergelson1999; Vila-Aiub et al. Reference Vila-Aiub, Gundel and Preston2015). Moreover, due to the possible linkage between herbicide-resistance gene(s) and other unknown gene(s), all the fitness costs cannot just be attributed to the allele(s) endowing resistance (Bergelson and Purrington Reference Bergelson and Purrington1996; Delye et al. 2013; Vila-Aiub et al. Reference Vila-Aiub, Gundel and Preston2015). As genetic diversity is common in weed field populations, we recommend including NILs or transgenic lines with different genetic backgrounds to study the interaction of genetic background and resistance genes. To obtain accurate results, it would be better to select R and S plants within a field, because the latter two issues (i.e., positional effects of transgene insertion and associated gene linkage) may not be important when the aim of fitness study is to find differences between R and S plants and employ them as a management tool in a particular field. NILs and transgenic lines are appropriate for self-pollinated weeds, but they, especially NILs, are technically difficult in cross-pollinated plants.
Segregating Population, Multiple Segregation Crosses, and Multiple Populations
In both the segregating population and segregation crosses methods, R and S plants are crossed, and the only difference between the two methods is the number of crossings. In the segregating population method, one R and one S population are crossed (1R×1S), (e.g., Babineau et al. Reference Babineau, Mathiassen, Kristensen and Kudsk2017b; Gassmann Reference Gassmann2005; Gassmann and Futuyma Reference Gassmann and Futuyma2005; Giacomini et al. Reference Giacomini, Westra and Ward2014; Jordan Reference Jordan1996; Vila-Aiub et al. Reference Vila-Aiub, Goh, Gaines, Han, Busi, Yu and Powles2014), while in the multiple segregation crosses method, one R population is crossed with many S populations (1R×nS) (e.g., Paris et al. Reference Paris, Roux, Berard and Reboud2008). In the multiple populations protocol, several field-collected populations of each R and S weed species are compared (e.g., Cousens et al. Reference Cousens, Gill and Speijers1997).
Even though multiple populations is a fast method, it makes use of R and S plants from different fields and therefore does not minimize genetic background variation (Vila-Aiub et al. Reference Vila-Aiub, Gundel and Preston2015), while segregating population and multiple segregation crosses do. The segregating population and multiple segregation crosses methods are also more time-consuming (2 to 3 generations) than the multiple population method (zero generation). In the multiple segregation crosses method, we propose, if possible, to include several R plants instead of just one.
Regardless of their significant power to detect fitness cost of the resistance allele(s), the segregating population, segregation crosses, and multiple populations protocols may not be applicable and useful when the final objective is the management of a specific weed population in a specific field. Fitness costs of a specific resistance gene may depend on the genetic background. For instance, in a multiple segregation crosses study, it was shown that fitness costs associated with the resistance allele axr1-3, endowing resistance to 2,4-D herbicide, varied between eight different mouse-ear cress [Arabidopsis thaliana (L.) Heynh.] accessions with different genetic backgrounds (Paris et al. Reference Paris, Roux, Berard and Reboud2008). In the previously mentioned study by Martin et al. (Reference Martin, Benedict, Sauder, Wei, da Costa, Hall and Beckie2017), the fitness cost of glyphosate resistance, endowed by 5-enolpyruvoylshikimate-3-phosphate synthase (EPSPS) gene amplification, in six B. scoparia populations was not constant across populations. Also, fitness costs differed between populations of glyphosate-resistant tall morningglory [Ipomoea purpurea (L.) Roth] when the multiple population protocol was used (Van Etten et al. Reference Van Etten, Kuester, Chang and Baucom2016). In another study using the multiple populations protocol, inconsistent fitness differences between ACCase-TSR and S sterile oat (Avena sterilis L.) populations were detected and attributed to non-resistance loci selected in different geographical locations (Papapanagiotou et al. Reference Papapanagiotou, Paresidou, Kaloumenos and Eleftherohorinos2015). Similarly, no consistent variation in traits of six glyphosate-resistant and four glyphosate-susceptible populations of A. palmeri were found, and the differences among populations were attributed to cropping system components, including crop rotation and crop canopy structure (Bravo et al. Reference Bravo, Leon, Ferrell, Mulvaney and Wood2017). These robust varietal differences point out the importance of case-by-case fitness assessment of herbicide-resistant weed species within each field.
It is difficult to apply the segregating population and multiple segregation crosses protocols to self-pollinated weed species, especially those having small flowers. An additional problem associated with the multiple population protocol is that, in practice, it might be impossible to select several field-collected R populations when weeds are at the early stages of evolution of resistance. Moreover, if it were possible to collect several R populations in a region at the early stages of resistance evolution, it would be unlikely that the collected R populations possess identical resistance alleles, which is an essential requirement for this protocol (Vila-Aiub et al. Reference Vila-Aiub, Neve and Roux2011). Nonetheless, Tardif et al. (Reference Tardif, Rajcan and Costea2006) used this method successfully, collecting six S and six R Powell amaranth (Amaranthus powellii S.Watson) populations carrying a Trp-574-Leu acetolactate synthase (ALS) mutation in Ontario, Canada. The first ALS-resistant A. powellii population was collected in Ontario in 1997 (Ferguson et al. Reference Ferguson, Hamill and Tardif2001) and reported in 1998 (Heap Reference Heap2018), while the fitness study was carried out in 2003 (Tardif et al. Reference Tardif, Rajcan and Costea2006), meaning that R plants were not collected at the early stages of resistance evolution.
As stressed earlier, the importance of controlling the genetic background of plant materials cannot be ignored. Genetic background of plant materials can either be “fully controlled” (almost identical) or “semicontrolled.” We propose that when the overall aim of a fitness study is to generate information needed to develop management strategies for herbicide-resistant species, minimizing the genetic background of R and S plants will be sufficient. In contrast, an identical genetic background in R and S plants is necessary when geneticists and evolutionary ecologists aim to track fitness cost of an exact allele. Minimizing the variation in the genetic background of plants can be done by selecting R and S populations from a population collected within a field, and this approach is very appropriate in studies where herbicide-resistance management programs are the final outcome.
Single Population and Pedigreed Lines Approaches
In both the single population and pedigreed lines approaches, plant materials (R and S plants) are collected within a field (Figures 3 and 4, Step I). Selecting accessions within the same habitat minimizes the variation within the plant material (Cousens et al. Reference Cousens, Gill and Speijers1997). To have a plant sample representing the field population, a large enough sample, considering the pollination behavior, should be collected (Burgos Reference Burgos2015; Burgos et al. Reference Burgos, Tranel, Streibig, Davis, Shaner, Norsworthy and Ritz2013). It was suggested that 20 to 40 plants should be sufficient for self-pollinated (autogamous) weed species, while only 5 to 10 plants are enough to make a bulk sample for cross-pollinated (allogamous) species (Burgos et al. Reference Burgos, Tranel, Streibig, Davis, Shaner, Norsworthy and Ritz2013). The time required for preparing plant material in both the single population and pedigreed lines methods is 2 yr/generations (Vila-Aiub et al. Reference Vila-Aiub, Neve and Roux2011); however, the time can be reduced by using early-detection methods such as the Syngenta Quick-Test (Syngenta Crop Protection, WST-149, Stein 4332, Switzerland) to identify R and S plants (Boutsalis Reference Boutsalis2001).

Figure 3 Procedure for the single population approach. The method consists of four steps. Step I: Seeds are collected from many plants within a field to make a bulk-seed collection. Step II: Seeds are germinated and seedlings are grown in a greenhouse. Step III: The seedlings are cloned and labeled, then half of the clones are treated with herbicide to detect the resistant (R) and susceptible (S) plants, while the remaining clones are not treated. (Note: It is not unusual to have more than two groups, e.g., R, S, and semi-resistant [RS] individuals. In this case, the RS plants can be discarded [e.g., see Keshtkar et al. Reference Keshtkar, Mathiassen, Beffa and Kudsk2017a; Pedersen et al. Reference Pedersen, Neve, Andreasen and Powles2007; Yanniccari et al. Reference Yanniccari, Vila-Aiub, Istilart, Acciaresi and Castro2016].) Step IV: Finally, the untreated R and S plants are grown in isolated places for seed production. Notice, intermating among the field-collected seeds (Step I) for some generations can reduce the effect of variation in genetic background before Step II.

Figure 4 Procedure for the pedigreed lines approach. The method consists of four steps. Step I: Seed samples are collected from each individual plants and labeled as a family. Step II: Seeds from each sample are grown in isolated places for selfing and intermating within each family. Step III: Seeds of each family are divided two subsamples, half for measuring resistance level and half for measuring fitness components. Step IV: Correlation between resistance level and fitness level is tested, leading to three possible scenarios (positive, negative, and neutral fitness), with negative fitness more expected. Notice, intermating among the field-collected seeds (Step I) for some generations can reduce the effect of variation on genetic background before Step II.
In the single population approach, field-collected seeds are grown to produce seedlings (Figure 3, Step II), then clones of parental plants are produced through asexual reproduction methods (e.g., plant dividing) (Figure 3, Step III), as has been done for grass species (Fernández-Moreno et al. Reference Fernández-Moreno, Alcántara-de la Cruz, Smeda and De Prado2017; Keshtkar et al. Reference Keshtkar, Mathiassen, Beffa and Kudsk2017a, Reference Keshtkar, Mathiassen and Kudsk2017b; Panozzo et al. Reference Panozzo, Scarabel, Rosan and Sattin2017; Pedersen et al. Reference Pedersen, Neve, Andreasen and Powles2007; Vila-Aiub et al. Reference Vila-Aiub, Neve, Steadman and Powles2005; Yanniccari et al. Reference Yanniccari, Vila-Aiub, Istilart, Acciaresi and Castro2016). One group of the clones is subjected to a herbicide assay to detect R and S plants, while the parental plants are not treated with herbicide but are classified based on the response of the corresponding clones (Figure 3, Step III). It is not unusual to end up with more than two groups, that is, in addition to the R and S plants, a third group of semi-resistant (RS) individuals may be detected within a population. In this case, the RS plants are typically discarded (Keshtkar et al. Reference Keshtkar, Mathiassen, Beffa and Kudsk2017a; Pedersen et al. Reference Pedersen, Neve, Andreasen and Powles2007; Yanniccari et al. Reference Yanniccari, Vila-Aiub, Istilart, Acciaresi and Castro2016). Of course, it is possible to have several groups if different discriminating herbicide doses are applied (e.g., Yanniccari et al. Reference Yanniccari, Vila-Aiub, Istilart, Acciaresi and Castro2016). The parental R and S plants are grown for seed production in two isolated places to prevent cross-pollination between the R and S phenotypes (Figure 3, Step IV). Finally, the seeds from each parental R and S phenotype are used in fitness studies. This method can be used for plant species for which cloning is possible, such as annual grass and perennial species. Lack of an asexual reproduction system in plants can be overcome by other herbicide-resistance detection methods, such as chlorophyll fluorescence imaging (Ahrens et al. Reference Ahrens, Arntzen and Stoller1981; Jordan Reference Jordan1996; Kaiser et al. Reference Kaiser, Menegat and Gerhards2013; Wang et al. Reference Wang, Peteinatos, Li and Gerhards2016) and biochemical (Reade and Cobb Reference Reade and Cobb2002), molecular (Délye et al. Reference Délye, Duhoux, Pernin, Riggins and Tranel2015), and leaf disk assays (Shaner et al. Reference Shaner, Nadler-Hassar, Henry and Koger2005). We suggest these methods to be used in future fitness studies, because fast methods for detecting fitness differences between S and R plants are in demand.
Briefly, for the pedigreed lines approach, appropriate seeds are collected from individual plants within a field, and each sample, which is referred to as the “line” or “family,” is labeled (Figure 4, Step I). Before producing seeds from cross-pollinated plants, line/family selfing or intermating among family members for some generations can increase the homogeneity of genetic background for each of the so-called maternal lines/families (Figure 4, Step II). Note, seeds of a plant species with a self-pollinated breeding system represent an inbred line (Mallory-Smith et al. Reference Mallory-Smith, Hall and Burgos2015; Vila-Aiub et al. Reference Vila-Aiub, Neve and Roux2011). Then, half of the produced seeds from each family are grown and subjected to different doses of herbicide, while the corresponding plants are used for fitness studies (Figure 4, Step III). Typically, plant fitness components such as seed germination, dormancy, phenology, establishment, growth rate, pollination, seed size, seed yield per plant, biomass production, adaption with environment, competition with neighboring plants, and so on are measured (Holt Reference Holt1990; Warwick and Black Reference Warwick and Black1994 ). Finally, fitness costs can be calculated by fitting a regression model between resistance levels of each family and the measured level of fitness components, that is, the mean value of each fitness component for each family (Figure 4, Step IV) (e.g., Baucom and Mauricio Reference Baucom and Mauricio2004). It is a suitable method for reducing the variation in genetic background of cross-pollinated weed species having only sexual reproduction systems. For instance, for those annual broadleaf weed species (Figure 4) that do not have asexual reproduction methods, the pedigreed lines approach can be used to identify R and S plants in the absence of a cloning technique. However, while the pedigreed lines approach is an appropriate method for quantitative polygenic resistance (i.e., NTSR) and gene amplification herbicide-resistance mechanisms, it may not be appropriate for weeds possessing major monogenic resistance mechanisms (i.e., TSR). The reason is that the statistical correlation between independent variables (measured resistance levels) and dependent variables (measured fitness levels) of TSR may not be as robust as statistical correlations of the NTSR mechanism. It is noteworthy that in a case in which only one major gene controls resistance and results in two phenotypes (R or S), a t-test is an option. The number of independent variables for weeds possessing major monogenic resistance mechanisms might only be two (R and S), which is less than the number of independent variable for weeds carrying quantitative polygenic resistance, in which a range of responses between alive and dead (highly susceptible, moderately susceptible, susceptible, resistant, and highly resistant) may exist. Thus, choosing the appropriate method of controlling genetic background not only depends on the objectives of the study, but also on plant material characteristics, experimental facilities, and knowledge of the molecular basis of resistance (Vila-Aiub et al. Reference Vila-Aiub, Neve and Roux2011).
If practicable, we consider the single population and pedigreed lines approaches, with some modification, to be the most appropriate methods to employ in studies in which the aim is to generate information relevant for the management of herbicide-resistant species. The main reasons are that these two methods (1) require less time, (2) in practice, are easier to use than the other methods, and (3) allow the selection of R and S plants within a field population, that is, they are closer to practical conditions.
It should be noted that these methods only comprise one population and therefore they do not provide information on the interaction of genetic background and resistance gene(s). To overcome this, we propose applying these methods to several populations rather than only one population, and we rename them the “recurrent single population” and the “recurrent pedigreed lines approaches,” respectively. These proposed approaches also overcome the flaw of the multiple population protocol in minimizing genetic background of plants. Importantly, whenever no consistent results are found between populations, the results for each population can still be applied in a field-specific manner to manage herbicide resistance. Accordingly, we strongly suggest the recurrent single population and the recurrent pedigreed lines approaches be used in future fitness studies.
Molecular and Biochemical Basis of Herbicide Resistance
A detailed understanding of the biochemical and molecular basis of resistance is necessary to attribute detected pleiotropic effects to specific gene(s) and mutation(s) (Vila-Aiub et al. Reference Vila-Aiub, Neve and Powles2009b). However, the precise biochemical mechanism of action of some herbicides, such as auxinic herbicides (Group O/4), is not entirely understood today—more than six decades after their discovery (Christoffoleti et al. Reference Christoffoleti, Figueiredo, Peres, Nissen and Gaines2015; Jugulam et al. Reference Jugulam, DiMeo, Veldhuis, Walsh and Hall2013; Mithila et al. Reference Mithila, Hall, Johnson, Kelley and Riechers2011). Also, our understanding of the molecular mechanism of NTSR is yet in its early stages (Gaines et al. Reference Gaines, Lorentz, Figge, Herrmann, Maiwald, Ott, Han, Busi, Yu, Powles and Beffa2014; Ghanizadeh and Harrington Reference Ghanizadeh and Harrington2017), and no high-throughput technology has been developed to easily identify multiple genes endowing the NTSR mechanism. Moreover, NTSR might not only be caused by a mutation(s), but could also be of epigenetic origin, which might increase the complexity of the relationships between a fitness trait and resistance (Catarine et al. Reference Catarine, Ales, Ratna and Aldo2018; Gressel Reference Gressel2009). Even if it were possible to identify all resistance genes, it might be more complicated in field-collected weed samples, because weed populations consist of plants with different genotypes and different resistance patterns (Petit et al. Reference Petit, Duhieu, Boucansaud and Délye2010). In weed science, the experimental unit is often a plot, whereas individual plants are often considered the experimental unit in ecological studies (Bergelson and Purrington Reference Bergelson and Purrington1996). More importantly, as highlighted previously, the purpose of weed science studies is often the identification of differences between R and S plants with mitigation of herbicide resistance being the objective.
Studying and Determining Fitness Cost
Fitness costs of resistance alleles can be determined using two main methods: the direct and indirect methods (Roux et al. Reference Roux, Giancola, Durand and Reboud2006). The direct methods compares fitness components (i.e., seed germination, dormancy, phenology, establishment, growth rate, pollination, seed size, seed yield per plant, and biomass production) between R and S individuals (Roux et al. Reference Roux, Giancola, Durand and Reboud2006). In indirect methods, also called the “allele frequency approach,” changes in the frequency of R and S alleles over several generations in the absence of herbicide application are measured (Roux et al. Reference Roux, Camilleri, Berard and Reboud2005, Reference Roux, Giancola, Durand and Reboud2006; Vila-Aiub et al. Reference Vila-Aiub, Neve and Powles2009b, Reference Vila-Aiub, Gundel and Preston2015; Wang et al. Reference Wang, Picard, Tian and Darmency2010). As fitness studies conducted in controlled growth conditions (e.g., in a glasshouse or incubator) may not reveal the ecological fitness of resistance genes expressed under field conditions, running fitness experiments in the field under weed–weed or weed–crop competitions has been suggested (Ashigh and Tardif Reference Ashigh and Tardif2009; Neve Reference Neve2007; Vila-Aiub et al. Reference Vila-Aiub, Neve and Powles2009b). It has also been proposed that fitness be evaluated throughout the entire life cycle of plants (Vila-Aiub et al. Reference Vila-Aiub, Neve and Powles2009b). As was mentioned previously, lack of multigenerational fitness studies is one of the three main reasons for flawed results (Vila-Aiub et al. Reference Vila-Aiub, Gundel and Preston2015).
To evaluate the frequency of R and S alleles, two approaches, the multigenerational method (Collavo et al. Reference Collavo, Strek, Beffa and Sattin2013; Roux et al. Reference Roux, Camilleri, Berard and Reboud2005; Wakelin and Preston Reference Wakelin and Preston2006) and the cline method (Roux et al. Reference Roux, Giancola, Durand and Reboud2006), have been suggested in which the cost of resistance is estimated indirectly (Vila-Aiub et al. Reference Vila-Aiub, Neve and Powles2009b). These indirect methods are the only approaches that evaluate the complete life cycle (Roux et al. Reference Roux, Giancola, Durand and Reboud2006). Before indirect methods can be conducted, the genetic background of the plants must be controlled through one of or a combination of the abovementioned approaches (see the section Approaches to Control the Genetic Background of Plant Materials). In spite of their ability to detect fitness, indirect experimental methods are not popular in plant fitness studies (Roux et al. Reference Roux, Giancola, Durand and Reboud2006; Vila-Aiub et al. Reference Vila-Aiub, Neve and Roux2011) and may not be a suitable method for fitness studies when the aim is management of R populations. The reasons are provided in the following sections.
Herbicide-Resistant Gene Flow Threatens the Environment
Similar to herbicide outputs in the environment, the gene flow of herbicide-resistance traits could cause severe damage to the ecosystem. Conducting field experiments and allowing R plants to produce pollen can cause genetic pollution of the environment through herbicide-resistance gene flow. Herbicide-resistance gene flow is generally an overlooked issue in fitness studies. For example, gene flow via pollen was considered a factor in spreading resistance to neighboring fields (Babineau et al. Reference Babineau, Mathiassen, Kristensen, Holst, Beffa and Kudsk2017a; Busi et al. Reference Busi, Yu, Barrett-Lennard and Powles2008; Mallory-Smith et al. Reference Mallory-Smith, Hall and Burgos2015; Watrud et al. Reference Watrud, Lee, Fairbrother, Burdick, Reichman, Bollman, Storm, King and Van de Water2004). As an example, Délye et al. (Reference Délye, Clément, Pernin, Chauvel and Le Corre2010) clearly demonstrated that conventional fields having a low density of R weed could act as “genetic sink” and facilitate the dissemination of resistance genes to adjacent organic fields.
Two out of the 12 BMPs to mitigate herbicide resistance proposed by Norsworthy et al. (Reference Norsworthy, Ward, Shaw, Llewellyn, Nichols, Webster, Bradley, Frisvold, Powles, Burgos, Witt and Barrett2012) are related to preventing dispersal of herbicide-resistance traits between fields, within fields, and even from field borders to fields by plant reproductive organs such as pollen and seed. Infestation of an agroecosystem with herbicide-resistant weed species is a major threat to the sustainability of cropping systems, because alternative control methods are less effective and more expensive, leading to limited management options. The problem is more pronounced with NTSR, because herbicide-resistance pattern is unpredictable and resistance may include several modes of actions (Délye Reference Délye2013; Délye et al. Reference Délye, Gardin, Boucansaud, Chauvel and Petit2011, Reference Délye, Jasieniuk and Le Corre2013b; Powles and Yu Reference Powles and Yu2010).
Variations in Fitness Cost at Different Growth Stages
Herbicide resistance can affect many traits (Cousens and Fournier-Level Reference Cousens and Fournier-Level2018). The indirect methods do not allow identification of particular fitness traits of the R plant. Fitness costs may be expressed only at specific development stages but not during the entire plant life history (Boege et al. Reference Boege, Dirzo, Siemens and Brown2007; Vila-Aiub et al. Reference Vila-Aiub, Neve and Powles2009a). On the other hand, measuring fitness at a single plant growth stage is precarious, because it might be the wrong stage in terms of finding differences between R and S genotypes (Cousens and Fournier-Level Reference Cousens and Fournier-Level2018). Furthermore, fitness cost at a certain growth stage may not influence final fecundity, because the plant can compensate for a fitness penalty by expression or even overexpression of polymorphic loci not related to the resistance locus or loci. In a field experiment, there was no fitness cost in vegetative (biomass and plant height) and fecundity (seed production) stages of A. myosuroides plants carrying the Leu-1781 ACCase mutant (Menchari et al. Reference Menchari, Chauvel, Darmency and Délye2008), while in another experiment, the same R population had a delay in germination relative to wild-type plants (Délye et al. Reference Délye, Menchari, Michel, Cadet and Le Corre2013c).
Recently, it has been argued that measuring the differences between R and S plants in separate experiments should not necessarily be described as fitness cost, because fitness is determined by the number of offspring a genotype produces (Cousens and Fournier-Level Reference Cousens and Fournier-Level2018). Assessing plant fitness at different life-history stages can increase the potential for identifying fitness changes (Osipitan and Dille Reference Osipitan and Dille2017) and the most sensitive growth stages within the whole life cycle (Norsworthy et al. Reference Norsworthy, Ward, Shaw, Llewellyn, Nichols, Webster, Bradley, Frisvold, Powles, Burgos, Witt and Barrett2012). Because of the time and effort needed to do that, all fitness components are rarely studied in plant fitness studies (Younginger et al. Reference Younginger, Sirová, Cruzan and Ballhorn2017). Fortunately, several approaches, such as standard multivariate regression, path analysis, principal components analysis, factor analysis, and G-matrix, may allow us to measure fewer plant traits but those that contribute more to the final plant outcome (Conner and Hartl Reference Conner and Hartl2004). The G-matrix, a matrix of additive genetic variance–covariance, is the most appropriate method for studying the evolution of quantitative traits (Roff Reference Roff2000; Steppan et al. Reference Steppan, Phillips and Houle2002) and fitness components (Cousens and Fournier-Level Reference Cousens and Fournier-Level2018). The G-matrix summarizes the multivariate genetic relation of different traits (Aguirre et al. Reference Aguirre, Hine, McGuigan and Blows2013), improves our ability to predict how the phenotypic traits evolve by selection and drift (Arnold et al. Reference Arnold, Bürger, Hohenlohe, Ajie and Jones2008), and shows the contribution of each trait to fitness and genetic correlation of traits. Several traits may be affected by same set of genes, which is known as the pleotropic effect. Thus, selection for a trait would indirectly change other traits (Cousens and Fournier-Level Reference Cousens and Fournier-Level2018). If the G-matrix is constant for a long time, it could be used to predict the evolutionary potential of a population. For more information about the G-matrix, see Arnold et al. (Reference Arnold, Bürger, Hohenlohe, Ajie and Jones2008), Pigliucci (Reference Pigliucci2006), Roff (Reference Roff2000), Steppan et al. (Reference Steppan, Phillips and Houle2002), and Texas A&M University (2018).
Among all fitness components, seed production is a good proxy for determining fitness (Cousens and Fournier-Level Reference Cousens and Fournier-Level2018; Menchari et al. Reference Menchari, Chauvel, Darmency and Délye2008; Vila-Aiub et al. Reference Vila-Aiub, Neve and Powles2009b). However, measuring fecundity fitness for species shedding seeds over a long time is almost impossible. To overcome this in field fecundity fitness studies, an indirect method determining seed production was suggested and employed in previous studies (Keshtkar et al. Reference Keshtkar, Mathiassen and Kudsk2017b; Melander Reference Melander1995). Indeed, to prevent gene flow by both seed and pollen, it can be advantageous to terminate an experiment with cross-pollinating weed species after plant heading or flowering but before pollen spreading. It should be noted that indirect measurement of fecundity cannot isolate female and male fitness. Also, the number of seeds calculated through this method is often higher than the seed number collected in the direct seed production method. But we find that these flaws can be neglected when the aim of the fitness study is to develop management strategies, because there is usually a strong relationship between vegetative and reproductive production in plants (Weiner Reference Weiner2004; Weiner et al. Reference Weiner, Campbell, Pino and Echarte2009; Younginger et al. Reference Younginger, Sirová, Cruzan and Ballhorn2017).
Time-consuming Method
More importantly, the time for herbicide resistance to develop relative to the time needed to conduct a fitness study is another issue that must be considered. In some cases, herbicide resistance develops much faster than the time required for studying fitness costs over several generations. For instance, herbicide-resistant L. rigidum biotypes were selected in less than four generations by applying sublethal doses of ACCase herbicides (Manalil et al. Reference Manalil, Busi, Renton and Powles2011; Neve and Powles Reference Neve and Powles2005). Sometimes the frequency of resistance genes can stay similar to the frequency of the susceptible ones in the absence of herbicide application for several generations. In this case, no practical advice can be provided concerning the management of the R population, because potential fitness changes between R and S populations are absent. For example, resistance to ACCase inhibitors was present in an R L. rigidum population even 7 yr after the use of ACCase-inhibiting herbicides was abandoned (Collavo et al. Reference Collavo, Strek, Beffa and Sattin2013). Thus, from a herbicide-resistance management point of view, it may not be worth running time-consuming studies to test the fitness of a population if resistance can be developed faster than the time required for a fitness study, especially for weed species with a high tendency to develop resistance and similar fecundity fitness.
The recently introduced methods called “rapid-generation technologies” and “speed-breeding” (e.g., Mobini and Warkentin Reference Mobini and Warkentin2016; O’Connor et al. Reference O’Connor, Wright, Dieters, George, Hunter, Tatnell and Fleischfresser2013; Stetter et al. Reference Stetter, Zeitler, Steinhaus, Kroener, Biljecki and Schmid2016; Watson et al. Reference Watson, Ghosh, Williams, Cuddy, Simmonds, Rey, Asyraf Md Hatta, Hinchliffe, Steed, Reynolds, Adamski, Breakspear, Korolev, Rayner, Dixon, Riaz, Martin, Ryan, Edwards, Batley, Raman, Carter, Rogers, Domoney, Moore, Harwood, Nicholson, Dieters, DeLacy, Zhou, Uauy, Boden, Park, Wulff and Hickey2018), which allow plant breeders to have more generations per year in fully controlled growth chambers, may overcome the issue of time associated with a multigenerational method. However, these methods are still in their infancy and have not been adopted for any weed species, or even for many crop plants. In addition, it has been noted that fitness studies carried out in controlled environments may not represent field conditions (Ashigh and Tardif Reference Ashigh and Tardif2009; Neve Reference Neve2007; Vila-Aiub et al. Reference Vila-Aiub, Neve and Powles2009b). Accordingly, the speed breeding methods may not be useful if the objective is developing herbicide-resistance management programs.
No Easy and Simple Screening Method to Identify R and S Individuals
Frequency of R and S plants is determined by genotyping and phenotyping techniques. To this end, it is necessary to test many individual plants (e.g., several hundreds or thousands of plants), which is a limitation of the indirect methods (Vila-Aiub et al. Reference Vila-Aiub, Neve and Roux2011). Fortunately, the frequency of R and S plants within hundreds of samples can be done using high-throughput DNA-based assays (e.g., allele-specific PCR techniques) when the molecular mechanism of resistance is known (Burgos et al. Reference Burgos, Tranel, Streibig, Davis, Shaner, Norsworthy and Ritz2013; Délye et al. Reference Délye, Duhoux, Pernin, Riggins and Tranel2015). Unfortunately, the molecular mechanism of NTSR has yet to be elucidated (Ghanizadeh and Harrington Reference Ghanizadeh and Harrington2017). Therefore, DNA-based assays are limited to TSR mechanism (monogenic resistance) for certain herbicide target sites such as D1 protein, ALS, ACCase, EPSPS, phytoene desaturase, and protoporphyrinogen oxidase (Burgos et al. Reference Burgos, Tranel, Streibig, Davis, Shaner, Norsworthy and Ritz2013). Phenotyping TSR plants is usually possible and often easy through single-dose screen experiments, especially where resistance is endowed by a major gene in a diploid species. In contrast, in cases of multiple mechanisms, polygenic resistance (NTSR), and gene amplification, there is no simple, straightforward, or high-throughput method to screen plants (Mallory-Smith et al. Reference Mallory-Smith, Hall and Burgos2015) and classify them as S and R plants similar to monogenic resistance cases. Actually, in these circumstances, we may be faced with a range of individuals having different resistance levels ( i.e., being highly susceptible, moderately susceptible, susceptible, moderately resistant, resistant, highly resistant, etc.), as was reported in TSR-polyploid plants such as hexaploid wild oat (Avena fatua L.) (Yu et al. Reference Yu, Ahmad-Hamdani, Han, Christoffers and Powles2013). Due to the abovementioned reasons, indirect approaches may not be appropriate when the objective is management of resistance.
Computer Simulation Modeling: A Tool for Studying Fitness Costs
Modeling might be an alternative approach to predict the frequency of R and S phenotypes and evolution of herbicide resistance (Cousens and Fournier-Level Reference Cousens and Fournier-Level2018). As a useful tool, computer modeling can integrate available information and theories regarding the various factors and processes that affect evolution of resistance (Renton et al. Reference Renton, Busi, Neve, Thornby and Vila-Aiub2014). Modeling not only can allow scientists to understand and predict the evolutionary, dynamic, and rate spread of R populations under different environmental conditions and management systems, but may also allow farmers and agricultural advisors to design, execute, and test herbicide-resistance management scenarios intended to delay, prevent, and manage R plants (Chun et al. Reference Chun, Les and Paul2017; Renton et al. Reference Renton, Busi, Neve, Thornby and Vila-Aiub2014; Vila-Aiub et al. Reference Vila-Aiub, Neve and Powles2007).
The accuracy of a herbicide-resistance model’s outcome depends on management, ecobiological, and genetic features—the main components of herbicide-resistance modeling (Bagavathiannan and Norsworthy Reference Bagavathiannan and Norsworthy2016; Renton et al. Reference Renton, Busi, Neve, Thornby and Vila-Aiub2014). As one of the ecobiological subfactors in models, the fitness component is of high importance. Of course, it is almost impossible to have and include all fitness components (i.e., all aspects of plant life cycle) in a model; however, we strongly suggest keeping in at least the most important components of the plant life cycle, especially fecundity and seed germination fitness. This means that experimental data are vital to develop models predicting frequency of R and S plants for different generations/years. Simulation modeling is a combination of the direct and the indirect methods assessing relative fitness. It should be noted that lack of data for model estimation is the main limitation and challenge in developing and applying simulation models (Bagavathiannan and Norsworthy Reference Bagavathiannan and Norsworthy2016). Due to limitations associated with modeling, predictions may not always be accurate, so whenever possible, models should be validated by direct methods. The value of simulation modeling is that it allows integration of hidden parameters (i.e., parameters that cannot be experimentally tested) with parameters obtained from several fitness studies conducted separately at different conditions, producing a “take-home message” that can be applied to herbicide-resistance management programs.
Many models have been developed for this purpose. For instance, Gressel and Segel (Reference Gressel and Segel1978, Reference Gressel and Segel1990a, Reference Gressel and Segel1990b), Maxwell et al. (Reference Maxwell, Roush and Radosevich1990), and Mortimer et al. (Reference Mortimer, Ulf-Hansen and Putwain1992), as pioneer scientists in herbicide-resistance modeling, used fitness as a key parameter in their models predicting the evolution of resistance in populations. Due to the increasing importance of NTSR species, it is expected that attention will be directed to NTSR weed species, as exemplified by Langemann et al. (Reference Langemann, Richter and Vollrath2013) and Chun et al. (Reference Chun, Les and Paul2017), who developed a polygenic fitness model simulating the emergence of metabolic resistance. More information on simulation modeling can be found elsewhere (Bagavathiannan and Norsworthy Reference Bagavathiannan and Norsworthy2016; Diggle and Neve Reference Diggle and Neve2001; Renton et al. Reference Renton, Busi, Neve, Thornby and Vila-Aiub2014).
Many of the simulation models for herbicide resistance aim at predicting the time it will take before facing a herbicide-resistance phenomenon (Figure 1A), that is, the “time to resistance selection” (Renton et al. Reference Renton, Busi, Neve, Thornby and Vila-Aiub2014). A herbicide-resistance model might also answer the question of how long it will take to get rid of R plants in the absence of herbicide selection pressure, that is, the “time to back selection of resistance” (Figure 1B). To our knowledge, the second question has not been answered by existing simulation models. It is expected that the outcomes of the models predicting time to back selection will be more accurate than those predicting time to selection, because in the first case, it is difficult to accurately measure and obtain the current initial frequency of R alleles in a certain population (Diggle and Neve Reference Diggle and Neve2001; Renton et al. Reference Renton, Busi, Neve, Thornby and Vila-Aiub2014), while it is possible to determine the current frequency and distribution of R plants within a field (Burgos et al. Reference Burgos, Tranel, Streibig, Davis, Shaner, Norsworthy and Ritz2013). In addition, the real frequency of R plants in infested fields (i.e., when farmers complain about failure of herbicides) is around 35×10−2 (Burgos et al. Reference Burgos, Tranel, Streibig, Davis, Shaner, Norsworthy and Ritz2013; Gressel and Segel Reference Gressel and Segel1978, Reference Gressel and Segel1990a), while in a natural population it is theoretically expected to be 1×10−5 to 1×10−6 (Diggle and Neve Reference Diggle and Neve2001). Thus, we encourage the computer simulation modelers to also deal with the latter question.
Conclusions
The inherent challenges associated with fitness cost studies that could result in misleading results have been highlighted. The challenges were considered from a herbicide-resistance management point of view. Whenever the aim of fitness studies is the management of resistant species, plant materials (R and S individuals) should be selected within the same field or a field nearby, as a specific resistance gene may show variable fitness costs in specimens with different genetic backgrounds from distant geographical locations. The single population protocol and pedigreed lines approaches seem to be the most appropriate methods to increase genetic background homogeneity in a study, if the overall objective is information of relevance to the management of herbicide-resistant species.
Although measuring changes in the frequency of R and S alleles through indirect multigenerational fitness studies can assess fitness costs, it is a time-consuming approach that may not reveal differences in plant traits between R and S individuals that could be exploited in a management program. Furthermore, it is a method prone to genetic contamination of the environment through herbicide-resistance gene flow. Therefore, if the aim of a fitness study is to detect differences between R and S populations and apply the results in a herbicide-resistance weed management program, it may be more appropriate to evaluate all fitness components from seed to seed. For instance, an ACCase-resistant A. myosuroides phenotype possessing a Gly-2078 ACCase allele showed vegetative and fecundity fitness cost in comparison with its corresponding S phenotype (Menchari et al. Reference Menchari, Chauvel, Darmency and Délye2008). Accordingly, using competitive crops such as rye (Secale cereale L.) and wheat (Triticum aestivum L.), sowing at high density, and planting competitive cultivars were suggested as management strategies to inhibit and suppress the development, pollen distribution, and seed production of this R A. myosuroides phenotype (Menchari et al. Reference Menchari, Chauvel, Darmency and Délye2008). Similar management practices can be suggested to control dicamba-fluroxypyr–resistant K. scoparia populations, due to vegetative and fecundity fitness penalties (Kumar and Jha Reference Kumar and Jha2016). Deep soil cultivation and delayed sowing of autumn-sown crops were suggested for managing an NTSR A. myosuroides population, as the NTSR subpopulation showed a germination fitness cost: lower and later germination than of the S subpopulation at low temperatures and deep burial (Keshtkar et al. Reference Keshtkar, Mathiassen, Beffa and Kudsk2017a). A higher level of resistance to diclofop-methyl, sethoxydim, clethodim, and sulfometuron-methyl correlated with greater seed dormancy (i.e., a coevolution of dormancy and herbicide resistance) in L. rigidum populations, so delayed crop sowing followed by herbicide treatment (e.g., glyphosate) would increase the control of the R populations (Owen et al. Reference Owen, Michael, Renton, Steadman and Powles2011, Reference Owen, Goggin and Powles2015). Seed burial through shallow cultivation was suggested as an agronomic practice to inhibit the seedling emergence of an ACCase TSR L. rigidum population, as the R population possessed higher dormancy than the S population in the absence of light (Vila-Aiub et al. Reference Vila-Aiub, Neve, Steadman and Powles2005). In a review paper, Darmency et al. (Reference Darmency, Colbach and Le Corre2017) showed that around 60% of ACCase-resistant weed species had higher dormancy and delayed germination in comparison to ACCase-susceptible weed species, so suitable management practices can be suggested based on crop type and sowing time. In summary, differences in plant traits between R and S plants can be used in management programs. As an alternative approach to indirect, multigenerational, time-consuming fitness studies, simulation modeling can integrate available and unavailable fitness component parameters (i.e., data for plant life cycle stages that cannot be determined or are very hard to determine experimentally, e.g., number of pollen grains) and provide valuable information for herbicide-resistance management programs.
Acknowledgments
The authors wish to thank Todd Gaines, Colorado State University, for his valuable comments on an earlier version of this paper. This research received no specific grant from any funding agency or the commercial or not-for-profit sectors. No conflicts of interest have been declared.