Introduction
Wildlife trafficking is one of the most widespread and lucrative illicit activities worldwide (Lawson & Vines Reference Lawson and Vines2014, UNODC 2016), comprising the capture, poaching and trade of living and dead wildlife for pets, sport, human consumption and ornamental, medicinal or religious purposes (Barber-Meyer Reference Barber-Meyer2010, Hansen et al. Reference Hansen, Li, Joly, Makaru and Brownstein2012). Aside from the legality issues (see Lawson & Vines Reference Lawson and Vines2014, Ratchford et al. Reference Ratchford, Allgood and Todd2013), unregulated wildlife trade generates serious environmental consequences, including the introduction of exotic species, spread of wildlife diseases, disruption of ecosystem processes and ecological services such as pollination, seed dispersal and population control of other animals and, in the medium and long term, extinction of exploited species (e.g., Dai & Zhang Reference Dai and Zhang2017, Fernandes-Ferreira et al. Reference Fernandes-Ferreira, Mendonça, Albano, Ferreira and Alves2012, Nascimento et al. Reference Nascimento, Czaban and Alves2015). Although it is difficult to measure the number of illegally traded animals (Barber-Meyer Reference Barber-Meyer2010, Duffy Reference Duffy, Elliott and Schaedla2016), characterizing the origin of the trafficking chain could aid in efforts to reduce or eliminate indiscriminate captures (Lawson & Vines Reference Lawson and Vines2014, Primmer et al. Reference Primmer, Koskinen and Piironen2000).
Brazil has one of the richest avifaunas in the world, with many species currently threatened by illegal trade (Freitas et al. Reference Freitas, Oviedo-Pastrana, Vilela, Pereira, Loureiro and Haddad2015, Marini & Garcia Reference Marini and Garcia2005) including, for example, the family Thraupidae (e.g., Destro et al. Reference Destro, Pimentel, Sabaini, Borges, Barreto and Lameed2012, Fernandes-Ferreira et al. Reference Fernandes-Ferreira, Mendonça, Albano, Ferreira and Alves2012, Licarião et al. Reference Licarião, Bezerra and Alves2013). The interaction of factors in the commercial chain of illegal trade begins in rural regions and farmland areas (Fig. 1), where collectors capture animals using various techniques (see Souto et al., Reference Souto, Torres, Sousa, Lima, Vieira and Pereira2017). Once illegally captured, most birds are delivered to traders who negotiate the sale of specimens in public and street markets (Licarião et al. Reference Licarião, Bezerra and Alves2013, Regueira & Bernard Reference Regueira and Bernard2012), or they are sent to both authorized and unauthorized breeders and enterprises by intermediary traffickers (Destro et al. Reference Destro, Pimentel, Sabaini, Borges, Barreto and Lameed2012, Kuhnen et al. Reference Kuhnen, Remor and Lima2012) who subsequently trade them mainly through the Internet (Ratchford et al. Reference Ratchford, Allgood and Todd2013, Souto et al., Reference Souto, Torres, Sousa, Lima, Vieira and Pereira2017). Although the stages involved in wild animal trafficking, from capture in the wild to final consumers, are relatively well understood in Brazil, there is still incomplete information available on some links in this chain and on the factors underpinning illegal trade, which makes it difficult for the government to control this activity.
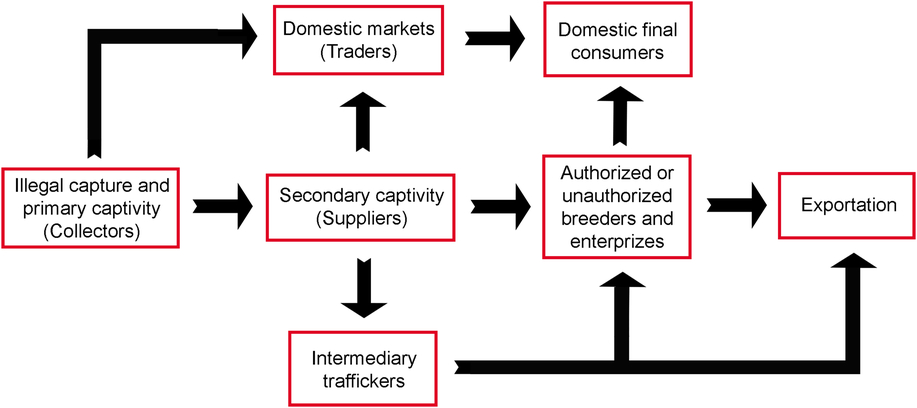
Fig. 1. Wildlife trafficking chain in Brazil from capture in the wild to final consumers.
For instance, illegal captures of wild birds and their trade are widespread throughout Brazil (Alves et al. Reference Alves, Lima and Araújo2013a), yet identifying source areas is not an easy task, as the site of apprehension by law enforcers generally differs from that of capture (Hernandez & Carvalho Reference Hernandez and Carvalho2006). However, it is possible to infer that most illegally traded animals come from the north, northeast and mid-west regions of Brazil (e.g., Destro et al. Reference Destro, Pimentel, Sabaini, Borges, Barreto and Lameed2012, Ferreira & Glock Reference Ferreira and Glock2004, RENCTAS 2001) and are then smuggled to both the south and southeast regions along federal highways (Supplementary Material S1, available online). Rivers are equally utilized routes for the sale of trafficked animals in the Amazon states (north region of Brazil); however, birds are less represented among the total number of wild vertebrates trafficked and seized through these routes (Nascimento et al. Reference Nascimento, Czaban and Alves2015). Much like other countries that report issues with illegal wildlife trade (e.g., Dai & Zhang Reference Dai and Zhang2017, Daut et al. Reference Daut, Brightsmith, Mendosa, Puhakka and Peterson2015, Gastañaga et al. Reference Gastañaga, Macleod, Hennessey, Nunez, Puse and Arrascue2011), the main purpose for illegally captured wild animals in Brazil is internal trade (Destro et al. Reference Destro, Pimentel, Sabaini, Borges, Barreto and Lameed2012).
Despite Brazil’s vast territory and extreme socio-environmental complexity, socioeconomic factors have been used to empirically explain and characterize the regional wildlife trade in the country (e.g., Alves & Rosa Reference Alves and Rosa2010, Regueira & Bernard Reference Regueira and Bernard2012). In order to better understand the nationwide dynamics of wildlife trafficking and to propose effective measures for the federal protection of exploited species, it is necessary to aggregate local socio-environmental peculiarities (e.g., Alves et al. Reference Alves, Lopes and Alves2016, Gama & Sassi Reference Gama and Sassi2008, Licarião et al. Reference Licarião, Bezerra and Alves2013). Thus, the present study aimed to analyse the drivers that are most influential in illegal animal captures in source countries worldwide and to evaluate, at a broad scale, which of these most often contribute to illegal bird capture in Brazil. The specific objectives were to map the main regions that supply trafficked wild animals in Brazil and to assess the factors that promote this illicit activity in each region of the country. This is the first broad-scale study aiming to identify the main drivers of illegal animal captures in source countries, which, if primarily considered in efforts for the regulation of animal trafficking, would render public policies towards overexploited species conservation more successful.
Methods
Selection of source municipalities and main drivers of illegal capture
An extensive literature search was carried out using the Portal de Periódicos (Brazilian National Electronic Library; CAPES 2016) to identify papers related to trafficked wild animals in Brazil. The terms ‘illegal’ AND ‘trade’ AND ‘Brazil’ were used, without restricting journal, language, year of publication or information platform. A total of 139 papers were identified. The Google Search Engine (www.google.com), in both Portuguese and English, was also used to search for grey literature, such as newsletter articles, magazines and newspapers, published abstracts, books, book chapters and technical reports, which added another 60 publications. Seven drivers cited by researchers that could explain the practice of illegal wild animal capture in source countries were identified (Table 1). The literature review was further used to list the source municipalities for animal trafficking in Brazil (i.e., the main municipalities described in the literature as wild animal suppliers, referred to herein as source areas; Supplementary Material S1 & S2).
Table 1. Drivers addressed by researchers that could explain the practice of illegal wildlife capture in source countries.

EPA = environmental protection area.
To obtain data for the analyses of these seven drivers, the most recent decennial census in Brazil (year 2010) was used as reference – except for native vegetation coverage (NVC) and road systems data, which were available for 2008. All geographic databases were obtained from Brazilian governmental websites, such as the Ministry of the Environment (MMA 2008, 2017), Ministry of Transport (MT 2008), the Instituto Nacional de Pesquisas Espaciais (INPE 2008) and the Instituto Brasileiro de Geografia e Estatística (IBGE 2010a, 2010b), or international organizations such as the United Nations Development Programme (UNDP 2010) (Table 1). Environmental protection areas were excluded from the analysis regarding quantitative protected areas (PAs), as this category displays a low degree of land-use restriction, which is closer to a mechanism for land-use management than an actual PA (Rylands & Brandon Reference Rylands and Brandon2005). A combination of distribution maps for the ten most seized native bird species in Brazil (Supplementary Material S3), according to a survey conducted by Destro et al. (Reference Destro, Pimentel, Sabaini, Borges, Barreto and Lameed2012), was used as a surrogate to obtain a richness map of the most trafficked species. Thus, exotic and domestic species listed by Destro et al. (Reference Destro, Pimentel, Sabaini, Borges, Barreto and Lameed2012) were excluded, as well as animals belonging to the other vertebrate classes. The ten bird species used here belong to the Thraupidae (7), Passerellidae (1), Icteridae (1) and Cardinalidae (1) families, which are highly coveted by traffickers due to their beautiful plumage, shape and singing ability (e.g., Fernandes-Ferreira et al. Reference Fernandes-Ferreira, Mendonça, Albano, Ferreira and Alves2012, Licarião et al. Reference Licarião, Bezerra and Alves2013, Teixeira et al. Reference Teixeira, Thel, Ferreira, de Azevedo, Telino and Lyra-Neves2014). Moreover, species like the saffron finch (Sicalis flaveola) and red-cowled cardinal (Paroaria dominicana) are also used in fights, as roosters are used in cock-fighting (Alves et al. Reference Alves, Nogueira, Araujo and Brooks2010, Gama & Sassi Reference Gama and Sassi2008, Souto et al., Reference Souto, Torres, Sousa, Lima, Vieira and Pereira2017). In fact, researchers have pointed to a need to understand the scale and breadth of the illegal trade of species that are not global conservation flagships, since these categories represent a significant proportion of live wildlife seizures in tropical countries and often have no guarantee of financial resources for protection (Gray et al. Reference Gray, Marx, Khem, Lague, Nijman and Gauntlett2017). The ten species accounted for over 60% of the wild birds seized in the country from 2005 to 2009 (Destro et al. unpublished data Reference Destro, Pimentel, Sabaini, Borges, Barreto and Lameed2012). In this sense, although all are categorized as being of Least Concern by the International Union for Conservation of Nature (IUCN 2016) and present a wide geographic distribution (Sick Reference Sick1997), many have suffered severe population reductions, and some have already become locally extinct (Fernandes-Ferreira et al. Reference Fernandes-Ferreira, Mendonça, Albano, Ferreira and Alves2012). We obtained the distribution polygons of the most seized species from the BirdLife website (BirdLife 2016) and all geoprocessing steps were performed using ArcGis 10.2.2 software (ESRI 2014).
Multivariate modelling
The values corresponding to the seven selected predictors were extracted for each of the 5563 Brazilian municipalities: (1) percentage of NVC; (2) Municipal Human Development Index (MHDI); (3) municipal gross domestic product (GDP); (4) most trafficked species richness (RIC); (5) percentage of PAs; (6) road density (m2/m2 × 1000) (ROA); and (7) municipal population density (person/km2) (MPD).
Multivariate modelling approaches were applied using a general discriminant analysis (GDA), a multivariate statistical technique that uses information available from a group of independent variables with normal distribution to predict the value of a categorical dependent variable (Ragsdale Reference Ragsdale2001). The GDA was performed using the Statistica 7.0 software first for Brazil nationwide and then by individual units of the north, northeast, mid-west and south/southeast regions. We grouped the south and southeast regions together due to their low incidence of municipalities with illegal capture and because they are considered to be the main consumers for Brazilian trafficked wildlife nationwide (e.g., Destro et al. Reference Destro, Pimentel, Sabaini, Borges, Barreto and Lameed2012, Ferreira & Glock Reference Ferreira and Glock2004, RENCTAS 2001). As the dependent variable, all Brazilian municipalities were categorized into two groups as binary factors: (1) source areas – main municipalities for illegal wildlife capture according to the literature; or (2) control group – the remaining municipalities. In this sense, the GDA was used to determine which independent variables (drivers) most contributed to the differences among groups (source areas and controls) (Malhotra Reference Malhotra2001). To obtain simple correlations between the variables and the discriminant function (i.e., to identify which independent variables cause the discrimination between the dependent variables), we used factor structure coefficients, also called structure correlations or discriminant loadings. Positive factor structure coefficients indicate a positive correlation among variables and negative values represent a negative correlation. The metric is useful to assign substantive, meaningful labels to the discriminant functions, akin to the interpretation of factors in a factor analysis (Hair et al. Reference Hair, Black, Babin, Anderson and Tatham2009). Variables that reached factor structure coefficients >0.4 were considered relevant (Hair et al. Reference Hair, Black, Babin, Anderson and Tatham2009). We standardized all data for the statistical analyses and used a stepwise method, which considers the step-by-step inclusion of significant variables only (Hair et al. Reference Hair, Anderson, Tatham and Black2005). In addition, we used Wilks’ λ statistic test in order to evaluate differences between means for each variable among groups, where λ = 1 indicates no significant difference (Subramanian et al. Reference Subramanian, Coutinho and Silva2007).
Results
In total, 195 municipalities displaying incidences of illegal capture of wild animals were identified: 40 in the north, 71 in the northeast, 45 in the mid-west and 39 in the south/southeast regions (Supplementary Material S1 & S2). The R2 obtained from χ2 tests with successive roots removed (Table 2) explained between 3.48% (northeast) and 10.85% (mid-west) of the variation observed among dependent variables. The Wilks’ λ statistic test indicated that, although significant, there is little difference between the source areas and control group.
Table 2. χ2 tests with successive roots removed.

Environmental factors, such as NVC and PAs, were the most important drivers of illegal wild bird captures in Brazil, followed by ROA and RIC (Table 3). Socioeconomic factors, such as MPD, GDP and MHDI, had secondary or null roles. An exception was observed for the northeast, where the high MHDI was noteworthy as an important discriminant driver of illegal wild bird captures (see also Supplementary Material S4).
Table 3. Factor structure coefficients of the variables nationally and in the four regions.

The most important variables are presented in bold (coefficients greater than ±0.4).
MPD = municipal population density; GDP = municipal gross domestic product; MHDI = Municipal Human Development Index; NVC = native vegetation coverage; ROA = road density; PA = protected areas; RIC = most trafficked species richness.
Discussion
Our study demonstrated on a broad scale that environmental factors were the most important drivers of illegal avifauna captures and not socioeconomic factors, reinforcing the need for multi-scale approaches in similar such studies (Cumming et al. Reference Cumming, Allen, Ban, Biggs, Biggs and Cumming2015, McGarigal et al. Reference McGarigal, Wan, Zeller, Timm and Cushman2016). Greater NVC was the most important driver of illegal wild bird captures in Brazil nationwide, as well as in the mid-west and south/southeast regions. This had been previously described in the Brazilian Cerrado, where the highest vegetation coverage was associated with greater biodiversity availability for harvesting by the resident population (Santos & Araujo Reference Santos and Araujo2015). In Iowa (USA), Haines et al. (Reference Haines, Elledge, Wilsing, Grabe, Barske, Burke and Webb2012) observed that poachers preferred to be active in areas next to forests and in riparian cover types containing variable topography. In addition, the higher concentration of PAs also boosts illegal capture in the country in its entirety, as well as in the northeast and south/southeast regions, probably due to a greater supply of specimens compared to unprotected sites, revealing a possible fragility of federal PAs regarding poachers or trappers (Carvalho Jr & Morato Reference Carvalho and Morato2013, Tebaldi et al. Reference Tebaldi, Fiedler and Dias2012). In contrast, Wright et al. (Reference Wright, Toft, Enkerlin-Hoeflich, Gonzalez-Elizondo, Albornoz and Rodriguez-Ferraro2001) revealed that Neotropical parrot poaching was higher in unprotected sites when compared to protected sites. New studies are needed to elucidate the true role of PAs in the conservation of species exploited by trafficking.
ROA also influences illegal bird capture in Brazil, especially in the north (Brazilian Amazon), which has the lowest ROA in the country, although rivers are also important means of transport for trafficked animals (Nascimento et al. Reference Nascimento, Czaban and Alves2015). Large numbers of roadways and pathways favour animal capture and poaching (Haines et al. Reference Haines, Elledge, Wilsing, Grabe, Barske, Burke and Webb2012, Maingi et al. Reference Maingi, Mukeka, Kyale and Muasya2012), since it is more convenient to transfer the animals to vehicles and trade them in public shops and street markets (Alves et al. Reference Alves, Lima and Araújo2013a, Shepherd et al. Reference Shepherd, Compton and Warne2007), besides facilitating the access and escape of poachers (Webb et al. Reference Webb, Dzialak, Wondzell, Harju, Hayden-Wing and Winstead2011). However, in the long term, regions that display lower highway concentrations tend to sustain larger stocks of targeted fauna, because roads make it easier to open forests for hunting and may also cause negative environmental effects, such as chemical and nutrient pollution, edge and barrier effects, animal car accidents, invasion of exotic species and other impacts on local soil, hydrology and aquatic ecosystems, especially if not previously planned for (Clements et al. Reference Clements, Lynam, Gaveau, Yap, Lhota and Goosem2014, Laurance et al. Reference Laurance, Goosem and Laurance2009). River routes were not included in our analysis, even though they are an important means of transport in regions such as the Amazon biome.
Species richness was also noteworthy as an important driver of fauna capture in the north and mid-west, as in African countries where poachers have focused efforts on areas that contain higher numbers of species and individuals (Atuo et al. Reference Atuo, O’Connell and Abanyam2015, Maingi et al. Reference Maingi, Mukeka, Kyale and Muasya2012). In fact, in the northern region of Brazil, most of the ten species display a marginal distribution to the eastern Amazon, coinciding with the location of source municipalities, while in the mid-west region they present a great overlap with the Pantanal biome, where most of the selected municipalities are located. Herein, we emphasize the limitations of our results, since the source areas used here came from bibliographical research, so that other relevant municipalities may not yet have been detected by enforcement efforts or research.
Contrary to our expectations, socioeconomic drivers were relevant only in north-eastern Brazil, one of the main supply regions of animals for the illegal wildlife trade (e.g., Destro et al. Reference Destro, Pimentel, Sabaini, Borges, Barreto and Lameed2012, Godoy & Matushima Reference Godoy and Matushima2010, RENCTAS 2001), comprising 36.41% of all summarized source municipalities. A positive relationship between source municipalities and MHDI was noted; illegal fauna capture occurred mainly in the municipalities presenting higher MHDI. This is contrasted with a common association between the high incidence of captive birds in areas with low socioeconomic index scores (Alves et al. Reference Alves, Leite, Souto, Bezerra and Loures-Ribeiro2013b, Regueira & Bernard Reference Regueira and Bernard2012). In fact, previous studies have pointed out that socioeconomic factors are the best predictors of poacher participation in the avian trade in Brazil (e.g., Gama & Sassi Reference Gama and Sassi2008, Santos & Araujo Reference Santos and Araujo2015, Souto et al., Reference Souto, Torres, Sousa, Lima, Vieira and Pereira2017) and other developing countries, such as Mexico (González-Marín et al., Reference González-Marín, Moreno-Casasola, Castro-Luna and Castillo2016), Zimbabwe (Lindsey et al. Reference Lindsey, Romañach, Matema, Matema, Mupamhadzi and Muvengwi2011), the Democratic Republic of the Congo (Nasi et al. Reference Nasi, Taber and Vliet2011) and Nigeria (Atuo et al. Reference Atuo, O’Connell and Abanyam2015). In this context, where high unemployment rates and low levels of formal education conditions prevail, activities related to the illegal bird trade would be very lucrative (Alves et al. Reference Alves, Lima and Araújo2013a) and would provide additional income sources to families (Souto et al., Reference Souto, Torres, Sousa, Lima, Vieira and Pereira2017). Brazil fits this description, presenting high social inequality, including in its main large cities (Regueira & Bernard Reference Regueira and Bernard2012), and high biodiversity, with 1919 bird species catalogued so far (Piacentini et al. Reference Piacentini, Aleixo, Agne, Maurício, Pacheco and Bravo2015) and many of them threatened (IUCN 2016).
A thorough look at the relationship between poverty and illegal wildlife consumption reveals that knowledge on this subject is limited and that conservationists should broaden their views of what constitutes illegal wildlife trade, what motivates people to hunt illegally and how to tackle the problem (Duffy et al. Reference Duffy, St John, Buscher and Brockington2016). Thus, although the illegal wildlife trade is frequently characterized as the result of economic poverty or greed, links among wealth, poverty and engagement in the wildlife trade are usually far more complex (Duffy et al. Reference Duffy, St John, Buscher and Brockington2016, TRAFFIC 2008). In fact, besides birds’ colour, singing ability and behaviour, access to birds and cultural habits seem to have a direct influence on common bird captures in Brazil (Alves et al. Reference Alves, Nogueira, Araujo and Brooks2010, Souto et al., Reference Souto, Torres, Sousa, Lima, Vieira and Pereira2017), making the internal trade in Brazil the main target for animals illegally collected from the wild (Destro et al. Reference Destro, Pimentel, Sabaini, Borges, Barreto and Lameed2012). This interpretation was evident in our results, since the seven drivers explained between 3.48% (northeast) and 10.85% (mid-west) of the variation observed among the source areas and the control group, revealing the participation of other variables that are difficult to measure or describe in the literature. In summary, the demand for songbirds in Brazil presents a high cultural bias (Licarião et al. Reference Licarião, Bezerra and Alves2013, Souto et al., Reference Souto, Torres, Sousa, Lima, Vieira and Pereira2017) and is widespread among the local population independent of socioeconomic factors (Alves et al. Reference Alves, Lopes and Alves2016), making surveillance and enforcement difficult and time consuming (Silva & Bernard Reference Silva and Bernard2015). In fact, the low risk of detection, relatively small penalties and minimal consequences for perpetrating wildlife crime, allied with the lack of enforcement and basic governance structures of local authorities, constitute attractive incentives to participate in this illegal activity (Ratchford et al. Reference Ratchford, Allgood and Todd2013), although this is difficult to measure and evaluate.
In sum, our results highlight that conservation measures for trafficked bird species should incorporate interactions between environmental, cultural and socioeconomic drivers, promoting actions capable of reducing the current level of exploitation (e.g., Alves et al. Reference Alves, Leite, Souto, Bezerra and Loures-Ribeiro2013b, Atuo et al. Reference Atuo, O’Connell and Abanyam2015, Tella & Hiraldo Reference Tella and Hiraldo2014). Thus, in order to reduce illegal captures, it is essential to implement effective public policies that involve education, policing/enforcement, the creation of alternative sources of income and proactive ecosystem management and conservation (e.g., Fernandes-Ferreira et al. Reference Fernandes-Ferreira, Mendonça, Albano, Ferreira and Alves2012, Santos & Araujo Reference Santos and Araujo2015, Souza & Alves Reference Souza and Alves2014), while always considering a multiple spatial-scale approach (Cumming et al. Reference Cumming, Allen, Ban, Biggs, Biggs and Cumming2015, McGarigal et al. Reference McGarigal, Wan, Zeller, Timm and Cushman2016). Expert opinions suggest that only improving the incomes or livelihood status of harvester communities often does not reduce their participation in the wildlife trade (TRAFFIC 2008), and well-intentioned policies may collapse at a local scale and ultimately fail to reduce the risks associated with environmental insecurity and biodiversity exploitation (Gore et al. Reference Gore, Lute, Ratsimbazafy and Rajaonson2016).
In many countries, especially those located in tropical regions with great fauna diversity, the illegal commerce of wild animals removes many species from their natural environments and is certainly among the gravest threats to native populations (Alves & Souto Reference Alves and Souto2015). We present a diagnostic method capable of discriminating drivers related to illegal bird capture in supply countries. However, other factors should be diagnosed and evaluated in future studies, especially those related to local and national governance power. In fact, the illegal wildlife trade requires broader debates on associated science and policy, since these debates have historically been focused on only a few high-profile species (i.e., rhinoceroses, tigers, elephants) and often overlook or combine complex actors, networks and contexts (Phelps et al. Reference Phelps, Biggs and Webb2016). Understanding the dynamics related to the drivers of wildlife trafficking is part of this transnational effort aimed at better geographical allocation of conservation actions and resources worldwide, leading to more precise and effective public policies for wildlife crime prevention and control (Haines et al. Reference Haines, Elledge, Wilsing, Grabe, Barske, Burke and Webb2012).
Supplementary material
To view supplementary material for this article, please visit https://doi.org/10.1017/S0376892919000316
Financial support
The authors thank the Instituto Brasileiro do Meio Ambiente e dos Recursos Naturais Renováveis (IBAMA) for supporting our research. LCT and PDM also thank the support provided by CNPq productivity grants.
Conflict of interest
The authors have no conflicts of interest to declare.
Ethical standards
None.