Introduction
Age at menarche (AAM) is a key indicator of the timing of the later phases of pubertal maturation in girls. In part because of its ease of measurement and predictive value, it is also the most common measure in research evaluating the correlates and causes of pubertal timing and has been linked to several reproductive, health, and psychosocial outcomes. One key predictor of earlier AAM is father absence. Whether the association between father absence and earlier AAM is causal remains unclear, as well as the potential mechanisms that can explain the association. To advance our understanding of these mechanisms and potential confounds linking father absence and earlier AAM, genetically informed research is needed. The goal of the present study is to investigate two forms of gene–environment interplay that may be important for understanding how father absence and AAM are linked: gene–environment correlation (rGE) and Gene × Environment interaction (G×E). In doing so, this study attempts to replicate and extend previous research that has used a molecular genetic approach to investigate the father absence/AAM association.
Father absence and age at menarche
Because AAM is related to a host of physical health (Gong, Wu, Vogtmann, Lin, & Wang, Reference Gong, Wu, Vogtmann, Lin and Wang2013; Prentice & Viner, Reference Prentice and Viner2013) and psychosocial factors (Mrug et al., Reference Mrug, Elliott, Davies, Tortolero, Cuccaro and Schuster2014; Skoog, Bayram Ozdemir, & Stattin, Reference Skoog, Bayram Ozdemir and Stattin2016), etiological research on AAM variability has been the subject of research over a number of years (Ellis, Reference Ellis2004). Much of this research has focused on putative environmental causes of individual differences in AAM including stress exposures during development, socioeconomic status (SES), and family structure (Richardson, La Guardia, & Klay, Reference Richardson, La Guardia and Klay2018). Emergent from this research is a corpus of studies that link biological father absence to earlier AAM, largely based on reasoning from life history theory (Ellis, Figueredo, Brumbach, & Schlomer, Reference Ellis, Figueredo, Brumbach and Schlomer2009). Derived from work by Draper and Harpending (Reference Draper and Harpending1982), Belsky, Steinberg, and Draper (Reference Belsky, Steinberg and Draper1991) hypothesized that psychosocial stressors in and around the family, such as father absence and other stressors, experienced early in life serve as cues that regulate life history strategy development, including AAM. According to this perspective, father absence that occurs early in development (the first 5–7 years) signals that paternal investment is unnecessary for successful child-rearing in the likely future reproductive environment. A faster life history strategy, characterized by less stable pair bonds and less discriminate sexual behaviors, would be more evolutionarily favorable in this environment. Unique to Belsky et al. (Reference Belsky, Steinberg and Draper1991) relative to Draper and Harpending was the proposition that father absence would also influence physical maturation as part of an organized life history strategy (see Belsky, Reference Belsky2012). Parallel developments by Ellis and colleagues (Ellis, Reference Ellis2004; Ellis, Schlomer, Tilley, & Butler, Reference Ellis, Schlomer, Tilley and Butler2012; James, Ellis, Schlomer, & Garber, Reference James, Ellis, Schlomer and Garber2012) further posited a special role of father absence in regulating sexual development via neurobiological mechanisms separate from other family-level and ecological stressors. Although some studies using these perspectives have failed to detect the association between father absence and AAM (e.g., in countries where malnutrition—potentially a stronger predictor of AAM—is relatively high; Kyweluk, Georgiev, Borja, Gettler, & Kuzawa, Reference Kyweluk, Georgiev, Borja, Gettler and Kuzawa2018; Sohn, Reference Sohn2017), meta-analytic findings of more than 30 studies show a reliable, though small, association between father absence and earlier AAM (Webster, Graber, Gesselman, Crosier, & Orozco Schember, Reference Webster, Graber, Gesselman, Crosier and Orozco Schember2014).
Genetic confouding in the father absence/AAM association
Extant research utilizing life history theory as the predictive framework for the association between father absence and AAM has generally presumed that father absence exerts its effect on AAM via environmental means. This assumption was challenged based on AAM heritability (Rowe, Reference Rowe2002) and suggests the father absence/AAM association could arise from a genetic confound reflecting passive rGE. Subsequently, several attempts have been made to disambiguate genetic versus environmental explanations, with contrasting results. In one of the earliest studies, Mendle et al. (Reference Mendle, Turkheimer, D'Onofrio, Lynch, Emery, Slutske and Martin2006) used a children-of-twins design to test the father absence genetic confounding hypothesis, and found that for youth of identical twin mothers, having a step-uncle was just as predictive of AAM as having a step-father (for which father absence is a requirement). These findings suggest that genetic transmission or a shared environmental confound could explain the association between father absence and AAM. Tither and Ellis (Reference Tither and Ellis2008) used a differential sibling exposure design, which controls for genetic and shared environment confounds by examining within-family associations, and found that the sister with greater exposure to father absence showed earlier AAM compared to her sister with less exposure, especially among fathers with high psychopathology. This finding suggests that father absence (and/or associated stressors) exerts a nonshared environmental effect on daughter's AAM. Findings across studies that use family-based research designs such as these have been critical for moving research on father absence and AAM further and suggests that there is likely some familial confounding that could be genetic in origin, but that familial or genetic confounding is unlikely to fully explain the association (Barbaro, Boutwell, Barnes, & Shackelford, Reference Barbaro, Boutwell, Barnes and Shackelford2017; D'Onofrio & Lahey, Reference D'Onofrio and Lahey2010). However, thus far, family-based designs have only been used to explore genetic and environmental mechanisms as alternative explanations for the father absence/AAM association, thus overlooking aspects of gene–environment interplay important for development (Rutter et al., Reference Rutter, Dunn, Plomin, Simonoff, Pickles, Maughan and Eaves1997). In addition, family-based designs operationalize genetic and familial confounds latently and are not directly measured. That is, because these designs infer genetic contributions in-lieu of examining DNA, these designs cannot inform the biological mechanisms through which father absence may exert an effect on AAM. Examining the genomic influences—through specific biological pathways and/or genomic aggregates—may help to explain associations between father absence and AAM (measured rGE; Jaffee & Price, Reference Jaffee and Price2007) and clarify if and how they are modified by structural environmental cues (G×E) critical for life history hypotheses.
As costs of genotyping and whole-genome assays have decreased, a growing body of literature has begun to incorporate measured genotypes into research on parenting and developmental outcomes. An initial study on father absence and AAM found support for the androgen receptor (AR) genetic confounding hypothesis when examining the GGC variant (Comings, Muhleman, Johnson, & MacMurray, Reference Comings, Muhleman, Johnson and MacMurray2002). This finding was not replicated, however, in a pair of independent follow-up studies (Jorm, Christensen, Rodgers, Jacomb, & Easteal, Reference Jorm, Christensen, Rodgers, Jacomb and Easteal2004; Schlomer, Murray, Yates, Hair, & Vandenbergh, Reference Schlomer, Murray, Yates, Hair and Vandenbergh2019). Notably, a better-characterized CAG variant within AR was related to earlier AAM independent of father absence and moderated the association between father absence and women's sexual behaviors (Schlomer et al., Reference Schlomer, Murray, Yates, Hair and Vandenbergh2019). Although not specific to father absence, two additional primary and replication studies found that variation in the estrogen receptor-α gene (ESR1) moderated the effect of family relationships on AAM (Hartman, Widaman, & Belsky, Reference Hartman, Widaman and Belsky2015; Manuck, Craig, Flory, Halder, & Ferrell, Reference Manuck, Craig, Flory, Halder and Ferrell2011). Following this line of research, Schlomer and Cho (Reference Schlomer and Cho2017) tested the hypothesis that father absence would moderate the association between lin-28 homolog B (LIN28B) variants (rs364663 (A/T) and rs314273 (G/T)) and AAM based on finidngs from genome-wide association studies (GWAS) that reliably linked LIN28B variation with later AAM and functional studies that showed overexpression of lin28 genes was related to an overall phenotype that resembled a slow life history strategy. Results of G×E models showed that for both single nucleotide polymorphisms (SNPs), daughters with the T/T genotype showed later AAM, but only if they were from father-present households. A genetic index analysis that combined the two SNPs into a composite showed that having at least one T/T genotype was related to later AAM among father-present women but not father-absent women.
These initial studies that integrate genomic information into the father absence/AAM literature reflect an important next step in the field by directly testing the interplay between genes and environments. The research to date, however, also has limitations. Perhaps most salient is that this work has primarily relied on candidate gene methods. Although often informed by biological and/or empirical evidence, candidate G×E (cG×E) studies, which focus on only one or a few variants, have proven difficult to replicate (Dick et al., Reference Dick, Agrawal, Keller, Adkins, Aliev, Monroe and Sher2015) and, at the extreme, some have argued that all cG×E research should be considered suspect given concerns about multiple testing (Duncan, Pollastri, & Smoller, Reference Duncan, Pollastri and Smoller2014). Stemming from this issue, replication attempts of cG×E research is of critical importance (Culverhouse et al., Reference Culverhouse, Saccone, Horton, Ma, Anstey, Banaschewski and Bierut2018).
An additional critique of the cG×E approach is that single-gene analyses do not reflect the biological reality that complex phenotypes, such as AAM, are underlain by many genes of small effect (Fisher, Reference Fisher1918; Lohmueller, Pearce, Pike, Lander, & Hirschhorn, Reference Lohmueller, Pearce, Pike, Lander and Hirschhorn2003). As a result, genomic research in psychological science has moved toward utilizing PGSs that aggregate hundreds to thousands of SNPs into an overall composite (Belsky & Israel, Reference Belsky and Israel2014). PGSs demonstrate large phenotypic main effect associations relative to candidate polymorphisms and are a promising avenue for G×E research (Conley, Laidley, Boardman, & Domingue, Reference Conley, Laidley, Boardman and Domingue2016). Indeed, recent research on the genetics of pubertal development showed that a PGS consisting of variants linked to AAM (Day et al., Reference Day, Thompson, Helgason, Chasman, Finucane, Sulem and Perry2017) was related to indicators of pubertal timing and tempo in both boys and girls (Horvath, Knopik, & Marceau, Reference Horvath, Knopik and Marceau2019). Further, the father absence/AAM genetic confounding hypothesis was recently tested by Gaydosh, Belsky, Domingue, Boardman and Harris (Reference Gaydosh, Belsky, Domingue, Boardman and Harris2018) who found that a similar AAM PGS was not related to father absence and that both father absence and the PGS uniquely predicted AAM. These results suggest that the association between father absence and AAM was not confounded by the PGS. These findings have not been replicated, however, and research examining possible G×E between father absence and PGSs on AAM has not been conducted.
Present study
The present study is designed to replicate and extend recent advances in the literature on father absence and AAM using genomic approaches by utilizing data from a large prospective longitudinal cohort study. First, we sought to replicate Gaydosh et al. (Reference Gaydosh, Belsky, Domingue, Boardman and Harris2018) by using an AAM PGS to test a possible measured rGE confound between father absence and AAM. Following prior findings, we hypothesized that SNPs related to pubertal timing would correlate with AAM, be uncorrelated with exposure to father absence, and that father absence and the PGS would predict AAM while controlling for the other. If supported, the results would show no evidence of measured rGE—in other words, any genetic confounding of the association would not be driven by puberty-related genetic variation captured by the PGS. Next, we sought to replicate Schlomer and Cho (Reference Schlomer and Cho2017) by testing G×E using a targeted, candidate gene approach. Following Schlomer and Cho, we hypothesized first that T alleles in LIN28B rs364663 and rs314273 SNPs would be associated with later AAM and second that this association would be found for father-present girls and not for father-absent girls. Finally, we extended work in this area by testing G×E using father absence and the PGS. Consistent with the LIN28B hypotheses, we expected that the later AAM associated with the PGS would be found for father-present girls only.
Methods
Participants
Data for this study were drawn from the Avon Longitudinal Study of Parents and Children (ALSPAC). ALSPAC is a population-based, longitudinal cohort study that initially enrolled 14,541 pregnant women in the United Kingdom whose expected date of delivery was between April 1st 1991 and December 31st 1992 (Boyd et al., Reference Boyd, Golding, Macleod, Lawlor, Fraser, Henderson and Davey Smith2013; Fraser et al., Reference Fraser, Macdonald-Wallis, Tilling, Boyd, Golding, Davey Smith and Lawlor2013). Of these initial pregnancies, there was a total of 14,676 fetuses, resulting in 14,062 live births and 13,988 children who were alive at 1 year of age. Data collection began when mothers were 8 weeks pregnant and includes 68 data collection time points between birth and child age 18 years, including mother and child reports. An additional 913 children were enrolled over the course of the study. Thus, the total sample available after age 7 years included 15,454 pregnancies, resulting in 15,589 fetuses of which 14,901 were alive at 1 year of age. Written informed consent was obtained from all study participants. Ethical approval for the study was obtained from the ALSPAC Ethics and Law Committee and the Local Research Ethics Committees. Consent for biological samples has been collected in accordance with the Human Tissue Act (2004). Analysis of the ALSPAC data for the current study was approved by the University at Albany, SUNY, Institutional Review Board.
Specific to the current study, the ALSPAC sample included N = 7,262 cases who self-identified as female. Of the female sample, genome-wide data were available on N = 4,355 participants. By design, the ALSPAC genomic sample is entirely European ancestry. Participants were excluded from the current study if they were missing data on AAM or father absence (N = 1,488, see Measures below). Additional cases were excluded from analysis due to missingness on other variables due to nonresponse or quality controls (N = 182). The final analytic sample for the current study was N = 2,685 female participants.Footnote 1 Comparisons between the analytic sample and the larger ALSPAC female sample revealed few differences. For example, average AAM was somewhat later among the included sample (M = 151.38 vs. 150.31 months; r = .035), and rates of father absence (14.6% vs. 22.2%, kappa = .078) and financial difficulties (M difference = 0.250 SDs, r = .12) were somewhat lower in the included sample than in the excluded sample, although effect sizes for these differences were small.
Measures
Please note that the ALSPAC study website contains details of all the data that are available through a fully searchable data dictionary and variable search tool: http://www.bristol.ac.uk/alspac/researchers/our-data/.
Age at menarche
Daughter's AAM was prospectively assessed up to 9 times during approximately annual assessments between the ages of 8 and 17 years of age. At each time point, surveys addressed if menstruation had begun and if so at what age. AAM responses from the first available wave were used in the current study to minimize recall bias (Culpin et al., Reference Culpin, Heron, Araya, Melotti, Lewis and Joinson2014; Gaydosh et al., Reference Gaydosh, Belsky, Domingue, Boardman and Harris2018). Cases that were ±3 SDs away from the mean were removed from the analysis (N = 13). Mean AAM in months was 151.382 (SD = 13.802) and ranged from 114 to 192.5.
Father absence
ALSPAC mothers completed household composition surveys when children were 1 year 7 months, 2 years 7 months, 3 years 9 months, and 7 years old (Culpin et al., Reference Culpin, Heron, Araya, Melotti, Lewis and Joinson2014). Mothers were asked if the current live-in father figure was the biological father of the target child. Response options were “Yes,” “No,” “No live-in father figure,” and “Don't know.” For each assessment, “Yes” responses were coded as biological father present. Cases where the live-in father figure was not the target child's biological father (Ns = 35 to 121 across waves) or there was no live-in father figure (Ns = 103 to 175) were coded as biological father absent. “Don't know” responses (< 0.5%) were dropped from the analysis. Children were coded as 1 = father absent prior to age 7 years if their mother reported father absence at any of the four assessments (N = 392, 14.6%). The remaining children were coded 0 = father present (N = 2,293, 85.4%).
Genotyping
DNA were collected on approximately 11,000 children at age 7 years using blood, cell line, and mouthwash samples and genotyped using the Illumina HumanHap550 platform (Boyd et al., Reference Boyd, Golding, Macleod, Lawlor, Fraser, Henderson and Davey Smith2013; Pembrey, Reference Pembrey2004). Raw genotype data were subjected to quality control measures and participants were excluded based on gender mismatch, minimal or excessive heterozygosity, >3% missingness, cryptic relatedness (IBD > 0.10), and non-European ancestry. Genomic data were imputed using 1000 Genomes phase 1, version 3. Following quality controls, a total of N = 4,355 female participants with genome-wide data were available.
Polygenic score. The PGS used in the current study was derived from findings by Day et al. (Reference Day, Thompson, Helgason, Chasman, Finucane, Sulem and Perry2017), who conducted the largest GWAS to date on AAM, comprising a sample of over N = 300,000 women from the ReproGen Consortium, 23andME, and the UK Biobank. GWAS results revealed 389 nonredundant genome-wide significant (p < .05 × 10−8) signals that collectively explained approximately 7.0% of the variance in AAM (see Day et al., Reference Day, Thompson, Helgason, Chasman, Finucane, Sulem and Perry2017).
Supplemental information provided by Day et al. (Reference Day, Thompson, Helgason, Chasman, Finucane, Sulem and Perry2017) were used to create the PGS in the ALPAC data. Of the 389 independent SNPs, N = 372 were available in ALSPAC. Following quality control procedures that account for excess missingness (> 1%), low minor allele frequency (<1%), and Hardy–Weinberg violations (p < 5 × 10−7), 339 variants remained. The 339 variants were then weighted by their respective effect sizes (Dudbridge, Reference Dudbridge2013) provided by Day et al. and averaged, resulting in a normally distributed PGS, which was then mean centered via standardization within the analytic sample (M = 0.00, SD = 1.0).
LIN28B. Following Schlomer and Cho (Reference Schlomer and Cho2017), rs364663 and rs314273 were analyzed as tag-SNPs that reflect variation in 26 total SNPs within a LIN28B LD block. Genotype frequencies for rs364663 were A/A = 846 (31.5%), A/T = 1,350 (50.3%), T/T = 489 (18.2%); and for rs314273 G/G = 1,185 (44.1%), G/T = 1,213 (45.2%), T/T = 287 (10.7%). These frequencies were similar to those reported in Schlomer and Cho (Reference Schlomer and Cho2017). Both SNPs were in Hardy–Weinberg equilibrium (χ 2s (1) < 1.52, ns). SNPs were dummy coded (Aliev, Latendresse, Bacanu, Neale, & Dick, Reference Aliev, Latendresse, Bacanu, Neale and Dick2014) such that the reference group for each SNP was for no copies of the T allele (A/A and G/G for rs364663 and rs314273, respectively) given prior research that suggests the T allele is associated with later AAM (see Schlomer & Cho, Reference Schlomer and Cho2017).
Covariates. Two covariates were used in all analyses. Prior work on the ALSPAC genetic sample showed little population structure (Jones et al., Reference Jones, Stergiakouli, Tansey, Hubbard, Heron, Cannon and Zammit2016; Okbay et al., Reference Okbay, Beauchamp, Fontana, Lee, Pers, Rietveld and Benjamin2016). However, to help adjust the results for potential additional undetected population structure, principal coordinates analysis (PCA) was conducted on the genome-wide data using the --pca command in plink (Chang et al., Reference Chang, Chow, Tellier, Vattikuti, Purcell and Lee2015). Eigenvalues examined in a scree plot (Cattell, Reference Cattell1966) leveled off following the first PC (PC1), which was used as a covariate in all analysis (D'Agostino & Russell, Reference D'Agostino, Russell, Armitage and Colton2005). In addition, a financial difficulties measure was created to help control for possible socioeconomic confounds, which prior research suggests is related to both father absence and AAM (Boynton-Jarrett & Harville, Reference Boynton-Jarrett and Harville2012). When children were 8, 33, and 85 months of age mothers responded to a series of questions about difficulty affording food, clothing, rent, heat, and things for the child (1 = very difficult, 4 = not difficult). Scale reliabilities within each wave were good (α = 0.885–0.892) and the three assessments showed mean correlation of r = .524. The three assessments were reversed coded so that higher values equal more financial difficulties and then averaged. The resulting scale ranged from 0 to 3 (M = 0.484, SD = 0.522). The financial difficulties variable was mean centered via standardization for use in regression models.
Analysis plan
To formally test the genetic confounding hypothesis using the AAM PGS, a path model was conducted in Mplus (Muthen & Muthen, Reference Muthen and Muthen1998–2015) using father absence to predict AAM and the PGS predicting both (see Figure 1). PC1 and financial difficulties (not shown in Figure 1) were also included as exogenous predictors of father absence, AAM, and the PGS. Following this model, G×E analyses were conducted in a series of four hierarchical regression models conducted in R (lm function; R Core Team, 2018). All models included PC1 and financial difficulties as covariates in the first block, then the main effects were added in the second, and finally the interactions in the third block. The first three models were designed to test the hypothesis that the association between LIN28B SNPs and later AAM would be found for father-present girls and not for father-absent girls, consistent with models reported by Schlomer and Cho (Reference Schlomer and Cho2017). Model 1 included father absence, dummy coded rs364663, and the product terms between father absence and the dummy variables. Model 2 was an identical model including rs314273 instead of rs364663. Model 3 included a genetic index combining the two SNPs: following Schlomer and Cho (Reference Schlomer and Cho2017) the rs364663 and rs314273 SNPs were combined into a genetic index such that 0 = No T/T genotypes from either SNP (N = 2,196) and 1 = at least 1 T/T genotype (N = 489). The fourth model tested the hypothesis that the later AAM associated with the PGS would be found for father-present girls only and was identical to the prior G×E models except that the AAM PGS was included as the genetic indicator.
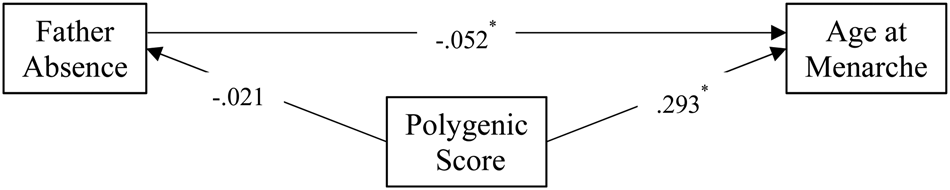
Figure 1. Testing the father absence genetic confounding hypothesis using an age at menarche polygenic score (AAM PGS).
Results
Preliminary analyses
Table 1 shows the correlations among variables used in the current study. AAM was correlated with father absence, rs314273, the PGS, and financial difficulties in the expected directions. rs364663 and rs314273 were strongly correlated with each other, reflecting their common LD block within LIN28B. Both were also modestly correlated with the PGS and a small correlation was detected between rs314273 and PC1. Father absence was related to more financial difficulties and not correlated with the LIN28B SNPs nor the PGS, which suggests any detected G×E is unlikely to be an artifact of rGE (Bakermans-Kranenburg & van IJzendoorn, Reference Bakermans-Kranenburg and van IJzendoorn2015).
Table 1. Correlation matrix of analysis variables

Note: rs364663 and rs314273 are linearly coded (0/1/2). PC1 refers to the first principal coordinate.
* p < .05; N = 2,685.
Father absence and PGS genetic confounding
Standardized results from the path model (Figure 1) showed that father absence (β = −0.052, p < .05) and the PGS (β = 0.293, p < .05) were related to AAM in predicted directions. There was no association between father absence and the PGS (β = −0.021, ns). These findings suggest that the PGS cannot account for the father absence/AAM association and that the PGS and father absence have unique associations with AAM. Constraining the father absence → AAM path equal to the PGS → AAM path resulted in significant model misfit (Δχ 2 (1) = 244.719, p < .05) indicating the association between the PGS and AAM was stronger than the father absence association.
Model 1: Father absence and rs364663
Regression results showed that father absence was related to approximately 2.3 months earlier AAM (b = −2.289, p < .05) while controlling for rs364663, financial difficulties, and PC1. No main effects were found for the difference between daughters with the rs364663 A/A genotype versus A/T (b = 0.191, ns) or between daughters with A/A versus T/T (b = 0.893, ns; model R 2 = .006). Adding the product terms to the model (ΔR 2 = .004) showed an interaction between father absence and A/A versus A/T genotypes (b = 4.205, p < .05) and no interaction between father absence and A/A versus T/T (b = −1.218, ns). An additional analysis using A/T as the reference group showed an interaction between father absence and A/T versus T/T genotype (ΔR 2 = .004; b = −5.423, p < .05; no main effect of A/T vs. T/T was detected, b = 0.703, ns).
Follow-up simple effects tests were conducted by re-centering model variables to obtain conditional effects (Frazier, Tix, & Barron, Reference Frazier, Tix and Barron2004; see Figure 2a). Qualifying the interaction between father absence and A/T versus T/T was a trend for later AAM between girls with A/T versus T/T genotypes who were father present (b = 1.507, p = .06). Specifically, AAM was approximately 1.5 months later among T/T father-present girls compared to A/T father-present girls. Among father-absent girls, the T/T genotype was related to nearly 4 months earlier AAM compared to A/T girls (b = −3.916, p < .05; see Figure 2a). Reflecting the interaction between father absence and A/A versus A/T, AAM was 3.8 months earlier among father-absent girls who had the A/A genotype compared to the A/T (b = 3.790, p < .05), whereas the A/A versus A/T slope for father-present girls was flat (b = −0.415, ns). This overall pattern of results suggests that having the rs364663 T/T genotype may be related to later AAM among father-present women, consistent with Schlomer and Cho (Reference Schlomer and Cho2017), and that having either the A/A or T/T genotype is related to earlier AAM among father-absent women.

Figure 2. Interactions between father absence and dummy coded rs364663 (a) and rs314273 (b).
Model 2: Father absence and rs314273
Like Model 1, father absence was related to approximately 2.3 months earlier AAM (b = −2.311, p < .05) when controlling for financial hardship, PC1, and rs314273 (model R 2 = 0.008). No AAM difference was found for women with G/G vs G/T genotypes (b = 0.640, ns). Girls with the T/T genotype showed 2 months later AAM compared to G/T girls, irrespective of father absence status (b = 2.011, p < .05). In addition, an interaction was detected between father absence and G/G versus T/T genotypes (b = −4.680, p < .05) as well as between father absence and G/T versus T/T (b = −6.345, p < .05); no interaction was found between father absence and G/G versus G/T (b = 1.667, ns; ΔR 2 = .003). Qualifying these interactions (see Figure 2b), AAM was nearly 2.5 months later among father-present girls with the T/T genotype compared to G/T (b = 2.467, p < .05) or G/G (b = 2.892, p < .05) genotypes. Among father-absent girls, there was a trend toward earlier AAM, by approximately 4 months, for T/T genotype girls compared to G/T (b = −3.878, p = .07) and no difference was found for G/G vs T/T (b = −1.786, ns). Similar to Model 1, these results indicate that the rs314273 T/T genotype is related to later AAM among father-present girls and may be related to earlier AAM among father-absent girls.
Model 3: LIN28B genetic index
Model 3 was identical to Models 1 and 2 except that the LIN28B index was used, which combines rs364663 and rs314273 into an overall score (0 = no T/T genotypes, 1 = at least 1 T/T genotype). Results are depicted in Figure 3a. After adjusting for the covariates, there was a main effect of father absence (b = −2.291, p < .05) and no main effect of the LIN28B index (b = 0.776, ns; R 2 = .006); the interaction was statistically significant (b = −3.701, p = .05; ΔR 2 = .001). AAM for girls with at least one rs364663 or rs314273 T/T genotype was approximately 5 months later if they were father present compared to father absent (b = 5.273, p < .05); this difference, approximately 1.5 months, was marginal among girls without a T/T genotype (b = −1.572, p = .07), consistent with findings reported in Schlomer and Cho (Reference Schlomer and Cho2017).

Figure 3. Father absence by lin-28 homolog B (LIN28B) genetic index interactions. (a) at least 1 T/T genotype between rs364663 and rs314273. (b) number of T/T genotypes.
Expanding on this analysis, the LIN28B genetic index was re-coded such that 0 = No T/T genotypes (N = 2,196), 1 = At least 1 T/T genotype (N = 202) and 2 = Two T/T genotypes (N = 287). Dummy coded analyses showed an interaction between father absence and 0 versus 2 T/T genotypes (b = −5.474, p < .05; ΔR 2 = .002). Results are depicted in Figure 3b. Among father-present girls, AAM was nearly 3 months later for girls with 2 T/T genotypes compared to having 1 (b = 2.980, p < .05) or 0 (b = 2.645, p < .05). Among father-absent girls, more copies of the T/T genotype was not related to AAM (2 vs. 1: b = −1.600, ns; 2 vs. 0: b = −2.830, ns).
Model 4. G×E analysis with the PGS
Model 4 was identical to prior models except the PGS was used as the genetic variable. Results showed father absence continued to predict earlier AAM when controlling for financial difficulties, PC1, and the PGS (b = −2.023, p < .05) and the AAM PGS predicted later AAM (b = 4.025, p < .05), as expected; for every one standard deviation increase in the PGS, AAM was 4 months later. The interaction between father absence and the AAM PGS was not detected (b = .058, ns; ΔR 2 < .001). To test for possible nonlinear associations, squared and cubed versions of the PGS were evaluated. No main effects were found for the PGS beyond the linear association (squared: b = −0.062, ns; cubed: b = 0.014, ns) nor did the squared or cubed PGSs interact with father absence (bs = 0.283 and 0.143, ns, respectively).
Sensitivity analyses
The dummy coded analyses reported above for rs364663 and rs314273 allow potential nonlinear relationships between SNPs and AAM to be evaluated (Aliev et al., Reference Aliev, Latendresse, Bacanu, Neale and Dick2014). In GWAS, however, the SNP-phenotype association is presumed to be linear. As a follow-up test, analyses were conducted to examine the linear association between the LIN28B SNPs and AAM as well as their interactions with father absence. While controlling for covariates, results showed that rs314273 was related to later AAM by nearly 1 month per T-allele, irrespective of father absence (b = 0.879, p < .05). No linear association was found for rs364663 (b = 0.411, ns). Interactions for both SNPs with father absence were nonsignificant (rs364663: b = 0.009, ns; rs314273: b = −1.186, ns).
An additional consideration is whether the father absence/AAM association is driven by the presence of a stepfather or other nonbiologically related adult male (e.g., the mother's live-in boyfriend), which can be a consequence of father absence (Ellis & Garber, Reference Ellis and Garber2000; Mendle et al., Reference Mendle, Turkheimer, D'Onofrio, Lynch, Emery, Slutske and Martin2006). To test this possibility, an analysis of covariance (ANCOVA) was conducted using a nominal family status variable with three levels: father-present (N = 2,293), biological father absent (N = 220), and nonbiological adult male present (N = 172). PC1 and financial difficulties were included as covariates. Results showed a null interaction between the LIN28B index and family status in the omnibus model (F (2,2677) = 2.279, ns). A Helmert contrast showed that AAM was later among father-present girls (M = 152.69) compared to biological father-absent/nonbiological adult male present girls (M = 149.32; t = 3.47, p < .05), reflecting prior results. No difference was found for the contrast between biological father-absent (M = 148.72) and nonbiological adult male present girls (M = 149.911; t = 0.67, ns). This result suggests that the reported father absence findings are not driven by stepfather/nonbiologically related adult male presence.
It is also possible that chronicity of father absence during early development may be driving the dichotomous father-present/-absent effect reported in this study. To evaluate this possibility, a father absence “dosage” variable was created that quantified the number of waves a participant reported being father absent (0 to 4) and was used in place of the dichotomous variable in the models described above. To ensure the accuracy of the dosage variable, analyses were limited to cases with complete father absence data at all four waves (N = 2,075). Results of main effects models showed that father absence dosage was not significantly related to AAM when covariates and the respective genetic variables were included. These nonsignificant findings are likely attributable to smaller standardized effect sizes observed for the dosage (βs ~ −0.033, ns) compared to the dichotomous (βs ~.060, ps < .01) father absence variable as well as the larger standard errors that resulted from the sample reduction. Given the lack of main effects of father absence dosage, moderation analyses were not further pursued.
In addition, because financial difficulties could be theoretically conceptualized as either a mechanism by which father absence could affect AAM (e.g., James et al., Reference James, Ellis, Schlomer and Garber2012) or a component of a larger environmental stress factor that also includes father absence (e.g., Belsky et al., Reference Belsky, Steinberg and Draper1991), using financial difficulties and a control variable could potentially remove important variance in AAM. To evaluate this possibility, models were conducted with financial difficulties uncontrolled. Results were highly similar; the largest change in the beta coefficients was 0.09 months and the pattern of significance was the same. We also examined the components of financial difficulties separately to provide further context for the role of financial difficulties. To do so, each item of the composite was separately averaged across the three assessment waves (child age 8 months, 32 months, and 85 months, see Methods section) resulting in five indicators of financial difficulties (i.e., difficulty affording: (a) food, (b) clothing, (c) heat, (d) rent, and (e) items for the child). These five indicators were included in analysis models described above in place of the overall financial difficulties composite. Across all models, none of the individual indictors were significantly related to AAM in the main effects analyses (bs < 0.80, ns), including the difficulty affording food indicator (bs < 0.40, ns). Using the individual items as covariates in place of the composite did not affect the pattern of results observed in the interaction models.
Last, the LIN28B findings reported above could be interpreted as evidence for differences in susceptibility to father absence. Differential susceptibility theory (Belsky & Pluess, Reference Belsky and Pluess2009; Ellis, Boyce, Belsky, Bakermans-Kranenburg, & van IJzendoorn, Reference Ellis, Boyce, Belsky, Bakermans-Kranenburg and van IJzendoorn2011) posits that intrapersonal characteristics that would otherwise be conceptualized as risk factors within adverse environments may be related to more positive outcomes in more supportive environments. To test the hypothesis that LIN28B conveys differential sensitivity to father absence, a reparametrized regression model (Widaman et al., Reference Widaman, Helm, Castro-Schilo, Pluess, Stallings and Belsky2012) was conducted to test the differential susceptibility hypothesis for the interaction between the LIN28B index (coded 0/1) and father absence. The reparametrized regression model includes the cross-over point of the conditional slopes as an estimated model parameter, which permits 95% confidence intervals to be calculated. The cross-over parameter and its confidence interval can be used to infer the likely form of the interaction in the population. Disordinal interactions are consistent with differential susceptibility theory whereas ordinal interactions indicate diathesis stress or, alternatively, vantage sensitivity, which posits sensitivity to ostensibly positive environments, such as father presence (see Pluess & Belsky, Reference Pluess and Belsky2013; Widaman et al., Reference Widaman, Helm, Castro-Schilo, Pluess, Stallings and Belsky2012). In the current analysis, the cross-over point reflects the estimated point where the conditional slopes for the association between the LIN28B index and AAM intersect (one slope for father present and one for father absent). Results of the reparametrized regression showed the cross-over point was estimated at −0.516 with a 95% confidence interval that ranged from −1.336 to 0.304 (Figure 4). Because the cross-over is estimated beyond the range of the predictor (LIN28B index, 0/1) the interaction form is ordinal, which we interpret as consistent with vantage sensitivity given the later AAM associated with LIN28B was present for father-present girls and not for father absence girls. It is important to note, however, that because the upper 95% CI falls within the range of the LIN28B index, a disordinal interaction in the population cannot be ruled out. Notably, the ordinal interaction is also consistent with findings from Schlomer and Cho (Reference Schlomer and Cho2017).

Figure 4. Test of the interaction form (ordinal vs. disordinal). Shaded area reflects the 95% confidence interval for the slope cross-over point (−1.336 to 0.304).
Discussion
The purpose of this study was to replicate and extend recent research on the father absence/AAM association that used genomic approaches. The genetic confounding hypothesis was tested first following prior research that suggested a similar AAM PGS did not confound the association (Gaydosh et al., Reference Gaydosh, Belsky, Domingue, Boardman and Harris2018). Contrary to the genetic confounding hypothesis, independent associations were found in the current study for both father absence and the PGS in a path model and the PGS was not correlated with father absence. In line with the prior study, these findings indicate that father absence is not confounded by the AAM PGS evaluated in this study. It is important to point out, however, that the PGS reflects a narrow genetic control given it explains approximately 7% of the additive genetic contribution to AAM (Day et al., Reference Day, Thompson, Helgason, Chasman, Finucane, Sulem and Perry2017). Any remaining association between father absence and AAM after controlling for the PGS could still be accounted for by additional unmeasured additive genetic variance, epistasis, epigenetics, or a number of other biological and/or nonbiological factors. Although the current and prior studies cannot resolve the ongoing debate about whether father absence constitutes an environmental pressure that regulates pubertal development posited by life history theory, these studies indicate that the genetic variance captured by the PGS cannot account for the father absence/AAM association. In addition, and perhaps more importantly, the current results are informative regarding the relative magnitude of the genetic and putative environmental effects captured by the PGS and father absence, respectively. As evidenced by the model misfit when the father absence → AAM and PGS → AAM paths were constrained equal, the PGS was much more strongly related to AAM relative to father absence. Although father absence may exert a small environmental effect on AAM, the additive genetic contribution captured by the PGS explained a much larger portion of the variance. An implication of this observation is that the small effect of father absence may be more likely to go undetected in genetically informed studies than the other way around, particularly given other factors known to influence the father absence/AAM association (e.g., SES, ethnicity). Thus, failure to detect an effect of father absence on AAM, particularly in genomic study, may not necessarily indicate a lack of association but may reflect the inability of a given sample to detect what amounts to a relatively small main effect of father absence, which will be more subject to study variances (e.g., sampling, measurement) compared to the relatively larger genomic associations. That is not to say that father absence or other family environmental effects on AAM should be dismissed as relatively unimportant. For example, half of menstrual cycles are anovulatory for approximately 1 year among girls whose menarche occurs prior to age 12 years old. Among girls whose menarche occurs at age 13 or older, the anovulatory period lasts for 4.5 years. Small effect sizes can translate into substantial implications for reproductive outcomes (Ellis, Reference Ellis2004).
Further, it may come as a surprise find such a relatively large genetic-phenotype association given the effect size discrepancies often found between molecular genetic studies and family-based genetic designs (e.g., twin studies; Manolio et al., Reference Manolio, Collins, Cox, Goldstein, Hindorff, Hunter and Visscher2009). Maximizing phenotypic variance explained is precisely the goal, however, of PGS methods (Wray et al., Reference Wray, Lee, Mehta, Vinkhuyzen, Dudbridge and Middeldorp2014). By scanning the entire genome via GWAS for statistically relevant signals and then aggregating them, PGSs are designed to explain substantially more variance in a phenotype than any one signal could on its own. This approach reflects an important and necessary advance in genomic research, particularly as it applies to behavioral and psychological science. Nonetheless, the systematic scan and aggregation of phenotypically relevant factors on only one side of the gene–environment equation could lead to underestimating the importance of the environment, broadly defined, when comparisons are made between PGSs and single environmental factors that on their own may explain only tiny amounts of variation. This imbalance—reflecting advances in genomic measurement unparalleled in environmental measurement—has not gone unnoticed in epidemiological research of disease etiology and calls have been made to measure the “exposome” in a manner inspired by genotyping arrays (Wild, Reference Wild2005).
Relevant to the current study, father absence associations are commonly detected and are reliably small (Webster et al., Reference Webster, Graber, Gesselman, Crosier and Orozco Schember2014) and, arguments about the environmentality of father absence and related factors aside, maximal environmental prediction of AAM could be achieved in a similar manner as PGSs via systematic scans of the likely many environments of small effect that influence AAM. In this framework, father absence is only one of potentially many environments that could be aggregated into a poly-environment score that could maximize phenotypic prediction in a similar manner as PGSs. Indeed, this approach of aggregating environmental effects is theoretically consistent with Belsky et al.'s (Reference Belsky, Steinberg and Draper1991) original proposition, which did not privilege father absence above other psychosocial stressors but posited that multiple stress-related factors would influence AAM. Environment-wide association studies that systematically evaluate the “exposome” in a manner conceptually similar to GWAS have been successfully applied in primary and replication research on Type 2 diabetes (Hall et al., Reference Hall, Dudek, Goodloe, Crawford, Pendergrass, Peissig and Ritchie2014; Patel, Bhattacharya, & Butte, Reference Patel, Bhattacharya and Butte2010) and offer a promising avenue for studying environmental influences on reproductive health (Buck Louis, Yeung, Sundaram, Laughon, & Zhang, Reference Buck Louis, Yeung, Sundaram, Laughon and Zhang2013) and early-life exposures on developmental outcomes (Vrijheid et al., Reference Vrijheid, Slama, Robinson, Chatzi, Coen, van den Hazel and Nieuwenhuijsen2014). Though beyond the scope of the current study, additional research should examine whether greater environmental prediction, and potential G×E, would be observed when aggregating sets of environmental factors hypothesized to influence AAM, such as father absence, financial difficulties, and others.
A second goal of this study was to replicate findings from Schlomer and Cho (Reference Schlomer and Cho2017). The initial evidence from Schlomer and Cho suggested that the later AAM linked to LIN28B SNPs rs364663 and rs314273 is specific to father-present girls. The potential reliability of this initial evidence was undermined by its limitations, which included a relatively small sample of college students (N = 300), retrospective self-reports of AAM, and father absence operationalized as parent's current marital status. In addition, because the study used a candidate gene approach to evaluate G×E, the prior probability that detected associations would be meaningful could be considered low (Dick et al., Reference Dick, Agrawal, Keller, Adkins, Aliev, Monroe and Sher2015; Ioannidis, Reference Ioannidis2005). The current study improves upon these limitations via a larger community-based sample (N = 2,685), contemporaneously measured AAM, and a father absence variable based on repeated measures of household composition drawn from mother reports. With these methodological improvements in mind, the results of the current replication attempt were largely consistent with the prior work: The association between LIN28B SNPs and later AAM typically found in GWAS was specific to father-present girls. Evidence for this pattern was found both in the individual SNP analyses and, most strongly, in the genetic index analyses, consistent with Schlomer and Cho. In addition, the ordinal interaction pattern found in this study, supporting a vantage sensitivity interpretation of the genetic index interaction, was also replicated. The larger sample size of the current study also afforded an additional sensitivity analysis that showed these findings were not driven by stepfather/nonbiologically related adult male presence. Taken together, primary and replication studies find evidence to support a LIN28B-by-father absence G×E on variation in AAM such that LIN28B is linked to later AAM among father-present girls and not among father-absent girls.
The hypothesis that father absence would moderate the association between LIN28B and AAM in Schlomer and Cho (Reference Schlomer and Cho2017) was based on prior father absence research using life history theory, which posits early developmental experiences in and around the family provide evolutionarily relevant cues that regulate the development of life history strategies. Interestingly, father absence—hypothesized as one of these cues—only modified the effect of LIN28B, and not the PGS, on AAM. This finding suggests that father absence has a discriminating effect on the pathway(s) that LIN28B operates. LIN28B was chosen for evaluation based on its reliable association with AAM across genome-wide studies (Day et al., Reference Day, Thompson, Helgason, Chasman, Finucane, Sulem and Perry2017), studies that showed LIN28B is highly evolutionarily conserved (Thornton & Gregory, Reference Thornton and Gregory2012), nonhuman animal research that suggests over expression of a lin28b homologue results in a phenotype that resembles a slow life history strategy (i.e., larger growth and later reproduction; Zhu et al., Reference Zhu, Shah, Shyh-Chang, Shinoda, Einhorn, Viswanathan and Daley2010), and functional studies that link LIN28B with gene expression via the lin28/let-7 pathway that is key for metabolic regulation (Zhu et al., Reference Zhu, Shah, Shyh-Chang, Shinoda, Einhorn, Viswanathan and Daley2010). Nonetheless, the precise biological mechanism that would directly link father absence to LIN28B regulation via G×E is unknown.
Recent research suggests LIN28B has a more fundamental role in regulating developmental processes in humans and other animals beyond metabolic regulation (Leinonen, Chen, Tukiainen, Panula, & Widen, Reference Leinonen, Chen, Tukiainen, Panula and Widen2019). LIN28 genes are found in all metazoan organisms, are highly pleiotropic, and are critical for stem cell differentiation and other developmental processes across disparate species (Moss & Tang, Reference Moss and Tang2003; Pasquinelli et al., Reference Pasquinelli, Reinhart, Slack, Martindale, Kuroda, Maller and Ruvkun2000). Functionally, lin28 proteins produced by LIN28 genes bind to let-7 micro-RNA (miRNA) precursors preventing their biogenesis. miRNAs like let-7 regulate the expression of other genes post-transcriptionally by binding to messenger RNA (mRNA) and interfering with translation. Accumulating research suggests the lin28/let-7 axis plays a role in hypothalamic-pituitary-gonadal (HPG) axis regulation relevant for pubertal timing. More specifically, research on rodents has found that lin28b and its homolog lin28a are highly expressed in hypothalamic, pituitary, and gonadal tissues (see Corre et al., Reference Corre, Shinoda, Zhu, Cousminer, Crossman, Bellissimo and Palmert2016). lin28b mRNA, indicating lin28b expression, is abundant in the hypothalamus of neonatal mice and declines with age, reaching a low point prior to puberty. At the same time, levels of let-7 are also low during the neonatal period and increase during development (Sangiao-Alvarellos et al., Reference Sangiao-Alvarellos, Manfredi-Lozano, Ruiz-Pino, Navarro, Sanchez-Garrido, Leon and Tena-Sempere2013). This research suggests that reduced expression of lin28b with age may lead to higher let-7 availability in the hypothalamus, which could repress the expression of other genes that serve as a “break” for hypothalamic onset of puberty (Aylwin, Toro, Shirtcliff, & Lomniczi, Reference Aylwin, Toro, Shirtcliff and Lomniczi2019; Plant, Reference Plant2001).
Research on the lin28/let-7 axis in humans is sparse, however, though LIN28B mRNA expression in human hypothalamic and pituitary tissue is related to the LIN28B rs7759938 C-allele, which is in high linkage disequilibrium with the rs314273 T-allele (R 2 = 1.0; ldlink.nci.nih.gov), a focal SNP in the current study (Leinonen et al., Reference Leinonen, Chen, Tukiainen, Panula and Widen2019). Thus, it is hypothesized, though not yet tested, that the T alleles of the LIN28B SNPs tested herein would be similarly associated with increased LIN28B expression during development and that early father absence exposure (and/or related factors) would be related to reduced expression. Research on early environmental exposures in and around the family, such as father absence and associated stressors, also points to hypothalamic regulation as a possible mechanism for earlier puberty via hypothalamus–pituitary–adrenal (HPA) axis regulation (Ellis, Essex, & Boyce, Reference Ellis, Essex and Boyce2005; Essex, Klein, Cho, & Kalin, Reference Essex, Klein, Cho and Kalin2002; Mackrell et al., Reference Mackrell, Sheikh, Kotelnikova, Kryski, Jordan, Singh and Hayden2014). Few studies, however, have simultaneously tested the links between early stress, HPA regulation, and pubertal development (Belsky, Ruttle, Boyce, Armstrong, & Essex, Reference Belsky, Ruttle, Boyce, Armstrong and Essex2015) and no studies have looked specifically at father absence and AAM. Additional research is needed on possible environmental regulation—by not only father absence but also broader indices of environmental factors key to life history strategy development—of the lin28/let-7 pathway in humans and its implications for hypothalamic onset of puberty.
Also notable are two additional interesting and somewhat unexpected patterns that emerged in the current study. First was the earlier AAM associated with the rs364663 A/A genotype among father-absent girls. It is unclear what might be driving this finding but an admittedly post-hoc explanation could be heterozygote advantage or so-called “hybrid vigor” (Birchler, Yao, & Chudalayandi, Reference Birchler, Yao and Chudalayandi2006). Hybrid vigor refers to the evolutionary condition where a hybrid organism has higher fitness by virtue of heterozygosity compared to homozygotes of the same species. A classic example is malaria resistance found in humans heterozygous for sickle-cell anemia genetic risk (Tchuenche, Reference Tchuenche2007). Although purely speculative, perhaps heterozygosity at rs364663 might convey resistance to environmental factors that lead to earlier AAM, such as father absence. Additional research, including further replication of the current findings, is needed to explore this and other possible explanations.
A second interesting observation of these results is the possibility that LIN28B genotypes that show later AAM among father-present girls may also be related to earlier AAM among father-absent girls, in an ostensibly “for better and for worse” manner (Belsky, Bakermans-Kranenburg, & van IJzendoorn, Reference Belsky, Bakermans-Kranenburg and van IJzendoorn2007). The findings of the present study most consistently support the proposition that LIN28B genotypes are related to later AAM at menarche in father-present households, however the overall pattern of results (as depicted in Figures 2 and 3) are at least suggestive that LIN28B variants may be related to earlier AAM in father-absent households. Indeed, given that relatively smaller number of participants who reported being father absent, failure to statistically detect this pattern may be the result of relatively low power within this group rather than true lack of association. Additional research with even larger samples will be needed to further evaluate this possibility.
Last, the third goal of this study was to expand prior G×E research on father absence and AAM by testing moderation using an empirically derived PGS score. Main effect results were consistent with path model findings, though no G×E was detected. Based on these findings, a critical question is why G×E was detected for LIN28B and not for the PGS? One explanation is that the LIN28B G×E is a false positive. We view this interpretation as unlikely given there is now primary and replication evidence that suggests otherwise. In addition, the mechanistic and evolutionary research that link LIN28B and father absence as well as qualities unique to this research question, such as the strong theoretical foundation and measurement features (see Schlomer & Cho, Reference Schlomer and Cho2017 for a discussion), suggest that the LIN28B interaction with father absence is reliable. It is possible that LIN28B and father absence influence AAM through a common biological pathway, such as HPG/HPA regulation, and that the PGS, which reflects a broader range of biological processes related to menarche, is a more general measure that is effective at detecting phenotypic main effects but perhaps less well-suited for G×E when specific pathways transact with specific environments.
Another possibility is that there may be nonlinear associations between AAM and SNPs that make up the PGSs. PGSs assume additive linearity whereas the current data and the data from Schlomer and Cho (Reference Schlomer and Cho2017) as well as from others (Aliev et al., Reference Aliev, Latendresse, Bacanu, Neale and Dick2014) indicate that linear associations between SNPs and phenotypes may not always be the most precise model. To the degree that linearity assumptions are untrue, PGSs will underestimate phenotypic associations and additionally undermine moderation if the interaction is nonlinear as well, as was the case with the LIN28B findings. Indeed, at least some of the missing heritability issue might be due to nonlinear SNP associations. To improve PGS prediction, nonlinearity would need to be addressed at the SNP level prior to aggregation, though there are no established methods for doing so. We attempted to evaluate PGS nonlinearity using polynomial interactions in this study, but this approach does not address the potential issue at the SNP-level.
Last, it is currently on open question about whether we should expect PGSs to be moderated by environmental contexts at all. There has been a justified push for some time in psychological research to move away from candidate gene studies in favor of more polygenic approaches given PGSs have greater predictive power and reflect the biological reality that many genes of small effect underlay complex phenotypes. While PGSs are more powerful for detecting phenotypic main effects, there are some concerns about evaluating G×E. For instance, creating PGSs using methods that do not require stringent genome-wide significance thresholds, such as the Purcell method (International Schizophrenia Consortium, Reference Consortium2009), could lead to more error prone genetic variables given the goal of this approach is to find the signal-to-noise ratio that maximizes main effect prediction (Evans, Visscher, & Wray, Reference Evans, Visscher and Wray2009). Noisy genetic variables could lead to capitalizing on chance and spurious G×E, an issue that has been brought up many times in the candidate gene literature (Dick et al., Reference Dick, Agrawal, Keller, Adkins, Aliev, Monroe and Sher2015). Another issue of PGSs when it comes to G×E is that if markers within a score are moderated in different directions, an overall null effect could be detected (Belsky & Israel, Reference Belsky and Israel2014). It is possible that many G×E's using a PGS approach may simply not be found despite widespread acknowledgement that genes and environments work in tandem. Finally, by design PGSs only include SNPs that show a strong enough association in a given sample size to meet a given significance threshold. This also means that the main effect of these SNPs are able to penetrate through considerable environmental variability represented by the hundreds of thousands or more participants that typically make up GWAS samples. Given these properties, it may be the case that we should not expect to find G×E with SNP sets that are arguably unlikely to demonstrate conditional associations. From a differential susceptibility perspective, however, PGSs that capture developmental plasticity will be more likely to show environmental moderation. Moving forward, these issues will need to be addressed along with PGS replication research to avoid these studies leading to another body of literature that appears to be largely unreplicable.
Limitations and Conclusions
The findings of the current study should be evaluated in light of its limitations. Perhaps most salient is the homogenous sample, consisting entirely of European ancestry individuals. The generalizability of the current findings may be limited to this population and thus additional research with more diverse samples is needed. Nonetheless, the homogenous ancestry of the current sample could also be considered a strength since population stratification is unlikely to explain these results. Another possible limitation was that the PGS was constructed using weights from a meta-analytic GWAS (Day et al., Reference Day, Thompson, Helgason, Chasman, Finucane, Sulem and Perry2017)—a discovery sample that included the present sample in it. Although ALSPAC only contributed 3% of the participants in the discovery GWAS, this may inflate the effect of the PGS reported here. Sensitivity analyses in a similar study with a different pubertal outcome (timing and tempo of secondary sex characteristics; Horvath et al., Reference Horvath, Knopik and Marceau2019) suggested that this bias was negligible.
The present study replicated and extended recent genomic research on the association of father absence and AAM. We confirmed that puberty-related genes, in terms of a PGS, do not confound father absence/AAM associations. To the extent that there is some genetic confounding of the association, other biological mechanisms must be involved. In addition, we replicated the effects of a specific gene, LIN28B, which is frequently implicated in the timing of pubertal development and its effect is modified by father absence in that having a T allele (presumably resulting in greater LIN28B expression hypothalamic and pituitary tissues) is more predictive of later puberty among father-present girls, but this puberty-delaying effect is absent or possibly even reversed (e.g., related to earlier puberty) in father-absent girls. However, this finding did not extend to the PGS, which has two possible implications: (a) the additive construction of the PGS from multiple SNPs is inappropriate to understanding the effects of father absence on AAM and (b) LIN28B has a unique mechanistic role in the timing of puberty that is particularly open to early environmental influences, consistent with life history theory. Human studies on the mechanisms of LIN28B for puberty are scarce, though there is some evidence on which to hypothesize that the effects found here could be mediated through increased LIN28B expression in the hypothalamus and pituitary gland. Thus, LIN28B and the lin28/let-7 pathway particularly as expressed in the hypothalamus and pituitary may be the best target for future research aiming to understand the precise biological mechanisms that would link father absence to AAM via G×E.
Acknowledgements
We are extremely grateful to all the families who took part in this study, the midwives for their help in recruiting them, and the whole ALSPAC team, which includes interviewers, computer and laboratory technicians, clerical workers, research scientists, volunteers, managers, receptionists and nurses.
Funding
The UK Medical Research Council and Wellcome (Grant ref: 102215/2/13/2) and the University of Bristol provide core support for ALSPAC. This publication is the work of the authors and GLS and KM will serve as guarantors for the contents of this paper.
A comprehensive list of grants funding is available on the ALSPAC website (http://www.bristol.ac.uk/alspac/external/documents/grant-acknowledgements.pdf)
GWAS data were generated by Sample Logistics and Genotyping Facilities at Wellcome Sanger Institute and LabCorp (Laboratory Corporation of America) using support from 23andMe.
This research was additionally supported by K01DA039288 to KM.