1. Introduction
The magnitude of global fuel subsidy is massive (Bast et al., Reference Bast, Kretzmann, Krishnaswamy and Romine2012), and many countries that have been subsidizing fuel have decided to lift their subsidies. Ethiopia is one of the African countries to have done so when it removed all subsidies in October 2008 (IMF, 2009). Similar reforms have been carried out in Ghana, Egypt, Senegal, and Nigeria.
Despite its importance, there are very few studies that analyze the impact on trade of fuel subsidy reforms. One of the key channels through which the removal of fuel subsidies would have economy-wide effects is the transportation cost channel, i.e. by increasing shipping costs both internationally and within a country. In a country such as Ethiopia, where smallholder farmers rely on diesel trucks to transport produce to urban centers (Rancourt et al., Reference Rancourt, Bellavance and Goentzel2014; Adam, Reference Adam2011), removing a fuel subsidy could have a huge impact on food price dispersion (i.e. differences in prices of the same commodity across markets) and market integration.Footnote 1 The price dispersion could have significant welfare implications for those adversely affected by the fuel subsidy reform, such as net-producer farmers in remote rural areas from which shipping grains become expensive (Fuje, Reference Fuje2018). Urban net-buyers likely face higher prices and would lose from the high price dispersion. As observed in some countries, such as Nigeria in 2012, fuel subsidy reforms, if not well managed, are met with huge resistance and could pose a serious political economy challenge for policy makers.
There is a strong correlation between agricultural commodity prices and crude oil price globally, and their relationship has attracted considerable research attention due to the increased interest in biofuel, food price shocks, and the 2007/08 spike in oil price. Their long-run relationship, and causality from one to the other has been a subject of much contention. Zhang et al. (Reference Zhang, Lohr, Escalante and Wetzstein2009), Zhang et al. (Reference Zhang, Lohr, Escalante and Wetzstein2010), and Dillon and Barrett (Reference Dillon and Barrett2015) found no systematic, long-run relationship between fuel and grain prices in the international market. Similarly, analyses of monthly US price data have shown that there is no direct long-run relationship between fuel price and the price of maize, soybean, rice, sugar, and wheat (Zhang et al., Reference Zhang, Lohr, Escalante and Wetzstein2009; Zhang et al, Reference Zhang, Lohr, Escalante and Wetzstein2010). Zilberman et al. (Reference Zilberman, Hochman, Rajagopal, Sexton and Timilsina2013) also reviewed recent works on the topic, and concluded that biofuel prices have minimal impact on grain prices.
However, others find that fuel prices are transmitted into food prices (Nazlioglu et al., Reference Nazlioglu, Erdem and Soytas2013; Chen et al, Reference Chen, Kuo and Chen2010; and Mitchell, Reference Mitchell2008). In the US, high energy costs (2002–2007) contributed to 11.5% of the increases in production costs of maize, soybeans, but wheat (Mitchell, Reference Mitchell2008). Nazlioglu et al. (Reference Nazlioglu, Erdem and Soytas2013) documented that fuel price shocks were transmitted to agricultural commodity prices after the food price crisis (post-2005), and no such transmission risk existed before the crisis. Chen et al. (Reference Chen, Kuo and Chen2010) also found that crude oil price volatility significantly influenced prices of maize, soybean, and wheat.
In the investigation of the short-run dynamics and long-run relationship between the price of crude oil and food prices, the focus has predominantly been on assessing the substitution effects of biofuel as an alternative source of energy and/or fossil fuel as an input in grain production. Most of the studies cited above also focused on understanding these channels. However, in the majority of African countries, neither of these channels is relevant in explaining local grain prices, their spatial dispersion, and relationship with fossil fuel price volatility. The most important driver of this grain price–fuel price nexus is cost of transporting grains from farms to urban centers.
Some of the recent studies have examined the transportation cost channel and its relevance in explaining spatial dispersion and volatility of grain prices when the oil price changes. Distances from the national capital, high agriculture potential areas, and major ports of entry (for imported grains), coupled with high fuel cost, dictate spatial variation in grain prices, integration into global markets, price pass-through from global to local markets, welfare and overall economic development (see Dillon and Barrett (Reference Dillon and Barrett2015); Mitchell (Reference Mitchell2008); Storeygard (Reference Storeygard2016); Fuje (Reference Fuje2018) and Adam (Reference Adam2011)). Co-movement of oil and maize prices might be due as much, or more, to changes in shipping costs than to changes in the global prices of the grains. In the breadbasket markets of Eastern Africa, fuel price increase makes shipping grains from these markets to national centers less profitable and drive the price down (Dillon and Barrett, Reference Dillon and Barrett2015). Therefore, high transport cost somehow insulates national grain markets that are located farther from port of entry from global grain market (Adam, Reference Adam2011; Dillon and Barrett, Reference Dillon and Barrett2015). Similarly, during 2002–2007 period, transport costs added about 10.2% to the export prices of maize and wheat in the US (Mitchell, Reference Mitchell2008). Following global oil price shocks, cities located farther from ports have registered lower development than comparable cities by the ports (Storeygard, Reference Storeygard2016). Analysis of the 2008 fuel subsidy reform in Ethiopia shows that farmers located farther from major consumer centers faced lower prices for their produce and experienced welfare losses (Fuje, Reference Fuje2018).
However, analyses that are based on a simple observation of the global crude price and its association with local grain prices might overlook country-specific policies that might have been protecting the domestic transportation sector from global fuel price shocks. For instance, during the 2007–2008 global oil price shock, the domestic fuel price in Ethiopia remained stable due to the government's commitment to price stabilization through fuel subsidy. When the subsidy was lifted later, the price of different types of fossil fuels changed differently (e.g. gasoline price was increased by a much smaller percentage than diesel). In addition, for a country such as Ethiopia, where grain imports and exports are generally limited, the focus when assessing the transport cost channel must be based on analyzing price dispersion from local grain producing areas to consumer centers within the national boundary instead of price at port of entry and local consumer centers. The share of grain imports and exports in total consumption/production is quite small. Even though agriculture is subsistence, the urban centers rely heavily on supply of grains from rural areas, instead of imported grains.
This study analyzes the impact of fuel subsidy reform in Ethiopia using monthly grain prices from about 300 markets between 1996 and 2013. As noted above, Ethiopia removed its subsidy in October 2008, and diesel price increased by 39% (see Fuje (Reference Fuje2018) for detail description of the reform). The increase in the price of diesel likely caused a surge in transportation cost, and hence drove a wedge between prices observed in rural areas and urban centers. It should be noted that analysis of the impact of fuel subsidy reform on prices of other commodities must identify the key energy sources used for production and transportation of the goods in question. Even if mechanized agriculture is not common in Ethiopia, diesel is a key energy source for transportation of grains within the country. Hence, the purpose of this study is to investigate the change in spatial price dispersion associated with the increase in diesel prices.
Spatial DID on distance from the major national market in the capital is employed to causally attribute changes in agricultural commodity price dispersion across markets to fuel price increases following the policy reform. To conduct this estimation, I use GIS network analysis to impute the driving distance between each rural market and Addis Abeba (aka Addis), which is the national and economic capital. This distance is used as a continuous treatment variable because the impact of high fuel cost on freight costs (and hence on grain price dispersion) is expected to be cumulatively higher the farther a market is from the capital, holding other factors constant.
The results show that the removal of fuel subsidy has increased dispersion of grain prices observed in rural markets and the price paid by consumers in the capital city. For all of the five grains analyzed in this study, I find that the reform increased price dispersion. In effect, farmers selling their produces in remote rural markets likely faced lower prices for their produces, and/or consumers in the capital city paid higher prices for goods shipped from farther markets. This is important evidence for one of key channels through which fuel subsidy reforms could have economy wide indirect effects.
The remainder of the paper is organized into two sections: section 2 discuss the methodology, data and empirical results; and section 3 presents a brief discussion of the results and policy recommendation.
2. Empirical Approach, Data and Result
2.1 Spatial Difference-in-Difference
From the law of one price, we know that price difference between a rural market (i) and the market in the capital (j) at a given time (t), Pjt − Pit, is a function of transportation cost (Tijt) and associated transaction costs. The fuel subsidy reform basically increases the cost of transportation and hence could increase the dispersion between price in rural markets and the price prevailing in the capital city.
The removal of the fuel subsidy increased the price of diesel, which is an important component of grain trade cost in all parts of the country. This, however, does not mean that all markets would be affected equally by the increase in fuel cost. The reform could affect the markets in the remote districts and those near Addis differently. In fact, transportation cost (because of higher fuel price) are cumulatively higher the farther a district is from major consumer centers. For instance, holding other factors (such as agricultural potential, road infrastructure, consumption behavior, etc.) constant, markets within 100 km from the capital would face very little increase in transportation cost relative to, say, those 400 km away. I leverage this fact to conduct sDID estimation, by using distance from Addis as a continuous treatment variable. The driving distance on the existing road network is imputed using GIS network analysis.
Specifically, the sDID involves estimating the equation below:

where Yit is the absolute value of difference between grain price Addis and prices of the same grain in rural market i at time t (henceforth price dispersion). Distancei is the total driving distance (in kilometers) from market i to Addis. Postt is a dummy variable equal to 1 if the time is after October 2008, and 0 otherwise. And X is a vector of other covariates, including commodity grade, month of the year (MOY) fixed effects (FEs), region FEs, and the market's distance from the regional capital or another major regional city.
This framework is used to estimate the impact of removing fuel subsidy on price dispersion between Addis and rural markets.Footnote 2 Surplus grain producing districts that are close to the capital are expected to experience lesser difference in relative price, relative to Addis. Whereas, shipping grains from surplus grain-producing districts that are located farther away is anticipated to become more expensive; hence price differential between these districts and the capital to increase. On the other hand, remote districts with low agriculture potential, and hence purchase grains from other districts, might face increases in relative price.
One might argue that not only distance from national capital, but also distance from other major markets within the country, could be relevant. Even if Addis is the economic powerhouse, there is at least one major consumer center in each region, which is mainly the regional capital. As a robustness check, I re-estimate equation (1) using population weighted distance between market i and Addis as well as distance from the corresponding regional capital.
2.2 Data
The study uses monthly grain price data collected by the Central Statistics Agency in its Producer Price and Retail Price Surveys. For rural markets, producer/farmgate price is used. While, the median retail price in the capital is used as an indicator of the price of the same quality grain in the major consumer center of the country. The study period is between 1996 and 2013. Prices of five major cereals – teff, wheat, barley, maize, and sorghum3 – are analyzed. Table 1 presents the number of markets and price observations for these five grains.
Table 1. The number of markets and total number of observations
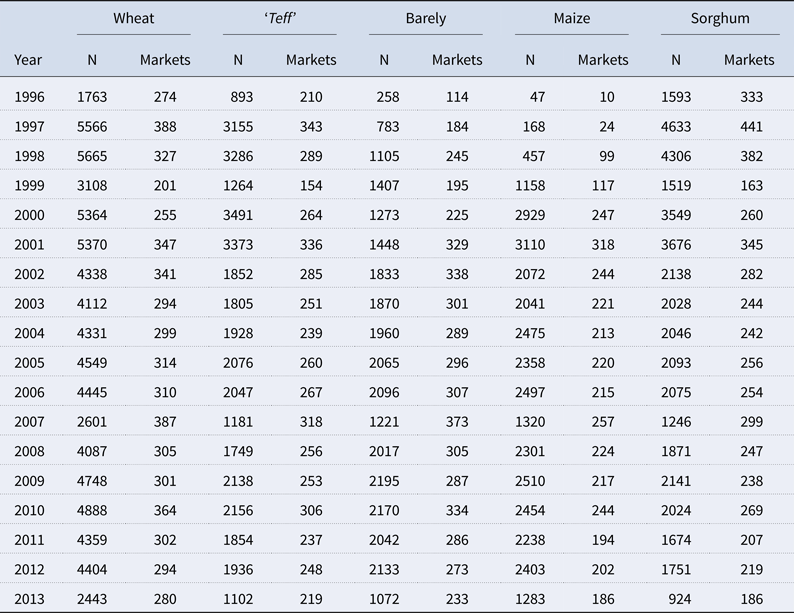
Note: N stands for number of observations. Markets represents the number of districts from which price data was collected.
Distance of the 300 rural markets from Addis and the regional capital is imputed using road network data from the Ethiopian Road Authority. The imputation of distance is done using GIS network analysis by taking the centroid of the district in which a rural market is located as a starting point. Figure 1 shows the centroids of the districts, the road network, and location of the major consumer centers/cities. Some of the district centroids are located off the road network. In such cases, Euclidean distance to the nearest road is added to the total distance on road network.

Figure 1. Location of markets and road network.
2.3 Empirical Results
Before proceeding into the discussion of sDID estimation results, I present a brief description of the price dispersion and the change following the reform across markets at different distances. Figure 2 shows the price dispersion before and after the reform for markets at various traveling distances from Addis.

Figure 2. Price dispersion between Addis and other markets, before and after the reform.
Prior to the 2008 fuel subsidy reform, grain price dispersion was generally low, and the relationship with distance from Addis was quite weak in that the dispersion did not increase substantially as one goes farther from Addis. As noted above, it is anticipated that the removal of fuel subsidy would affect grain price patterns in districts that are close to the capital and those farther away differently. Those districts that are near the capital would face a very small increase in transport costs, relative to remote districts. With other factors, such as agricultural potential, distance from other markets, local demand/consumption patterns, and so on, remaining constant, the change in price dispersion is anticipated to be lower for nearby districts than those farther from Addis, the major consumer center in the country. Figure 2 shows that, after the fuel subsidy reform, price dispersion increased. In addition, and more importantly, dispersion was higher for markets located farther from Addis.
In addition, there is an interesting price dispersion pattern that holds across the five grains. For districts within 500 km from the capital, we observe a widening gap between pre- and post-reform price dispersion as we go farther away from the capital. For districts that are very far from the capital (by more than 500 km), we observe a narrowing gap in (pre- and post-reform) median price dispersion as we go farther from the capital. These districts, which are close to borders and also tend to have relatively lower agricultural potential, are likely to be trading across national borders, instead of with the capital.Footnote 3 As a result, the distance from markets across the borders might be more relevant than the distance from Addis. This explains the smaller gaps between pre- and post-reform price dispersion for the districts, say, 900 km away from the capital. In addition, in almost all directions, districts closer to the border have lower agricultural potential. They tend to be net-buyers, purchase their grains from nearby highland farming districts.Footnote 4 Therefore, another relevant distance might be the distances from these highlands. Finally, districts closer to the border might, in general, face higher prices both before and after the reform due to the more vibrant economic activities (relative to other remote districts) close to the borders. This might explain the higher prices – comparable to those prevailing in the capital – in these very distant, border districts.
As discussed above, the spatial DID estimation is implemented using distance from the capital as a continuous treatment variable. The impact of the reform on spatial price dispersion is represented by π3, the coefficient of Post ∗ Distance in equation (1). The only difference between standard DID and the spatial DID is that in the latter specification, the treatment variable is continuous and hence the estimated impacts will vary based the location of the treated market, relative to Addis.
The results from sDID are presented in Table 2. I have controlled for other relevant covariates such as commodity grade, year FEs, MOY FEs, region FEs, and distance from the nearest major regional town. Standard errors are multi-way (district–year) clustered. The impact estimates capture the changes in price-dispersion due to the reform for every 100 km a district is from Addis.Footnote 5 The coefficient of the distance variable captures the difference in price dispersion among markets at different distances before the fuel subsidy reform. While, the coefficient of the post-treatment variable captures the price dispersion recorded after the fuel subsidy reform for all markets regardless of distance.
Table 2. Impact of the reform on price dispersion (ETB/MT): sDID result

Note: Multi-way cluster (year and district) robust standard errors (see Cameron et al.,2008) in parentheses: *** p < 0.01, ** p < 0.05, * p < 0.1. Covariates included as control variables are commodity grade, MOY FEs, region FEs, and the district's distance from the regional capital or another major regional town.
The reform increased price dispersion for all of the five grains analyzed in this study. Because of the reform, the price dispersion for teff, wheat, maize, sorghum, and barley increased by 103, 99, 98, 204, and 159 Ethiopian Birr (ETB)/metric ton (MT) for every 100 kilometer a market is farther from Addis, respectively. This is quite a substantial increase considering the fact that the average distance of the markets/districts is about 350 kilometers. Farmers selling their produce in markets very far from the capital likely faced much lower price than the price prevailing in Addis. Similarly, consumers in the capital could face much higher prices for grains shipped from surplus markets farther away.
The estimates also reveal that, before the reform, there was minimal differences in price dispersion between markets at different distances from the capital. Except for teff, the coefficient of the distance variable is insignificant. In addition, most of the price dispersion observed among markets in the county occurred after the October 2008 reform. The coefficient of the post-reform dummy is statistically significant and much larger in magnitude.
The result is robust to changes in clustering of standard errors and the use of population weighted distance from multiple consumer centers. Clustering standard errors at district/market level, instead of a multi-way (district–year), does not change the statistical significance and the results are presented in Table 3.
Table 3. Impact of the reform on price dispersion

Note: (District) Clustered robust standard errors in parentheses: *** p < 0.01, ** p < 0.05, * p < 0.1.
In addition, the result is robust to changes in the relevant distance. In the main specification, distance of each district from the national capital was used as a continuous treatment variable, while distance from the nearest regional city was a covariate. The main argument for doing so was that Addis is the country's major consumer center and its population is far larger than that of the next nine regional cities combined. One might, however, argue that distances from the regional cities could also matter. To investigate this, I constructed a weighted distance indicator by weighting the distance of districts from Addis and the corresponding regional cities by the population size of the capital and the regional city.
The re-estimation of equation (1) using population weighted distance from the corresponding regional capital and Addis does not change the overall message that the reform increased price dispersion for markets farther from major consumer centers. The results (Table 4) show that markets far from either regional and/or nation consumer centers experience larger price gaps, relative to the price prevailing in Addis.
Table 4. Impact estimates, with population weighted distance as a treatment variable

Notes: Multi-way cluster (year and district) robust standard errors (see Cameron et al, Reference Cameron, Gelbach and Miller2008) in parentheses: *** p < 0.01, ** p < 0.05, * p < 0.1.
Covariates included as control variables are commodity grade, MOY FEs, region FEs, and the district's distance from the regional capital or another major regional town.
The change in relative prices will have heterogenous impacts on welfare of households depending on their net-buyer/net-seller status and relative locations from major consumer centers. This is well documented in Fuje (Reference Fuje2018). Rural net-seller farmers that are farther from Addis have experienced higher welfare losses. The losses were larger for farmers in the upper two income/consumption quartiles. The urban residents, who tend to be net-buyers, experienced welfare losses because of higher food prices.
3. Conclusion and Policy Implications
In this study, I examine an important but a less understood issue: the impact of fuel subsidy reform on grain price dispersion through its effects on transportation cost. Even though this is highly relevant for policy makers, there are very few studies that analyze the trade impact of such reforms. A key channel through which the removal of fuel subsidy would have economy-wide effects is the transportation cost channel, i.e. by increasing domestic and international shipping cost.
To study the trade impact of fuel subsidy reform, I compile monthly grain price data from 300 markets in rural Ethiopia during 1996–2013, and analyze the relative price in rural areas against the prices prevailing in major consumer centers. The analysis leverages the distances of rural markets from Addis to conduct sDID analysis, by using distance as a treatment variable.
The results show that the removal of fuel subsidy has caused a sharp increase in the dispersion of grain prices observed in rural markets and the price paid by consumers in the capital city. Markets that are located farther from the capital likely have higher transportation costs and hence larger increases in price dispersion. This increase in price dispersion could be due to either increase in prices paid by consumers in Addis and/or a decline in prices received by farmers in remote rural areas. This is an important evidence for one of key channels through which fuel subsidy reforms could have economy wide indirect effects.
To ease the impacts of such reforms, policy makers could devise short-term and long-term programs that protect adversely affected poor farmers and urban residents. For instance, as partially perused in Ethiopia, providing a temporary food subsidy to the urban poor could be a tool to manage the short-term unintended consequences of this reform. In rural areas, providing market information could improve farmers’ chance of earning higher prices in alternative markets. A rather long-term solution is to transform the transportation sector by introducing more cost-effective shipping systems such as railways, preferably powered through green energy sources such as hydro power.