1 Introduction
There has been tremendous worldwide growth in using computer-based methods for learning different language skills and components. One of the ultimate goals of computer-assisted language learning (CALL) is to provide learners with a good environment that facilitates acquiring communicative competence in the L2. Since the advent of Second Language Acquisition (SLA) theories, a number of crucial factors have been revealed for improving students’ productive conversational skills: (1) comprehensible input (Krashen, Reference Krashen1985), (2) comprehensible output (Swain, Reference Swain1985), (3) corrective feedback (Long, Reference Long2000), and (4) motivation and attitude (Masgoret & Gardner, Reference Masgoret and Gardner2003).
In relation to oral understanding, accumulated work on the process of listening suggests that comprehension can only occur when listeners place what they hear in context, i.e. the knowledge of who the participants are (sex, age, personality, relationship), the setting (where the linguistic situation takes place), the topic (the thing being talked about) and even the purpose (what language is used for) (Brown & Yule, Reference Brown and Yule1983; Byrne, Reference Byrne1986). What is really retained after understanding is not the literal meaning but some mental representation mainly provided by contextual information (Garrod, Reference Garrod1986). Hence it has become quite clear that in giving students comprehension activities out of context we set them a difficult task (Brown, Reference Brown1986).
While comprehensible input is invaluable to the acquisition process, it is not sufficient for students to fully develop their L2 proficiency. The output hypothesis claims that production makes the learner move from ‘semantic processing’ prevalent in comprehension to more ‘syntactic processing’ that is necessary for improving accuracy in their interlanguage (Swain, Reference Swain1985). Specifically, producing output is one way of testing one's hypotheses about the L2. Learners can judge the comprehensibility and linguistic well-formedness of their interlanguage utterances against feedback obtained from their interlocutors, leading them to recognize what they do not know, or know only partially. The recognition of problems may then prompt the learners to attend to the relevant information in the input, which Schmidt (Reference Schmidt2001) claims to be “the first step in language building.” Additionally, output processes enable learners not only to reveal their hypotheses, but also to reflect on them using language. Reflection on language may deepen the learners’ awareness of forms, rules, and form-function relationships if the context of production is communicative in nature.
On the other hand, it has been argued that corrective feedback plays a beneficial role in facilitating the acquisition of certain L2 forms which may be difficult to learn through input alone, including forms that are rare, are low in perceptual salience, are semantically redundant, do not typically lead to communication breakdown, or lack a clear form-meaning relationship. Johnson (Reference Johnson1992) contends that if there is no concern for feedback in terms of linguistic correctness, meaning-based activities per se may accelerate language progress but in the long term lead to “fluent but fossilised students.”
Motivation and attitude is another crucial factor in L2 achievement (Masgoret & Gardner, Reference Masgoret and Gardner2003). For this reason it is important to identify both the types and combinations of motivation that assist in the successful acquisition of a foreign language. In order to make the language learning process a more motivating experience, instructors need to put a great deal of thought into developing programs which maintain student interest and have obtainable short term goals. The use of an interesting computer-based method can help to increase the motivation level of students, and computer-based learning has an advantage over human-based learning in that it seems to be a more relaxed atmosphere for language learning (Liang & McQueen, Reference Liang and McQueen1999; Roed, Reference Roed2003; Yi & Majima, Reference Yi and Majima1993).
There have been few serious attempts to provide students with natural contexts that embody most of the aforementioned attributes. Therefore, we have provided an opportunity to learn English in an immersive environment in which learners experience free conversations about everyday life in real situations with intelligent robots. They can perceive the utterances of learners, especially Korean learners of English, and can provide corrective feedback to erroneous utterances. Recent development of robot-related technologies has drawn attention to the utilization of robots in real life, and increased interest in robots can give students integrative motivation to have a successful conversation with a robot. A major purpose of this investigation is to estimate the magnitude of the contributions that robot-assisted language learning (RALL) makes to the achievement of oral skills in the foreign language.
The remainder of this paper is structured as follows: Section 2 describes related studies; Section 3 introduces the technologies for Human Robot Interaction (HRI); Section 4 presents a detailed description of the experimental design; Section 5 includes the results and discussion, and finally, Section 6 gives our conclusion.
2 Related work
Computers have been viewed as a potentially beneficial tool for second language learning for several decades. With the explosion of Internet communication tools, several computer-mediated communication (CMC) contexts have emerged such as instant messages, e-mails, chat rooms and discussion boards. CMC is widely discussed in language learning because CMC provides opportunities for language learners to practise their language. Early CMC research qualified and quantified language production from a mainly socio-cultural perspective (learner-learner and learner-teacher interactions). In recent years, a number of studies have investigated the role of written feedback for L2 development and have found a positive relationship between feedback and L2 development (Bryan, Reference Bryan2005; Lai, Fei & Roots, Reference Lai, Fei and Roots2008; Lai & Zhao, Reference Lai and Zhao2006; Loewen & Erlam, Reference Loewen and Erlam2006; Sachs & Suh, Reference Sachs and Suh2007; Smith, Reference Smith2004).
With the advances in natural language processing (NLP) technologies, a number of intelligent computer-assisted language learning (ICALL) applications have emerged which employ sophisticated NLP techniques to provide dynamic, individualized feedback to learners’ errors. Contrary to CMC applications which rely solely on human-human interactions, ICALL applications play crucial roles of both interlocutors and teachers. For example, BANZAI (Nagata, Reference Nagata2002) employs artificial intelligence and NLP technology to enable learners to freely produce Japanese sentences and to provide detailed feedback concerning the specific nature of the learner's errors. E-tutor (Heift & Nicholson, Reference Heift and Nicholson2001; Heift & Schulze, Reference Heift and Schulze2007) also provides error-specific and individualized feedback by performing a linguistic analysis of student input and adjusting feedback messages suited to learner expertise. Many studies have shown that students learn better with feedback that explains the particular error they are making and that considers their knowledge of the language.
However, the systems employed in this line of investigation can be described as non-communicative in that the primary focus of task interaction was on linguistic form, since no model of knowledge representation was present to facilitate meaning-focused exchanges. In recent years, there has been a shift in CALL research towards conversational interaction. This trend has been motivated by rapid globalization and great emphasis on communicative competence in the target language in a variety of situations. Recent development of spoken dialog systems has enabled CALL systems to bear a closer resemblance to oral conversation than the earlier CALL applications. We call such a communicative ICALL system a Dialog-based CALL (DB-CALL) system. Many research projects have provided pronunciation training for oral skills using a speech recognizer in a forced recognition mode (Dalby & Kewley-Port, Reference Dalby and Kewley-Port2005; Neri, Cucchiarini & Strik, Reference Neri, Cucchiarini and Strik2001), but a few systems exist that allow the user to engage in some form of meaningful dialog with embodied or disembodied agents in virtual words. DEAL (Brusk, Wik & Hjalmarsson, Reference Brusk, Wik and Hjalmarsson2007) is a spoken dialog system for providing a multidisciplinary research platform, particularly in the areas of human-like utterance generation, game dialogue, and language learning. The domain is the trade domain, specifically a flea market situation. DEAL provides hints about things the user might try to say if he or she is having difficulties remembering the names of things, or if the conversation has stalled for other reasons. SPELL (Morton & Jack, Reference Morton and Jack2005) provides opportunities for learning languages in functional situations such as going to a restaurant, expressing (dis-)likes, etc. Recast feedback is provided if the learner's response is semantically correct but has some grammatical errors. SCILL (Seneff, Wang & Zhang, Reference Seneff, Wang and Zhang2004) covers the topics of weather information and hotel booking. Researchers also implemented the simulated user to produce example dialogs to expose language learners to language use and to expand the training corpus for the system. Let's Go (Raux & Eskenazi, Reference Raux and Eskenazi2004) is a spoken dialog system that provides a bus schedule for the area around Pittsburgh, PA, USA. The researchers modified an extant system for the native speaker to adapt non-native speakers’ data for the use of language learning. Modifications include the addition of new words, new constructs and the relaxation of some syntactic constraints to accept ungrammatical sentences. Within the DB-CALL literature, however, there has been a dearth of empirical research on the developmental benefits engendered by the task environments in this line of research. Most discussions of the publications are largely system descriptions.
Generally, it has been difficult to reconcile CALL research with SLA research due to contextual differences between computer-centered and human-centered tasks. Thus, making task conditions comparable to tasks performed under more traditional language learning conditions is one of the important challenges for CALL research. Unlike DB-CALL systems based on virtual worlds, robots as conversational agents bear a closer resemblance to human-centered tasks than DB-CALL applications because the only difference is the replacement of humans with robots in real life situations. In Japan, the educational use of robots has been studied, mostly with Robovie (Kanda, Hirano, Eaton & Ishiguro, Reference Kanda, Hirano, Eaton and Ishiguro2004) in elementary schools, focusing on English language learning. Robovie has one hundred behaviors. Seventy of them are interactive behaviors such as hugging, shaking hands, playing paper–scissors–rock, exercising, greeting, kissing, singing, briefly conversing. For the purpose of English education in this study, the robot could only speak and recognize English. In total, the robot could utter more than 300 sentences and recognize about 50 words. To identify the effects of a robot in English language learning, the researchers placed a robot in the first grade and sixth grade classrooms of an elementary school for two weeks, and compared the frequency of students’ interaction with their English test score. While the interaction between the children and the robots generally diminished in the second week, a few children sustained a relationship with the robot. The results showed that the amount of time children spent with the robot during the first week had no effect on their improvement in English by the second week, but the amount of time that children interacted with the robots during the second week did have a significant and positive impact on improvement in English in the second week. This implies that robots which can maintain long-term relationships with students can be effective for language learning. Yet, Robovie has tended to be extremely restrictive in the number of words it can recognize so that the conversations have been confined to a chain of short-time interactions. IROBI (Han, Jo, Park & Kim, Reference Han, Jo, Park and Kim2005) was recently introduced by Yujin Robotics in Korea. IROBI was specifically designed and trialled for tutoring and educational services. IROBI, which has a sitting child-like appearance, is designed with an LCD panel on its chest to support easy communication with children, allowing voice and touch screen input without face and gesture recognition. IROBI was used to compare the effects of non-computer-based media and web-based instruction with the effects of robot-assisted learning for children. Robot-assisted learning is thought to improve children's concentration, interest, and academic achievement. It is also thought to be more user-friendly than other types of instructional media. But the discourse context of IROBI is slightly different from DB-CALL applications in that language learners interact with the robot largely though the virtual agents displayed in the LCD panel. The physical actions of the robot are merely employed to magnify the expressive power of content displayed in the LCD panel. To the best of our knowledge, there have not been approaches combining authentic situations in the real world and real robots, which can provide a more realistic and active context than other approaches. Specifically, Engkey, the robot we developed, acts as a sales clerk in a fruit and vegetable store, and in a stationery store, so that it can interact in real life situations with language learners who play the part of customers. Given that studies on RALL are still relatively new and most are in the early stages, this study aims to find general and approximate effects of RALL which can motivate subsequent in-depth research. There is a need for much more research into the use of robots for educational purposes, and the effects of their use in this field.
The following section gives an account of the Human Robot Interaction (HRI) technologies used in the project.
3 Human Robot Interaction (HRI) technology
We developed our robots as educational assistants called Mero and Engkey. They were designed with expressive faces, and have typical face recognition and speech functions allowing them to communicate. Mero is a head-only robot. The penguin-like robot Engkey is 80 cm tall and weighs 90 kg, and is equipped with stereo vision. In recent robotics research, several pioneering studies have suggested that humans can also establish relationships with pet robots. Many people actively interact with animal-like pet robots (Friedman, Kahn & Hagman, Reference Friedman, Kahn and Hagman2003; Fujita, Reference Fujita2001; Wada, Shibata, Saito & Tanie, Reference Wada, Shibata, Saito and Tanie2002).
3.1 Speech and language processing
This section describes the speech and language processing component of the robots. At the high level, the speech and language processing component consists of a series of sub-components connected in a classical, pipeline architecture (see Figure 1). The audio signal for the user utterance is captured and passed through a speech recognition module that produces a recognition hypothesis (e.g. “apple”). The recognition hypothesis is then forwarded to a language understanding component that creates a corresponding semantic representation (e.g. [item = apple]). Next, the dialog manager integrates this semantic input into the current discourse context, and produces the next system action in the form of a semantic output (e.g. {request quantity}). A language generation module produces the corresponding surface form, which is subsequently passed to a speech synthesis module and rendered as audio output.

Fig. 1 The architecture of the speech and language processing component.
3.1.1 Automatic speech recognition
The goal of automatic speech recognition (ASR) is to map from an acoustic signal to a string of words. Modern general purpose speech recognition systems are based on Hidden Markov Models (HMM). They take an acoustic model (AM), a dictionary of word pronunciations, and a language model (LM) to output the most likely sequence of words. The AM computes the likelihood of the observed acoustic signal given linguistic units such as phones or subparts of words for each time frame. The dictionary is a list of word pronunciations, each pronunciation represented by a string of phones. The language model (generally an n-gramFootnote 1 grammar) expresses the probability that a given string of words is a sentence in English.
In this study, speech recognition is performed by the DARE recognizer (Ahn & Chung, Reference Ahn and Chung2004), a HMM-based speaker independent continuous speech recognizer. The target speech to recognize is the conversational speech of Korean elementary school students for shopping situations in a fruit and vegetable store, and a stationery store. Generally, speech is easier to recognize if the speaker is speaking a standard dialect, thus recognition is harder on foreign accented speech. Besides, it is rarely practical to collect enough training data to build an AM for a particular user group because it requires ASR experts to design a significant number of phonetically rich texts (hundreds of thousands of sentences), to record hundreds of hours of audio files with equal numbers of male and female speakers carefully chosen for diversity of voice quality and dialect. Therefore most previous studies on non-native speech recognition employed acoustic model adaptation techniques which improve the recognition performance with a small amount of non-native data (Goronzy, Reference Goronzy2002; Oh, Yoon & Kim, Reference Oh, Yoon and Kim2007). We used a small amount of Korean children's transcribed speech (17 hours) to adapt acoustic models that were originally trained on the Wall Street Journal corpus (Paul & Baker, Reference Paul and Baker1992) using standard adaptation techniques, both of maximum likelihood linear regression (MLLR) (Leggetter & Woodland, Reference Leggetter and Woodland1995) and maximum a posteriori (MAP) adaptation (Zavaliagkos, Schwartz & McDonough, Reference Zavaliagkos, Schwartz and McDonough1996). The Korean children's speech was collected by a hundred Korean elementary school students (equal numbers of female and male students) using educational materials for the shopping domain (Section 4.2) which include small talk, purchases, exchanges and refunds.
ASR systems usually expect words to be pronounced in a certain way. If they are pronounced differently, which happens frequently in non-native speech, the automatic system is incapable of relating the ‘wrong’ pronunciation to the right word. Solely applying speaker adaptation techniques is therefore not sufficient to achieve a satisfactory performance for non-native speakers, so an additional modification of the pronunciation dictionary is necessary. We detected the occurrence of pronunciation variants with a speech recognizer in forced-alignment using a lexicon expanded according to all the possible substitutions between confusable phonemes. Korean speakers tend to replace the following consonants with the correspondingly similar consonants; the eight pronunciation variants of vowels shown in Table 1 are common to Korean speakers.
Table 1 List of possible substitutions

While large-vocabulary ASR systems focus on transcribing any sentence on any topic, for domain-dependent dialog systems it is of little use to be able to transcribe such a wide variety of sentences. The sentences that the speech recognizer needs to be able to transcribe are just those that are related to an ongoing dialog context. We call such a dialog-state dependent LM a restrictive LM. When we require the system to improve recognition accuracy, we can use a restrictive LM, thus achieving better accuracy at the cost of input diversity. We made different LMs around combinations of the study theme (small talk, fruit and vegetable store, and stationery store) and the student's English proficiency level (beginner and intermediate). The speech recognizer loads and unloads LMs dynamically according to the student's English proficiency level and the study theme. The student's level is indicated by the radio frequency ID (RFID) person identification process when every student starts a learning session by scanning their RFID card (see Section 3.3). The study theme is updated by the dialog manager which tracks dialog states during a conversation (see Section 3.1.3).
The standard evaluation metric for speech recognition systems is word error rate (WER). When given a pair of the reference sentence (supposedly the correct one) and the recognized one, WER can be computed as: WER = (S + D + I)/N, where S is the number of substitutions, D deletions, I insertions, and N is the number of words in the reference. By virtue of adaptation of acoustic model and pronunciation dictionary, and use of restrictive grammars, the average WER was about 22.8% at the vocabulary size of 1250.
3.1.2 Spoken language understanding
The spoken language understanding (SLU) component of dialog systems must produce a semantic representation that is appropriate for the dialog task. Many speech-based dialog systems, since as far back as the GUS system (Bobrow, Kaplan, Kay, Norman, Thompson & Winograd, Reference Bobrow, Kaplan, Kay, Norman, Thompson and Winograd1977), are based on the frame-and-slot semantics. A shopping task would have a frame with slots for information about items and price, thus a sentence like “Here is twenty five dollars” might correspond to the following filled-out frame:
IntentionFootnote 2:
Speech Act: declare
Main Goal: payment
Additional Information:
Num: twenty five
Unit: dollar
To generate this semantic representation, some dialog systems use general-purpose unification grammars with semantic attachments (Shieber, Reference Shieber1986). Other dialog systems rely on simpler domain specific semantic analyzers, such as semantic grammars (Burton, Reference Burton1976). Since language learners commit numerous and diverse errors, CALL systems should be able to understand language learners’ utterances in spite of these obstacles. To accomplish this purpose, rule-based systems (i.e., general-purpose unification grammars and semantic grammars) usually anticipate error types and hand-craft a large number of error rules, but this approach makes these methods weak in dealing with ambiguity and insensitive to unexpected errors and diverse error combinations (Morton & Jack, Reference Morton and Jack2005; Raux & Eskenazi, Reference Raux and Eskenazi2004; Schneider & McCoy, Reference Schneider and McCoy1998). An alternative to rule-based systems that is probabilistic and also avoids hand-coding of grammars is machine learning-based techniques such as classification models and sequence labeling models. The task of classification is to take an utterance, extract some useful features describing the observation (e.g., bag of words, bag of n-grams), and then, based on these features, to classify the observation to one of a set of discrete classes (e.g., one of user's intentions).
There are often many ambiguities in interpreting a user's intention. For example, the following utterance looks like a yes-no question.
Can you give me a list of healthy foods?
In fact, however, this person was not interested in whether the system was capable of giving a list; this utterance was a polite form of a request. To resolve these ambiguities we need not only features from utterance itself but also features based on conversational context. In addition, the learners’ numerous and diverse errors can make the classification of user's intention even harder, so systems should rely more on conversational context, as human tutors do.
Therefore we statistically infer the actual learner's intention by taking into consideration not only the utterance itself but also the dialog context. We can achieve this goal by employing features from dialog context and utterances together to make a classification model for intention recognition. For CALL, however, such approaches can be problematic, because separate handling for each of the proficiency levels is important in a language learning setting. Given a dialog scenario, the dialog-context model is relatively invariant; thus we prefer a hybrid model that combines the utterance model and the dialog-context model in a factored form, as shown in Figure 2. This approach allows us to adjust the hybrid model to a required proficiency level by replacing only the utterance model (Lee, Lee, Lee, Noh & Lee, Reference Lee, Lee, Lee, Noh and Lee2010).
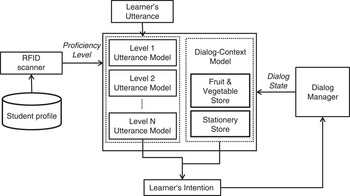
Fig. 2 Hybrid model of language understanding.
The hybrid model merges n-best hypothesesFootnote 3 from the utterance model with n-best hypotheses from the dialog-context model to find the best user's intention. In the language production process, user intentions are first derived from the dialog context; subsequently the user intentions determine utterances (Carroll, Reference Carroll2003). By using this dependency and the chain rule, the most likely expected user's intention I(U, D) given the utterance U and the dialog context D can be stated as follows:



By using Bayes’ rule, Eq. (3) can be reformulated as:

P(U), P(D), and P(U, D) can be ignored, because they are constant for all I (Eq. 5):

In this formula, P(I | U) represents the utterance model and P(I | D) represents the dialog-context model.
In order to distinguish the user's intention from the utterance itself, we use maximum entropy model (Ratnaparkhi, Reference Ratnaparkhi1998) trained on linguistically motivated features. The objective of this modeling is to find the I that maximizes the conditional probability, P(I | U) in Eq. (5), which is estimated using Eq.
(6):

where K is the number of features, fk denotes the features, λk the weighted parameters for features, and Z is a normalization factor. This model offers a clean way to combine diverse pieces of linguistic information. We used the following linguistic features for the utterance model:
• Lexical word features: Lexical word features consist of lexical trigrams using current, previous, and next lexical words. They are important features, but the lexical words appearing in training data are limited, so data sparseness problems can arise.
• Part-of-speech (POS) tag features: POS tag features also include POS tag trigrams matching the lexical features. POS tag features provide generalization power over the lexical features.
Determining the user's intention from the dialog state can be solved by finding similar dialog states within a dialog-state space (see Figure 3), which was inspired by example-based dialog modeling (Lee, Jung, Kim & Lee, Reference Lee, Jung, Kim and Lee2009). Each dialog segment is represented as one dialog state (Table 2). A dialog-state space is built by first collecting a dialog corpus. Semantic tags (e.g., speech act, main goal, and additional information) are then manually annotated to utterances. A hand-crafted automatic system is also used to extract discourse contextual features (e.g., previous intentions and exchanged information status) by keeping track of the dialog states for each point in the dialog. Then the possible user intentions can be selected from dialog states similar to the current dialog state. The best user's intention is obtained from the dialog state that maximizes the similarity.
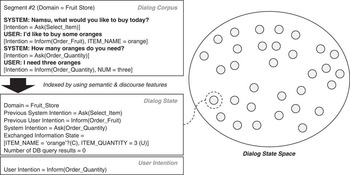
Fig. 3 Indexing scheme for building a dialog-state space for the shopping domain.
Table 2 Representation of dialog context and an example for the shopping domain

This idea can be formulated as the k-nearest neighbors (KNN) problem (Dasarathy, Reference Dasarathy1990) which provides high controllability for incrementally tuning the model during operation, which is in practical terms a very desirable property. The similarity function is defined as the following equation:

where D and D′ are dialog states, K is the number of features, fk denotes the feature functions, λk the weighted parameters for features. Our feature functions first include the simplest tests, whether a feature is shared or not, for each feature of a dialog context (Table 2). In addition, we include a number of feature functions based on general discourse and world knowledge. For example, if the system's intention is “inform(list_items)”, the number of database query results becomes an important feature. If the number of results is greater than one, the most likely expected user's intention would be “declare(select_item)”. If the number of results equals one, “delcare(buy_item)” would be the most probable intention. To let the dialog-context model be a probability distribution, the score function is divided by the normalization factor:

The task of sequence labeling is to assign a label to each element in some sequence, for which the assigned tags capture both the boundary and the type of any detected entities (e.g., values of additional information). This approach makes use of IOB encoding (Ramshaw & Marcus, Reference Ramshaw and Marcus1995); ‘I’ is used to label tokens inside an entity, ‘B’ is used to mark the beginning of an entity, and ‘O’ labels tokens outside any entity of interest. Consider the following sentence:
Here/O is/O twenty/B-NUM five/I-NUM dollars/B-UNIT
From the IOB tagging result, ‘twenty five’ is identified as a numerical expression and ‘dollars’ detected as a unit of money. To extract additional information, we use a linear-chain conditional random field (CRF) model (Lafferty, McCallum & Pereira, Reference Lafferty, McCallum and Pereira2001). A linear-chain CRF is defined as follows. The objective of this modeling is to find the S that maximizes the conditional probability, P(S | X) in which S = {St} and X = {Xt} for t = 1,…,T, such that S is a semantic class labeling of an observed word sequence X. The conditional probability is estimated using Eq.
(9):

where K is the number of features, gk denotes the features, μk the weighted parameters for features, and Z is a normalization factor. This model offers a clean way to combine diverse pieces of linguistic information. As in the utterance model, we use lexical word features and POS tag features for the sequence labeling model.
The parameters of the hybrid model for intention and the sequence labeling model for additional information were trained on the labeled training corpus, for which we annotated the educational materials (Section 4.2) with speech act, main goal, additional information, and discourse features aforementioned. Table 3 shows the inventory of speech act, main goal, and additional information for the shopping domain.
Table 3 A portion of the inventory of semantic labels for the shopping domain

3.1.3 Dialog management
The dialog manager plays a key controlling role in any conversational spoken language interface: given the semantic input corresponding to the current user utterance and the current discourse context, it determines the next action of the system. In essence, the dialog manager is responsible for planning and maintaining the coherence of the conversation. To accomplish this goal successfully, the dialog manager must maintain a history of the discourse and use it to interpret the perceived semantic inputs and a representation of the system task is typically required.
The simplest dialog manager is a finite-state manager. This system completely controls the conversation with the user. It asks the user a series of questions, ignoring anything that is not a direct answer to the question and then going on to the next question. Systems that control the conversation in this way are called system-initiative systems. System-initiative dialog managers may be sufficient for simple tasks such as entering a credit card number, but pure system-initiative dialog managers are probably too restrictive for a relatively complicated task like shopping. The problem is that pure system-initiative systems require that the user answer exactly the question that the system asked. But this can make a dialog awkward and annoying. In addition, it is theoretically possible to create a finite-state system that has a separate state for each possible subset of questions that the user's statement could be answering, but this would require a vast explosion in the number of states. Therefore we avoid the pure system-initiative approach and use an architecture that allows mixed initiative, in which the conversational initiative can shift between system and user at various points in the dialog.
In this study, dialog management is performed by RavenClaw (Bohus & Rudnicky, Reference Bohus and Rudnicky2009), a plan-based, task-independent dialog management framework. RavenClaw isolates the domain-specific aspects of the dialog control logic from domain-independent conversational skills, and facilitates rapid development of mixed-initiative systems operating in complex, task-oriented domains. System developers can focus exclusively on describing the dialog task control logic, while a large number of domain-independent conversational skills such as error handling, timing and turn-taking are transparently supported and enforced by the RavenClaw dialog engine. Consider for instance error handling. System developers construct a dialog task specification under the assumption that inputs to the system will always be perfect, therefore ignoring the underlying uncertainties in the speech recognition channel. The responsibility for ensuring that the system maintains accurate information through confirmation actions (e.g., explicit/implicit confirmation) and that the dialog advances normally towards its goals is delegated to the dialog engine. Apart from the error handling strategies, the RavenClaw dialog management framework provides automatic support for a number of additional domain-independent conversational strategies. Examples include the ability to handle timeouts, requests for help, for repeating the last utterance, suspending and resuming the conversation, or starting again.
The dialog task specification describes a hierarchical plan for the interaction. More specifically, a dialog task specification consists of a tree of dialog agents, where each agent is responsible for handling a subpart of the interaction. For instance, Figure 4 depicts a portion of the dialog task specification for the shopping domain.

Fig. 4 A portion of the dialog task tree for the shopping domain; clean circles – dialog agency, filled circles – dialog agent, dotted circles – concepts.
The root node subsumes several ‘children’: SmallTalk, which engages the user in a daily conversation; ItemQuery, which obtains the fruit and vegetable properties from the user; GetItems, which executes the query against the backend; Payment, which presents the obtained results and handles the forthcoming negotiation for total price and performs payment. Moving one level deeper in the tree, the SmallTalk agent decomposes into Welcome, which provides a short welcome prompt and calls the user to come toward the robot; AskFeeling, which has a chat with users about their feelings, and finally AskLikeEnglish, which asks users whether they like English or not. The dialog agents in a dialog task specification fall into two categories: fundamental dialog agents, shown grayed in Figure 4, and dialog agencies, shown in clear in Figure 4. The fundamental dialog agents are located at the terminal positions in the tree (e.g., Welcome, AskFeeling) and implement atomic dialog actions, or dialog moves. There are four types of fundamental dialog agents: Inform – produces an output (e.g., Welcome); Request – requests information from the user (e.g., AskFeeling); Expect – expects information from the user, but without explicitly requesting it (e.g., Organic), and Execute – performs a domain-specific operation, such as database access (e.g., GetItems). The dialog agencies occupy non-terminal positions in the tree (e.g., SmallTalk, ItemQuery); their purpose is to control the execution of their subsumed agents, and encapsulate the higher level temporal and logical structure of the dialog task. Each dialog agent implements an Execute routine, which is invoked at runtime by the dialog engine. The execute routine is specific to the agent type. For example, inform agents generate an output when executed, while request agents generate a request but also collect the user's response. For dialog agencies, the Execute routine is in charge of planning the execution of their subagents. In addition to the Execute routine, each dialog agent can define preconditions, triggers, as well as success and failure criteria. These are taken into account by the dialog engine and parent dialog agencies while planning the execution of the various agents in the tree. The tree representation captures the nested structure of dialog and thus implicitly represents context (via the parent relationship), as well as a default chronological ordering of the actions (i.e., left-to-right traversal). However, this developer-specified plan does not completely prescribe a fixed order for the execution of the various dialog agents. When the dialog engine executes a given dialog task specification, a particular trace through this hierarchical plan is followed, based on the user inputs, the encoded domain constraints and task logic, as well as the various execution policies in the dialog engine.
If the dialog agents are the fundamental execution units in the RavenClaw dialog management framework, the data that the system manipulates throughout the conversation is encapsulated in concepts. Concepts can be associated with various agents in the dialog task tree, for instance feeling and preference in Figure 4, and can be accessed and manipulated by any agent in the tree. Several basic concept types are predefined in the RavenClaw dialog management framework: Boolean, string, integer and float. Additionally, the framework provides support for more complex, developer–defined concept types such as (nested) structures and arrays. Internally, the “value” for each concept is represented by a set of value/confidence pairs, for instance item_name = {apple/0.35; pineapple/0.27}. The dialog engine can therefore track multiple alternate hypotheses for each concept, and can capture the level of uncertainty in each hypothesis (Bohus & Rudnicky, Reference Bohus and Rudnicky2005; Bohus & Rudnicky, Reference Bohus and Rudnicky2006). Additionally, each concept also maintains the history of previous values, as well as information about the grounding state, when the concept was last updated, etc.
When it is desirable to offer corrective feedback, the robot provides fluent utterances which realize the learner's intention. Corrective feedback generation takes two steps: (1) Example Search: the dialog manager retrieves example expressions by querying the Example Expression Database (EED) using the learner's intention as the search key. (2) Example Selection: the dialog manager selects the best example which maximizes the similarity to the learner's utterance based on lexico-semantic pattern matching. If the example expression is not equal to the learner's utterance, the dialog manager suggests the example as recast feedback and conducts a clarification request to induce learners to modify their utterance. Sometimes, students have no idea about what to say and they cannot continue the dialog. In such a case, timeout occurs and the utterance model does not generate hypotheses. Hence, the dialog manager searches EED with only the intention from the dialog-context model and suggests the retrieved expression so that students can use it to continue a conversation (Lee et al., Reference Lee, Lee, Lee, Noh and Lee2010).
3.2 Emotional expression
The human perception of a robot's emotional expressions plays a crucial role in human robot interaction. Mero and Engkey were designed with expressive faces that can represent different emotions: pleasure, dislike, neutrality, hope, fear, joy, distress, surprise, embarrassment, pride, shame and sadness (see Figure 5). By virtue of its movable body, Engkey can also make diverse gestures by conducting a series of facial and body motions such as winking, yawning, cheering, sulking, etc, in accordance with the meaning of a verbal response:

Fig. 5 Facial expressions for various emotions.
3.3 Person identification
As previous research on interpersonal communication indicates, it is vital that two parties recognize each other for their relationship to develop (Kanda et al., Reference Kanda, Hirano, Eaton and Ishiguro2004). We can develop a unique relationship with individuals because we can identify each of them (Cowley & MacDorman, Reference Cowley and MacDorman1995; Hinde, Reference Hinde1987). Although person identification is an essential requirement for an educational robot, current visual and auditory sensing technologies cannot reliably support it. Lighting conditions may vary, and the shapes and colors of the objects in the environment may be too complex for current computer vision technologies to function. In addition, the method of person identification must be robust because misidentification can ruin a relationship. Here, the robots identify individuals using a RFID system. Recent RFID technologies enabled using contactless ID cards in practical situations. Consequently, the robots can show some human-like behavior in which the robot can call a child's name if that child is at a certain distance. This behavior is useful for encouraging the child to come and interact with the robot.
4 Experimental design
To find general cognitive and affective effects of RALL approaches which can motivate subsequent in-depth research, we designed and performed a field study at a Korean elementary school. The following subsections describe the method of the study in more detail.
4.1 Setting and participants
A total of 24 elementary students (12 male and 12 female) were enrolled in English lessons two days a week for a total of about two hours per day and had chant and dance time on Wednesdays for eight weeks during the winter vacation. However, three students left the study, resulting in a total of 21 students. Because the program was administered during the vacation, there was no other English class. The students ranged from third to fifth grade (nine students for third grade, seven for fourth, and eight for fifth); in general, there are six grades in a Korean elementary school and students start learning English from third grade. All of them were South Korean, spoke Korean as their first language and were learners of English as a foreign language. The participants were recruited by the teachers at the school from volunteers, through interviews, according to motivation and English proficiency. Then they were divided into beginner-level and intermediate-level groups, according to the pre-test scores. The evaluation rubric in Table 6 shows that the pre-test scores reflect the students’ initial proficiency: students’ pronunciation was understandable with some confirmation and misunderstanding; students’ responses showed heavy reliance on beginner-level expressions with some communication breakdowns; students’ responses contained grammar errors that are sometimes distracting to listeners and cause confusion about meaning; students replied with relatively short answers, requiring encouragement. The design of the field study, however, makes the precise role of RALL approaches in facilitating L2 development less than clear. This is due to the lack of a control group which was necessitated by financial and scheduling constraints. Figure 6 shows the layout of the classroom: (1) PC room where students took lessons by watching digital content; (2) Pronunciation training room where the Mero robot performed automatic scoring of pronunciation quality for students’ speech and provided feedback; (3) Fruit and vegetable store, and (4) Stationery store where the Engkey robots acted as sales clerks and the students as customers.

Fig. 6 Students interacting with Mero and Engkey.
4.2 Material and treatment
The researcher produced training materials including a total of 68 lessons, with 17 lessons for each combination of the level (beginner and intermediate) and the theme (fruit and vegetable store and stationery store). Among other things, the course involves small talk, homework checking, purchases, exchanges and refunds. When dealing with task assignment, the instructors proceeded in subtle gradations, moving from the simple to the complex. Throughout the course of the study, each student was asked to enter the four rooms in the order of PC room, Pronunciation training room, Fruit and vegetable store, and Stationery store so that students were gradually exposed to more active oral linguistic activities. Students were expected to spend about ten minutes in each training room. Although there were assistants, their roles were confined to fixing any technical problems with the robots. There was no English instruction in addition to the interaction with the robots during the period of this study.
4.3 Data collection and analysis
4.3.1 Cognitive effects
In order to measure the cognitive effects of the RALL approach, i.e., improvement of listening and speaking skills, all students took a pre-test at the beginning of the study and a post-test at the end. For the listening skill test, 15 multiple-choice questions were used, which were developed by experts in evaluation of educational programs (see Figure 7). The items in the test were mainly selected from the content taught during the course, as shown in Table 4.

Fig. 7 A multiple-choice question for the listening skill test.
Table 4 Assessment items for listening tests

The test was used as the assessment tool in both the pre-test and the post-test phases of the study. The internal consistency estimates of reliability, Cronbach's alpha (Cronbach, Reference Cronbach1951), were computed for the listening test. The value of Cronbach's alpha for the pre-test was .87 and the value for the post-test was .66, each indicating satisfactory reliability. The speaking skill test consisted of 10 one-on-one interview items. All speaking assessment tasks were carried out by a teacher from the participating school with an advanced degree in Education. The topics of the interviews were selected from the content taught (see Table 5).
Table 5 Assessment items for speaking tests
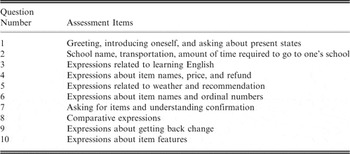
The evaluation rubric measured speaking proficiency on a five-point scale in four categories: pronunciation, vocabulary, grammar, and communicative ability, as shown in Table 6.
Table 6 Evaluation rubric for speaking tests

The value of Cronbach's alpha for the pre-test was .93 and the value for the post-test was .99, each indicating satisfactory reliability. A paired t-test was performed using the mean scores and standard deviations to determine if any significant differences occurred.
4.3.2 Affective effects
In order to investigate the effects of RALL on affective factors such as satisfaction in using robots, interest in learning English, confidence with English, and motivation for learning English, a questionnaire was designed by ten teachers and experts in the evaluation of educational programs. It consisted of some personal information and 52 statements in accordance with a four-point Likert scale, which had a sliding answer scale of 1–4, ranging from “strongly disagree” to “strongly agree”, without a neutral option. Mean and standard deviation were used to evaluate the effect on students’ satisfaction, whereas a pre-test/post-test method was used for other factors. The internal consistency estimates of reliability, Cronbach's alpha, was computed to indicate satisfactory reliability (see Table 7).
Table 7 Internal consistency estimates of reliability

Na = Number of questions,
Rb = Cronbach's alpha in the form of pre-test (post-test).
5 Results and discussion
5.1 Cognitive effects
The achievement of the students in the beginner group on pre- and post-test is presented in Table 8. According to the findings in this table there were large improvements in the participants’ speaking skills achievement in the post-test. The score in the post-test is significantly better than that of the pre-test. The effect sizes, which were calculated following the formula proposed in Rosnow and Rosenthal (Reference Rosnow and Rosenthal2007), range over 0.82–0.90, showing large effects. We conducted the Bonferroni test (Kutner, Nachtsheim, Neter & Li, Reference Kutner, Nachtsheim, Neter and Li2004) for a simultaneous inference to test whether or not the four categories under speaking skills have significant differences simultaneously. The result showed that there was a significant difference in a simultaneous inference at the significance level of 0.01. The listening skill, however, showed no significant difference.
Table 8 Cognitive effects on oral skills for the beginner group

*p < .01, SDa = Standard Deviation.
Significant differences in speaking skills were also found in the result of the intermediate group and the effect sizes are also large, whereas the listening skill showed a significantly negative effect (see Table 9). In addition, the result of the Bonferroni test showed that the four categories under speaking skills have a significant difference in a simultaneous inference at the significance level of 0.01.
Table 9 Cognitive effects on oral skills for the intermediate group

*p < .01, SDa = Standard Deviation.
The combined results of both groups showed no significant differences in listening skills (see Table 10). This finding can be explained by a number of factors such as the unsatisfactory quality of the text-to-speech component and the robots’ various sound effects (e.g., alarms, musical instruments) which can distract learners’ attention from the robots’ speech. However, significant differences in speaking skills were found in the overall results and the result of the Bonferroni test showed that the four categories under speaking skills have a significant difference in a simultaneous inference at the significance level of 0.01. The large improvement in speaking skills in the overall results agrees with the findings of previous studies in general. Specifically, based on the evaluation rubric, the gain in the vocabulary area reveals that before the treatments students were limited to heavy reliance on very simple expressions with some communication breakdowns, but after the treatments their responses became informationally appropriate with only one or more imprecise words. This may indicate that the authentic context facilitated form-meaning mapping and the vocabulary acquisition process. The improved accuracy of pronunciation shows that the treatments made students’ pronunciation more intelligible. Before the treatments, their pronunciation was understandable only with some confirmation and misunderstanding. The improvement in the grammar area shows that after the treatments there were at most minor lapses that did not cause confusion about meaning, compared to serious errors before the treatment that sometimes distracted listeners and caused confusion about meaning. This may support the output hypothesis and the effects of corrective feedback. The fact that learners had feedback at any related point made them reflect on their erroneous utterances. The increase in communicative ability means that before the treatments students required encouragement even for replying with short answers, yet they could sustain coherent discourse by themselves after the treatments. This may show that learners were getting accustomed to speaking English. It can also be attributed to the fact that when using robot-assisted learning the student gained confidence in a relaxed atmosphere. A lack of confidence and a feeling of discomfort were more related to students’ participation in face-to-face traditional discussions, and less to participation in computer-based learning. Although the absence of a control group makes the result less than clear, given the results of a previous study (Petersen, Reference Petersen2010) showing decreased scores in control groups in which learners do not participate in any treatment sessions and the positive affective effects (Section 5.2) related to oral skills, the likely interpretation is that the treatments contributed to the improvement in oral skills.
Table 10 Cognitive effects on oral skills for overall students

*p < .01, SDa = Standard Deviation.
5.2 Affective effects
As shown in Table 11, the students were highly satisfied about using robots for language learning. It is worth noting that a large portion of the satisfaction was associated with students’ recognition of robots as intellectual beings capable of human-like social interactions such as watching, listening and moving toward students. This result supports the benefit of the robots’ capacity to create interpersonal relationships with the students (Cowley & MacDorman, Reference Cowley and MacDorman1995; Hinde, Reference Hinde1987). In comparison to the other questions, the questions about the robot's outer appearance (e.g., “The robot's body looks comfortable for moving around in a classroom” and “The robot's facial expression looks comfortable to you) and voice (e.g., “You like the robot's voice”) showed the lowest level of satisfaction, showing the need to develop a more anthropomorphic appearance and a natural voice. The low level of satisfaction regarding the robot's voice can explain, in part, the lack of improvement in students’ listening skills. The robot's speech synthesizer has only addressed comprehensibility whereas CALL places demands on naturalness, accuracy, and expressiveness as well. In order to fully meet the requirements of CALL, further attention needs to be paid to accuracy and naturalness, in particular at the prosodic level, and to expressiveness (Handley, Reference Handley2009).
Table 11 Students’ satisfaction in using robots
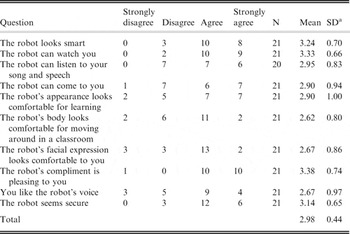
SDa = Standard Deviation.
The students’ responses to the questions about their interest in learning English on pre- and post-test are presented in Table 12, showing a large improvement of interest with a significance level of 0.01. The response to the question “Singing a song, chanting, and other games are interesting” shows that the robot's physical body, one of its unique features, had a great influence on students’ interest by enabling the robots to dance, make gestures and use facial expressions. In addition, the large improvement shown by the response to the question “You think English is easier and more familiar than before” may support the affective filter hypothesis (Krashen, Reference Krashen2003) which states that the blockage can be reduced by sparking interest and providing low anxiety environments. The question “You want to use the expressions learned” also showed a large improvement, which may be attributable to the immediate application of the learned expressions through conversations with robots. The only question that had a score of less than three is “You want to talk with other people in English”. This result agrees with the findings of previous studies that a feeling of discomfort was more related to students’ participation in face-to-face traditional conversations and less to participation in robot-assisted learning.
Table 12 Students’ interest in learning English
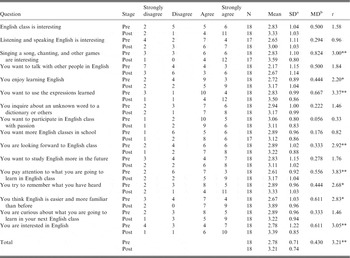
*p < .05, **p < .01, SDa = Standard Deviation, MDb = Mean Difference.
A significantly large increase in confidence was found in the responses to the questions about confidence in English on the pre- and post-test with a significance level of 0.01 (see Table 13). This can also be attributed to the fact that robot-assisted learning allows the students to achieve academically and gain confidence through repeated exercises in a relaxed atmosphere. However, relatively low scores were given to the questions relating to individual levels of fear or anxiety associated with either real or anticipated communication with another person or persons (e.g., “You are not afraid of speaking English,” “You are not afraid of being questioned by the English teacher,” and “You feel no shame about your English mistakes”). Therefore robots should help students to feel that they can learn the foreign language well by using more encouragement and praise. Classroom atmosphere is very important; it should be happy, lively, friendly and harmonious to help students overcome their psychological barriers, and lower their anxiety. Robots should also tolerate a few small mistakes made by students provided those mistakes do not affect the communication process, because this can release pressure and strengthen their confidence. Given the large improvement shown by the response to the question “You can greet foreigners with confidence”, we can infer that the confidence gained through the repeated greetings with robots was transferred, to some extent, to greetings with foreigners. We can also find some grounds for assuming an improvement in the students’ cognitive abilities from the large gain in respect of the question “You can answer the questions about what you have learned with confidence”. Finally, the great improvement regarding the question “You think that you can speak English better if you study harder” is very impressive, given that many Korean people regard English as a very difficult language to learn, however hard they study.
Table 13 Students’ confidence with English

*p < .05, **p < .01, SDa = Standard Deviation, MDb = Mean Difference.
The responses to the questions about motivation for learning English are presented in Table 14. There has been a large enhancement of motivation, with a significance level of 0.01. Interestingly, there was a relatively large increase in the score for the question “You are aware of the necessity of studying English” compared to the small difference for the question “You recognize the importance of English to your present and future life.” It may mean that the experience of having a conversation with a robot enabled the learners to focus on their practical needs in English, thus overcoming some of their communicative difficulties. The low scores for the questions related to preparing to study English (e.g., “You want to buy English books and materials,” “You make plans to study English,” and “You enjoy preparing for English classes”) may illustrate that traditional education does not work for the new generation of children. The popularity of e-Learning in Korea is promoting an increasing disengagement of the “Net Generation” or “Digital Natives” from traditional instruction.
Table 14 Students’ motivation for learning English

*p < .05, **p < .01, SDa = Standard Deviation, MDb = Mean Difference.
6 Conclusion
In this study, we described the rationale of RALL from a theoretical view of language learning, briefly summarized earlier CALL approaches in comparison with RALL approaches, and introduced the HRI technologies we used to implement the educational assistant robots. To investigate the cognitive and affective effects of robot-assisted learning, a course was designed in which intelligent robots act as sales clerks in a fruit and vegetable store, and in a stationery store so that they can interact in real life situations with language learners who play the part of customers. A pre-test/post-test design was used to investigate the cognitive effects of the RALL approach on the students’ oral skills. The results showed no significant difference in the listening skill, but the speaking skills improved with a large effect size at the significance level of 0.01. This may mean that RALL approaches can provide valuable leads in helping students to enhance their speaking ability, particularly in the case of Korean students where teaching is generally focused predominantly on vocabulary and grammar. Descriptive statistics and pre-test/post-test design were used to investigate the affective effects of the RALL approach. The results showed that RALL promotes and improves students’ satisfaction, interest, confidence, and motivation at the significance level of 0.01. In addition, the result of the Bonferroni test showed that the three categories under affective factors (i.e., interest, confidence, motivation) have a significant difference in a simultaneous inference at the significance level of 0.01. Throughout all affective areas, unique features of robots made a great influence on the students’ responses showing that RALL can be an enjoyable and fruitful activity for students. Although the results of this study bring us a step closer to understanding RALL approaches, subsequent in-depth research should be conducted to ascertain the detailed effects of each possible factor involved in RALL methods. Also, the results are only valid for Korean elementary students. More studies are needed to consolidate/refute the findings of this study over longer periods of time using different activities with samples of learners of different ages, nationalities, and linguistic abilities. Given that studies on RALL are still relatively new and most are in the early stages, further research is needed into the use of robots for educational purposes and the effects of their use in this field.
Acknowledgement
We would like to thank Dr. Jaechun Ban for his expertise and efforts on evaluation of educational programs. This research was performed for the Intelligent Robotics Development Program, one of the 21st Century Frontier R&D Programs funded by the Ministry of Knowledge Economy (MKE).