Introduction
There are over 2.2 billion children worldwide. With the improvement of economic level and parents’ education concept, the proportion of child-related expenditure in the family budget is proliferating. According to the United States Department of Agriculture (Lino et al., Reference Lino, Kuczynski, Rodriguez and Schap2017), a middle-income family spends around $12,980 annually per child, and child care/education takes the third position in all their housing accounts. According to the data disclosed by the National Bureau of Statistics of China, at the end of 2016, China's population aged 0–14 has exceeded 230 million, and this data will exceed 300 million in 2020. At the same time of population growth, with the improvement of economic level and parents’ education, the proportion of children-related products in the family expenditure is also growing. Within 80% of China's families, consumption for children accounts for 30–50% of the total household expenditure.
Early childhood education has long-lasting influences in terms of children's school competences, attitudes, developed abilities, and even the family (Lazar et al., Reference Lazar, Darlington, Murray, Royce, Snipper and Ramey1982). However, it is difficult for parents to accompany their children and educate them all the time, while smart toys can be an alternative way to stimulate their development and entertain them (Gutiérrez García et al., Reference Gutiérrez García, Martín Ruiz, Rivera, Vadillo and Valero Duboy2017). An excellent smart toy can help in children's brain development and enhance their physical and mental health.
Since the smart toy is a newly emerging concept, there is no clear definition in the toy industry and academia. This paper generally defines the smart toy by referring to the definition of a smart system. A smart system is a computer system that can produce intelligent human behavior, which can acquire and apply knowledge automatically, the ability of thinking and reasoning, the ability of problem-solving, and the ability of automatic learning. A smart toy is defined as the integration of traditional toys and intelligent technology such as speech synthesis, artificial reality, and machine vision to interact with children. For example, through the built-in electronic chips, sensors, actuators, and other components, smart toys can perceive external information and give intelligent feedback to users’ operation. It is a new type of toy with digital characteristics, which can carry out functions such as state perception, real-time analysis, independent decision-making, precise execution, and learning promotion.
A large number of children's intelligent products have sprung up. The scale of children's electronic goods is considerable. For example, the market for children's smartwatches was 20 billion in 2017. Children's intelligent products have an average annual growth rate of 75%, far exceeding the average yearly growth rate of 6% in the toy industry. Chinese parents spend more than 50 billion RMB on toys for early childhood education every year. What is more, the application of artificial intelligence (AI) technology such as speech recognition, speech synthesis, and human–computer interaction will significantly increase the price and gross margin of children's intelligent products.
Existing smart toys include Anki CogniToys, Google's alpha dog intelligent robot, Sony's Aibo robot dog, and Olpay Cady robot (Fig. 1). Despite the promising market of intelligent products for children, serious problems exist. With the increasing popularity of the market, a large number of low-quality children's story machines have appeared in the market without after-sales investment. According to the authors’ interviews with practitioners including toy manufacturing companies in Shanghai and Zhejiang Province in 2020, the entry barriers of children's intelligent hardware has been reduced to a superficial level with the free opening of the primary AI open sources, especially in cities where the supply chain is quite mature. Many small-sized companies only invest 1 month as the product development period and adopt the open-source, public models for the appearance. They only change the wake-up words for the software before it is put into the market. They are equipped with low-quality batteries, which is easy to explode. What is worse, the low-end toys are made by waste materials, so that the hardness of the shell is not guaranteed. Those toys are captured by messy button design, low interaction, built-in immutable stories, and poor user experience. This fast track product has spawned a bubble market. The most intuitive phenomenon is that the homogeneity of smart toys is very serious. The interaction performance is far from satisfactory. The robot is talking to itself and can barely communicate with users. Last but not least, the design of current companion toys has not incredibly involved experts in children's education, so the product cannot precisely meet children's needs.
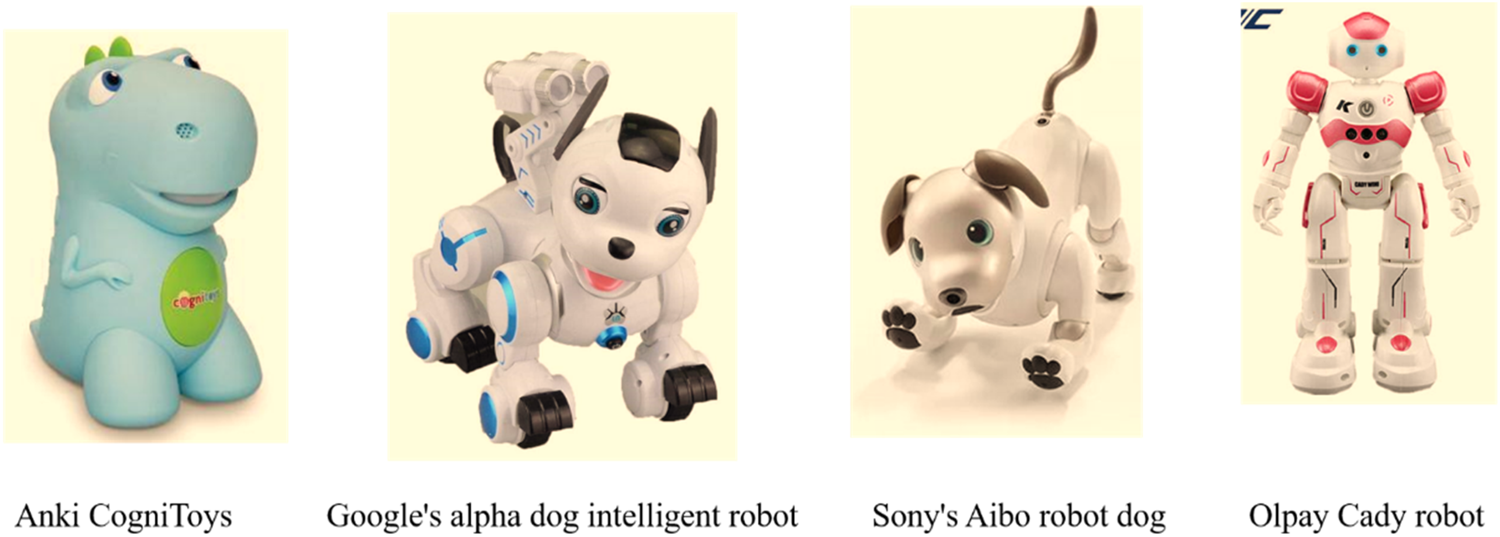
Fig. 1. Examples of smart toys.
On the other hand, current companion toys are mostly held by human designers, and the design process can be quite tedious. After the toy is put into use, it is hard to realize continuous updates to cater to the children's specific needs or catch up with the new trends.
This paper holds the idea that machines and people have their advantages. Machines can accumulate knowledge, calculate quickly, and solve closed-form problems. People have the ability to imagine, create, and solve open problems. In terms of design, it is necessary to collect materials and process information, which accounts for nearly 30% of the designer's workload. When machines can undertake more work, human designers can focus more on management, creative creation, communication, and other capabilities to maintain competitiveness. Therefore, people can ask a machine to assist in integrating their advantages in designing products.
This paper aims to establish a framework, which guides to smartly design the intelligent companion toys for children, which is child-centered and environment-oriented. Smart design refers to the application of modern information technology, so that computers can simulate human thinking activities and improve the level of intelligence. In this way, AI can better undertake various complex tasks in the design process and become an essential auxiliary tool for designers. The design process is completed through the collaboration of human and AI. The state-of-the-art technologies such as speech recognition, speech synthesis, human–computer interaction, big data, and deep learning will be applied to the toy, while various stakeholders will be involved in the design.
AI enhances human ability rather than replace humans. The entire smart design process of the intelligent companion toys gets multiple stakeholders involved and needs the support of various advanced technologies. It is divided into three main parts (Fig. 2), which is requirement confirmation, collaborative design, and iterative update after the sale during the utilization process.

Fig. 2. The overall smart design process of intelligent companion toys.
The remaining paper is organized as follows. Section “Related work” reviews related work on the design of general products and children's products. Section “Requirement confirmation” covers requirement confirmation. Section “The smart design process before sale” introduces collaborative design before the sale, and Section “The iterative update process after sale” presents the iterative update after the sale. Section “Case study” offers a brief case study, while Section “Conclusion” concludes.
Related work
Two streams of the literature are mainly reviewed, where the first stream reviews paper on general product design, and the second one focuses on the design of children's products.
Design of general products
Quite a few papers are discussing the smart design of products with advanced technologies, and customers’ personalized needs are much more valued.
Abramovici et al. (Reference Abramovici, Göbel and Dang2016) propose an approach of managing data for interdisciplinary development and configuration of smart products. Their framework is flexible to handle the feedback of the product along the whole lifecycle using digital product twin. Lin et al. (Reference Lin, Yu, Zheng, Qiu, Wang and Xu2017) conduct a group of product configuration activities in multiple types of product configurators. They prove that the virtual reality-based approach helps understand customers’ mental and physical reaction to realize their personalized needs. Mourtzis et al. (Reference Mourtzis, Doukas and Vandera2017) establish some APPs to facilitate the integration of customers in product design and manufacturing network design. Their case study demonstrates that the APP (applications) can help tailor to the personalized needs of users. Abramovici et al. (Reference Abramovici, Göbel, Savarino, Gebus, Ríos, Bernard, Bouras and Foufou2017) propose an approach for the design and configuration of smart products. Their framework utilizes virtual product twin to consider dynamic changes of product instances. Tao et al. (Reference Tao, Cheng, Qi, Zhang, Zhang and Sui2018) state that the digital twin can help use the cyber-physical data to drive product design and manufacturing processes. Poeppelbuss and Durst (Reference Poeppelbuss and Durst2019) analyzes the role of canvas to stimulate the discussion during product design and emphasizes the importance of smart service. Bilal Ahmed et al. (Reference Bilal Ahmed, Imran Shafiq, Sanin and Szczerbicki2019) suggest a smart virtual product development system that facilitate decision making and enhances activities in product development.
The smart design of products has a much higher requirement on the knowledge among different stakeholders. Blanco et al. (Reference Blanco, Casas, Manchado-Pérez, Asensio and López-Pérez2017) clarify that knowledge sharing and efficient communication are vital in designing smart electronic products. They develop some constructivist tools to tackle the problems in technological literacy among designers and facilitate the knowledge sharing dialogue. Irani et al. (Reference Irani, Sharif, Papadopoulos and Love2017) introduce the role of social media in knowledge sharing for supporting decision making of both internal and external stakeholders.
These papers contribute to proposing novel methods of the smart design of products, and they also pay attention to the entire lifecycle. Different from previous work papers, this paper zooms in to focus on the smart design of children's companion toys, so children's unique characteristics are considered. What is more, both the design process and the children's product are both captured by “smart” here.
Design of children's products
The intelligent toy or robot can play an important role in accompanying or educating children, which has been verified by the previous literature. Kanda et al. (Reference Kanda, Hirano, Eaton and Ishiguro2011) probe into the idea that robots may form relationships with children and children can learn from robots. The results reveal that robots establish influence more successfully when children have initial proficiency in English. Janssen et al. (Reference Janssen, Van Der Wal, Neerincx and Looije2011) discover the role of adaptive robot game in motivating children to learn arithmetic. Heerink et al. (Reference Heerink, Diaz, Albo-Canals, Angulo, Barco, Casacuberta and Garriga2012) perform a study on the interaction between children and pet robots. They identify prevalent social behaviors, including petting robots, and find that children only treat robots as artifacts less than one percent of the study time. Short et al. (Reference Short, Swift-Spong, Greczek, Ramachandran, Litoiu, Grigore and Scassellati2014) observe the interaction between a robot and 26 children in learning about food choices. Results suggest that children show a significant level of enjoyment in interacting with that robot. Evidence is found to prove the relationship built between children and robots. Chandra et al. (Reference Chandra, Alves-Oliveira, Lemaignan, Sequeira, Paiva and Dillenbourg2016) explored the children's learning behavior given a robotic facilitator is present, and they discover that children tend to disclose themselves to the robot. Reich-Stiebert and Eyssel (Reference Reich-Stiebert and Eyssel2015) search about people's attitudes to education robots. They find that people in the areas of science, engineering, technology, and mathematics hold a positive attitude. García et al. (Reference García, Ruiz, Rivera, Vadillo and Duboy2017) design an experiment where the smart toy help 65 toddlers make the tower with five cubes. Druga et al. (Reference Druga, Williams, Park and Breazeal2018) ask 30 children aging between 4 and 10 to solve the maze by navigating a smart robot, through which their social-emotional attributes can be observed and developed. Fachada (Reference Fachada2018) organizes a play among a robot dog, 6-year-old preschool children, and 80+-year-old seniors. Their study verifies the smart toy robot can facilitate the social engagement between two generations and enhances the conversation skills of children.
There are some papers making efforts on the design of intelligent toys or robots for children. Zaraki et al. (Reference Zaraki, Mazzei, Giuliani and De Rossi2014) introduces a gaze-control system within a humanoid robot that is context-dependent and equipped with social functions. Tozadore et al. (Reference Tozadore, Pinto, Romero and Trovato2017) carries out a test within the school environment to examine the educational interaction between children and the robotic system. Three communication ways are involved, including speech, vision, and gesture. Banse and Scherer (Reference Banse and Scherer2014) conduct an experiment with multiple 9-year-old children and two robots to explore the impacts of robots’ adaptive emotional expression on children's behavior and opinions. They discover that children react relatively positively and expressively when the robot can adaptively express itself. Cameron et al. (Reference Cameron, Fernando, Collins, Millings, Moore, Sharkey and Prescott2015) investigate the influence of robot facial expressions on children's behavior and attitude. They point out that boys show a positive response and greater liking to the robot when robots have richer facial expressions. Henkemans et al. (Reference Henkemans, Bierman, Janssen, Looije, Neerincx, van Dooren and Huisman2017) develop a coding scheme designed for personal robots that helps manage and educate children with diabetes. Wood et al. (Reference Wood, Zaraki, Walters, Novanda, Robins and Dautenhahn2017b) review the design of a humanoid robot called Kaspar, which is developed iteratively based on the needs of children with autism. Wood et al. (Reference Wood, Dautenhahn, Robins and Zaraki2017a) outline the standard assessments to measure autism children's progress when interacting with a robot. Zheng et al. (Reference Zheng, She, Liu, Chen, Shu and Xiahou2019) introduces the design of a companion robot that has real-time multimodal computing. Axelsson et al. (Reference Axelsson, Racca, Weir and Kyrki2019) present the design process of the robotic tutor that helps to teach sign language to children with autism spectrum disorder problems.
These papers contribute by proposing some ideas on children's intelligent robots. However, little research has established a whole framework guiding the entire design process of the intelligent companion robots for children, including the iterative update after it is put into use.
Especially, previous research barely discussed the role of AI in the design process of smart toys. Currently, there are some papers that have introduced AI into the design process of other products. For example, Quan et al. (Reference Quan, Park, Economou and Lee2019) adopted AI-aided design to reinforce the decision-making process of urban design and their smart design framework integrated advanced AI search techniques and performance simulation methods. Bouchard et al. (Reference Bouchard, Bouchard and Bouzouane2012) applied AI in the design of hardware and software of smart home architecture. Zhang and Kantarci (Reference Zhang and Kantarci2019) used AI to facilitate the design of security and privacy in mobile crowdsensing systems
In contrast, this paper highlights the role of AI in the entire design process of smart toys, from demand collection to the iterative update after it is put into use. What is more, this paper takes the characteristics of children in each step of the design.
Requirement confirmation
This section will introduce the requirement confirmation process and the key requirements on intelligent companion toys.
Requirement confirmation process
Requirement confirmation (Fig. 3) is basically completed by the product manager via several major steps: demand collection, authenticity, and prioritizing.

Fig. 3. Requirement confirmation process.
Demand collection
The objective of collecting demands is to maintain the core competitiveness of the intelligent child companion toy. By carrying out the market research, product managers can grasp a deeper understanding of users’ needs, and the development trend of the toy industry. During the study, product managers should refer to the company's vision as the guidelines and target the appropriate user groups.
It is undoubtedly very delicate and challenging to design and develop products for children. This is an inevitable phenomenon caused by the inconsistency between product decision-making people and users, and the fact that most designers cannot have the same level of empathy. Therefore, concerning the user-centered idea, they are advised to consult the suggestions of children, parents, babysitters, and kindergarten teachers. Multiple collection methods are available, including brainstorming, interviews, questionnaire, benchmarking analysis, and user behavior analysis. Different collection methods will affect the authenticity of the demand. For example, major users of companion toys are children that might not be capable of fulfilling detailed questionnaires. In this case, product managers have to find a better way to interact with children, such as observing their preferences during toys picking to learn about their tastes and supplement their ideas with parents’ questionnaires. The predetermined expectation should be avoided since children's interests can be quite different from those of adults.
Especially, in the coming era of 5G, companies can apply big data technologies to pull mass information from various texts, images, and social networks online to learn about people's tastes. Information collection can bring mental stress to designers and influence their design quality or performances (Zhao and Zeng, Reference Zhao and Zeng2019), so it can be quite helpful to make use of smart technologies to release their pressure. Rich user experience data can be isolated from online customers’ reviews on competing products to establish a knowledge base accordingly (Yang et al., Reference Yang, Liu, Liang and Tang2019). Appropriate methods are needed to handle mass data, such as Kalman filter methods and Bayesian methods (Jin et al., Reference Jin, Liu, Ji and Liu2016). By integrating the data and formalizing the network, designers are enabled to uncover vital factors, including customers’ preference and their usage contexts.
Demand authenticity
Product managers are required to classify the demands collected into real different categories. Some requests are real, strong, and rigid. Mainstream users are willing to pay money and time for this demand. What is more, they tend to share this product with friends, given that this requirement is satisfied. However, there are some false, weak, or soft demands. Users may come up with some demands due to their lack of “imagination” in solution. It is likely that they do not know accurately what they want. Alternatively, they may raise ideas that are not feasible.
Demand prioritizing
Managers are expected to prioritize all demands referring to multiple grading methods and distinguish functions and affordances since not all demands should be satisfied. The first is the user satisfaction model. Users’ basic demands have to be met, while the expectation demand or exciting demand can be optional. For instance, some functional iteration updates can be dealt with later, considering their minimal impact on the market shares. The second is the return on investment ratio. The third is technology risk. An overlong R&D period leads to increased risk since the market can be occupied by other toy companies. Requirements requiring fewer server resources should take precedence. Those trade-offs and the confirmation of functions can be accomplished using some modeling approaches (Summers et al., Reference Summers, Eckert and Goel2017). To better analyze the requirements, an appropriate requirement culture is demanded (Elena et al., Reference Elena, Wentzky and Summers2019). Employees from different departments and levels should have different amounts of engagement with the requirements and form an excellent collaboration relationship. Some decision tools, such as the Kesselring method, can be used to support the conceptual design in early stages and make it more transparent for people involved (Zeiler et al., Reference Zeiler, Savanovic and Quanjel2008).
Key requirements on companion toys
There are some critical requirements for intelligent companion toys for children.
Safety
The top concern of parents on intelligent companion toys is safety. Children, especially those preschool ones, have not acquired abilities to protect themselves. They have no sense of danger and cannot restrain their behavior. Active children may likely disassemble toys and destroy components. On the other hand, the development of body organs is not mature enough. Hence, their physical and mental health is vulnerable to contact with low-quality materials or improper operation. Especially in children's amusement area with more than one child, such as kindergartens and parks, it is difficult for parents or teachers to take care of the safety of each child. Therefore, special attention should be paid to safety in the design of intelligent companion toys. Toxic or radiative materials that are harmful to children or the environment are forbidden. There are many industry regulations to refer to. The configuration should be round and smooth to protect children from cutting by sharp shapes. All parts are connected tightly so that no small pieces will accidentally fall off, which might be swallowed by preschool children.
Entertaining aspect
Children are not good at self-control, and their persistence is relatively weak so that they are easily distracted. On the other hand, they are often energetic, active, and curious. The intelligent toys need to take children's cognition and emotion as the core consideration to provide a more natural and exciting interactive experience. In this case, they can arouse children's interest and meet the needs of a company, education, and entertainment. For example, children can play with toys in full accordance with their personality, without the need of following stylized interactive ways. Intelligent toys with more fun will be more attractive to children. Thus, a toy that gives a strong sense of participation to children can further promote the development of their memory, language abilities, observation, and reaction abilities. Such toys with a high added value can extend its service life.
Age matching
A narrow age gap can mean a lot of differences for children. Therefore, the design of companion toys should consider the ages of potential users. Here are some examples. The children of 2–3 years old are gradually learning various kinds of actions, so their toys should be stable and appropriate for the repeated operation. The size of icons and the spacing between them should be large enough to reduce the probability of incorrect operation. Children of this age can draw vertical lines but no horizontal lines. Therefore, the operation gesture of dragging up and down should be adopted. What is more, their pronunciation is fuzzy, and vocabulary is limited, so the voice instructions of intelligent toys should use simple voice instructions as much as possible, and the voice fault tolerance rate should be high.
When children are 4–5 years old, all of their short-term memory, language abilities, and body balance are further developed. Therefore, more complicated voice instructions and gesture operations can be adopted. Also, children of this age are imaginative and tend to imitate often. In this case, companion toys can be a tool to cultivate their good habits through situational education, such as role-playing.
When children are older, companion toys can play a more critical role in education in addition to amusement. They can be designed for enriching their knowledge, broadening their horizons, and improving their social skills.
Coordinated development of multiple senses
The cognitive development of preschool children is reflected in some major aspects, such as vision, hearing, touch, depth perception, shape perception, orientation perception, and time perception. Product managers are required to consider them all when passing requirements on toys to designers. The following are two examples.
(1) Vision. Children's color vision is developed rapidly in a period of 3–6 years old. Around the age of 3, children can distinguish primary colors, but they cannot tell subtle differences in hue, lightness, and saturation of various colors. This ability is not improved until the age of 5. Therefore, the brightness of the toys’ screens should be appropriate to avoid hurting children's eyes. The speed of image transformation needs to be set reasonably so that the development of children's vision and depth perception are not negatively affected. What is more, pictures should be more extensive, and the contrast should be more substantial, which is conducive to the improvement of children's visual acuity.
(2) Hearing. Children's hearing ability is developing fast, including the recognition of sounds and the understanding of the speech content. To protect their hearing, toys are prevented from sending noisy music, and decibels are under careful control. Companion toys can offer multiple choices of different languages and dialects to promote their understanding. What is more, their auditory perception can be heightened by listening to various kinds of music, which they may dance with.
The smart design process before sale
The design process before the sale can be divided into input, analysis, function, output, user interface, evaluation, and testing, all of which are collaboratively completed by humans and AI (Fig. 4). Because children have their unique characteristics, co-creation is vital to guarantee final companion toys can satisfy children and other stakeholders. Children psychologists, educators, parents, and teachers should be invited to offer opinions to avoid bias due to lack of expertise. They can provide more useful content for the system and suggest a more reasonable way of interaction with children. The whole design cycle is not necessarily long since committing a longer period does not strictly associate with more successful designs (Yang, Reference Yang2005), and it is vital to catch up with the latest trend.

Fig. 4. The smart design process before sale.
At the technical level, technology must be deeply integrated with content and product. Instead of the single “storytelling” mode of current so-called robots, the intelligent companion toy should provide a closed-loop experience for smart education in the form of two-way and multi-round interaction. Bionics experts, programmers, and engineers of different backgrounds should work collaboratively to realize such requirements. Meanwhile, the overall design process can take advantage of modern technologies such as graphics-based modeling software, simulation software, and optimization software (Horváth, Reference Horváth2004).
Input
Various ways to rouse companion toys from the standby mode should be available, such as voice, a physical button, touch, and infrared sensing. The most commonly used “voice input” is introduced here as an example.
Companion toys can be roused by voice instruction, such as “Hello + name.” It can further support the sound source following function. No matter which direction users are standing to wake up the toy, it takes the initiative to turn its head around and always focus on the user. Such an ingenious function is likely to make children proud and interested since the toy acts as a little attendant. It can be roused at a distance of about 3 m, even in the case of noisy or background music. This can be realized with improved algorithms, which makes the system occupy fewer resources.
At the same time, the intelligent toy supports multiple rounds of dialog, so there is no need to wake it up repeatedly in the whole dialog process, leading to a smoother dialog process. This depends on the excellent design of the decision tree. Information needs to be presented according to context, and each conversation will determine the information of the next discussion. The information structure of products is mainly a decision tree, which requires the change of designer's thinking. Designers need to turn from the process design of the interface to the strategy design based on the decision tree. They are expected to establish a complete decision tree to meet the needs of children and find the optimal path and think more about the presentation logic of information in the context.
The smart toy should be equipped with the function of voiceprint recognition and face recognition. It can not only respond to users’ questions in time but also accurately identify whether children are talking, or parents are talking, to provide more accurate answers. A smart toy should show high tolerance to “false wakeup.” It is expected to stay the standby mode when people are not talking to it, but some similar rousing words occur. What is more, intelligent toys should realize friendly and fault-tolerant to accent and pronunciation to a certain extent on the premise of minimizing false wakeup. It is expected to show a high tolerance to dialects and respond quickly, leading to an improved experience of children, since children's pronunciation is usually not standard.
Analysis
After the input from the child, the toy processes the data. In the aspect of speech recognition, children's speech is not necessarily organized, nor obeys grammar. Children's commonly used language is also unique. Data accumulated by many AI companies apply to adult interaction but cannot fit the specific needs of children. Therefore, designers are expected to collect data on children's voice dialogue and build a speech recognition system for children's user groups. For example, the system can use keywords of their sentences to infer their meaning.
It should be tolerant of various accents and children's unique expression ways with effective probabilistic grammar induction methods (Whiting et al., Reference Whiting, Cagan and Leduc2018). Children's voice is not necessarily organized, nor necessarily follow the grammar of adults. With a built-in knowledge map suitable for children's communication, the toy should identify the general module and comprehend their meanings.
The generalization ability of the intelligent voice platform can directly affect whether the system can understand what a child is saying and the workload of the designer. At the same time, it can also reflect the level of AI of the companion toy. Generalization refers to different expressions of the same intention. For example, children may say, “can you speak louder” or “I cannot hear you,” while adults will directly say “increase the sound volume, please.” They all belong to the intention of adjusting the volume, but the difference of expression may directly lead to the design failure of the slot. Children's expression ways are much less “standardized.” Therefore, it is impossible to identify the meaning of this sentence. Designers need to write different expressions into the system continuously. The good generalization capability of an intelligent voice platform is realized by NLU (natural language understanding). Primarily, this intelligent voice platform is designed for children, so it has to be fuzzy matching with the adoption of the keyword recognition method. Taking the sound volume increase as an example, the system can recognize “louder” as a keyword and match the corresponding intention, while other words “can you speak” will be ignored. The ability of fuzzy matching improves the generalization ability of purpose, which can significantly reduce the workload of designers.
In addition to the less standardized expression, children's speaking is also captured by fuzzy pronunciation. Automatic speech recognition can convert speech directly into text. Some words in the sentence may not be heard clearly. Meantime, different words can have the same pronunciation. They can lead to errors.
Function
Multiple functions can be offered depending on the specific category of the toy. Basically, the functions of toys can be classified into two types: interaction with children and interaction with parents.
First, the intelligent toy is expected to interact with children about music/story/textbook/animation. The toy may have a chat with children, answer their questions, or finish some tasks.
For example, a toy can act as a reading partner. It is equipped with a camera that can identify the textbook. It also has an external device, a small hat with a reflector function. When the child places the reflector cap directly above the toy's head, the toy automatically enters the accompaniment reading function. By putting the textbook in front of the toys’ camera, the toy identifies the content of each page of the book with the support of image recognition technologies. When using the toy, the child only needs to choose the corresponding course, and then reads sentence by sentence along with the course content. After reading, the toy grades fluency, completion degree, and pronunciation one by one. At the same time, the toy also supports listening to standard pronunciation and playback of reading. Such toys can help children who are just beginning to learn the language to carry out pronunciation training. This function is mainly supported by voice evaluation technology and natural language processing technology to help.
Another example function is to optimize children's drawings automatically. Currently, available examples include the AutoDraw of Google and Prisma, which are officially known as the automatic correction painting tools. This kind of function is supported by image-to-image matching technologies. They can automatically generate real photos from hand-painted outline drawings, color line pictures, and realize black-and-white photo colorization.
Second, the intelligent toy is required to complete some tasks demanded by parents, such as remote interaction, reminders setting, and data management.
Parents set schedules in the “growth management” APP within the toy, such as the schedule of getting up, brushing teeth, bathing, writing homework, and going to bed. After completing the settings, at the required time, the toy can help remind the child to complete the corresponding tasks.
For example, parents might find it troublesome to make children develop the habit of brushing their teeth on time every day. On the one hand, the child can be reluctant to cooperate. On the other hand, parents are too busy to take care of them all the time and children can be rebellious. In this case, the reminder function of the toy can be of great help. Smart toys can keep reminding children regularly once the program is set. Their childish expression can make children feel genial and their rewarding system can further encourage children to strengthen habits. A Portuguese experiment verified that after the intervention of toys and games, the observed 4.5-year-old child Laura's defecation habit had been strengthened. She gained the behavioral patterns by learning about the character's behavior (toilet training) and being encouraged by the following rewarding mechanism (Coelho, Reference Coelho2008). For another example, the toy can sense abnormal situations such as fire around through its rich sensors and compare them against the built-in description or quickly search the Internet. If they match, the toy gives a warning in time and immediately informs the parents.
The intelligent companion toy should be able to transform problems into closed problems with precise inputs and corresponding outputs. Specifically, it is good at decomposing problems into various small solvable issues. Each solvable problem is clearly defined in terms of input and output, which connect the whole situation in the series. What is more, the intelligent toy should be able to find current rules or algorithms for the function to transfer inputs to outputs.
There are three standard methods. The first is to input rules similar to “if … then…” one by one. The second is to use mutual information to mine questions and answers to form common sense, which is similar to reasoning by association. The third is to build a more complex knowledge map, which is similar to causal reasoning.
Output
The design of the output should consider children's characteristics. We still mainly take voice output as an example.
First, in the aspect of speech synthesis, the toy is expected to achieve voice synthesis, so that it can imitate children's sound. For example, when a child proposes a question to a toy and the toy answers using the voice of adults, the child likely feels the toy is unfriendly. Alternatively, if the toy can use the voice of children to interact, the child becomes on intimate terms with the toy. Therefore, it is a good choice to establish a soundbank full of children's synthetic voices.
The second is the tone of the child. Children's questions should not be answered with the standard way for adults. Instead, they should be responded in a way that children like and the knowledge should be explained in a way they understand. One typical example is Siri, the voice assistant of the iPhone, which fluently chats with users in the human's tone. While, the corpus has to be redesigned for children, which is completed by human designers.
Last but not least, it is essential to get emotionally involved. Designers can realize IP role customization through multimodal human–computer interaction and customize content output according to IP role. According to the script of animation, each IP topic is generated uniquely. For example, when a child asks, “have you ever been to Paris?”, Piggy Page answers that “Yes, France is quite near to my place,” while Batman answers “Yes, I can fly to wherever I want to go.” In this way, the companion toy has a personality attribute that makes it a “living” robot. In the traditional education mode, parents usually tend to place games or toys on a secondary role compared with intellectual development and knowledge acquirement. Therefore, children bear the pressure of learning knowledge at an early stage. In contrast, researches have proved that children's self-motivated learning in a game-centered way is the most effective. Smart toys have the basic characteristics of strong human–computer interaction, functional diversity, and rich experience, which can attract children's attention, expand the scope of children's knowledge in play, and provide more opportunities to acquire new information (Copple and Bredekamp, Reference Copple and Bredekamp2009). Such multimodal interaction can help the toy meet children's colorful dreams and bring children more fun in learning.
In addition to voice output, there are many other forms of outcomes, such as images, texts, and even artificial reality (AR). The enhancement of AR experience is supported by intelligent technologies such as recognition, automatic separation of characters, and background.
User interface
In addition to waking up, input, and output interaction with children, the toy should be well designed in its appearance. When designing its exterior, designers can refer to bionics to make it look like cartoon animation, cute animals, or food to get children's love. This can help cultivate children's good moral qualities such as love and compassion, enrich children's imagination, and meet children's need to return to nature. What is more, it can alleviate the apathy and alienation brought by high technology to children, narrows the distance between products and children, and enrich the fun.
For example, a popular companion robot Woobo is covered by a plush coat with skin-soothing properties, instead of the bare plastic or silica gel shell. After its launch, it attracted wide media attention and won the first prize in the crowdfunding of children's robots in North America. Alternatively, the design of the user interface can also be referred to as cartoon IPs, which is popular among children.
The aesthetic design of the interface can be in charge of by AI. There have been real practices in other areas. For example, Alibaba developed an "intelligent design platform” called Luban with AI algorithm and training on big data, machine learning, and output design (Alibaba, 2019). On the shopping festival “Double 11” in China, Luban served in 2016 for the first time by producing 170 million product display advertisements. If designers had done them all, it would have taken 100 designers 300 years. In 2017, “Alibaba intelligent design platform” had learned millions of designers’ creative content and had evolved excellent design capabilities. The intelligent platform can draw 8000 pieces per second.
What is more, AI specially designs each poster according to the characteristics of the product image. In other words, none of the posters designed by Luban will be exactly the same. Machine intelligence has become new social productivity. The cooperation of robot and human not only improves efficiency and working quality but also makes the Double 11 super project so easy.
Similarly, AI can automatically assist in designing the user interface, including the color combination, image sizes, distances between icons and font sizes.
The first solution is to take AI as the design assistant, which suggests human designers the trend of logo, color distribution, pattern composition, font style, and other design data of the industry with big data.
The second solution is that AI directly acts as a designer, which can automatically generate design schemes. Designers input requirements, and then AI automatically generates unlimited design solutions to be chosen. Current examples include Luban and Arkie that provide massive design solutions.
We take the smart color combination as an example. First, the smart design system obtains the color data set by identifying the color of each pixel of famous paintings found on the Internet. Then, it clusters the colors with K-means algorithm and extracts several main color distributions to form the data set. Colors are expressed in the form of text. By transforming them into feature vectors and collecting color vectors of many pictures, the AI system obtains color models through repeated training. When designing the user interface of the toy, AI randomly generates one color each time, and then obtain a set of color schemes by matching the remaining colors through the models.
Evaluation and testing
Before mass production, some prototype can be produced with 3D printing quickly, so that designers can have a more intuitive observation of product modeling, structure, details, and functions. The integration of sketches and prototypes can help designers to explore in more depth and gain more creative ideas (Bao et al., Reference Bao, Faas and Yang2018).
Target users such as children and parents can help complete the usability test. Different testing methods concerning human factor engineering can be adopted, such as focusing group, thinking aloud, and probing. Some systematic methods to evaluate the satisfaction and novelty are available (Ranjan et al., Reference Ranjan, Siddharth and Chakrabarti2018). Given that there is any feedback, the design should be updated. Some common evaluation methods are briefly introduced here.
One commonly used evaluation method is heuristic evaluation, and Nielsen heuristic is an example. Experts need to evaluate the toy according to some laws: (1) Whether the companion toy matches the real world, especially the children's habits and capabilities. (2) Whether children can control the toy freely. (3) Is the toy up to industry standards. (4) Are the toy performing well in error prevention. (5) Do the toy ask for recognition rather than recall, so children's memory burden is reduced. (6) How is the flexibility and efficiency of toy. (7) Is the design aesthetic and minimalist. (8) Can the toy help children recognize, diagnose, and recover from errors. If it is not easy, is there documentation available so that adults can help.
In the heuristic evaluation process, experts search for problems according to the laws. For example, for Law (5), experts can test whether the toy offers repeated reminders and highlights important messages, so that children do not need to remember a lot of things. Experts utilize a unified standard to grade their problems, such as Jeff Robin grading method. Then, they can discuss the questions they have collected and vote for different opinions.
In addition to experts, target users, especially children and parents can offer reliable opinions. One common user evaluation method is the usability test. The users are required to use the prototype to complete a task given by the designer. For example, a child is asked to finish a dialogue with the toy about having dinner. When the user is using the prototype, an observer watches to record the problem. After the user completes the task, he or she needs to fill in a questionnaire as well. After the whole process, designers sort out the recorded problems and classify them.
After the evaluation of experts and potential users, designers improve the design draft and modify the prototype according to the importance of problems.
The iterative update process after sale
The smart iterative update process after it is put into use is also essential with two objectives. From the perspective of users, personalized update can make the toy better cater to children's or parents’ needs. On the other hand, from the perspective of the company, the personalized update or recommendation is an effective way to make continuous income.
Through frequent interaction with users and the environment, the toy consistently collects relevant knowledge. Simple data can be analyzed locally to update the system. Alternatively, users can authorize the company to collect their data so that more complex information is handled by the company cloud. Big data can be used to analyze user behaviors better. Using experience feedback can also be captured by abstracting users’ online reviews (Yang et al., Reference Yang, Liu, Liang and Tang2019). The environment-based design and update are recursive to consistently improve users’ using experience and bring positive changes to their life (Zeng, Reference Zeng2011).
Personalized update for users
After the toy is put into use, it will assist the child to make progress. At the same time, the smart companion toy is required to have a simultaneous evolution according to the child's needs, as children's psychological and behavioral characteristics keep changing when they grow up. What is more, each child and their living environment is unique. Therefore, the toy should be updated to conform to the personalized characters.
Figure 5 shows the process of coevolution of the smart toy and the child, with the information from the environment and the update suggestions from the cloud.

Fig. 5. Child's improvement and iterative update of the smart toy.
Arc arrows of each pair of different colors stand for a group of interaction between the toy and the child. For example, each time when the child has a conversation toy or has a reading, the smart toy tries to identify the child's specific grammar or accent, so that it can better recognize the meaning of the child. In return, the toy will correct the child's speaking habit with appropriate grading or encouragement, which enables the child to reduce the errors in speaking. The toy should be personalized in “waking up” calls. Children can assign a unique name to their toys.
The other example is the preference for contents, which will be introduced in detail in the section “Personalized recommendation for continuous income.” By learning the pattern of the child when listening to stories and music, such as their existing time, countries, categories, and authors, the toy knows more about the child's preference. In this way, the toy can make a personalized recommendation to the child and feeds him/her with updated contents, so the child's knowledge is enriched little by little. In addition to voice content, the toy should update the content of audio, video, text, pictures, and books. A wider variety of content access methods should be provided. To update fast and iteratively, the cloud system should continuously analyze the interaction with users, conduct the monitoring on the dialogue content, and sort scripts through both automatic analysis and manual operation. With a larger group of users, companies will find it easier to accumulate user data and develop stable updates, by better data acquisition, opinion recognition, feature summarization, and sentiment analysis (Jin et al., Reference Jin, Liu, Ji and Kwong2019). What is more, it is not sustainable in the long run when relying entirely on the free content of the third party online. To have a competitive advantage, companies should screen and reprocess the content manually which are integrated on the Internet. Then, they should better invest in high-quality paid resources developed by related experts.
The toy enhances its sense of existence by learning to interact with the specific child actively. For example, when a child comes without speaking, the toy still recognizes the child by calling its name. The child can distinguish the child's mood, such as happy or sad by observing its facial expression. This is a major challenge in cognitive, physiological engineering to determine the meaning of a given physiological measurement (Nguyen et al., Reference Nguyen, Nguyen and Zeng2016). For instance, the user can be a shy, self-conscious, and insecure girl. The toy can identify such characters by identifying her high-frequency words such as “afraid” and “where mom has gone.” The toy can analyze and imitate the mother's speaking habits and timbre using machine learning. Meanwhile, they can acquire some encouraging words from the cloud accumulated from other users. The toy can also learn the living environment of the child, such as the bed location using its sensors. When the mom is out, the smart toy can appease the child by telling the child stories using mom's tone and supervising her to go to bed and encouraging her in time.
What is more, the toy may react to the child's unsatisfactory behavior, such as regular late wakeup and excess snacks. The toy can memorize the child's daily routine and his/her usual behavior, so it can send out habit reminder. With the toy's help, the child is equipped with better behaviors and habits.
The toy should establish a unique memory system. Based on system corpus, the toy can learn and memorize different personalized data of each user. If the user's personal habits can be recorded continuously and the corresponding personalized adjustment can be made, the product's user loyalty can be further enhanced. Compared to the simple identification chips in the previous years, current AI is more intelligent. Through machine learning technologies, the toy can grow. It can answer children's questions the second time they ask even if they have no idea for the first time. With big data and deep learning, AI can accurately understand users and provide personalized services.
In addition to the mutual input with the child, the toy also evolves in line with the environment. It keeps grasping the characteristics of the environment, such as guardians’ voice, habits, the furniture location within the house, so it can send a personalized recommendation, emergency alerts and the feedback on the child's behavior to the guardians.
Apart from the mentioned common children, there are some children or families that have special needs. The update of the intelligent companion toys can be more complex and specific for them.
For example, users can be bilingual children who speak more than one language and mixes them in speaking. Each language has its own syntax and characteristics, which makes NLU unable to support all kinds of languages well. For example, Alexa, Google assistant, and Siri are all engaged in English recognition and understanding, but their knowledge of Chinese is relatively poor. The intelligent voice platforms such as Dueros and Aligenie in China have the opposite performance. It is suggested that the system automatically collect the characteristics of bilingual children's speaking and develops better algorithms to understand their input.
In addition to the typical nuclear families, there are restructured families, families with single parents, families with parents outside and children left to grandparents, and families with special needs (e.g., disabled children). Within these families, the companion is more urgent. In this case, the intelligent toy can learn from the parents speaking and gradually master the skills of simulating the voice of the parents and accompany the children with the voice of the parents.
Personalized recommendation for continuous income
In addition to the update of the system, the intelligent toy can make a personalized recommendation to parents on the new APPs or new content, such as paid stories, songs, and quizzes to obtain continuous revenue.
The core of personalized recommendation is the recommendation system, which is widely observed in e-commerce websites. For example, Amazons recommend specific products according to uses’ frequent inputs. The intelligent recommendation system can prompt the impression of various APPs, help users save searching energy, and ultimately improve the user experience. First, the system can recommend APPs or functions that parents or children may need and are also suitable for them.
It will be better if the system recommends parents or children new APPs based on their personalities, interests, and other user portrait labels. These APPs might have never been thought of by them, but they can bring surprises. Such a recommendation system is built according to the user portrait with Machine Learning and completed by four steps (Fig. 6).

Fig. 6. Steps of personalized recommendation.
The vital part of completing the personalized recommendation system is the construction of user portrait, which was first proposed by Alan Cooper, the father of interactive design. User portrait is to label users and it is divided into the following steps: (1) User modeling. It refers to determining the feature dimensions of users and identify the data source to be used. (2) Data collection. Through data collection tools, such as scripts written by programmers, the data needed to be used is stored uniformly in the toy company cloud. (3) Data cleaning. The process of data cleaning can be carried out independently or at the same time with data collection. This step is mainly to extract the data collected from various sources and disorderly fields to get structured data. (4) Model training and attribute prediction. Some features, such as parents’ consumption level, may not be directly obtained from data cleaning or data missing. Machine learning and prediction can be carried out through the collected features. For example, the system can estimate parents’ acceptable prices for new APPs/stories based on the previous contents they have purchased or the price of the intelligent toy they had. (5) Data consolidation. The user features extracted from various data sources are combined and given a certain degree of credibility. (6) Data distribution. The combined structured data is applied to personalized recommendation and output to the banner automatic synthesis system call.
After the user profile data is prepared, the data of APPs should be prepared. The recommendation system model should be trained. With obtained children's favorite contents, colors, and styles, the system will automatically synthesize and select contents such as selecting stories or synthesizing color combinations through intelligent matching. The contents are arranged in order from high quality to low quality according to the effects.
An evaluation system is needed to grade the contents or designs generated by the system. The evaluation system can be trained with a neural network.
Finally, the output is pushed to the children or parents according to the result from high grade to low grade. It can be deployed as a back-end service for users to call. After each call, the output of a personalized recommendation system can include children's favorite contents, their preferred colors, and their preferred design styles. It is noteworthy that even the same contents or APP can be introduced by different words according to the users. The automatic recommendation system can adjust the sales scripts according to each customer's personality or preferences. An example is the adobe Sensei that is linking customer intelligence directly to valuable and relevant personalized experiences.
A more accurate recommendation system is built by deep learning. Through the “mapping” method, children are mapped into a vector, and contents are mapped into a vector with the same dimension and meaning. Through neural network modeling, a recommendation system is formed.
Case study
A simple trial experiment carried out by the authors is briefly introduced to demonstrate the smart design of the intelligent companion toy.
The entire product and design process are captured by “smart” (Fig. 7). To facilitate the design process by shortening the production cycle and saving costs, virtual models were built first using SolidWorks to evaluate the appearance, components, and functions. After that, fast prototype technologies were adopted, so that a physical item was quickly obtained. As can be seen, the smart companion toy is equipped with colorful LEDs, sound pickups, infrared detectors, ultrasound identification, LCD screen, and wheels.
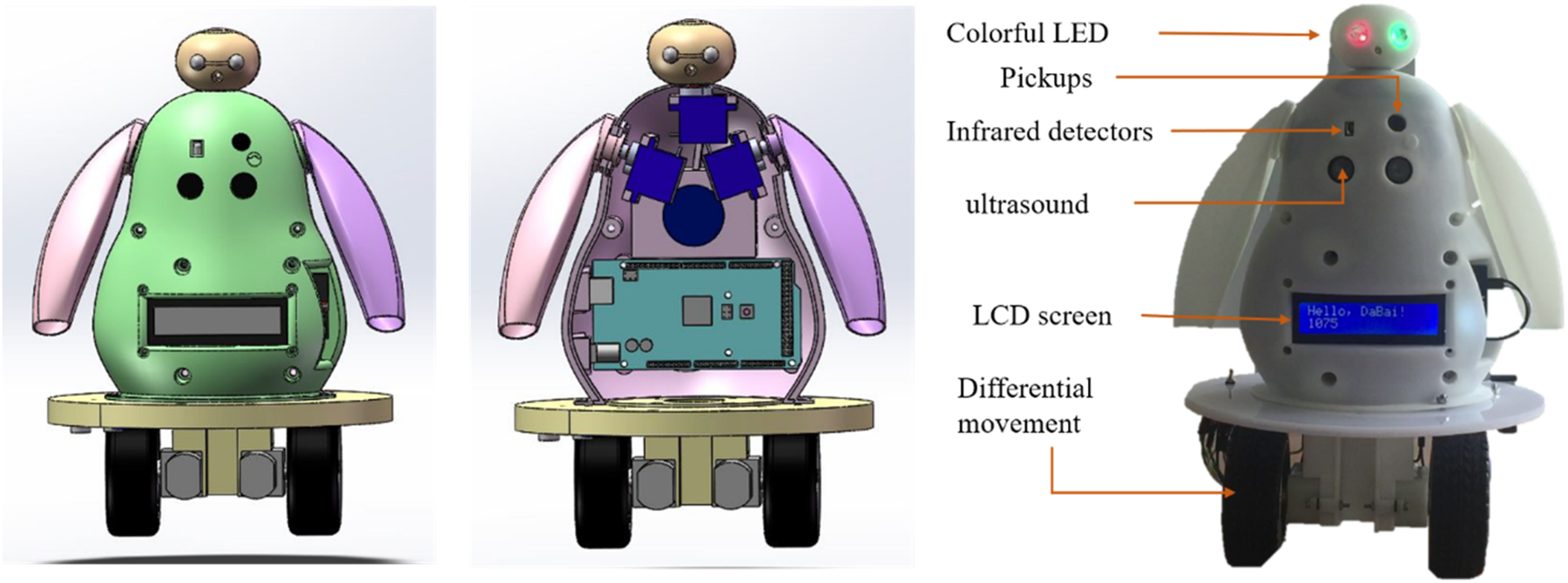
Fig. 7. Virtual model and the prototype of the smart toy.
Figure 8 offers a comparison between the suggested new design framework with the current design mode. Currently, companies make decisions on the toy's functions or appearances according to their competitive products. Meanwhile, their target interviewees when collecting customers’ requirements are mainly related adults such as parents and teachers, since they are expected to understand children and they are able to express themselves clearly. The following iteration is based on the complaints received from parents, according to which they improve the design and put new versions of smart toys onto the market.

Fig. 8. The comparison of the new design mode with the current design mode.
However, in our case study, the new design framework is adopted. Basically, it follows the process introduced in the section “Requirement confirmation and the smart design process before sale.”
Children's opinions are more valued. Though they are not capable of freely delivering their thoughts, designers are required to learn first-hand ideas of children in different ways, such as getting them involved in games and observing their behavior. Thirteen children were invited to the pre-design games and the designer had an interview with kids in two kindergartens. The appearance of the smart toy was designed based on the popular cartoon character among kids, which was Baymax.
Experts from various backgrounds such as children educators, offered professional opinions on the design.
At first, there were only 8 built-in stories and 11 songs, and it could have simple conversations with users. Then, a small batch of toys was produced and delivered to 10 families. During utilization, parents did not need to purchase a new toy to replace the old one as the child changed or had new demands. Instead, the smart Baymax toy was updated iteratively with the help of AI. It analyzed the characteristics of the child while assisting the child in enriching the knowledge, develop better characters and cultivate greater habits. After 2-month iterative updates, the number of stories and songs stored in each smart toy climbed to 26 and 31 on average, which are recommended and downloaded according to the analysis on the child's preference. The conversation was also greatly enriched. The robot system adopted the algorithm that identified the keywords within the child's dialogues and inferred his meaning. Then, the robot would confirm or modify its deduction by monitoring his following behavior. In this way, the mapping between the child's keywords and the corresponding meanings are gradually established to form a unique corpus was established. Accordingly, the robot could have a smoother communication with the child, and coevolution is well achieved smartly.
To briefly summarize, a wider range of interviewees or observed people can better characterize the features and needs of children. The application of AI makes the design process easier and faster. The smarter toy can cater to children's needs with richer functions especially with the help of iterative update.
Conclusion
The paper puts forward a complete process to smartly design and update the smart companion toys for children, which is user-centered and environment-oriented. The whole process is completed collaboratively by human designers and AI.
In theory, this paper suggests the principles and design frameworks of companion toys that are suitable for preschool children's cognitive development. It enriches the research of smart companion toys that better conforms to children's cognition features and receive little attention before. On the other hand, this paper highlights the role of AI in each step of smart design.
In terms of practical significance, this not only provides guidance for enterprises to design children's intelligent toys but also helps children's cognitive development. It is suggested that product designers and toy manufacturers take children's unique characteristics into consideration when designing smart toys, such as their ambiguous pronunciation and chaotic grammar. What is more, they are advised to take advantage of AI technologies to facilitate the designing process such as fuzzy matching, image-to-image matching, and big data technologies. In this way, the design process can be accelerated, and the iterative update is feasible.
However, there are some problems that are not discussed, such as the risk of user privacy disclosure and short endurance. Especially, the commercialization of supporting technologies are meaningful to be dealt with in future research, and it would be the core competence of smart toy manufacturers.
Acknowledgments
This research is supported by Special Program for Innovation Methodology of the Ministry of Science and Technology of China (No. 2016IM010100).
Xin Wang received her B.Eeng degree in the Faculty of Engineering in 2017 from the University of Hong Kong. She is now pursuing the PhD degree in HKU. Her research focuses on e-commerce logistics, supply chain management, and strategic decisions of new business models.
Nian Yin received his B.S. degree and M.D. in Mechanical Engineering in 2017 and 2020, respectively, from Shanghai Jiao Tong University, Shanghai, China. He is now working as a PhD student in School of Mechanical Engineering, Shanghai Jiao Tong University. His research focuses on computational design of materials, contact electrification, and development of tribology testing machine.
Zhinan Zhang received his PhD in Shanghai Jiao Tong University. From 2011 to 2013, he worked as a Postdoc in Shanghai Jiao Tong University. He is currently an associate professor and the dean assistant in School of Mechanical Engineering, Shanghai Jiao Tong University. His research interests include triboinformatics, contact electrification, computational design and analysis of tribosystems.