In this note, we propose new ways to study the effects of unmanned aerial vehicles that play an important role in America's wars. Drones, fitted with missiles to track and target individuals or groups often in remote areas, are a weapon increasingly employed in conflict zones around the world (Weidmann, Reference Weidmann2015; Berman et al., Reference Berman, Felter and Shapiro2018). They can loiter above battlefields for 24 hours while pilots remain out of harm's way (Williams, Reference Williams2013). People on the ground, including the targets, do not know of an impending attack until seconds before a missile makes impact. The US army and the CIA have launched drone strikes targeting suspected militants in Pakistan, Yemen, and Somalia.
Existing attempts to study the effects of drone strikes confront a paucity of information. Some scholars assail them as ineffective and even counterproductive (Boyle, Reference Boyle2013; Hazelton, Reference Hazelton2017). Others contend the opposite: they inflict little harm on civilians and decimate terrorist organizations (Jordan, Reference Jordan2014; Mir, Reference Mir2018; Mir and Moore, Reference Mir and Moore2019). It has proven difficult to settle these debates as these new forms of stealth warfare are by their very nature hard to study using existing methods such as in-the-field interviews, survey experiments, or randomized controlled trials. Even when possible, these means of data collection are likely to be confronted with several sources of bias (Beath et al., Reference Beath, Christia and Enikolopov2013; Blair et al., Reference Blair, Fair, Malhotra and Shapiro2013, Reference Blair, Imai and Lyall2014). Our research note highlights how tools from emerging technologies such as big data and improved computational methods could be effectively brought to the task.
Specifically, we overcome obstacles associated with data collection by examining how exogenous drone strikes impact levels of communication, with an original dataset of over 9-billion call detail records (CDRs). These CDRs consist of time- and antenna-stamped indicators of calls, offering high temporal and spatial resolution along with extensive coverage. Researchers have drawn on cellphone data generally (Gonzalez et al., Reference Gonzalez, Hidalgo and Barabasi2008; Eagle et al., Reference Eagle, Pentland and Lazer2009; Blumenstock, Reference Blumenstock2012, Reference Blumenstock2016) and CDRs specifically, to examine the change in patterns of communication after emergencies (Candia et al., Reference Candia, González, Wang, Schoenharl, Madey and Barabási2008; Bagrow et al., Reference Bagrow, Wang and Barabási2011). But leveraging CDRs to study conflict is both novel and, we believe, a promising way to shed light on the impact of opaque phenomena, such as drone strikes, on civilians in data poor contexts (Lazer et al., Reference Lazer, Kennedy, King and Vespignani2014; Bertolotti et al., Reference Bertolotti, Jadbabaie and Christia2020).
We spatially combine call data with information on drone strikes from the New America Foundation and the Bureau of Investigative Journalism (SI Data Appendix). We focus our examination on the effects of drone strikes on nationwide cell phone usage in Yemen from 2010 to 2012, a critical time when al-Qaeda took control of swathes of territory and the United States escalated its campaign of drone strikes in response.
The large scale of our data lends itself to anomaly detection methods analysis, which enables us to examine the intensity and duration of individual strikes. Our anomaly detection methods achieve this by constructing a statistical model for “normal behavior” using a training dataset, and then calculate the likelihood that a test instance has been generated from the learnt model. If a test instance has sufficiently low probability of being generated, it is considered an anomaly. Anomaly detection analysis overcomes the limitations of traditional fixed effects methods (which only provide a reliable estimate of the average effect of drone strikes), while also giving us better contextual understanding of the effects as it allows for comparisons of drone strikes to other violent and non-violent events.
Our study suggests that the impact of drone strikes in Yemen is not purely surgical. Violence causes persistent disruptions to those living nearby—even when it is as “precise” as a drone strike. Rather than affecting only militants, drones appear to have a wider ripple effect on the civilian population in the broader strike area. As the United States increasingly moves away from deploying boots on the ground and turns to indirect means of warfare, these effects are worth bearing in mind. As well, we find that drone strikes have a higher impact than al-Qaeda attacks in Yemen, even though the latter get more media attention.
These findings suggest that CDRs can be leveraged for modeling and predicting the impact of conflict, including hard-to-measure phenomena such as drone strikes or militant attacks. Furthermore, our findings allow for the comparison of drone strikes to other violent and non-violent events such as conventional strikes and civilian targeted attacks, as well as religious holidays and popular sports events. Although we identify an increase in call volume, we are unable to assess how this increased communication facilitates the continuation or end of conflict, and how militant groups might use such events to recruit supporters in affected areas as we have no information on call content. These remain important questions for future study.
Our note highlights the need for a broader research agenda on the theories and mechanisms behind big data empirical research on covert warfare. With our discipline shifting toward big data empirics and machine learning tools, traditional in-the-field survey work and qualitative research will remain an essential complement for scientific inference.
1. Theory
Existing literature has examined the effects of drone technology on a variety of dependent variables. These include the organizational longevity or capacity of a warring group (Price, Reference Price2012; Jordan, Reference Jordan2014; Shah, Reference Shah2018), the group's ability to carry out terrorist attacks (Johnston et al., Reference Johnston, Sarbahi, Dylan, Gabriel, Don and Muhammad2016), and long-term stability of and relations with the target country and its population (Johnston, Reference Johnston2012; Boyle, Reference Boyle2013; Horowitz et al., Reference Horowitz, Kreps and Fuhrmann2016). We extend this research by examining the broader impact of drone strikes on civilian daily life, as civilians have a significant impact on the dynamics of conflict, given their capacity to either aid insurgents or cooperate with the government (Berman et al., Reference Berman, Felter and Shapiro2018). We measure the impact of drone strikes on civilian lives by utilizing changes in real-time call volume during drone strikes. In the broader literature, call volume has been frequently leveraged as a proxy to measure the scale and duration of an emergency among the general population (Wang et al., Reference Wang, Li, Zhao, Feng and Luo2020). It has been used in diverse contexts ranging from natural disasters (Tomaszewski, Reference Tomaszewski2014), to the spread of disease (Baldo and Closas, Reference Baldo and Closas2013; Lima et al., Reference Lima, De Domenico, Pejovic and Musolesi2015; Tompkins and McCreesh, Reference Tompkins and McCreesh2016; Mari et al., Reference Mari, Gatto, Ciddio, Dia, Sokolow, De Leo and Casagrandi2017), and population displacement (Bozcaga et al., Reference Bozcaga, Christia, Daskalakis, Harwood, Papadimitriou, Salah, Pentland, Lepri and Letouzé2019). Beyond emergencies, call volume has served as a proxy for other social phenomena such as poverty and socio-demographics (Blumenstock and Eagle, Reference Blumenstock and Eagle2012; Blumenstock, Reference Blumenstock2016).
As noted by Bertolotti et al. (Reference Bertolotti, Christia and Jadbabaie2019), changes in call volume are also associated with other broader negative outcomes such as civilian displacement. We argue that measuring changes in call volume can proxy to what extent people register drone strikes as physical threats and social disruptions. Such disruptions are also likely to impact civilian support for operations against militants (Boyle, Reference Boyle2013; Horowitz et al., Reference Horowitz, Kreps and Fuhrmann2016).
2. Data
Drone strikes in Yemen have a relatively long history: indeed, the first American drone strike outside of a war zone occurred in Yemen in 2002, when the USA killed a member of al-Qaeda believed to be behind the attack on the USS Cole in 2000. According to the New America Foundation, there have been over 370 US drone strikes in Yemen that have killed over 1200 militants. Between January 2010 and October 2012, the United States launched 108 drone strikes and other covert actions in Yemen. Drone strikes peaked in 2012 as part of a larger campaign to retake territory from Islamic movements in the aftermath of the 2011 Yemeni revolution. Each entry in the strike dataset includes the date, location, and estimates for militant and civilian casualties. For most strikes, we also know the estimated time of day of the strike, the type of target, and whether militant leaders were killed. Figure 1 shows drone strike locations for the period under study. Many strikes occurred in the southwestern towns of Zinjibar and Jaar, where government forces battled Islamist groups for control. The majority of strikes caused fewer than ten casualties (Table S1).
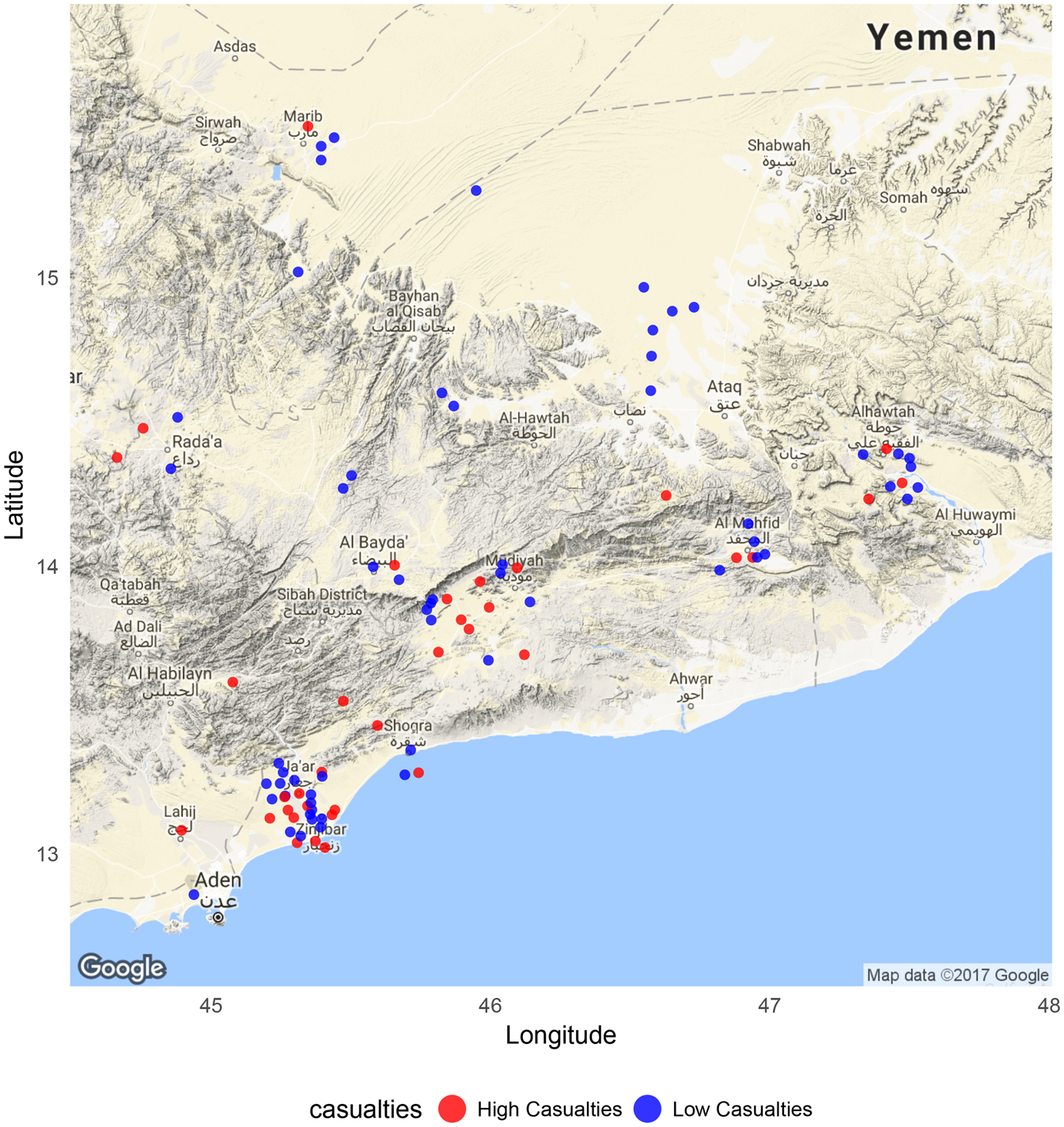
Figure 1. Drone Strikes in Yemen from 2010 to 2012. We label as high-casualty strikes with ten or more dead. See Table S1 for summary statistics.
With the cooperation of a major cellphone service provider in Yemen, we obtained nationwide CDRs for our three years of interest. This trove of data, encompasses more than 9 billion incoming and outgoing calls by 40 million distinct and fully anonymized phone numbers. For each call, we possess a unique anonymized identifier for who initiated the communication and for who received the call; the start and end time of the communication; and an identifier of the tower that serviced the communication on the side of the initiator and recipient. We also obtained information on the location of each cellular tower.
The data are broadly representative of communication patterns among Yemenis. In 2010, Yemen's population stood at 23.5 million, and although it had low Internet penetration and no mobile data network available at the time, a large proportion of Yemenis owned cell phones and predominantly drew on them during this period to make calls, rather than access the Internet or social media sites (Gelvanovska et al., Reference Gelvanovska, Rogy and Rossotto2014). Figure 2 plots the total number of daily calls serviced by all towers across Yemen in our data. Daily call volume increased on average from around 7 million in early 2010 to over 13 million in late 2012.

Figure 2. Daily call volume across Yemen including incoming and outgoing calls. Ramadan periods are highlighted in yellow.
3. Results
We present two sets of empirical results. First, we use a traditional panel setup with two-way fixed effects that exploits the temporal and spatial variation in drone strikes. Thus, our strategy is similar in spirit to a difference-in-differences approach that uses the variation of drone strikes over space and time to control for possible space- or time-specific effects. However, this design does not strictly allow for making causal inferences (Papadogeorgou et al., Reference Papadogeorgou, Imai, Lyall and Li2020).
Our main dependent variable is call volume (incoming and outgoing), which captures levels of civilian communication in Yemen. To account for the fact that people's call patterns differ by time of day, we divide the day's calls into three tighter 8-hour intervals: morning, midday, and evening. Subsequently, we aggregate our spatial data by 8-hour intervals for each unique tower location. To account for overall call volume increases over time between 2010 and 2012, we normalize call volume. Our main treatment variable is the occurrence of a drone strike within a given proximity.
The results in Figure 3 indicate that the impact of drone strikes is strongest for towers within a short range of 5 to 25 miles. Drone strikes increase call volume by about one-fifth of a standard deviation on average, representing about 850 calls during an 8-hour span for each of the surrounding towers. The impact remains significant on towers within a 100-mile range, although the effects are weaker (about one-tenth of a standard deviation).

Figure 3. Impact of drone strikes on call volume. The plot shows the coefficients and 95 percent confidence intervals from separate regressions using our OLS panel two-way fixed effects regression. Our model includes fixed effects for the tower and month and a covariate for uncertainty of the time of the strike. Standard errors are clustered by tower.
Our results are robust to different model specifications (Tables S2 and S3) and varying measures of call volume (Tables S4 and S5). We also show that our results are not driven by tower shutdowns, drone strikes during conflict, the holy month of Ramadan, multiple drone strikes on the same day, or bots (Table S6). We also test whether call volume depends on the nature and number of targets. We rerun our main specification with a series of interaction models. We find that drone strikes have a larger impact on call volume when there are more casualties. Although militant casualties prove more influential than civilian ones, militant rank does not play a role (Figure 3).
Although the fixed effects methods provide a reliable estimate of the average effect of drone strikes, we also employ anomaly detection methods to learn about the impact of individual strikes, including the duration and intensity. Our anomaly detection methods apply a statistical test to compare the observed volume of calls during each five-minute interval against a baseline sample of “normal behavior,” and determine whether the realization is likely to be part of normal behavior, or rather is anomalous (Figure 4).

Figure 4. Spikes in the call volume due to strikes. Call volume over time on the day of the strike, in red, as compared to call volume on 20 baseline days (same day of the week, for the ten weeks preceding and the ten weeks following the attack), in blue, for four strikes that our anomaly detection methods detect.
We test three prominent anomaly detection methods on the classification task of deciding between strikes and non-strikes, based only on the volume of calls, and without knowledge of whether a strike happened or not.Footnote 1
Using the selected anomaly detection settings, we detect up to 58.1 percent of all strikes with a tower within 15 miles in the dataset, indicating that the majority of strikes have a significant impact on communication patterns in Yemen. Across our selected detection settings, we find that the median duration of a call volume anomaly among the detected strikes is between 75 and 100 minutes (Figure S3). The median largest deviation in the volume of calls (z-value) during a five-minute interval is about four to five standard deviations above the mean volume (Figure S3). Overall, we find strong evidence that drone strikes have a notable impact on patterns of communication, and that our results are not driven by a few well-publicized strikes.
Drone strikes are just one type of shock detected by our anomaly detection methods. To place them in context, it is important to analyze other shocks as different event attributes (type of violence, localization, and duration) can contribute to how people react to an event. Thus, we compare the effects of drone strikes to other violent episodes, such as al-Qaeda militant attacks and the bombing of Yemen's Presidential Palace during the Arab Spring; the religious holiday marking the end of the Islamic holy month of Ramadan (Eid al-Fitr); and the big sports event of 2010 men's soccer FIFA World Cup final.
Our results suggest that instantaneous violent events, such as drone strikes and the Presidential Palace bombing during the Arab Spring, yield significant but localized effects on call volume. On the other hand, Al-Qaeda attacks, which are violent but protracted events in the Yemeni context as they include battlefield combat, register no such effect (Figure 5). As for nonviolent phenomena, consistent with expectation, we find they have a countrywide rather than a localized effect: Yemenis across the country make many more calls on the religious celebration of Eid al-Fitr than on a typical weekday during Ramadan. The World Cup final, a popular event in soccer-loving Yemen, causes nationwide spikes in call volume corresponding to key junctures during the game (Figure 5).

Figure 5. Detection of non-drone strike events. The call volume the day of the event, in red, is compared against the baseline call volume, in blue.
4. Discussion
Three US presidents since 2001 have embraced drones as a preferred means for striking suspected militants. As demand for such actions continues, we find that drone strikes are disruptive, leaving a clear and measurable communications footprint. The methods and data presented here provide a framework for combining machine learning techniques with other analytic tools to capture the effects on cellphone communication brought about by exogenous violent shocks such as drone strikes. Although we hope this starts a broader research agenda on the theory and mechanisms behind this effect, we want to emphasize the added value of combining results from a traditional panel fixed effects model with anomaly detection methods. Beyond providing robust empirics, this combination allows us to quantify the effects of drone strikes and compare them to other shocks such as bombings, al-Qaeda attacks, or important religious and social events. Our findings suggest that drone strikes have a disruptive effect with a notable local increase in communications, a result consistent with other studies suggesting that drone strikes cause information cascades within networks, as well as increased displacement (Bertolotti et al., Reference Bertolotti, Christia and Jadbabaie2019).
The nature of the data, however, divulges nothing about call content or how militants, religious leaders, or other influential figures may use them as rallying cries for increased violence (Hudson et al., Reference Hudson, Owens and Callen2012; Dafoe and Lyall, Reference Dafoe and Lyall2015; Shapiro and Weidmann, Reference Shapiro and Weidmann2015). In-the-field data collection such as interviews or survey work will remain an important complement to contextualizing such big data work.
Supplementary material
The supplementary material for this article can be found at https://doi.org/10.1017/psrm.2021.22.
Acknowledgments
Fotini Christia and Ali Jadbabaie recognize support from ARO MURI award No. W911NF-121-0509.