Mastitis is inflammation of the mammary gland that follows bacterial infection (although rare, other agents can cause mastitis i.e. viral and fungal infections). The bacterial infections that cause mastitis occur in the udder quarter following entry of bacteria through the teat canal. In response to this bacterial infection, the somatic cell count (SCC) of the milk will increase, with a SCC of 200 000 cells/ml generally accepted as an indicator of the presence of a mastitis infection (International Dairy Federation, 1997). However other literature suggests that the SCC for a healthy lactating cow should not exceed 100 000 cells/ml (Doggweiler & Hess, Reference Doggweiler and Hess1983; Kromker et al. Reference Kromker, Grabowski, Redetzky and Hamann2001). In Ireland, as per EU regulations the SCC cut off for milk purchasers is 400 000 cells/ml. In 2011 the Irish national mean bulk milk SCC (BMSCC) was estimated at 252 000 cells/ml (National Farm Survey, Dairy Enterprise, 2011).
Bacterial infections that cause mastitis have significant financial impact on the dairy industry at both farm and processor level. While there are a number of published estimates on the costs of mastitis at farm level (Malcolm et al. Reference Malcolm, Hook, Montgomerie, Winkleman, Sim and Brennan2005; Huijps et al. Reference Huijps, Lam and Hogeveen2008; Geary et al. Reference Geary, Lopez-Villalobos, Begley, McCoy, O'Brien, O'Grady and Shalloo2012a) less focus has been paid to the impact mastitis has on the processing sector and ultimately its effect on milk price. Hogeveen et al. (Reference Hogeveen, Huijps, Halasa and Lam2010) and Malcolm et al. (Reference Malcolm, Hook, Montgomerie, Winkleman, Sim and Brennan2005) characterised the impacts of mastitis at processor level indicating lower product quality, more complex processing requirements, lower cheese and casein yield, shorter shelf life and flavour problems as significant factors, however those studies did not quantify the impact of these factors on the value of milk. These issues impact processor income, costs or both, which indirectly impacts the returns to the farmer in terms of milk price paid. Estimating the financial impact of mastitis or any animal diseases on the milk processing sector has not to date been reported in the international literature.
The effect of SCC on raw milk and cheese composition has been examined extensively however there is considerable variability within the literature on the direction and scale of these effects. Meta-analysis is a useful tool to synthesise the available literature to estimate relationships between SCC and raw milk composition, cheese processing and cheese composition. A meta-analysis combines the results of many studies, has greater power than individual studies to detect small but significant effects of various components and gives more precise estimates of the size of the effects (St-Pierre, Reference St-Pierre2001; Crombie & Davies, Reference Crombie and Davies2009). Using the meta analysis methodology to quantify the impact of SCC on the composition of raw milk, cheese processing and composition using available published literature provides a method to determine the impact that elevated SCC (due to mastitis infection) has on the processing sector which currently is not well understood.
The objectives of this paper were firstly to examine the relationship between BMSCC and raw milk composition, cheese processing and cheese composition via a meta-analysis and secondly to utilise this to determine the impact of SCC on the volume of products that can be produced (independent of the effects of mastitis on milk volume), total processing costs, market returns, net revenue, milk price and the values per kg of fat and protein within the Irish context.
Materials and methods
Meta-analysis methodology
For the purpose of the meta analysis only SCC was converted to somatic cell score (SCS), to normalise the data, based on calculations by Wiggans and Shook (Reference Wiggans and Shook1987): SCS=log2 (SCC) (BMSCC was used throughout the processing model analysis). Two sets of analyses were carried out, the first to determine relationships between SCS and raw milk composition and the second to determine relationships between SCS and cheese processing characteristics and cheese composition.
Model
The change in (1) the milk composition variables and (2) the cheese processing and composition variables as SCS changed were analysed with random regression models with linear, quadratic and cubic effects using the MIXED procedure in SAS 9.3 (SAS, 2010).
The model used was:

where ykm is observation k in study m for any of the dependent variables (i.e., fat content, protein content, etc.), bi are fixed polynomial regression coefficients of SCS on variable y (b 0=intercept, b 1=linear effect, b 2=quadratic effect and b 3=cubic effect), αim are random regression coefficients of SCS on variable y in study m (α0m=intercept, α1m=linear effect, α2m=quadratic effect and α3m=cubic effect), x kmi is the kth observation of SCS in study m at the power 0, 1, 2 and 3, and ekm is the residual error associated with observation ykm.
The regression coefficients were not weighted by their se, as many of the scientific articles had not reported se in their findings. Linear, quadratic and cubic effects were declared to be significant at a probability of less than 0·10.
Inclusion criteria
A systematic review of the literature was carried out using Google Scholar (index includes most peer-reviewed online journals of Europe and America's largest scholarly publishers). All relevant articles were eligible for inclusion regardless of publication date. The search terms included: SCC, mastitis, milk composition, cheese, processing, dairy products and milk quality. References of every identified article were reviewed to identify any omitted articles. For a study to be included in the analysis it had to report milk composition and/or cheese processing and/or cheese composition by SCC. Data must be reported in a usable format, i.e. data presented in graphs were not inferred and so were excluded in the analysis. Systematic reviews were excluded from the analysis, while they provided an overview of the literature they did not report numerical values which could be included in the meta-analysis, in this instance the original publications proved superior data sources. In total 32 and 13 published articles were included in the meta-analysis of raw milk composition and cheddar cheese composition, respectively. The articles spanned from 1980–2009 and were representative of the international literature with data from New Zealand, US, Australia, mainland Europe etc.
Databases
Two databases were constructed: D1 related to SCC and raw milk composition and D2 related to SCC and cheese processing and composition. In the databases rows represented treatments or groups and columns represented treatment characteristics and measured variables. Each experiment included in the database was assigned an individual study number. Where multiple years of data were reported each year of data was included in the database.
Database 1
The data captured in D1 were SCC, crude protein (CP), true protein (TP), total nitrogen (TN), non protein nitrogen (NPN), non-casein nitrogen (NCN), casein (CN), casein as a percentage of true protein ratio (CN/TP), whey protein, whey fat, fat, lactose, total solids (TS) and solids non-fat (SNF) content of milk.
Not all variables were reported in all studies, where possible these variables were calculated. As per industry standard, TP was calculated by multiplying CP by 94 % (Barbano & Lynch, Reference Barbano and Lynch1999). Total nitrogen was calculated by dividing CP by 6·38 and NPN was calculated by subtracting TP from CP (Barbano & Lynch, Reference Barbano and Lynch1999).
Database 2
The data captured in D2 were SCC, protein, fat, protein-to-fat-ratio, protein recovery, fat recovery, fat in whey, protein in whey, NCN in whey, CN in whey, moisture and TS in cheese and cheese making. As before, each of the variables captured in the database were not consistently reported in all studies included in the D2 database, no variables were calculated in this instance.
Moorepark processing sector model
The Moorepark Processing Sector Model (MPSM) (Geary et al. Reference Geary, Lopez-Villalobos, Garrick and Shalloo2010, Reference Geary, Lopez-Villalobos, Garrick and Shalloo2012b) was used to determine the impact of BMSCC on the milk processing sector. The study outcomes were characterised by five BMSCC categories of ⩽100 000, 100 001–200 000, 200 001–300 000, 300 001–400 000 and >400 000 cells/ml, similar to Geary et al. (Reference Geary, Lopez-Villalobos, Begley, McCoy, O'Brien, O'Grady and Shalloo2012a). The MPSM estimated the volume of product that can be produced, market returns, processing costs, net revenue (total revenue−total costs), milk price and the component values of milk for each BMSCC category. For each of the five categories, the product returns were determined for a fixed national milk volume of 5377 million litres (representative of the volume of milk processed in Ireland in 2011; Central Statistics Office, 2011). The national average BMSCC in Ireland is 252 000 cells/ml (National Farm Survey, Dairy Enterprise, 2011), therefore the BMSCC category 200 001–300 000 cells/ml represented this baseline.
Model description
The MPSM is described in detail by Geary et al. (Reference Geary, Lopez-Villalobos, Garrick and Shalloo2010, Reference Geary, Lopez-Villalobos, Garrick and Shalloo2012b). Briefly the approach uses a mass balance milk processing-sector model that accounts for all inputs, outputs and losses involved in dairy processing. The model is a mathematical representation of the conversion of milk into dairy products. Within the model the production of cheese, casein, butter, whole milk powder (WMP), skim milk powder (SMP) and fluid milk are simulated, with the by-products of butter milk powder (BMP), whey powder (WP) and cream being further processed or sold. The proportion of milk that is directed toward the production of each product is specified in the model. The model separates a proportion of the milk into cream and skim milk based on the composition of: (a) the milk and (b) the final product to be manufactured. The quantities of products and by-products that can be produced from the available milk pool, with given product specifications, are calculated. Processing costs are estimated, the returns from the products produced are calculated, the net revenue is determined (total revenue – total costs) and the values per kg of fat and per kg of protein are derived in the model. The model can provide annualised (Geary et al. Reference Geary, Lopez-Villalobos, Garrick and Shalloo2010) or seasonal inputs and outputs (Geary et al. Reference Geary, Lopez-Villalobos, Garrick and Shalloo2012b). In this analysis annualised inputs and outputs are presented.
Model inputs
Processing costs
The processing costs assumed in this analysis were representative of those incurred by Irish dairy processors (Geary et al. Reference Geary, Lopez-Villalobos, Garrick and Shalloo2010, Reference Geary, Lopez-Villalobos, Garrick and Shalloo2012b) and are presented in Table 1. The unit processing costs were applied either to the volume of milk being processed or the volume of product produced. Storage and finance costs were included in the analysis.
Table 1. Volume and product related processing costs assumed in the Moorepark Processing Sector Model†
WMP=Whole milk powder, SMP=Skim milk powder, WP: Whey powder; BMP=Butter milk powder
† Source: Geary et al. (Reference Geary, Lopez-Villalobos, Garrick and Shalloo2012b)
‡ Quinlan et al. (Reference Quinlan, Keane, O'Connor, Shalloo, Gosh and Murray2010)
Fixed costs
Fixed costs were included at a rate of €0·015 cents per litre which was validated in consultation with representatives of the dairy industry. These costs include rents and rates, depreciation, quality control, management, research and development, marketing, administration and IT.
Product mix
The type of products and volume of milk used to produce each product assumed in this analysis was representative of production in Ireland in 2010 (FAOSTAT, 2010). Milk intake throughout the year was apportioned 31% butter, 16% SMP, 40% cheese and 13% WMP. Surplus cream from cheese, SMP and WMP production would have gone into butter production. Cheese production capacity was capped at 150 million litres of milk per month, as per the current cheese processing capacity in Ireland (Industry consultation). When cheese production capacity was met, the remainder of the milk pool was apportioned to butter/SMP (fat to butter, skim milk to SMP) and WMP at a ratio of 78 : 22, respectively (FAOSTAT, 2010). Cheese was not produced in the months of January or December due to milk quality issues associated with late lactation milk from spring calving herds in these months, this reflects processor practice in Ireland (Guinee et al. Reference Guinee, Mulholland, Kelly and O'Callaghan2007). During these months when cheese was not produced, the milk pool was apportioned 43, 43 and 14% to butter, SMP and WMP, respectively.
Market values
The market values assumed in this analysis were from the Dutch official quotation system (Productschap Zuivel, 2011), as used by the Irish Dairy Board in financial analyses. The monthly market prices were representative of the 3-year average from 2009 to 2011. The market values for butter, WMP, SMP and WP were representative of market prices in the Netherlands. The market price for cheese was representative of the UK cheddar cheese market price (Datum UK, 2011) and the market price for casein was representative of the US casein market price (CLAL, 2011). As in Geary et al. (Reference Geary, Lopez-Villalobos, Garrick and Shalloo2010, Reference Geary, Lopez-Villalobos, Garrick and Shalloo2012b) the market price for BMP was assumed equivalent to the market price for WMP. The annual average market values assumed per tonne of product were cheese €3169, butter €3293, WMP €2573, SMP €2107, BMP €2573 and WP €673.
Analysis assumptions
The findings of the meta-analysis were applied with the assumption that the baseline (200 001–300 000 cells/ml) was representative of the national raw milk composition, cheese processing and cheese composition. Subsequently, the other BMSCC category information was calculated from this baseline using the meta-analysis results to estimate the BMSCC effect for the other BMSCC categories. These assumptions were then incorporated into the MPSM to examine the impact of increasing BMSCC on processing returns.
Milk
Relationship between BMSCC and raw milk composition
The volume of raw milk being processed in this analysis was 5377 million litres/year (CSO Ireland, 2011), the baseline fat (3·89%) and protein (3·37%) content for this milk pool was taken from CSO data (2011). The lactose content of milk was based on outputs from the Moorepark Dairy Systems Model (Shalloo et al. Reference Shalloo, Dillon, Rath and Wallace2004) as it is not reported by the CSO. The milk supply and composition are representative of a national mean calving date of mid-March. The CSO milk data is representative of the national average BMSCC (baseline BMSCC category). Applying the outputs of the meta-analysis to the baseline milk composition showed that as BMSCC increased the fat and protein content of milk increased, the lactose content decreased and the amount of usable protein decreased (Table 2).
Table 2. Annual average fat, protein and lactose content of milk for each bulk milk somatic cell count category†

† These percentages were applied to the national volume of milk being produced in Ireland (5377 million litres)
‡ Baseline milk is assumed to account for the national mean somatic cell count. Baseline milk volume, fat and protein content of milk were sourced from CSO Milk statistics (2011)
§ Fat, protein and casein in protein content of milk for the bulk milk somatic cell count category was calculated using the results of the meta analysis
¶ Baseline lactose content of milk was predicted using the Moorepark Dairy Systems Model (Shalloo et al. Reference Shalloo, Dillon, Rath and Wallace2004), the lactose content of milk for the other SCC categories was calculated using the results of the meta analysis
Final products
Relationship between BMSCC and cheese production
Utilising the assumed baseline (200 001–300 000 cells/ml) fat (93%) and protein (99%) recoveries and applying the results of the meta-analysis the following values were assumed in the analysis. As BMSCC increased fat recovery was estimated at 94·12, 93·42, 93·00, 92·72 and 92·70% for BMSCC levels of <100 000 cells/ml, 100 001–200 000 cells/ml, 200 001–300 000 cells/ml, 300 001–400 000 cells/ml, and >400 000 cells/ml, respectively. As BMSCC increased protein recovery was estimated at 99·91, 99·34, 99·00, 98·77 and 98·76% for BMSCC levels of <100 000 cells/ml, 100 001–200 000 cells/ml, 200 001–300 000 cells/ml, 300 001–400 000 cells/ml, and >400 000 cells/ml, respectively.
Relationship between BMSCC and cheese composition
The findings of the meta-analysis were applied to the baseline (200 001–300 000 cells/ml) cheese moisture (35·26%) and cheese protein (24·50%) from industry consultation to calculate the BMSCC adjusted moisture and protein content of cheese. Table 3 summarises the annual average moisture and protein content of cheese across each of the BMSCC categories.
Table 3. Composition of dairy products produced for each bulk milk somatic cell count assumed in the Moorepark Processing Sector Model
† Baseline composition values sourced from Geary et al. (Reference Geary, Lopez-Villalobos, Garrick and Shalloo2010, Reference Geary, Lopez-Villalobos, Garrick and Shalloo2012b)
‡ Protein and moisture percentage of cheese calculated using results of the meta analysis. Fat and lactose content of cheese was not found to be significantly affected by increasing somatic cell count, therefore these values are fixed
§ SMP: Skim milk powder; WMP: Whole milk powder; BMP: Butter milk powder; WP: Whey powder
¶ SMP composition <100 and 100–200 000 cells/ml assumed generic values, unaffected by SCC
†† Incremental changes in SMP composition calculated based on Rogers & Mitchell (Reference Rogers and Mitchell1989)
‡‡ WMP composition <100 and 100–200 000 cells/ml assumed generic values, unaffected by SCC
§§ Incremental changes in WMP composition calculated based on Auldist et al. (Reference Auldist, Coats, Sutherland, Clarke, McDowell and Rogers1996)
¶¶ No evidence to suggest a change in the composition of butter, BMP, or WP as SCC increases
Relationship between BMSCC and the composition of other dairy products
The impact of BMSCC on the processing and composition of SMP, WMP and butter, has seldom been reported in the scientific literature. The composition of SMP and WMP assumed in the analysis for the baseline category (200 001–300 000 cells/ml) was reflective of the average composition of these products in Ireland (Industry consultation). The composition of SMP and WMP for each of the other BMSCC categories was calculated using the incremental changes from the baseline as reported by Rogers & Mitchell (Reference Rogers and Mitchell1989) and Auldist et al. (Reference Auldist, Coats, Sutherland, Clarke, McDowell and Rogers1996), respectively. The effect of BMSCC on butter was not included in the analysis due to a lack of quantifiable published research in this area. The composition of SMP, WMP and butter produced from milk across each of the BMSCC categories are presented in Table 3.
Model outputs
Effect of BMSCC on the value of milk
The net revenue in this model is calculated as total revenue minus total costs. To calculate the milk value (€ cents/l), the net revenue was divided by the total volume of milk processed (5377 million litres). The value per kg of milk solids (€/kg) was calculated by dividing the net revenue by the total kg of fat and protein in the milk. The value per kg of fat and protein were calculated using the marginal rate of technical substitution (MRTS) (Geary et al. Reference Geary, Lopez-Villalobos, Garrick and Shalloo2010) which accounts for the change in milk solids and net revenue as BMSCC increased. The value per kg of fat and protein presented in this analysis for each BMSCC category accounted for the change in milk and product composition and the volume of product produced as BMSCC increased.
Sensitivity analysis
All dairy products receive a grading which represents the quality of the product. This grading relates to the market value that products receive: products with high grading receive full market value and products with low grading, indicating inferior quality, receive lower market values. Dairy products produced from high SCC milk have shorter shelf life and poorer organoleptic properties (flavour, texture, colour, odour) than products produced from low SCC milk (Ma et al. Reference Ma, Ryan, Barbano, Galton, Rudan and Boor2000; Santos et al. Reference Santos, Ma and Barbano2003; Hickey et al. Reference Hickey, Kilcawley, Beresford, Sheehan and Wilkinson2006), which can negatively impact their grading. To explore the impact of a change in the product market values due to elevated BMSCC, sensitivity analysis was carried out. Data on the relationships between BMSCC, grade and market value are not available; therefore the following assumptions were made: the baseline market values (200 001–300 000 cells/ml) were representative of the values currently received; the market values were assumed 10% higher for the <100 000 cells/ml; 5% higher for the 100 001–200 000 cells/ml, 5% lower for the 300 001–400 000 cells/ml and 10% lower for the >400 000 cells/ml BMSCC categories (Table 4).
Table 4. Product market values assumed in the sensitivity analysis

† Market values assumed 10% higher than the baseline
‡ Market values assumed 5% higher than the baseline
§ Baseline market values were assumed in the base case analysis
¶ Market values assumed 5% lower than the baseline
†† Market values assumed 10% lower than the baseline
‡‡ WMP: Whole milk powder; SMP: Skim milk powder BMP: Butter milk powder; WP: Whey powder
Results
Meta-analysis results
Relationship between SCS and raw milk composition
Linear. Somatic cell score had significant positive relationships with CP (P<0·01), TP (P<0·01), TN (P<0·01), NPN (P<0·05), whey protein (P<0·01) and fat (P<0·05) (Table 5) content of milk, with the proportion of each component in milk increasing as SCS increased. A significant negative relationship between SCS and lactose (P<0·01) and CN/TP (P<0·01) was identified by the model, with the content of both decreasing as SCS increased (Table 5).
Table 5. Significant effects of somatic cell score on raw milk composition
CP=Crude protein, TP=True protein, NPN=Non protein nitrogen, CN=Casein
The relationship between SCS and CN and TS content of milk was not found to be significant. The effect of SCS on NCN, whey fat and SNF could not be determined by the model.
Quadratic and Cubic. The quadratic and cubic effects were found to not be significant.
Relationship between SCS and cheese processing and composition
Linear
Cheese moisture increased by 0·546% (P<0·05) as SCS increased by one unit (Table 6). Somatic cell score had a significant negative relationship with cheese protein (P<0·10), protein recovery (P<0·10) and fat recovery (P<0·10) (Table 6).
Table 6. Significant effects of somatic cell score on cheese processing and cheese composition

The relationship between SCS and protein in whey and protein:fat ratio was not found to be significant. The relationship between SCS and fat in whey and cheese fat could not be estimated by the model.
Quadratic and Cubic
None of the quadratic or cubic models were found to be significant, with the exception of moisture where SCS had a significant positive quadratic relationship with cheese moisture (P<0·01).
Moorepark processing sector model
Quantity of product produced. As BMSCC increased from <100 000 to >400 000 cells/ml the quantity of cheese, butter, WMP, SMP and WP were all reduced by 4217, 623, 9881, 7568, 2052 tonnes, respectively; while production of BMP increased by 682 tonnes (Table 7).
Table 7. Annual volume of products produced from 5377 million litres/year for each bulk milk somatic cell count category

WMP=Whole milk powder, SMP=Skim milk powder, BMP=Buttermilk powder, WP=Whey powder
Total revenue. Increasing BMSCC from <100 000 to >400 000 cells/ml resulted in total revenue decreasing by 2·8% or €56·6 million nationally (Table 8).
Table 8. Annual total revenue, total costs, net revenue, milk price and component values of milk for each bulk milk somatic cell count category when 5377 million litres/year were processed into a representative mix of dairy products in the Irish dairy industry
† Net revenue (total revenue – total costs)
‡ Average milk price paid throughout the year (net revenue/total volume of milk processed)
§ Average value per kg of fat paid throughout the year within the milk pricing system
¶ Average value per kg of protein paid throughout the year within the milk pricing system
Processing costs. Total processing costs reduced from €375·3 million at a BMSCC of <100 000 cells/ml to €370·1 million at a BMSCC of >400 000 cells/ml, a reduction of €5·2 million (Table 8).
Net revenue. As BMSCC increased from <100 000 to >400 000 cells/ml the net revenue generated decreased by €51·3 million per annum (Table 8).
Value of milk. The average milk price decreased from €0·3008 cents/l at a BMSCC <100 000 cells/ml to €0·2912 cents/l at a BMSCC >400 000 cells/ml (Table 8). The value per kg of milk solids decreased by €0·40 cents/kg as BMSCC increased from <100 000 to >400 000 cells/ml.
Component values of milk within the milk pricing system. As BMSCC increased from <100 000 to >400 000 cells/ml the value per kg of fat and protein decreased by €0·04 and €0·24 cents/kg, respectively (Table 8).
Sensitivity analysis
Incorporating the market values into the analysis resulted in the net revenue ranging from a high of €1782·2 at a BMSCC of <100 000 cells/ml to a low of €1372·3 at a BMSCC of >400 000 cells/ml (Table 9), thus highlighting how sensitive the model outcomes are to changes in product market values.
Table 9. Sensitivity analysis: Total revenue, total costs, net revenue, milk price and component values of milk for each bulk milk somatic cell count category
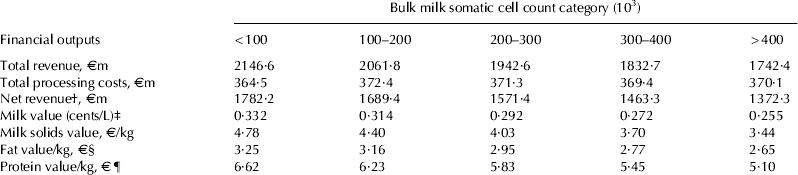
† Net revenue (total revenue – total costs)
‡ Average milk price paid throughout the year (net revenue/total volume of milk processed)
§ Average value per kg of fat paid throughout the year within the milk pricing system
¶ Average value per kg of protein paid throughout the year within the milk pricing system
Discussion
Meta-analysis
There is consensus in the literature that as SCC increases, milk TN increases, CN/TP decreases, whey protein increases and milk lactose decreases. Evidence on the effect of SCC on other milk components is varied in terms of direction, scale and significance. Similarly, authors agree that as SCC increases fat in whey increases, moisture in cheese increases and protein in cheese decreases. The literature is varied on the effect of SCC on other cheese production and composition variables. Therefore the inclusion of this data in a metaanalysis allows the direction and scale of the effect to be quantified. However, relying on the information that is published in studies creates a number of issues which should be highlighted: there may be information gaps in what is reported, there is potential for publication bias as generally only statistically significant effects are reported in the literature. Ideally a controlled trial examining milks of well-defined SCC categories (no pooling of milks) and using this milk to produce various dairy products is the optimal strategy to meet the current data gaps; utilising the available published data in a meta-analysis was the most practical and readily available solution.
Processing model analysis
The current analysis highlighted that there are gains in the region of at least €19·8 million to be made for the Irish dairy processing sector if the national mean SCC of 252 000 cells/ml could be reduced to <200 000 cells/ml. In New Zealand the annual cost of mastitis to the industry is estimated at NZ$300 million (Denis et al. Reference Denis, Wedlock, Lacy-Hulbert, Hillerton and Buddle2009), while in the US it is estimated at over $2 billion (Cazoto et al. Reference Cazoto, Martins, Ribeiro, Durán and Nakazato2011) however these estimates only capture treatment, lost production costs and other on-farm costs. As the current analysis demonstrates additional costs of mastitis are incurred at processor level, over and above the on-farm costs.
Raw milk & cheese composition
The meta-analysis showed there were milk and cheese compositional changes as SCC increased. Each of these changes has an impact on the quantity and quality of product that can be produced. The higher moisture content of cheese affects the organoleptic properties of cheese (Auldist, Reference Auldist2000) which can result in lower cheese grades, ultimately resulting in lower product market value and lower returns for the processor and the farmer. The sensitivity analysis examined in the current paper demonstrated how sensitive the model outcomes were to changes in the product market values. Product produced from low BMSCC (<200 000 cells/ml) would be of higher quality and should theoretically receive higher market values.
Cheese yield
As BMSCC increased from <100 000 to >400 000 cells/ml there was a reduction of 2·05% in cheese yield. The cheese yield calculated in the model is theoretical cheese yield based on the Van Slyke & Price (Reference Van Slyke and Price1949) equation which takes the form:

where Y=yield of cheese, Xf=percentage fat in the milk, Xp=percentage protein in the milk, and W=water content of the cheese. The meta-analysis found that as BMSCC increased the protein (Xp) and fat (Xf) content of milk significantly increased, in addition as BMSCC increased the water content of cheese (W) significantly increased, each of these changes positively impact cheese yield. Milk protein is made up of casein and whey. The casein proportion is used in curd formation and the whey proportion leaves the cheese process in a liquid form.

where Xp=percentage protein in the milk, Y=yield of cheese, Xf=percentage fat in the milk, 99%=efficacy of casein utilisation, and 98%=adjustment for non-casein protein in the cheese. The cheese yield is calculated by dividing the volume of cheese protein by the required protein content of the final cheese product.
A change in CN/TP and a reduction in protein recovery due to elevated SCC has a negative impact on cheese yield as shown in this study. Politis & Ng-Kwai-Hang (Reference Politis and Ng-Kwai-Hang1988) found an increase in SCC from 100 000 to 500 000 cells/ml resulted in a 5% decrease in adjusted cheese yield and 11% in yield efficiency, they found that the reduction in cheese yield was progressive as SCC increased. Barbano et al. (Reference Barbano, Rasmussen and Lynch1991) also concluded that any increase in milk SCC above 100 000 cells/ml would negatively impact cheese yield efficiency.
Other products
Relative to cheese, little research has been published on the effect of SCC on the production of other dairy products namely SMP, WMP and butter (Auldist, Reference Auldist2000). Rogers & Mitchell (Reference Rogers and Mitchell1989) found that as SCC increased the moisture and lactose content of SMP decreased significantly and the protein content increased significantly. The results of that analysis were incorporated into the MPSM. Auldist et al. (Reference Auldist, Coats, Sutherland, Clarke, McDowell and Rogers1996) examined the effect of SCC and stage of lactation on WMP and found that WMP made from late lactation, high SCC milk had significantly higher protein and lower lactose content. Again, the findings of Auldist et al. (Reference Auldist, Coats, Sutherland, Clarke, McDowell and Rogers1996) were incorporated into the current analysis.
Payment schemes
The current analysis showed that reducing BMSCC from 200 001–300 000 cells/ml (Irish national mean SCC) to a BMSCC of <100 000 cells/ml resulted in higher values per kg of fat and per kg of protein within the milk pricing system. This indicates that there is scope to incorporate a targeted milk pricing system where higher fat and protein values are paid out when BMSCC is <100 000 cells/ml. Many Irish milk processors implement bonus and/or penalty schemes, however currently there is no uniformity across the industry in relation to milk payment. Barbano et al. (Reference Barbano, Rasmussen and Lynch1991) argued that for a milk payment system that more correctly reflects the true differences in the functional value of milk, a better quantitative index of differences in cheese yield capacity and overall milk quality is required; the analysis presented here provides that for the Irish dairy industry. Valeeva et al. (Reference Valeeva, Lam and Hogeveen2007) found Dutch farmers were more motivated by a price decrease for high SCC milk than a price increase for low SCC milk, Huijps et al. (Reference Huijps, Hogeveen, Antonides, Valeeva, Lam and Oude Lansink2010) found that the average penalty needed to change management on Dutch farms at a BMSCC of 350 000 cells/ml were 0·65 times lower than the bonus needed. Examining Dutch Dairy Farmers Berry et al. (Reference Berry, O'Brien, O'Callaghan, Sullivan and Meaney2006) concluded it is just as important to encourage farmers to maintain low BMSCC as well as to encourage farmers to reduce high BMSCC. Nightingale et al. (Reference Nightingale, Dhuyvetter and Schukken2008) advocated a blended penalty (high SCC)/premium (low SCC) programme to provide strong incentive for improvement in milk quality. Similar analysis could be conducted in Ireland to understand what the optimal penalty/bonus scheme would be to motivate Irish dairy farmers to reduce BMSCC.
The Irish dairy industry with a national average BMSCC of 252 000 cells/ml is losing €19·8 million per annum in net revenue relative to a BMSCC of 100 001–200 000 cells/ml when only accounting for the processing sector. The methodologies demonstrated in this paper and the findings of the analysis could be utilised to develop payment systems that reflect the functional value of milk and support the production of low SCC milk for the Irish dairy industry.