Policymakers and scholars have long been concerned with the efficacy of international efforts to reform recalcitrant states. Studies have focused on the effectiveness of a range of tools from military interventions and sanctions to softer methods such as shaming and persuasion or facilitative instruments such as treaty commitments, education, and capacity training to achieve political reform. One area that has elicited close scrutiny has been efforts to improve human rights. Have norms ensconced in international law helped guide the development of better practices and protections? Have efforts to expose human rights violations incentivized better government behavior? For a long time, research concentrated on demonstrating positive effects; the null hypothesis was ineffectiveness.
As human rights research has matured, scholars have started asking whether well-intended interventions sometimes may have benefits while also causing harm.Footnote 1 For example, has civil society advocacy simply caused flagrant rights violations to become stealthier? Have international legal commitments proscribing specific behaviors, such as political imprisonment, diverted abuses to worse outcomes like disappearance? Have rights commitments crowded out development objectives? Is all the attention on negative rights responsible for fleeting attention to social justice and equality? In short, is the rights agenda virtually doomed not only to be ineffective but to cause more damage to humankind than it has done good?
Such questions are important for both scholars and practitioners. Given their focus on constrained or adaptive human behavior, these questions can inform the ongoing theoretical debates about the relationship between international and national actors, law and politics, and civil society and government agents. Such questions hold tremendous policy relevance. Advocates and policymakers must consider the benefits and the costs of their actions, as well as their distributional and moral consequences. This is not possible, however, if unintended consequences are not fully examined and incorporated. Scholars are right to ask questions about possible negative effects of policy interventions.
As important as it is to study an intervention's negative spillovers, it is also important to recognize that this study is fraught with challenges. Appropriately, tests for first-order consequences have confronted the plethora of typical methodological criticisms surrounding causal inference, but claims of second-order, unintended consequences often do not garner such a hard kick to the evidentiary tires. Social scientists should not give unintended consequences a causal pass. Good social science and sound policy advice demand that we test for the mechanisms suspected of negative effects just as thoroughly as we do the causal mechanisms associated with first-order positive claims and articulate clearly the evidence for the mechanism leading to net negative outcomes. The famous Downs, Rocke, and Barsoom caution that we cannot attribute compliance to states that ratify treaties because they were going to improve anyway is conversely apt.Footnote 2 When bad states do bad things and get criticized for it, the same logic must apply, with a symmetrical burden of proof. Too few researchers ask whether the bad news about repression is bad news about international law and/or human rights advocacy, or were these states going to misbehave anyway?
We call attention to the evidentiary deficits in much of the research on negative spillovers from the promotion of human rights norms and offer a rigorous way to test such claims. We first describe three distinct mechanisms through which human rights interventions are said to cause negative consequences: backlash, trade-offs, and counteraction. Claims of these consequences crop up repeatedly in the critical human rights literature as unintended spillovers caused by well-intended but naive human rights interventions, such as international legalization, enhanced monitoring, or human rights advocacy.
After discussing these mechanisms, we focus specifically on counteraction and propose a rigorous way to detect its presence. We highlight how finding evidence of counteraction can be understood as a form of mediation analysis and illustrate how the approaches taken in the existing literature can be formulated as instrumental variables (IV) designs. In attempting to identify the effect of a variable targeted by an intervention on an unintended spillover quantity, the intervention is essentially assumed to act as an instrument, affecting the spillover only through its effect on the targeted outcome. Such a relationship is often implausible and so our proposed method provides a sensitivity analysis to relax this implicit assumption.Footnote 3 The goal is to decompose the observed effect of the intervention (for example, a treaty commitment, an advocacy effort) into indirect and direct components (the former flow through the change in the intended target quantity, while direct components are unintended by the intervener, and might include a range of reactions or trade-offs). This sensitivity analysis holds fixed the direct effect at a range of feasible values and provides estimates of the implied indirect effects. We apply this approach to two prior studies that warn of counteraction to illustrate what this method can reveal when the evidence for counteraction is weak to non-existent. Although our substantive focus here is on human rights, our approach applies to other issue areas as well. The broader purpose is to strengthen causal inference about specific mechanisms through which spillovers might occur.
Human Rights and Unintended Consequences
International efforts to promote human rights have been said to “produce” harm in several ways. The research can be distilled to three mechanisms: backlash, trade-offs, and counteraction (Table 1).
Table 1. The logic of three spillovers: backlash, trade-offs, and counteraction

Note: M = targeted violation; Y = spillover effect
Backlash is often said to be a consequence of external norms and rights advocacy. Backlash refers to a strong, negative, public, and often angry societal reaction to political or social change. It is a social reaction rather than an individual response or a calculated policy decision,Footnote 4 and it may worsen other rights, or lead to entrenchment or regress on the targeted right.Footnote 5 For example, in some societies, external pressure to promote women's rights could lead to a doubling down on more conservative social demands by opponents of such rights, and eventually even alter policies. In contrast to trade-offs and counteraction (discussed later), backlash tends to be public and defiant. Backlash can be and often is instigated at the top by power holders who are in some way threatened by the assertion of a rights claim and thus are motivated to stir up opposition to particular human rights practices or to foreign influence in general.Footnote 6 It can be a direct response to criticism (for example, nationalist sentiments in response to foreign shaming)Footnote 7 or it can be indirect (e.g., operate through a rights improvement which in turn provokes social or political opposition). While backlash can describe any form of opposition—some scholars describe “liberal” backlash against rights violations, for exampleFootnote 8—we understand it as reasonably broad-based countermobilization against international human rights law, advocacy, or practice, whether or not rights improvements are realized on the ground.Footnote 9
Theorists of social movements see backlash as a common though hardly an inevitable occurrence in response to challenges to existing power structures or norms.Footnote 10 Some go further to claim a causal chain of events that runs from international human rights law/advocacy, to domestic anti-government mobilization,Footnote 11 which then triggers backlash, which in turn contributes to worsening repression. The exact consequences are not always clear, but Hopgood, Snyder, and Vinjamuri claim that backlash may be associated with “pernicious, longer-term effects that may lock in regressive practices,”Footnote 12 including strategically altering laws and institutions that are more likely to violate rights than to protect them. This is an important claim that runs in the opposite direction of much research claiming that law and advocacy helps rights claimants to mobilize for human rights, with generally positive consequences.Footnote 13 Importantly, however, such claims need to be tested with carefully specified models; if the claim is that human rights law and advocacy have caused backlash, then it is critically important to show that any regression in rights is not simply a symptom of broader authoritarian trends.Footnote 14
Tradeoffs. A second genre of critique is that the international human rights regime promotes specific legal rights at the expense of other human values. The claim is constructed around a notional resource constraint that implies that effort dedicated to human rights necessarily reduces resources available for other desirable ends. There are several variations on the trade-offs theme. Interventions that press for human rights could unintentionally divert funds from development projects;Footnote 15 they could increase attention to negative rights, while distracting attention from economic and social rights;Footnote 16 they could denigrate human needs that cannot be formulated in rights terms at all;Footnote 17 they could favor one protected category of people at the expense of others,Footnote 18 and so forth. Eric Posner, perhaps the bluntest proponent of this argument, asserts that “human rights obligations interfere with welfare-promoting activities of the government, and these welfare-promoting activities should be given priority.”Footnote 19
These arguments all assume that a human rights trade-off occurs when power holders improve their policies in the area the intervention targets, but these improvements worsen nontargeted values, such as economic growth, justice, or equality. The choice to respond to the external intervention is strategic, but the result (better rights leading to poorer growth, worsening equality, or shoddy social justice) is undesired and unintended by both the pro-rights intervenors and likely even the targeted respondent.
Logically, for a trade-off to exist, the targeted rights and the outcome would have to be strict substitutes and not complements.Footnote 20 That is, we would have to believe that the prioritized rights such as fair trials, women's political equality, freedom of conscience, or a child's right to health care do not themselves make a positive net contribution to other aspects of human welfare, such as economic development, tolerance, or justice. “Foregrounding” arguments—the idea that advocating right M causally reduces attention and therefore attainment of right Y—have a similar trade-off structure, though typically they are made in cognitive rather than strict budgetary terms. Elizabeth Hurd argues, for example, that protecting religious freedom renders groups other than Christians, Hindus, and Jews “inaudible.”Footnote 21 Moreover, when religious freedom is a priority, “violations of human dignity that fail to register as religious infringements languish beneath the threshold of national and international recognition as the international community dedicates limited resources to rescuing persecuted religionists.”Footnote 22 For Posner, advocating human rights competes with human welfare; for Hurd, advocating religious rights competes with the rights of women, racial minorities, and the disabled. For these claims to hold, there must be a negative effect of a targeted right on a “spillover” outcome; otherwise, there is no reason to believe that a causal resource trade-off, whether tangible or cognitive, exists. So far, we have seen little systematic evidence of these claims, and none that would pass traditional tests for causality.Footnote 23 And yet, such claims easily morph into causal claims and, even more dangerously, claims that rights advocates are inflicting real harm.Footnote 24
Finally, we define a particular type of harm that we call counteraction, our focus in the rest of this article. Assuming an intervention seeks to spur improvements in human rights, counteraction occurs when power holders respond to the intervention by improving their behavior for the specific rights the intervention targets but then offset these improvements by increasing other types of misconduct. It is a strategic response by a government to circumvent international pressure to improve its conduct in a particular issue area. Like trade-offs that may be made under budgetary or cognitive constraints, counteraction spurs improvements in the targeted area M but reduces rights in the spillover areas Y. It differs from interventions that merely displace the same or nearly identical type of misconduct by simply moving it to a different time or place, thus rendering the intervention (merely) ineffective in changing the net effect on the targeted behavior.Footnote 25 Instead, counteraction involves shifts between different types of misconduct so that the intervention may cause new types of problems or increase the severity of another set of problems. It is fundamentally a causal hypothesis about the effect that changes in one form of behavior have on another area. It inherently involves first a rights improvement, the consequences of which government agents seek to reverse with alternative forms of repression. In contrast to backlash, which is a social reaction and can be either the direct response to shaming alone or a response to targeted rights improvements that social forces then lash back against, counteraction suggests that protecting targeted rights causes compensatory strategic repression in another area and may, depending on how one weighs the costs and benefits, do more harm than good.
One of the most in-depth discussions of counteraction is the qualitative study of torture by Darius Rejali who argues that high-quality human rights monitoring has led to innovative forms of stealth torture, even (or especially) in democracies.Footnote 26 Quantitative studies have also sought to document counteraction.Footnote 27 For example, Hafner-Burton claims that international shaming improves political rights, but that it also “often” correlates with more political terror,Footnote 28 and suggests that one possible explanation is that “governments are strategically using some violations to offset other improvements they make in response to international pressure to stop violations.”Footnote 29 Similarly, Conrad and McDermitt find that when the United Nations shames countries specifically for torture, political rights deteriorate.Footnote 30 Likewise, Lupu notes that countries that ratify the International Covenant on Civil and Political Rights (ICCPR) improve political rights but have more victims of disappearances. He cautiously suggests that “if the cost of using certain types of repressive techniques increases, governments may become more likely to use other, less costly options.”Footnote 31
Given their appeal to governments who want to maintain power by hook or by crook, such claims seem plausible and perhaps even intuitive. However, there are also reasons that the appearance of these various spillovers may be deceptive. Despite its theoretical appeal, counteraction may not be the most plausible—and certainly not the only—mechanism for explaining an observed correlation between a given behavior and a particular international (or for that matter, domestic) intervention.Footnote 32
All three mechanisms through which scholars make claims that international human rights interventions have had negative consequences have the same evidentiary burdens as any other causal claim. Such claims go well beyond skepticism that such interventions are merely ineffective. That human rights interventions have not eliminated rights abuses is hardly news, and we accept that it is the burden of the so-called optimists to demonstrate any improvements. But if backlash, trade-offs, and counteraction are claimed to worsen rights, then such claims are subject to similar demands that causality be scrutinized, possible spuriousness be taken seriously, and selection problems be considered.
Methodological Focus: Modeling Counteraction
The Problem: Modeling the Hypothesized Mechanism
Counteraction deserves attention because researchers have attempted to demonstrate its negative consequences. Yet the causal minefield is evident and there are several reasons to be skeptical about broad counteraction claims. The first issue is traditional selection effects. Arendt noticed well before there was much international shaming that dictators facing challenges to their rule often increase repression or torture.Footnote 33 Considering the obvious selection effects should give scholars pause before jumping to conclusions about law and advocacy.Footnote 34 Repressive governments behave badly in multiple areas; their patterns of rights violations tend to look more like complements than substitutes.Footnote 35 Interventions such as shaming typically target countries with worsening rights—a classic selection effect. Thus, although Conrad and DeMeritt argue that “when a state is put on notice for torture, it responds by securing its own grasp on power, limiting empowerment rights,”Footnote 36 they cannot rule out the possibility that the UN may simply shame countries with more, and possibly worsening torture,Footnote 37 rather than cause more torture.
A further methodological problem that may lead us to question broad counteraction claims is that so far scholars have used separate models to test separately for correlations between the intervention and each outcome. For example, Lupu's finding that ratification of the ICCPR correlates with improved political rights and with increased victims of disappearances is based on two separate models that do not connect these correlations to one another. Contrary to his cautiously worded conclusion,Footnote 38 this alone cannot demonstrate substitution. A difference in the intervention's effect on the targeted outcome and its effect on the substituted outcome implies only that there may be some combination of backlash, counteraction, trade-off, or simple spuriousness. Decomposing the underlying mechanisms requires additional assumptions.
Why should we be skeptical? First, the intervention may affect one outcome in one subset of observations but affect the other outcome in another subset of observations. Since effects are identified as averages only, we cannot immediately conclude that these patterns reflect substitution at the level of the individual state. Second, the assumption that governments have a “menu of manipulation,”Footnote 39 and can easily shift to new techniques of repression does not hold since repressors are already likely to choose the most efficient means of control.Footnote 40 Third, and crucial for our method here, the effects of the intervention alone are not the quantity of interest for assessing counteraction. Counteraction implies a causal pathway linking the intervention, the targeted outcome, and the spillover. Thus, the targeted outcome must affect the spillover. Separate models thus suffice if and only if we assume counteraction is the only possible causal mechanism. This restrictive assumption is unlikely to hold in any real-world situation. It also effectively assumes away what a researcher is obligated to examine: how, exactly, do better rights demands make things worse? Under what conditions can we expect such causal deterioration? Causal mechanisms must therefore be scrutinized, which is a more difficult task than to provide evidence for overall correlations.Footnote 41
A final reason to be skeptical of broad counteraction claims is that case studies often underscore the importance of heterogeneous contexts. Kuperman's findings that rhetorical support for human rights can backfire in civil war settings with heavily armed and revolutionary rebel groups is a highly conditional argument.Footnote 42 So are the scope conditions for Risse and Sikkink's “spiral model,” which requires a combination of transnational advocates, active domestic human rights organizations, and foreign government support to produce internalization of human rights norms.Footnote 43 Switching between strategies in response to external norms or monitoring is likely highly constrained—and certainly not similar—for such heterogenous cases.
Formalizing Counteraction Effects
We propose a method for assessing the plausibility of counteraction when we observe an intervention's contrasting effects on two outcomes.Footnote 44 We apply this approach to evaluate counteraction claims made by two influential studies. To start, we review the framework for causal mediation analysis developed in Robins and Greenland and Pearl and introduced to political science by Imai, Keele, Tingley, and Yamamoto.Footnote 45 The causal quantities of interest are formalized using the conventional Neyman-Rubin potential outcomes framework.Footnote 46 Assume a total of N units, each indexed by i. Let Y i be the observed spillover or offsetting outcome of interest, M i be the observed outcome targeted by the intervention, and A i be the observed level of the intervention. For simplicity, we focus on the case of a binary A i and binary M i but our approach can be extended to continuous variables with additional modeling assumptions. Define Y i(a) as the potential offsetting outcome that we would observe if unit i were assigned to take on an intervention value of A i = a. The intervention's total effect for unit i is the difference τ i = Y i(1) − Y i(0).
Causal mediation methods focus on different ways to decompose the total treatment effect into a “direct” component and an “indirect” component attributable to some mediator M i. To motivate these decompositions, we need to define additional counterfactual outcomes for both Y i and M i, following standard practice in the mediation literature. First, we define M i(a) as the targeted outcome we would observe if unit i were assigned intervention A i = a. Then, we define a joint potential outcome, Y i(a, m), which denotes the potential offsetting outcome we would observe if unit i were assigned A i = a, M i = m. We further make the “composition” assumption that Y i(a, M i(a)) = Y i(a).Footnote 47 Using this framework, we can define additional counterfactual comparisons that permit the decomposition of the overall treatment effect.Footnote 48 The indirect or “causal mediation effect” for unit i, holding fixed the intervention at some level a is defined as

In other words, the indirect effect is the difference in potential outcomes when a unit is assigned the mediator value it would have under treatment compared to when that unit is assigned the mediator it would have under control.
Conversely, the direct effect represents the change in the potential outcome for Y i if the treatment were manipulated while the mediator is held constant at the level it would take under either treatment or control, M i(a).

The total effect τ for individual i can be written as the sum τ i = δ i(a) + ζ i(1 − a) for a = 0, 1. In practice, researchers cannot directly estimate individual treatment effects and instead focus on decomposing the average treatment effect $\bar{\tau }\;$into average direct and indirect effects, denoted
$\bar{\zeta }\lpar a \rpar \;$and
$\bar{\delta }\lpar a \rpar $.
Figure 1 illustrates the connection between the causal pathways linking A, M, and Y and the three mechanisms discussed earlier.Footnote 49 It shows three possible causal arrows: (1) from A to M, (2) from M to Y, and (3) from A to Y. The combination of paths 1 and 2 reflects the indirect effect of the intervention that flows through the targeted outcome. Path 1 reflects the effect of the intervention on altering the targeted outcome and path 2 the corresponding effect of that change in the targeted outcome on the spillover. Conversely, path 3 reflects the direct effect unmediated by M. Counteraction exists when (1) is positive and (2) is negative, implying an overall negative indirect effect of A on Y through M.

Figure 1. Graphical representation of causal pathways
Trade-offs and rights-mediated backlash operate through an analogous mechanism. Direct backlash is captured in arrow (3), which denotes an effect of A on Y that works entirely through a non-M mechanism. Notably, for a fixed overall effect of A on Y, we can understand counteraction and direct backlash as competing explanations for the same phenomenon. The plausibility of counteraction as an explanation for A's negative effect on Y is strengthened when the direct effect (3) is either 0 or in a countervailing direction (positive). This intuition, which underpins many of the arguments for counteraction effects, motivates our proposed sensitivity analysis for assessing the indirect counteraction effect.
The path diagram approach to causal mediation in the social sciences has its roots in the structural modeling framework introduced by Baron and Kenny.Footnote 50 This approach assumes a set of simultaneous equation models for the outcome and mediator:



where $ \varepsilon _{i1}\comma\ \varepsilon _{i2}\comma\ \varepsilon _{i3} $ are mean zero-error terms, and X i denotes a vector of pre-treatment covariates.
In this framework, β 2 denotes the average effect of the treatment on the mediator, γ the mediator's effect on the outcome, and β 3 the treatment's effect on the outcome holding constant the mediator. Researchers have often interpreted the product β 2γ as the effect of A on M mediated by Y.Footnote 51 Imai and colleagues provide a formal justification for this estimator in the context of the potential outcomes framework and give the necessary assumptions required to interpret β 2γ as identifying the average causal mediation effect. In addition to correctly specified outcome and mediator models, identifying the causal mediation effect using the product of coefficients requires a sequential ignorability assumption. This consists of two parts: first, that the intervention is ignorable or assigned “as-if-random” conditional on pre-treatment covariates; second, that the mediator is ignorable conditional on covariates and treatment. This requires ruling out the presence of any confounders of the mediator and outcome that might be affected by treatment. While identifying controlled direct effects requires weaker assumptionsFootnote 52—permitting the presence of observed post-treatment confounders—it still requires researchers to justify two ignorability assumptions: one for the intervention and another for the targeted outcome. While Imai and colleagues develop a sensitivity analysis framework to relax the second part of sequential ignorability by allowing researchers to specify the degree of unobserved confounding of M and Y via the correlation between ε i2 and ε i3, we note that it is often difficult for researchers to develop reasonable beliefs about the magnitude of this unobserved confounder and thereby interpret a sensitivity analysis.
We develop an alternative strategy that does not use sequential ignorability and builds on the approach already taken by researchers studying counteraction—using the estimated effects of the intervention on the two outcomes. While we have pointed out that such an approach alone does not directly assess the presence of counteraction, it does provide the basis for a sensitivity analysis.
Connecting the “Two Effects” Strategy to Instrumental Variables
Among papers examining counteraction or trade-offs, it is extremely common to see researchers arguing for substitution based on an observed positive association between treatment A and targeted outcome M and an observed negative association between treatment A and offsetting outcome Y.Footnote 53 Our method starts from the necessary assumptions made to estimate these treatment effects: namely that there are no unobserved pretreatment confounders of A and M and A and Y. Formally, researchers assume:

What can those two effects tell us about the effect of M on Y? As Imai and colleagues illustrated, randomization of A does not suffice to identify the direct and indirect effects since it does not guarantee unconfoundedness of Y i(a, M i(a)) and M i(a). Thus, randomization of A doesn't guarantee there is no other omitted variable causing both the mediator and the outcome. Using an auxiliary variable to assess counteraction is structurally very similar to the well-studied problem of estimating effects via instrumental variables (IV). In this case, A acts as an instrument for M.Footnote 54 As in IV estimation, the researcher's goal is to compare correlations between the instrument and the two other variables to infer something about their causal relationship. Angrist, Imbens, and Rubin formalize the IV assumptions in the potential outcomes framework and show that for the case of a binary A and a binary M when A (1) is exogenous, (2) has a non-zero and monotonic effect on M, and (3) has no direct individual causal effect on Y that is unmediated by M (“exclusion restriction”), the treatment effect of M on Y is identified using the ratio of the effect of A on Y and the effect of A on M—the classic Wald ratio estimator.Footnote 55 As the appendix shows, under these assumptions, the IV estimator identifies M's direct effect on Y for the subgroup for which A has an effect on M (the “complier” group). Additionally, researchers can incorporate covariates by assuming the linear structural models from the previous section and estimating γ via two-stage least squares, even in the presence of an unobserved confounder of M and Y
What additional assumptions are needed to apply the IV framework to mediation analysis? We have already assumed (1) by virtue of assuming the (conditional) ignorability of treatment A. Likewise, (2) can be partially tested from the data and rejected in the absence of a strong first-stage effect of A on M. Assumption (3) is the necessary third element and is particularly questionable in the counteraction setting because it would imply that the intervention's direct effect on the offsetting outcome would be 0 for all units, regardless of the targeted outcome's level. Indeed, a strict exclusion restriction assumption would rule out all possible mechanisms by which A affects Y besides counteraction, effectively assuming what researchers set out to examine.
While researchers are unlikely to believe the exclusion restriction holds exactly with respect to the treatment A, they may nevertheless have beliefs about the size and direction of A's direct effect on Y. A sensible question to ask is: “Given varying beliefs about the exclusion restriction violation, do the observed effects of A on M and A on Y still support a counteraction story?” We suggest that applied researchers may still find the IV strategy useful for assessing counteraction when combined with a formal sensitivity analysis for the exclusion restriction. Our proposed approach resembles existing methods for conducting formal sensitivity analyses for the assumption of “no omitted variables” in observational studies.Footnote 56 These approaches assess a given causal effect's robustness by re-estimating the result under varying levels of assumed omitted variable bias. Findings that remain statistically significant even in the presence of a large potential omitted variable bias are considered robust to violations of the assumption. Our approach adopts the same linear structural equation framework as the sensitivity analysis for sequential ignorability proposed by Imai and colleagues, but manipulates a different sensitivity parameter. Instead of assessing the magnitude of omitted variable bias confounding M and Y that would break a result, it instead examines the magnitude of the direct effect of A on Y that would break the IV estimate of the effect of M on Y.
The particular approach to sensitivity analysis we propose here is similar to that proposed by Conley, Hansen, and RossiFootnote 57 for general violations of “exogeneity” in IV. To briefly describe our approach, suppose that we knew the true direct effect of A on Y for each unit. We could then subtract this effect from the observed outcome to generate a new outcome Y* which the exclusion restriction holds for by definition. Applying the standard instrumental variables analysis to the transformed outcome would then give a valid estimate for the counteraction effect under the assumed violation. Repeatedly carrying out this process for a range of feasible direct effects provides a set of possible estimates for the counteraction effect. Under the linear structural equation framework, we can estimate the effect of the mediator using a two-stage least squares (2SLS) estimator.Footnote 58 This commonly used approach to estimating IV effects allows researchers to parsimoniously incorporate covariates in addition to handling situations where A is not necessarily binary. While this approach does make some additional assumptions regarding effect homogeneity and correct model specification, these assumptions are similar to what researchers are already assuming to estimate the effects of policy interventions using linear regression.Footnote 59 Existing sensitivity analyses for mediation also rely on the linear structural equation framework for simplicity.Footnote 60 We discuss the assumptions in greater detail in the appendix.
The “first-stage” linear regression assumes the following model for Mi:

where X i denotes a vector of pretreatment covariates, $\xi _2^{\prime} $ a vector of parameters, and ε i2 is a mean zero-error term with finite variance. The parameter β 2 denotes the average effect of A on M. Under conditional ignorability of treatment, this can be estimated consistently via OLS since E[ε i2|A i, X i] = 0.Footnote 61 We assume another model for the outcome Y i:

Note that A i does not appear in this model, consistent with the exclusion restriction. After estimating the first-stage regression via OLS, the fitted values $\widehat{{M_i}}$ are plugged into a second regression with Y i as the outcome along with the same covariates X i from the first stage. This yields a consistent estimate of the coefficient γ even when sequential ignorability does not hold and E[ε i3|A i, M i, X i] does not equal 0—that is, the outcome and mediator model error terms are correlated.
As discussed, the exclusion of A i from the outcome model is an implausible assumption in most empirical settings. To implement our sensitivity analysis, we assume the following outcome model:

and assume that ρ is a known constant parameter. This corresponds to a model where, in addition to its effect on M i, A i also has a constant direct effect on Y i equal to ρ. This allows us to define an augmented outcome Y* for which the exclusion restriction holds by definition.

For a reasonable set of values of ρ, we propose researchers estimate the IV effect of M on Y* and plot the resulting point estimates and confidence intervals against each choice of ρ. While we are assuming a constant ρ—and by extension a constant treatment effect—this is not an absolute requirement. In principle, a researcher could allow ρ to vary across units, though visualizing and interpreting the analysis with more than two sensitivity parameters becomes rather challenging. In the appendix, we go further into the interpretation of ρ and its relation to sensitivity parameters used in other mediation sensitivity analyses.
How should researchers choose what values of ρ are feasible? By definition, ρ itself depends on the scale of Y. We propose two approaches to assessing what would be considered a “reasonable” direct effect. The first is to standardize ρ and compare against common benchmarks. A variety of possible standardizations exist—we consider a frequently used approach for standardizing effects of binary variables: Cohen's d. This quantity is defined as the unstandardized effect divided by the pooled standard deviation of the outcome Yi, denoted s.

Transforming ρ to d allows us to evaluate the posited direct effects on a common scale—in terms of standard deviations of the outcome.Footnote 62 Cohen provides a set of benchmarks for determining what constitutes a “small,” “medium,” and “large” effect which can be combined with theoretical expectations regarding the direct effect of Y i to determine the plausibility of each IV estimate.
As an alternative to using these generic benchmarks, which may not necessarily represent what is considered a “large” or “small” effect in the particular case being studied, we also suggest benchmarking against the estimated effects of Ai. This is similar in spirit to the strategy Imbens used for assessing the sensitivity of causal estimates to unobserved confounding which compares the amount of confounding that is found to break the finding to other known effects of variables in the data.Footnote 63 The estimated effect of Ai on Mi provides one reasonable benchmark because, in the case of human rights violations, researchers typically have some theoretical beliefs about what types of rights the policy intervention is likely to affect the most. It is often the case that a researcher believes that any effect of Ai will be largest for the targeted outcome and any direct effect on the spillover outcomes is unlikely to be any larger. Given these beliefs, a researcher would constrain the range of the sensitivity parameter d to be no larger than the standardized effect of Ai on Mi.
Examples: International Treaties, Norms, and Shaming
We apply our framework to two of the studies that make counteraction claims with respect to human rights: Hafner-Burton and Lupu.Footnote 64 Hafner-Burton has been well-cited for arguments about the negative consequences of international interventions.Footnote 65 Both studies, in whole or in part, use country-year measures of respect for rights drawn from the Cingranelli and Richards (CIRI) data set. Hafner-Burton evaluates the effect of international naming and shaming by nongovernmental organizations (NGOs), international organizations such as the UN, and the news media on measures of human rights, while Lupu estimates the effect of ICCPR ratification on the extent to which governments protect both civil rights (e.g., speech, association, assembly, religion) and physical integrity rights. In both studies, the presence of a positive correlation between an intervention and a country's respect for one set of rights and a second, negative correlation between that intervention and respect for another set of rights is taken as evidence for the counteraction hypothesis. We show that in both cases, the evidence is insufficient to support counteraction.
In replicating Hafner-Burton, we find no strong or statistically significant effect of NGO shaming on political rights and only weak evidence for an adverse effect on physical integrity rights. In this case, the argument for counteraction fails the first necessary criterion for counteraction: that the policy intervention affects the targeted outcome. Even if one is to accept the presence of the effect on physical integrity rights, the absence of any corresponding change in political rights directly contradicts the hypothesis that states are “trading off” rights. In the case of Lupu, we do find some evidence of the primary effect on the targeted outcome—ratification improves some measures of civil rights. However, illustrating the usefulness of the sensitivity analysis, in applying it, we find that the true, unmediated effect of ICCPR ratification on physical integrity rights would have to be much larger than is theoretically plausible to sustain the counteraction argument.
First, we turn to Hafner-Burton's analysis, which considers the effects that international naming and shaming campaigns have on governments’ respect for civil liberties and for personal integrity rights. The article's strongest results are for the effect of naming and shaming by an NGO—Amnesty International (AI)—on abuses of physical integrity rights. We focus specifically on replicating the country-year OLS results presented in Hafner-Burton's Table 2 with a few important modifications.
Hafner-Burton measures physical integrity rights violations using a composite index of repression obtained by adding together the CIRI scores for the four physical integrity measures: killing, torture, imprisonment, and disappearances. This yields a variable that ranges from 0 (no violations on any of the four measures) to 8 (worst scores on all four measures). This is then regressed using OLS on a measure of NGO advocacy and shaming obtained by summing the number of press releases and background reports that AI issued for a given country in a given year and then taking the log, rescaling the measure to have mean 0.Footnote 66 The regression models also include indicators for whether a state has signed the Convention Against Torture and the ICCPR respectively, a measure of democracy that coarsens the conventional Polity IV scale into a binary indicator based on whether the score is above or below 6, the logged GDP per capita in a given country year, the log of population, and whether the country is experiencing a civil war or an interstate war. All of these regressors are lagged by one year. In addition to these covariates, the models include lags of the outcome variable. For the physical integrity rights outcome, one-, two-, and three-period lags of the outcome are included as regressors along with time fixed effects. Hafner-Burton then regresses a measure of political rights using the same model specification except with only the one-period lagged outcome included as a regressor. The political rights measure comes from ratings done by Freedom House and ranges from 0 (no violations) to 6 (extreme repression).
While the results presented in this regression suggest AI shaming has the effect of worsening repression, they do not, in the same regression, present evidence that shaming also has a statistically significant positive effect on political rights. Rather, the argument for counteraction appears to come from the combination of different regressions on different time spans, with different identification strategies. Some of these analyses show an effect on one form of rights and some on another.Footnote 67 Even when using similar selection-on-observables identification strategies, the effects presented are identified on two different subsamples: Freedom House scores are available as far back as 1972 for some states while the CIRI scores start in the early 1980s. Combining these results to infer counteraction is problematic not only because they do not by themselves imply the existence of a counteraction effect, but they may also not even imply the existence of both effects for a common set of states. Effect heterogeneity, both over time and across units means that at a minimum, data-driven arguments for substitution or trade-off need to show effects on a common population.
We replicated these results with a few adjustments and corrections to the original data set. First, we estimated the effects on both outcomes only for the period for which data on both political and physical integrity rights (including the required lags) were available: 1984 to 2001. Second, we addressed an issue in the original data in which numerous observations were missing data on population. This gave us a slightly larger time-series cross-sectional data set than the one presented in the original paper (2,173 versus 1,828 country-years) and corrected for the possibility that these were not missing at random. Third, we rescaled the logged shaming measure so that it had a standard deviation of 1 to help aid in interpreting the regression coefficients. Finally, in addition to estimating the original specifications, we estimated two specifications for the physical integrity rights measure with fewer lagged outcome variables. Figure 1 presents the estimated effect of a one-standard-deviation increase in the AI shaming measure for all four models.
We find a slight but statistically insignificant effect of NGO shaming on improved political rights. Additionally, if we accept Hafner-Burton's original identification strategy and use three lags of the outcome in the regression, we fail to find a statistically significant effect of shaming on the physical integrity itself. Only when we drop all but a single lag do we recover the original, statistically significant finding. However, this is likely driven by omitted variable bias if we believe that Amnesty International's propensity to shame a given country is influenced by multi-year trends in abuses over time.
Even if we accept that identification is credible given a single period lag, the results would be enough to show an effect on only physical integrity rights and not counteraction between political and physical integrity rights. This is because, as we set out, showing the former using the dual correlation method requires a first-stage effect on the targeted outcome. This is both a theoretical and practical concern—if the policy is doing nothing to alter the targeted outcome then there exists no constraint in the way that the counteraction hypothesis would suggest. Practically, credible inference on treatment effects via instrumental variables techniques requires a strong first-stage effect. When instruments are “weak,” point estimates and standard errors based on asymptotic approximations, as in the case of 2SLS, are biased and have particularly poor performance.Footnote 68 This can be seen intuitively from the form of the 2SLS estimator in the single instrument/single endogenous variable case, which is a ratio of two OLS coefficients. When the denominator is close to 0 (no effect of A on M), the resulting 2SLS estimate will be extremely large and extremely variable. We therefore omit presenting a sensitivity analysis of the Hafner-Burton result since the results fail to show even the minimum necessary conditions for counteraction to be plausible and, indeed, any sensitivity analysis in this case is likely to be both extremely high-variance and potentially misleading.
To demonstrate counteraction through the dual-regression method, researchers must establish that, at minimum, the policy intervention affects the targeted outcome of interest. Without this first step, any counteraction is entirely speculative. Given what we find in replicating Hafner-Burton's main regression, we cannot conclude that the results provide evidence that “governments continue or expand their use of political terror … to cancel out other improvements governments make but do not want to work.”Footnote 69 The replication cannot establish any initial improvement to counteract and the results showing expansion of political terror are themselves questionable given what we expect regarding omitted variable bias. In sum, we find no support for the article's claims about counteraction.
Next, we turn to Lupu, who primarily focuses on assessing the extent to which the effect of international human rights treaties depends on domestic courts’ ability to credibly enforce those rights. He theorizes that civil rights violations are easier to prosecute compared to violations of physical integrity rights (e.g., torture) as a result of variation in the costs of producing evidence. Because governments’ abuses of physical integrity rights are much harder to document, courts will be much less able to force governments to abide by international legal commitments with respect to those rights. The theory predicts that ICCPR ratification will improve governments’ performance on indicators of civil rights (e.g., freedom of speech, association, etc.) while having no effect on performance for indicators of physical integrity rights. Rights measures are taken from the CIRI data set and take on values from 0 to 2 with 0 denoting frequent/severe violations, 1 denoting occasional violations, and 2 denoting no reported violations.
Using a series of ordinal probit regression models estimated on a country-year data set, Lupu finds a positive and statistically significant association between ICCPR ratification and three indicators of civil and political rights from the CIRI data set: freedom of association, freedom of speech, and religious freedom. He finds no statistically significant association between ICCPR ratification and three out of the four physical integrity variables (killings, torture, and imprisonment) but does find that ICCPR ratifiers have worse CIRI ratings on disappearances. The regression models control for measures of judicial independence, democracy (as measured by the Polity IV scale), regime durability, civil war, external war, logged GDP per capita, log population, and a measure of the number of international NGOs of which the state is a member. The models also control for the one-year lag of the outcome and include fixed effects for each year.
With the surprising finding of a negative effect for disappearance, Lupu suggests that counteraction may be to blame. While describing the claim as “tentative” he posits that “because ICCPR commitment constrains governments’ ability to restrict the freedoms of speech, association, assembly, and religion, they may turn to harsher methods, for which evidence is less costly to hide, to accomplish what they no longer can with less egregious human rights violations.”Footnote 70 This is clearly a claim about counteraction, in that the constraint placed on civil and political rights is hypothesized to directly influence the expanded use of disappearance.
We replicate these four regressions, fitting ordinary least squares regressions of the CIRI score on the same linear additive models proposed in the original paper.Footnote 71 We also make a few slight corrections to the data to obtain a larger sample size (2,155 versus 1,966 as reported in the data). Figure 3 presents our estimated effects of ICCPR ratification on each CIRI score. We replicate the originally reported pattern for measures of association and disappearances. If we assume that conventional significance levels are appropriate—an important normative question not considered here—we do not find a statistically significant effect (α = .05) on speech or religious freedom. On average, ICCPR ratifiers have a .06 higher score on association and a .05 lower CIRI score with respect to disappearances.
Implementing the Sensitivity Analysis
Our method stresses the importance of a sensitivity analysis to help interpret the observed effects and whether counteraction is a reasonable explanation. Given our finding, what can we say about the plausibility of counteraction between improvements in association rights and the worsening of disappearances? Figure 2 plots the sensitivity analysis for the 2SLS estimate of the counteraction effect for varying levels of the exclusion restriction violation. The light gray line denotes the naive estimate: what our estimated effect would be if we believed ICCPR ratification had no effect on disappearances aside from its effect on association rights. In this case, the effect is negative, consistent with counteraction, but imprecisely estimated and not statistically significant at any conventional rejection threshold (p = 0.177). Notably, although the two reduced-form estimates were statistically significant and in opposite directions, the implied estimate of the causal effect of one outcome on the other is not statistically significant. This highlights one possible drawback of our method—we give up some statistical power in exchange for more flexible assumptions. If we were to assume that there is no direct effect of ICCPR ratification on CIRI disappearance—in other words, assuming that counteraction or a trade-off is the only possible mechanism—then the estimated average effect of ICCPR ratification on CIRI disappearance would equal the indirect effect and the two-stage approach would be unnecessary unless the specific effect of association on disappearance scores was of interest. However, because we do not want to simply assert that counteraction is the only possible mechanism but instead assess the plausibility of an indirect effect for a variety of possible direct effects, we need to accept some reduction in power to properly conduct inference on the effect of the mediator. For reasonable sample sizes, we consider the reduction in power when d is close to 0 to be far outweighed by the risk of falsely concluding in favor of an indirect effect when there exists a strong direct effect. Since the goal of sensitivity analyses is to be conservative in drawing inferences, we consider the increase in estimation uncertainty to be a reasonable sacrifice.

Figure 2. Replication of main effects of NGO shaming from Hafner-Burton Reference Hafner-Burton2008
When we vary the assumed size of the direct effect of ICCPR ratification on CIRI disappearance score, we conclude that there is a small range of direct effects for which we would obtain a statistically significant (p < .05) and negative indirect effect of an increase in CIRI association scores on CIRI disappearance scores. Notably, if the direct effect is itself negative (the backlash argument), then the estimated indirect effect is either close to 0 or actually positive. This matches the intuition behind our sensitivity analysis—the presence of a negative direct effect explains away a counteraction or trade-off hypothesis. In a setting where counteraction is more plausible, we would see negative and statistically significant effects even when allowing for some amount of backlash. In the case of Lupu's study, this does not appear to be so.
Instead of asking how much of a negative direct effect is enough to “break” the result, for the Lupu analysis, we consider how much of a positive direct effect is enough to suggest some counteraction. In other words, do we think that the observed effect of the intervention on the spillover outcome is too small compared to what we would expect, implying some counteraction? In the Lupu data, we find that to reject the null hypothesis of no counteraction at α = .05, the direct effect of ICCPR ratification would have to be positive and equivalent to about .1 standard deviation of the outcome.
The sensitivity analysis is helpful because it helps probe the counteraction claim's plausibility. Importantly, counteraction becomes plausible only when our prior beliefs about the true effect of ICCPR ratification are particularly strong and we need to explain away the “surprise” of a smaller-than-expected finding. In the case of Lupu, we find that to reject the null hypothesis of no counteraction, the direct effect of ICCPR ratification would have to be positive and about .1 standard deviation of the outcome. While a .1 standard deviation effect may seem somewhat small, suggesting plausibility of counteraction, in this context, it is more sensible to compare to a known benchmark effect. The vertical dark gray line in Figure 4 denotes the estimated counteraction effect if we assume that the direct effect of ICCPR ratification on the disappearance score was of the same standardized size as ICCPR ratification's effect on the association rights score. Even if this were true, our analysis would fail to reject the null of no effect at α = .05.

Figure 3. Replication of main effects of ICCPR ratification from Lupu Reference Lupu2013
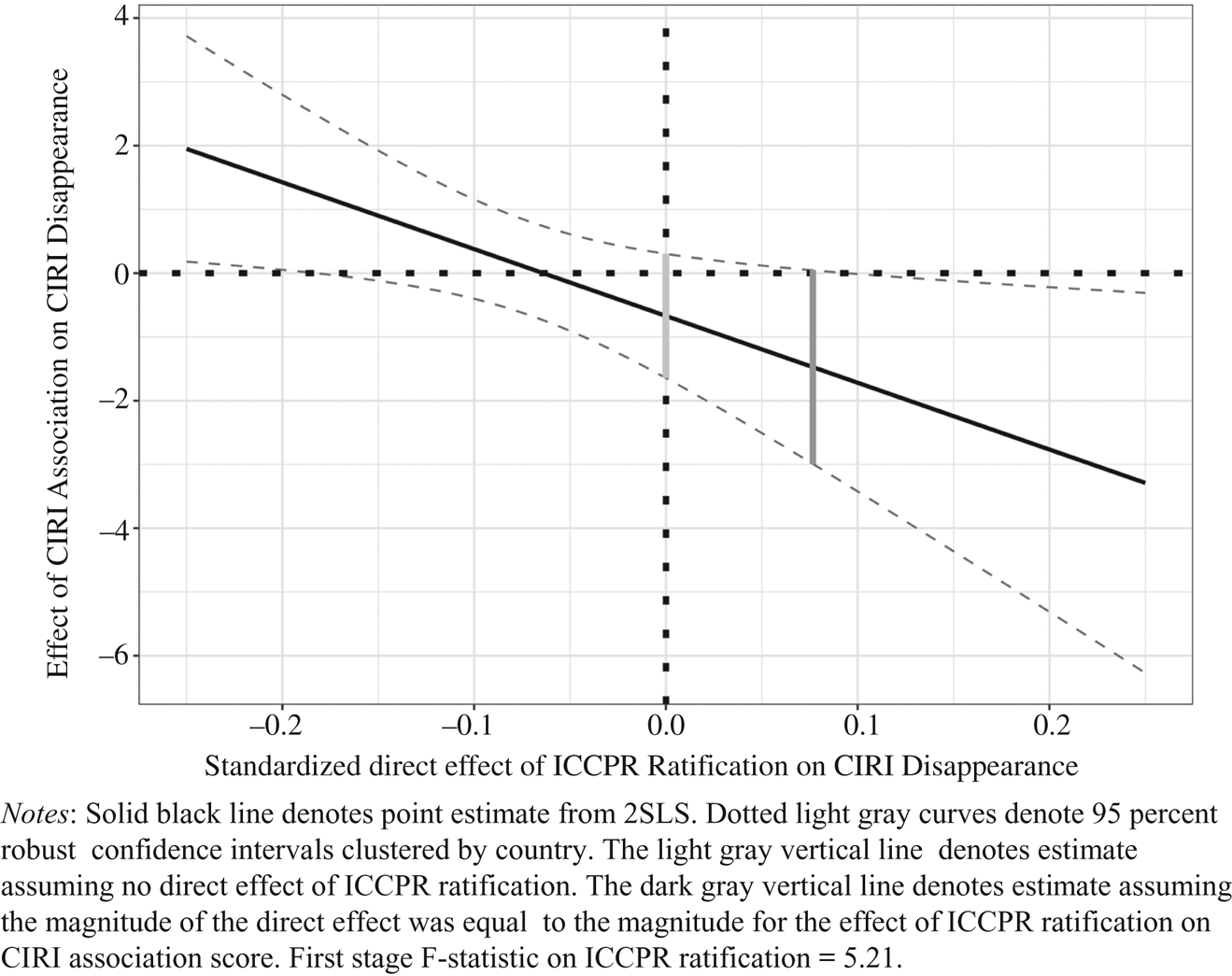
Figure 4. Sensitivity analysis for counteraction in Lupu Reference Lupu2013
We emphasize the importance of finding plausible bounding values for the direct effect when conducting the sensitivity analyses because it is always possible to find a range of direct effect parameter values that would yield both strong positive and strong negative indirect effect estimates. Analogous work on sensitivity analyses for the case of unobserved confounding has often proposed relying on relationships between observed quantities and the outcome of interest as “benchmarks” for determining what is a reasonable magnitude of unobserved confounding—for example, arguing that a result is robust to an unobserved confounder that is as strong as the strongest observed predictor of treatment and outcome.Footnote 72 However, we caution against using any single benchmarking approach that is not informed by substantive knowledge. As Cinelli and Hazlett argue, sensitivity analyses provide a basis for “principled argument” rather than an “automatic procedure” for assessing the underlying research design's correctness.Footnote 73 Therefore, our recommendation to begin with the “first-stage” effect of Ai on Mi as a benchmark for the direct effect of Ai on Mi should be understood as the first step in assessing plausible direct effect values and not the final one. A researcher's goal in selecting reasonable sensitivity parameters is to determine how large of an effect the intervention could have in general on any outcome and then assess how these effects would compare in size to the unobserved direct effect of Ai on the specific outcome Yi. Subject matter knowledge about the known effects of the intervention should guide researchers here.
If an intervention is known to have relatively weak effects on most relevant outcomes and Yi is not uniquely different from those outcomes, then that suggests values of the sensitivity parameter far away from 0 are unlikely. One reasonable approach to quantifying this may be to benchmark the direct effect of Ai on Yi using the largest known effect of Ai on any outcome. We recommend starting with the effect of Ai on Mi precisely because its identification is a prerequisite to the exercise of assessing counteraction and because researchers are likely to have existing beliefs about the relative size of this effect compared to others. However, estimating effects on other outcomes may be useful as well, with the caveat that the set of controls needed to identify the effect of Ai on Mi may not be the same set needed to identify the effect of the intervention on all relevant outcomes. The choice of reasonable bounding values will always involve a combination of quantitative knowledge about the general effects of the intervention with qualitative knowledge about how those effects compare with the unobserved direct effect on the outcome of interest.
We can illustrate this combination of quantitative and qualitative knowledge using Lupu's theoretical argument for counteraction. The domestic courts theory posits that because courts are more capable of enforcing civil rights compared to physical integrity rights as a result of differences in the availability of information, the effect of an international rights commitment will be larger for civil rights than for physical integrity rights. The direct effect of ICCPR ratification on personal integrity rights is therefore unlikely to be greater than the effect of ratification on civil rights, making the estimated effect of Ai on Mi a plausible extreme bound for the sensitivity parameter. Based on this benchmark, the most feasible values for ratification's direct effect will lie to the left of the vertical dark gray line in Figure 4, all of which imply no statistically significant counteraction effect. In other words, Lupu introduced a counteraction hypothesis to explain the appearance of an effect where he anticipated finding a null based on a theoretical argument that predicted a direct effect of ICCPR ratification close to zero—governments ratifying human rights treaties are unlikely to face significant litigation with respect to personal integrity rights compared to civil rights and therefore have fewer incentives for improvement. However, a formal analysis suggests that the gap between what was expected and what was observed does not provide much evidence for counteraction. The small but surprising negative effect on disappearances is not sufficiently surprising for us to conclude that counteraction is occurring.
Conclusion
We live in a world where, despite the best efforts of well-meaning advocates, human rights violations continue. The determination of some leaders to maintain power and privilege through repression has led to widespread speculation that human rights advocates may actually exacerbate rather than alleviate the problem.Footnote 74 Critics suggest that resources have been shifting to the hopeless quest of improving rights (at the expense of development, equality, or social justice); targeted governments and their populations are starting to lash back against international pressure; and clever despots have connived to replace open violations with stealth repression. These accounts allege that the international human rights project is worse than ineffective.
Such negative consequences are just as important to understand as any other effect, but they should be subject to the same evidentiary standards as the rest of social science. We have discussed at least three types mechanisms to explain negative spillovers of human right interventions—backlash, trade-offs, and counteraction—that scholars have recently offered as reasons to throttle back on international human rights efforts. These claims may be plausible; all merit serious attention. But only a few researchers provide systematic evidence for such claims. Even fewer provide evidence that support their causal arguments.
Unfortunately, evidence from the effects of interventions alone is insufficient to demonstrate the existence of a particular causal mechanism when the claimed effect is theorized to be indirect or mediated. As a first-order concern, inference on the overall effects of interventions is extremely challenging because interventions target problem-ridden countries, generating a significant degree of potential omitted variable bias. However, even if we grant that researchers have credibly identified the first-order effects of these interventions, their leap to making conclusions about the second-order effects and their underlying mechanisms requires additional assumptions regarding the underlying causal structure that may not be credible.
We have outlined three mechanisms by which interventions to improve human rights may have negative spillover effects on other outcomes. Counteraction is one such mechanism which we carefully define as a change in state behavior such that either the incidence or level of a spillover outcome increases in a way that is attributable to the change in the targeted outcome driven by the intervention. We connect this to the concept of a mediation or indirect effect in the causal inference literature and note the importance of showing a strong first-order effect of the intervention as a prerequisite to any theorizing about counteraction mechanisms. Unless the intervention has, ceteris paribus, some positive consequences, there is nothing to counter and there is no mediation.
This is not to claim that governments who sign human rights treaties or experience harsh external criticisms do not (often, perhaps) do very bad things. But the counteraction theory requires a demonstration both that the external treatment caused bad results, and that the causal mechanism driving this effect is an improvement in the targeted area of rights. In much of the literature, there is very little scrutiny given to such causal claims, even though such mechanisms are harder to assess empirically than “black box” correlations.Footnote 75
If researchers are going to use quantitative data to assess mechanisms like counteraction, they need to model it explicitly. We have presented a method for assessing counteraction that requires fewer assumptions than a full causal mediation analysis and can be easily adapted to the regression approaches researchers often use for estimating the effects of interventions. In the two cases we explore, both of which suggest that interventions caused compensatory actions by governments, we find that either there exists no first-order impact of the hypothesized intervention or the intervention's expected direct impact on the spillover outcome would have had to be so large and so positive as to be implausible given our theoretical expectations. We conclude that it is far more likely that deteriorating rights have more to do with garden-variety selection effects or possibly alternative mechanisms than they do with strategic counteraction.
We focused primarily on counteraction because quantitative research has been used to back such claims. But the approach we suggest is appropriate for any theory that links unintended outcomes to mediated, or indirect effects. For example, claims about budgetary trade-offs would have to show that by making human rights a priority, some other indicator of human welfare, such as social justice, suffered a reduction in resources that in turn accounts for growing inequality. Similarly, arguments that the very task of improving human rights has negative downstream consequences will be more convincing when they demonstrate that human rights law and advocacy have first had their intended effect of improving rights—that is, there is some positive rights outcome to counter. The methods we propose could be used to shed light on a range of claims about unintended consequences found loosely stated in the literature.Footnote 76
Our general approach can also be useful, in somewhat modified form, to test for unintended consequences in other issue areas. For example, a government facing a budget constraint might choose to fund technologies that replace coal power plants, but scale back efforts to reduce automobile emissions (trade-offs); might oppose plans to reduce carbon emissions by stimulating political or social resistance (backlash) or might respond to pressure to reduce carbon emissions by requiring an expensive technology, but counter the effects of that on companies by allowing them to lower their standards in another area to offset those costs, essentially then merely shifting a pollution problem from one type to another (counteraction).
Negative consequences to human rights advocacy warrant continued vigilance, and the concerns of researchers who have hypothesized trade-offs, backlash, and counteraction are utterly valid. But there is a danger to attributing harm to international human rights law and advocacy when they are not the cause. Counteraction is a particularly serious mechanism since its existence implies that no intervention could potentially improve rights across the board since improving rights in one area would cause denigration of human rights in other areas to compensate for the improvements. The risk of discouraging promotion of international human rights norms based on underidentified causal mechanisms is very serious indeed. Statistical science aside, we would argue that even if committing to and advocating international human rights sometimes cause some harm, this would not necessarily justify silence. Rather, it should prompt consideration of means to blunt the effects of counteraction. While this is not the place to elaborate, we think moral grounds alone might justify continued rhetorical and substantive support for international human rights. These are philosophical and practical questions for policymakers and the international community to answer together. Our purpose has been to avoid unsupported inferences and point a way toward investigating these issues more rigorously to implement the most effective policies to improve human rights for the world's most vulnerable populations.
Data Availability Statement
Replication files for this article may be found at <https://doi.org/10.7910/DVN/1NTFKO>.
Supplementary Material
Supplementary material for this article is available at <https://doi.org/10.1017/S0020818320000661>.
Acknowledgments
We thank Emilie Hafner-Burton and Yonatan Lupu for sharing their data and commenting on earlier versions of this idea. Thanks also to Aila Matanock, Sarah Bermeo, Jason Klocek, Charlie Clotfelter, Geoffrey Dancy, Chris Fariss, Yuqing Hu, Tana Johnson, Emily Ritter, John Singleton, Michael Ward, Will Moore, Yiqing Xu, and Wei Song for insightful comments on earlier drafts.