The Stroop Color-Word Test is a commonly used tool in clinical and experimental psychological settings as a measure of selective attention, cognitive flexibility, and processing speed (Strauss, Sherman, & Spreen, Reference Strauss, Sherman and Spreen2006). Stroop paradigms include an interference task where the subject has to inhibit a highly automatic response in benefit of a less automatic one (Jensen & Rohwer, Reference Jensen and Rohwer1966). This is generally achieved asking the subject to name the ink color of a word whose meaning is incongruent with it (e.g. to name the red ink color of the word “blue”; Jensen & Rohwer, Reference Jensen and Rohwer1966). Under these conditions a “color-word interference effect” emerges as a significant increase in the time required completing the incongruent task as compared to the control task (i.e., word reading or congruent color naming). While word reading and color naming conditions have been proposed as measures of processing speed, the interference effect is intended to provide a measure of executive attention (Ríos, Periáñez, & Muñoz-Céspedes, Reference Ríos, Periáñez and Muñoz-Céspedes2004; Strauss et al., Reference Strauss, Sherman and Spreen2006).
The generalized use of Stroop test versions in psychological assessment such as the one by Golden (Reference Golden1978) has boosted the publication of different norms stratified by a number of demographic variables. Thus, age, educational level, or sex have been suggested as relevant features modulating task performance (Moering, Schinka, Mortimer, & Graves, Reference Moering, Schinka, Mortimer and Graves2004; Van der Elst, Van Boxtel, Van Breukelen, & Jolles, Reference Van der Elst, Van Boxtel, Van Breukelen and Jolles2006). In addition, some studies have demonstrated that the specific weight of demographic variables in Stroop scores may vary when comparing different ethnic groups, even within the same country, thus justifying specific norms or demographic corrections for them (Norman et al., Reference Norman, Moore, Taylor, Franklin, Cysique and Ake2011). Following this rationale, recent works have provided Stroop norms for large samples of Dutch (Van der Elst et al., Reference Van der Elst, Van Boxtel, Van Breukelen and Jolles2006), Greek (Zalonis et al., Reference Zalonis, Christidi, Bonakis, Kararizou, Triantafyllou, Paraskevas and Vasilopoulos2009), Korean (Seo et al., Reference Seo, Lee, Choo, Kim, Kim, Youn and Woo2008), African and Caucasian North-America (Norman et al., Reference Norman, Moore, Taylor, Franklin, Cysique and Ake2011), and Spanish populations (Peña-Casanova et al., Reference Peña-Casanova, Quiñones-Ubeda, Gramunt-Fombuena, Quintana, Aguilar and Molinuevo2009; Rognoni et al., Reference Rognoni, Casals-Coll, Sánchez-Benavides, Quintana, Manero, Calvo and Peña-Casanova2013).
While the primary function of norms is to identify the presence of pathological performance, they have been secondarily used to scale cognitive impairment. At this regard, norms from healthy samples are generally sensitive to achieve the first aim. However, it has been shown that they may present serious limitations regarding the second one, i.e., a large percentage of patients often score out of the range established by healthy norms. For instance, in a previous normative study of another attentional test (the Trail Making Test; Periáñez et al., Reference Periáñez, Ríos-Lago, Rodríguez-Sánchez, Adrover-Roig, Sánchez-Cubillo, Crespo-Facorro and Barcelo2007) a 30 % of the schizophrenia patients, and a 70 % of the traumatic brain injury (TBI) patients from the high education groups scored below percentile 5 as established by healthy norms (see Tables 6, 7, and 8 in Periáñez et al., Reference Periáñez, Ríos-Lago, Rodríguez-Sánchez, Adrover-Roig, Sánchez-Cubillo, Crespo-Facorro and Barcelo2007). This fact highlights at least two main problems when assessing clinical performance using norms from healthy samples. Firstly, norms from healthy samples cannot accurately scale cognitive impairment. Secondly, they lack of sensitivity for detecting subtle clinical changes across time (even after correcting for learning effects in retest measures; Van del Elst, Molenberghs, Van Boxter, & Jolles, Reference Van der Elst, Molenberghs, Van Boxtel and Jolles2013). These are two central concerns in clinical settings for both prognostic purposes and assessment of patients’ clinical course (Kizilbash, Warschausky, & Donders, Reference Kizilbash, Warschausky and Donders2001; Periáñez et al., Reference Periáñez, Ríos-Lago, Rodríguez-Sánchez, Adrover-Roig, Sánchez-Cubillo, Crespo-Facorro and Barcelo2007). For these reasons, different works have provided clinical norms for different tests such as Wisconsin Card Sorting Tests (Iverson, Slick, & Franzen, Reference Iverson, Slick and Franzen2000), Trail Making Test (Periáñez et al., Reference Periáñez, Ríos-Lago, Rodríguez-Sánchez, Adrover-Roig, Sánchez-Cubillo, Crespo-Facorro and Barcelo2007), Rey’s Auditory Verbal Learning Test (Badcock, Dragovic, Dawson, & Jones, Reference Badcock, Dragovic, Dawson and Jones2011), or Boston Naming Test (Casals-Coll et al., Reference Casals-Coll, Sánchez-Benavides, Meza-Cavazos, Manero, Aguilar and Badenes2014). However, to our knowledge, no clinical norms exist for the Stroop test.
Impaired performance on the Stroop has been described in a wide variety of clinical groups characterized by executive control and prefrontal lobe dysfunction such as traumatic brain injury (Felmingham, Baguley, & Green, Reference Felmingham, Baguley and Green2004; Ríos et al., Reference Ríos, Periáñez and Muñoz-Céspedes2004), schizophrenia (Hepp, Maier, Hermle, & Spitzer, Reference Hepp, Maier, Hermle and Spitzer1996; Rodríguez-Sánchez et al., Reference Rodríguez-Sánchez, Crespo-Facorro, González-Blanch, Perez-Iglesias and Vázquez-Barquero2007), or even during normal ageing (Coubard et al., Reference Coubard, Ferrufino, Boura, Gripon, Renaud and Bherer2011; Mayas, Fuentes, & Ballesteros, Reference Mayas, Fuentes and Ballesteros2012). Among others, these groups may constitute target populations for the design of specific clinical norms. In spite of this, until now, most normative studies on the Stroop test have focused in non-clinical samples.
The main purpose of the present study was to provide clinical norms of Golden’s version of the Stroop test (Golden, Reference Golden1978; Reference Golden1999) for schizophrenia and TBI Spanish populations together with matching data for healthy individuals. These norms will offer the clinician a tool for a comprehensive description of patients according to severity, as well as a more sensitive measure of clinical course.
Method
Participants
A total of 592 subjects took part in the study: 285 healthy subjects (170 female); 158 closed traumatic brain injury patients (31 female). 149 first-episode schizophrenia patients (67 female). They all were Spanish speakers and had normal or corrected-to-normal vision.
Healthy Controls (HC) were recruited from undergraduate university classes, university staff, social organizations, hospitals, and health care centers from three different regions of Spain (Madrid, Bilbao, and Santander). Medical complications, psychiatric disturbance, substance abuse (excluding nicotine), or neurological disease diagnosis were criteria for exclusion in this sample.
The schizophrenia (SCH) sample was comprised of patients with diagnosis of schizophrenia spectrum disorders (schizophrenia, schizophreniform, or schizoaffective) in their first episode. All patients from this group attended at a program for first-episode psychosis (PAFIP) carried out at the Hospital Marques de Valdecilla in Santander (see a detailed description in Crespo-Facorro et al., Reference Crespo-Facorro, Pérez-Iglesias, Ramirez-Bonilla, Martínez-García, Llorca and Vázquez-Barquero2006). Diagnoses were confirmed by an experienced psychiatrist by means of the Structured Clinical Interview for DSM-IV (SCID-I) 6 months after the initial contact. Patients with mental retardation, neurological illness, or drug dependence (excluding nicotine) were excluded. None of the patients had received neuroleptic medication prior to contact with the program. However, they all were on neuroleptic medication and had reached clinical stabilization when neuropsychological assessment for the current study was conducted. Cognitive assessment was therefore performed at 10 weeks after pharmacological treatment initiation, which has been previously stated as the most appropriate for cognitive evaluation (González-Blanch et al., Reference González-Blanch, Álvarez-Jiménez, Rodríguez-Sánchez, Pérez-Iglesias, Vazquez-Barquero and Crespo-Facorro2006).
One hundred and fifty-eight moderate to severe closed traumatic brain injury (TBI) patients were recruited from the Brain Damage Unit at Beata María Ana Hospital in Madrid, and the Brain Damage Unit at Aita Menni Hospital in Bilbao. Glasgow Coma Scale (GCS; Teasdale & Jennett, Reference Teasdale and Jennett1974) was available for 139 subjects (mean 6.9 ± 3.6). Post-traumatic amnesia duration (PTA), assessed with the Galveston Orientation and Amnesia Test (GOAT; Levin, O’Donnell, & Grossman, Reference Levin, O’Donnell and Grossman1979) was also recorded in 110 patients (mean 41.8 ± 35.8 days). The mean time since injury was 505 ± 692 days. All TBI patients had at least one of the two scores (GCS and/or GOAT) recorded. Patients with impairments that may interfere with testing (visual difficulties, aphasia, or apraxia) were excluded from this sample. All patients were out of PTA at the time of testing. The specific site of lesion was not considered for the analyses given that closed TBI is generally characterized by a diffuse pattern of injuries difficult to identify using conventional neuroimaging methods (Arfanakis et al., Reference Arfanakis, Haughton, Carew, Rogers, Dempsey and Meyerand2002). None of the participants was involved in litigation regarding their injury at the time of testing. All participants were informed about the investigation prior to the psychological evaluation session and signed a consent form according to the Declaration of Helsinki.
Procedures
Participants were administered the Spanish adaptation of the Stroop test (Golden, Reference Golden1999) by expertise psychologists as a part of a larger battery. This version consists of three conditions: a word-reading condition (WR) with 100 color words in Spanish printed in black ink, a color-naming condition (CN) with 100 “Xs” printed in color (red, green, and blue), and a color-word condition (CW) with the 100 color words in Spanish from the first condition printed in incongruent colors. Subjects were asked to read down the columns starting by the top word in the leftmost column. After 45 seconds, the item last named on each condition was noted. Whenever an error occurred participants were instructed to correct it but time counting did not stop. Direct scores were measured as the number of items completed on each condition. An Interference score (IS) was also calculated as the difference between CW score and CW´, where CW´ equals (WR*CN)/(WR+CN). This formula is based on the assumption that the time to name a color-word item is equal to the time needed to suppress the reading of a word plus the time to identify a color (Golden & Freshwater, Reference Golden and Freshwater2002). In case of impaired interference control, reading the word in the color-word condition will actively interfere with naming the color and switching from one to the other will go slowly, resulting in a smaller color-word score relative to the predicted score and thus a negative Golden’s interference score (Lansbergen, Kenemans, & van Engeland, Reference Lansbergen, Kenemans and van Engeland2007).
Statistical analysis
Chi-square statistic was used to explore sex distribution among groups. One-way analysis of variance (ANOVA) was used to compare groups regarding age and years of education. A series of analyses of covariance (ANCOVA) were used to explore the presence of differences in Stroop scores among samples, thus justifying the need of different norms for each of them. Correlation analyses and contingency coefficients were carried out for continuous and dichotomous variables, respectively, to explore the most appropriate variables for stratification. In a further step, multiple regression analyses were used on each separate group to study the relative impact of each stratification variable on Stroop scores. Cut-off points for age and education were established using the percentile 50. Finally, independent sample t-tests were performed to assess the appropriateness of cut-off points for each sample. Scores from the resulting groups were transformed into percentile scores. A significance level of .05 was set for all contrasts. A Bonferroni-corrected significance level of p < .05 was adopted for all tests of simple effects involving multiple comparisons. Effects sizes (Cohens’ d) for all contrasts were calculated with G*Power 3.1 statistical software (Faul, Erdfelder, Lang, & Buchner, Reference Faul, Erdfelder, Lang and Buchner2007).
Results
Demographics
Descriptive statistics for age, education, sex, and Stroop scores are presented in Table 1. The three groups differed on sex distribution (χ2 = 65.7; p < .001; d = .65). They also differed in age, F(2, 589) = 15.85; p < .001; d = .24, and years of education, F(2, 589) = 21.27; p < .001; d = .27. Post hoc analyses revealed that healthy controls were older than TBI and SCH patients (p < .001), but SCH and TBI patients did not differ in age from each other. SCH patients had a lower educational level than both HC and TBI patients (ps < .001), being the difference between the latter groups not significant.
Table 1. Statistical properties of demographic and Stroop variables for each sample

Note: HC = Healthy Controls; SCH = Schizophrenia patients; TBI = Traumatic Brain Injury patients; Edu = Education in years; WR = Word Reading; CN = Color Naming; CW = Color Word; IS = Interference Score.
Between-Group comparisons
Univariate ANCOVAs were performed in order to address between-group differences in Stroop scores using age and education as covariates to control their influence in performance. There was a main effect of Group in WR, F(2, 587) = 113.3; p < .001; d = .58; in CN, F(2, 587) = 126.7; p < .001; d = .6; in CW, F(2, 587) = 133.4; p < .001; d = .57; and IS, F(2, 587) = 39.9; p < .001; d = .33. Post hoc analyses revealed that for WR and CN scores, all groups differed from each other (ps < .001; d > .4 in all cases). In both cases, HC outperformed both clinical groups, with TBI patients showing the worst performance (see Table 1). For CW score, HC participants outperformed both clinical groups (ps < .001; d > 1 in both cases), but clinical groups did not differ from each other (p = .34; d = .03). Finally, all groups differed from each other in the IS (ps < .002; d > .3 in all cases), with HC participants showing the lowest interference effect, and SCH the highest.
Correlation and Regression analyses
Correlation analyses and contingency coefficients provided an approach to study relationships between Stroop scores and demographic variables (Table 2).
Table 2. Correlation matrixes of demographic and Stroop variables for each sample

Note: HC = Healthy Controls; SCH = Schizophrenia patients; TBI = Traumatic Brain Injury patients; Edu = Education in years; WR = Word Reading; CN = Color Naming; CW = Color Word; IS = Interference Score. *p < .05; **p < .01 (Two-tailed).
For HC participants, age was negatively correlated with all Stroop scores (ps < .001), whereas education correlated positively with WR, CN and CW (see Table 2). For SCH participants education correlated with all Stroop scores but IS, and age correlated negatively with CW and IS. Finally, for the TBI group education correlated positively with all Stroop scores but IS, whereas age correlated negatively with CW and IS. The analyses of contingency coefficients revealed that sex was not significantly related to any Stroop score in any of the three groups (ps > .508 for HC; ps > .199 for SCH; ps > .221 for TBI). Accordingly, only age and education were considered for further analyses.
Multiple regression analyses were carried out to study the relative contribution of age and education to Stroop scores on each sample separately (see Table 3). For the HC sample, age and education considered together had a significant contribution to the prediction of all Stroop variables (ps < .001). They jointly accounted for 16.7% of variance of WR score (d = .18), 25.9% of CN score (d = .26), 38% of CW score (d = .38), and 22.4% of IS score (d = .23). Partial correlations from multiple regression suggested that age and education were the most appropriate stratification variables for HC. In fact, age accounted for a significant portion of variance of all Stroop variables followed by education that accounted for a significant portion of variance of WR, CN, and CW (see Table 3). Given the large number of participants in this sample, both variables were considered for stratification.
Table 3. Results from multiple regression analyses using Stroop scores as criterion and education and age as predictors

Note: HC = Healthy Controls; SCH = Schizophrenia patients; TBI = Traumatic Brain Injury patients; Edu = Education in years; WR = Word Reading; CN = Color Naming; CW = Color Word; IS = Interference Score; Var = Percent of explained variance.
For the SCH sample, age and education considered together had a significant contribution to the prediction of all Stroop variables (ps < .006). They jointly accounted for 10.7% of variance of WR (d = .12), 7% of CN (d = .08), 15% of CW (d = .16), and 8.5% of the IS score (d = .1). Examination of partial correlations from multiple regression revealed that education accounted for a significant portion of variance of all Stroop scores, while age only accounted for CW and IS scores (see Table 3). For this reason education was the variable considered for stratification.
For the TBI sample, age and education considered together had a significant contribution to the prediction of all Stroop variables (ps < .012). They jointly accounted for 10% of variance of WR score (d = .11), 8% of CN score (d = .09), 13.2% of CW score (d = .14), and 4.3% of IS score (d = .07). Examination of partial correlations from multiple regressions revealed a significant contribution of both age and education to three of the Stroop variables (see Table 3). However, only education was selected for stratification given its larger contribution to the explanation of the variance as compared to age.
Stratification
First, the HC sample was divided into two age groups according to percentile 50: young and middle-age (see Table 4 for descriptive statistics). Both groups differed in WR, t (283) = 4.8; p < .001; d = .58;, in CN score, t (283) = 8; p < .001; d = .98; in CW score, t (283) = 11.3; p < .001; d = 1.44; and IS score, t (283) = 8.8; p < .001; d = 1.15. Second, each age group was divided into two education groups: young-high education, young-low education, middle age-high education, and middle age-low education. In the young group, high education participants outperformed low education ones only in WR, t (147) = –3; p = .004; d = .51. On the contrary, in the middle age group high education participants outperformed low education ones in both WR, t (134) = –2.8; p = .006; d = .49 and CW, t (134) = –2.6; p = .009; d = .48, while differences between groups in CN were only marginally significant, t (134) = –1.9; p = .06; d = .33. Accordingly, only the middle-age group was divided into two education levels for stratification of norms (0–11 and 12+ years).
Table 4. Statistical properties of the demographic and Stroop variables for healthy controls (n = 285)

Note: Edu = Education in years; WR = Word Reading; CN = Color Naming; CW = Color Word; IS = Interference Score.
The SCH sample was divided into two groups of education (see Table 5 for descriptive statistics): low and high level of education. Both groups differed in WR score, t (147) = –3.80; p < .001; d = .64; in CN score, t (147) = –2.89; p < .004; d = .49; in CW score, t (147) = –3.92; p < .001; d = .71; and IS score, t (147) = –2.28; p < .024; d = .41.
Table 5. Statistical properties of demographic and Stroop variables for patients with schizophrenia (n = 149)

Note: Edu = Education in years; WR = Word Reading; CN = Color Naming; CW = Color Word; IS = Interference Score.
The TBI sample was divided into two groups of education (see Table 6 for descriptive statistics): low and high level of education. Both groups differed in WR, t (156) = –4.1; p < .001; d = .67; in CN, t (156) = –2.91; p = .004; d = .47; and in CW scores, t (156) = –3.2; p = .002; d = .53 but not in IS score, t (156) = –1.1; p = .277; d = .19.
Table 6. Statistical properties of demographic and Stroop variables for patients with (n = 158)
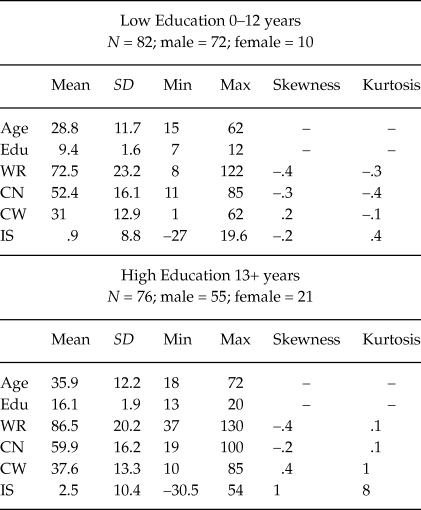
Note: Edu = Education in years; WR = Word Reading; CN = Color Naming; CW = Color Word; IS = Interference Score.
Tables 4–6 provide descriptive statistics (mean, standard deviation, maximum, minimum, skewness, and kurtosis) for age, education, and Stroop variables in the three different samples. Tables 7–9 provide normative data for Stroop variables stratified by age and education in the case of HC, and by education in the case of SCH, and TBI samples.
Table 7. Percentile ranks for healthy controls (n = 285) stratified by age and education

Note: Edu = Education in years; Perc = Percentile; WR = Word Reading; CN = Color Naming; CW = Color Word; IS = Interference Score.
Table 8. Percentile ranks for the schizophrenia sample (n = 149) stratified by education

Note: Edu = Education in years; Perc = Percentile; WR = Word Reading; CN = Color Naming; CW = Color Word; IS = Interference Score.
Table 9. Percentile ranks for the TBI sample (n = 158) stratified by education

Note: Edu = Education in years; Perc = Percentile; WR = Word Reading; CN = Color Naming; CW = Color Word; IS = Interference Score.
Discussion
The increase in the number of studies providing Stoop norms from different non-clinical populations has helped to avoid the risk of under- or over-estimating cognitive functioning due to cultural or socio-demographical differences (Norman et al., Reference Norman, Moore, Taylor, Franklin, Cysique and Ake2011; Seo et al., Reference Seo, Lee, Choo, Kim, Kim, Youn and Woo2008; Van der Elst et al., Reference Van der Elst, Van Boxtel, Van Breukelen and Jolles2006; Zalonis et al., Reference Zalonis, Christidi, Bonakis, Kararizou, Triantafyllou, Paraskevas and Vasilopoulos2009). Clinical norms have been also provided for different psychological tools such as Wisconsin Card Sorting Tests (Iverson et al., Reference Iverson, Slick and Franzen2000), Trail Making Test (Periáñez et al., Reference Periáñez, Ríos-Lago, Rodríguez-Sánchez, Adrover-Roig, Sánchez-Cubillo, Crespo-Facorro and Barcelo2007), Rey’s Auditory Verbal Learning Test (Badcock et al., Reference Badcock, Dragovic, Dawson and Jones2011), or Boston Naming Test (Casals-Coll et al., Reference Casals-Coll, Sánchez-Benavides, Meza-Cavazos, Manero, Aguilar and Badenes2014). The aim of this study was to provide clinical norms of the Golden’s (Reference Golden1999) version of the Stroop test for Spanish patients with TBI and schizophrenia, together with matching data for healthy individuals. In the following sections differences between groups and criteria for stratification will be discussed in relation to preceding literature.
Results from the ANCOVAs comparing Stroop WR and CN scores between the three samples showed that both groups of patients scored lower than healthy controls in all test conditions. Moreover, both TBI and SCH groups exhibited greater interference effects than healthy controls, as reflected by the IS. Regarding TBI patients, the results confirmed those from prior investigations suggesting that TBI is associated with a generalized slowing in task performance across all test conditions (Felmingham et al., Reference Felmingham, Baguley and Green2004; Ríos et al., Reference Ríos, Periáñez and Muñoz-Céspedes2004). In addition, differences in IS between TBI patients and healthy controls indicated that the TBI group displayed difficulties in the interference condition of the test. In fact, 42 % of the TBI participants obtained a negative score. These results support preceding studies suggesting that, in addition to slowed information processing speed, TBI might be associated to a deficit in selective attention (Summers, Reference Summers2006). Regarding SCH patients, our data agree with previous findings showing that slowness is a prevalent feature in this population when facing the Stroop task (Brébion et al., Reference Brébion, Smith, Gorman, Malaspina, Sharif and Amador2000; Rodríguez-Sánchez et al., Reference Rodríguez-Sánchez, Crespo-Facorro, González-Blanch, Perez-Iglesias and Vázquez-Barquero2007). However, attention deficits also seem to play a role that would account for the presence of differences between SCH patients and healthy controls in IS (Westerhausen, Kompus, & Hugdahl, Reference Westerhausen, Kompus and Hugdahl2011). Moreover, the comparison between both clinical samples revealed that, even when TBI patients were significantly slower than SCH patients (as revealed by differences in WR and CN conditions), SCH patients scored lower than TBI in IS. Thus, although results revealed the presence of a specific deficit in executive control in both clinical samples, SCH patients displayed the greatest interference effect as compared to TBI (66% of the SCH patients obtained a negative IS).
The analyses derived from the stratification of HC showed that age was the best predictor of individual’s performance in the Stroop test. In fact, it accounted for a significant portion of variance in all test conditions. Different authors have recognized that ageing involve a slowing in CN as well as an increase in the interference effect (Moering et al., Reference Moering, Schinka, Mortimer and Graves2004; Van der Elst et al., Reference Van der Elst, Van Boxtel, Van Breukelen and Jolles2006). Contrasting these results and those from the present study, Rognoni et al. (Reference Rognoni, Casals-Coll, Sánchez-Benavides, Quintana, Manero, Calvo and Peña-Casanova2013) found that age did not have any effect on Stroop scores in their Spanish sample. However, it has to be noted that their sample of healthy Spanish controls did not include participants over 50 years old. This fact may account for this apparent inconsistency regarding the role of age, since Stroop effects seem to be more evident in the last decades of life (Uttl & Graf, Reference Uttl and Graf1997). Like age, education resulted to be a good predictor of HC Stroop performance in the present study, accounting for significant portions of variance in both WR and CN scores. The demographic effects of education have been consistently reported for both Spanish and non-Spanish populations (Moering et al., Reference Moering, Schinka, Mortimer and Graves2004; Peña-Casanova et al., Reference Peña-Casanova, Quiñones-Ubeda, Gramunt-Fombuena, Quintana, Aguilar and Molinuevo2009; Rognoni et al., Reference Rognoni, Casals-Coll, Sánchez-Benavides, Quintana, Manero, Calvo and Peña-Casanova2013; Van der Elst et al., Reference Van der Elst, Van Boxtel, Van Breukelen and Jolles2006). In the present work, sex did not have an influence in any Stroop score. Although in some works women have tended to score higher in color-naming (Moering et al., Reference Moering, Schinka, Mortimer and Graves2004; Van der Elst et al., Reference Van der Elst, Van Boxtel, Van Breukelen and Jolles2006), sex differences on the CW condition are not always present (Golden & Freshwater, Reference Golden and Freshwater2002; Moering et al., Reference Moering, Schinka, Mortimer and Graves2004; Rognoni et al., Reference Rognoni, Casals-Coll, Sánchez-Benavides, Quintana, Manero, Calvo and Peña-Casanova2013). Taken together, these results highlight the importance of considering norms reflecting the specific impact of demographic variables in different populations.
Similarly to HC, both age and education impacted Stroop scores in the two clinical groups. The analyses revealed that education was a good demographic predictor of Stroop performance in both TBI and SCH samples. Age accounted for a portion of the variance of CW and IS in the SCH sample, and of CN, CW, and IS in the TBI sample. However, this portion was in most cases inferior to that accounted by education. This result fits prior TBI and SCH clinical norms where edu-cation was the main variable selected for stratification in a different attentional test (Periáñez et al., Reference Periáñez, Ríos-Lago, Rodríguez-Sánchez, Adrover-Roig, Sánchez-Cubillo, Crespo-Facorro and Barcelo2007). Thus, it is important to consider these demographic variables when interpreting Stroop performance in the clinical context. Ignoring their influence could lead to misinterpretations when monitoring evolution or recovery, over- or underestimating patients’ difficulties.
Regarding the generalization of the present data, it seems plausible that the influence of TBI and SCH is higher than the influence of cultural and demographic variables. The effect sizes found when comparing HC, TBI and SCH groups are higher than those found when studying differences due to age and education. It is also known that ethnicity (Moering et al. Reference Moering, Schinka, Mortimer and Graves2004; Norman et al., Reference Norman, Moore, Taylor, Franklin, Cysique and Ake2011), country (Buré-Reyes et al., Reference Buré-Reyes, Hidalgo-Ruzzante, Vilar-López, Gontier, Sánchez, Pérez-García and Puente2013), and language (Rosselli et al., Reference Rosselli, Ardila, Santisi, Arecco, Salvatierra, Conde and Lenis2002) may impact test scores (Strauss et al., Reference Strauss, Sherman and Spreen2006). However, no cultural or demographic variables show higher effect sizes than those found among healthy and pathological groups.
To summarize, the major value of the present study was to provide a set of clinical norms to determine more precisely the extent to which Stroop scores on WR, CN, CW, and IS reflect impairments in performance, and changes across time. This issue has implications for research, forensic, and clinical settings allowing a more precise description of patients, and a more sensible detection of changes in performance across time. However, it should be clearly established that the present clinical norms do not avoid the risk of misinterpreting undesired retest effects (i.e., practice effects or procedural learning) as real cognitive changes. Complementary normative methods have been recently proposed to solve this issue, and should be applied before using the present clinical norms in longitudinal assessments (Calamia, Markon, & Tranel, Reference Calamia, Markon and Tranel2013; Van del Elst et al., Reference Van der Elst, Molenberghs, Van Boxtel and Jolles2013).
This work was partially supported by the grant PSI2009–14415-C03–03 from the Ministerio de Ciencia e Innovación (MICINN) of Spain; by MAPFRE Medicina Foundation; Instituto de Salud Carlos III PI020499, PI050427, PI060507; Plan Nacional de Drogas Research Grant 2005- Ordensco/3246/2004; SENY Fundation Research Grant CI 2005–0308007; and Fundación Marqués de Valdecilla API07/011. The funders had no role in study design, data collection and analysis, decision to publish, or preparation of the manuscript.
We want to thank all patients and healthy participants who voluntarily and generously took part in the study.