Introduction
Since the formation of modern natural resource management institutions, the majority of wildlife resource exploitation assessments have been derived either from observations or formal declarations, typically made by those specifically employed as researchers or natural resource managers (from here, ‘researchers’). This has been the case for fisheries management, where such methods have been championed by fisheries science organizations like the International Council for the Exploration of the Seas (ICES), formed in 1902. The types of methods used by ICES have been exported globally, being used as the model for other fisheries management bodies (Rozwadowski Reference Rozwadowski2002). These now established methods for resource management generally rely on data-heavy sampling and complex statistics – a substantial barrier when time, financial capacity or personnel expertise is limited.
If we were to go back roughly 100 years, such intensive methods were not common. Instead, assessments were founded on the knowledge of those using natural resources, such as in Canadian (Murray et al. Reference Murray, Neis, Palmer and Schneider2008) and Scottish (Thurstan & Roberts Reference Thurstan and Roberts2010) fisheries. Although local knowledge (LK), based on both the observations and experiences of those not directly employed as researchers (Stephenson et al. Reference Stephenson, Paul, Pastoors, Kraan, Holm and Wiber2016), has attracted academic – and some bureaucratic – interest as an information source for resource management, to date there is a lack of quantitative evaluations of the relationship between LK and researcher-led observations.
Since recording LK is generally considered a cheap but effective process (Neis et al. Reference Neis, Schneider, Felt, Haedrich, Fischer and Hutchings1999, Anadón et al. Reference Anadón, Giménez, Ballestar and Pérez2009, Rist et al. Reference Rist, Milner-Gulland, Cowlishaw and Rowcliffe2010), the use of LK observations to assess various aspects of data-poor and capacity-limited fisheries is increasingly common (e.g., Moore et al. Reference Moore, Cox, Lewison, Read, Bjorkland and McDonald2010, Pilcher et al. Reference Pilcher, Adulyanukosol, Das, Davis, Hines and Kwan2017). Such situations are perhaps most evident in the fisheries of low- and middle-income regions, making the use of LK in these regions particularly attractive. Additionally, LK observations may be advantageous in documenting unusual or illegal events, which researcher-led observations are liable to miss (Peterson & Stead Reference Peterson and Stead2011, Slater et al. Reference Slater, Napigkit and Stead2013). Conversely, LK is vulnerable to interviewee subjectivity and bias, be it malicious or malign, such as through the provision of misleading information or biases in cognitive recall. Yet, ignorance of LK has, in some cases, resulted in fisheries mismanagement (Johannes et al. Reference Johannes, Freeman and Hamilton2000).
Despite uncertainties in both LK and researcher-led observations, there are few studies that cross-examine their outputs. The majority have been restricted to evidencing agreement (e.g., Anadón et al Reference Anadón, Giménez, Ballestar and Pérez2009, Rist et al. Reference Rist, Milner-Gulland, Cowlishaw and Rowcliffe2010, Daw et al. Reference Daw, Robinson and Graham2011) and fail to assess bias and precision among the methods. Evidence for agreement between LK and researcher-led observations is mixed (Anadón et al. Reference Anadón, Giménez, Ballestar and Pérez2009, Rist et al. Reference Rist, Milner-Gulland, Cowlishaw and Rowcliffe2010, O’Donnell et al. Reference O’Donnell, Molloy and Vincent2012), although LK is generally considered to be a useful indicator of long-term trends (Stead et al. Reference Stead, Daw and Gray2006, Daw et al. Reference Daw, Robinson and Graham2011, O’Donnell et al. Reference O’Donnell, Molloy and Vincent2012). The use of LK to assess shorter temporal ranges, such as intra-annual trends, has received relatively limited attention since a number of earlier publications outlined how knowledge accumulated in real time, over the shortest timescales, may be amongst the most unique knowledge possessed by fishers (Fischer Reference Fischer, Neis and Felt2000, Knapman Reference Knapman and Gray2005, Hind Reference Hind2012). Yet, intra-annual trends are often important in the formulation of management strategies.
The aim of this study is to assess the capability of LK observations to provide data for improved sustainable resource management in data-poor and capacity-limited settings. Furthermore, the case study presented, which assesses intra-annual patterns in small-scale fisheries effort and catch (in this case of mobulid rays, Mobula spp.), is, to our knowledge, the first of its kind. Mobulid rays are a valuable commodity in both local and international trade, which, when paired with an extremely conservative life history, threatens them with overexploitation (Croll et al. Reference Croll, Dewar, Dulvy, Fernando, Francis and Galván-Magaña2016). This case study facilitates an initial assessment of the potential use of LK observations as a proxy for researcher-led observations in data-poor and capacity-limited situations at intra-annual timescales.
Methods
Trained observers from the then Ministry of Livestock and Fisheries (now Ministry of Agriculture, Natural Resources, Livestock and Fisheries) collected researcher-led observations of fisheries effort (active vessels per day) and landed catch (individuals per day) of mobulid rays (n = 161) from bottom-set and drift gillnets, longlines and handlines at small-scale fisheries landings sites in Zanzibar (n = 8) (Fig. 1); 147 simultaneous days were observed over a complete 12-month period between June 2016 and 2017. In order to account for lunar-driven patterns in fishing effort and species availability, monitored days were selected using a stratified-random approach; the year was divided into lunar months, which were subdivided into four lunar phases (new moon, first quarter, full moon and third quarter), and three sampling days were randomly generated within each lunar phase. Landing sites were selected to account for the following criteria: the prevalence of longline and gillnet gears (the primary gear threats to rays); geographic spread (maximizing geographical coverage and potential links to species availability); and logistical constraints (e.g., sites needed to be accessible by road) (Temple et al. Reference Temple, Wambiji, Poonian, Jiddawi, Stead, Kiszka and Berggren2019). The resultant data were linearly scaled to monthly totals.
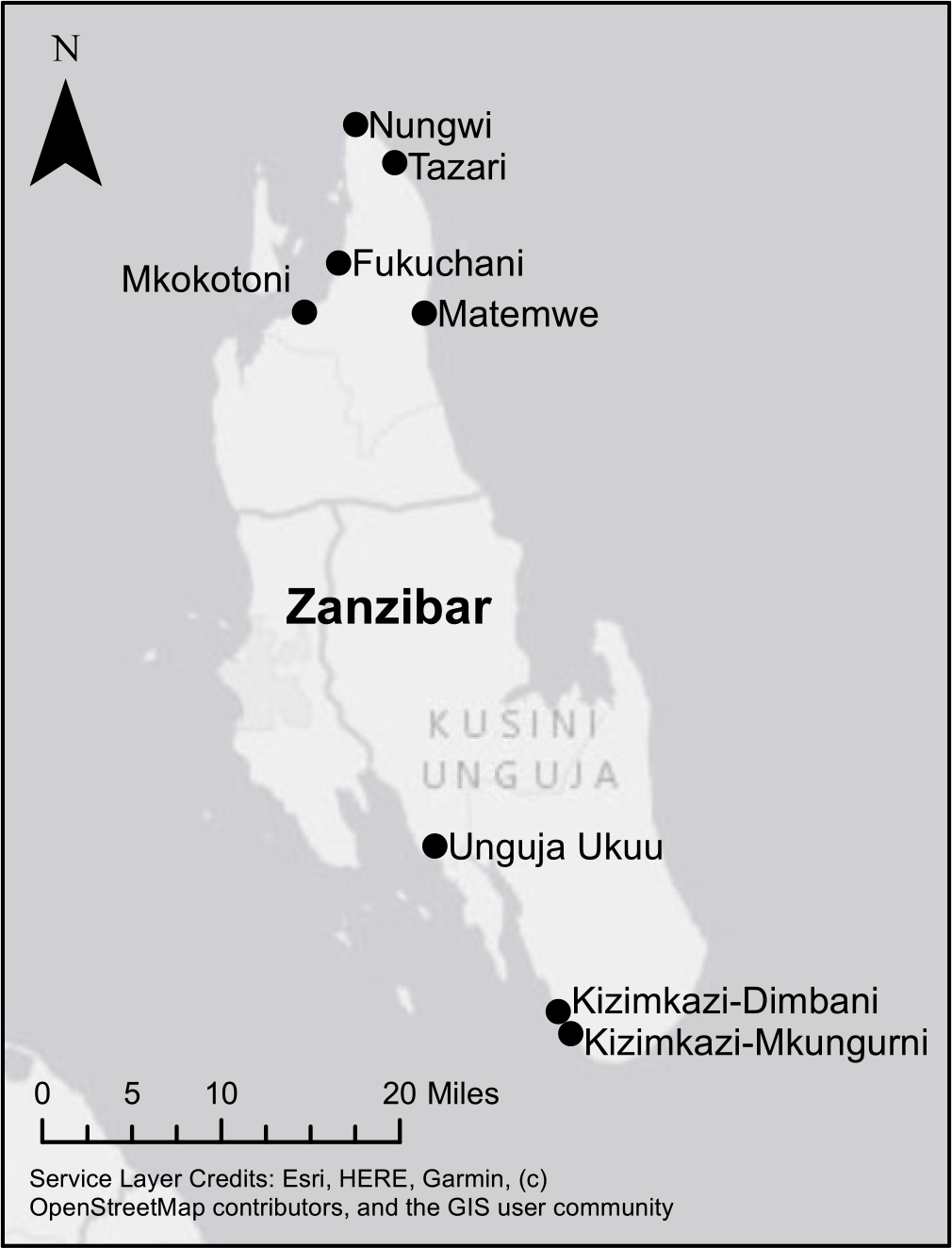
Fig. 1. Locations of landing sites in Zanzibar where both local knowledge and researcher-led observations were recorded for fishing effort and mobulid catch between June 2016 and June 2017.
Local knowledge observation data were collected using a modified rapid bycatch assessment (RBA) interview (e.g., Moore et al. Reference Moore, Cox, Lewison, Read, Bjorkland and McDonald2010, Alfaro-Shigueto et al. Reference Alfaro-Shigueto, Mangel, Darquea, Donoso, Baquero, Doherty and Godley2018) in September 2017. The RBAs targeted fishers and fishing vessel captains in the same small-scale fisheries landing sites, covering the same gears and temporal period (n = 204, captains = 99). The RBAs recorded declarations of average days fished per month (on an annual level), months in which fishing occurred, average mobulid catch per month (on an annual level) and months in which catches occurred. For a minimum of three or a quarter of the known vessels (whichever was largest), RBAs were conducted for each gear type at each site in order to achieve a representative sample. The RBAs were carried out in Swahili by NJ, who is a native speaker. Interviewees were selected opportunistically, avoiding multiple crew members from the same vessel. The RBAs lasted c. 20 minutes. Interviewees were informed of both the motivation and the intended use of the data collected, the anonymity of their responses, the right to decline answering any question and the right to end the interview at any stage. Verbal consent was sought before the RBA was undertaken. The RBAs were not facilitated with either monetary or material motivation.
Statistical analysis
All analyses were carried out using the R statistical software package v3.6.0 (R Core Team 2019). We used the Bland–Altman approach (Bland & Altman Reference Bland and Altman1999, Reference Bland and Altman2003) to compare intra-annual patterns (measured as a proportion of annual total) of fisheries effort and catch observations. Agreement was assessed using binomial generalized linear mixed models (GLMMs), with site treated as a random effect for both slope and intercept (R package lme4). Subsequently, bias was assessed by modelling the relationship between the means of the methods and the difference between the methods using linear mixed effect (LME) models, with site treated as a random effect for both slope and intercept (R package lme4). The precision of methods relative to one another was described by the exact limits of agreement (LOAs), equivalent to the 95% mean confidence interval (CI) of the differences between methods (Carkeet & Goh Reference Carkeet and Goh2018). Both GLMMs and LME models were weighted using the RBA sample size, reflecting increased confidence in data derived with larger sample sizes.
Results
The GLMM for intra-annual patterns in fishing effort showed a significant, but relatively weak, relationship between LK and researcher-led observations (Z = 2.04, p = 0.042, r 2 c = 0.006) (Fig. 2(a)) and found no evidence for any interacting effect of gear type on the relationship between methods (analysis of variance (ANOVA), χ2 = 0.801, p = 0.992). As there was sufficient evidence of a positive relationship between method outputs for fisheries effort, assessments of bias and precision were undertaken. The LME models demonstrated a significant deviance from the null model (ANOVA, χ2 = 37.181, p < 0.001), indicating a significant bias between method outputs, and there was no significant interacting effect of gear type on the bias between methods (ANOVA, χ2 = 6.12, p = 0.410). The RBA surveys produced higher fishing effort estimates than observer data at low mean effort and the inverse at high mean effort (Fig. 2(b)). The LOAs, once bias was accounted for, were estimated at ±3.67% (95% CI = 3.37–4.03%) of annual effort in any given month (Fig. 2(b)).

Fig. 2. Relationships between estimates of fishing effort and mobulid catch derived from local knowledge (LK) and researcher-led observations. (a) Regression line derived from binomial generalized linear mixed model for fisheries effort. (b) Bland–Altman plot showing significant bias between observations and the limits of agreement between observations for fisheries effort. (c) Regression line derived from binomial generalized linear mixed model for mobulid catch. (d) Bland–Altman plot showing significant bias between observations and the limits of agreement between observations for mobulid catch.
The GLMM for intra-annual patterns in fisheries catches showed a significant, but relatively weak, relationship between methods (Z = 3.49, p < 0.001, r 2 c = 0.101) (Fig. 2(c)). As there was sufficient evidence of a positive relationship between methods for fisheries catch, assessments of bias and precision was undertaken. The LME models demonstrated a significant deviance from the null model (ANOVA, χ2 = 15.5, p < 0.001). The results indicate the presence of significant bias between methods for mobulid ray catch, with RBA surveys producing higher catch estimates than observer data at low mean catches and the inverse at high mean catches (Fig. 2(d)). The LOAs, once bias was accounted for, were estimated at ±22.4% (95% CI = 19.3–27.0%) of annual mobulid catch in any given month (Fig. 2(d)).
Discussion
We found a positive relationship between LK and researcher-led observations of intra-annual patterns in fisheries effort and catches. This suggests that both approaches may act as a proxy, or complement, for one another when assessing such harvest effort and wildlife resource exploitation data. This outcome provides support for the expanded use of LK as an assessment tool with which to support the sustainable management of wildlife resource exploitation, particularly in data-poor and capacity-limited situations. Indeed, by demonstrating a real-world application, it strengthens representations already being made in the specific context of fisheries management for the greater integration of fishers’ LK (often termed ‘fishers’ knowledge’) into scientific assessments (Soto Reference Soto2006, Hind Reference Hind2012, Reference Hind2015, Stephenson et al. Reference Stephenson, Paul, Pastoors, Kraan, Holm and Wiber2016). However, the analyses also highlight the importance of considering bias and precision between LK and researcher-led observations in order to facilitate informed interpretation of their outputs. The significant bias and low level of precision between LK and researcher-led observations evidenced in this study undermine any baseline assumptions of equivalency, in spite of the general evidence for method agreement. Understanding and accounting for factors that drive inequivalences (which may be both generalized and/or case specific) between LK and researcher-led observations are important steps in supporting the decision-making for sustainable wildlife resource exploitation.
Equivalency between LK and researcher-led observations is a particularly important consideration here because natural resource management is an activity where it is readily identified that epistemic communities have formed around shared and coordinated knowledge bases, which they have then brokered. As communities are empowered through governing institutions prioritizing their knowledge in the policymaking process, they essentially determine which knowledge is used in management (Haas Reference Haas1989). Epistemic communities have typically been dominated by researchers because, firstly, their approaches have typically aligned with governing agendas of doing what is perceived as good by citizens and, secondly, it has suited governments to refer to a single group, as this creates economies of scale and results in quicker arrival at consensus (Weale Reference Weale1992). Natural resource management has been little different. The knowledge of those beyond the dominant epistemic community remains what might be considered ‘subjugated’ (Foucault & Ewald Reference Foucault and Ewald2003) or ‘lower on the competence hierarchy’, being integrated only at the discretion of the researchers, as is the case for fisheries management (Jentoft Reference Jentoft2005). Gaining perceived equivalence of utility in the eyes of researchers, or at least reaching such levels, is the most likely path to LK being used in management (Soto Reference Soto2006, Hind Reference Hind2012).
Perhaps the most important factor to consider, then, is simply: are LK and researcher-led observations measuring the same thing? Such disparities have been seen in studies compiling knowledge from various sources (e.g., Jennings & Polunin Reference Jennings and Polunin1995, Daw et al. Reference Daw, Robinson and Graham2011), where differences in selectivity and spatiotemporal coverage undermine equivalency. The same spatiotemporal disparities have even been promoted as a chance to manage at scales seen as desirable, but at which this has not yet been possible based solely on data derived from researcher-led observation (Griffin Reference Griffin2009, Hind Reference Hind2012). Regarding the present study, there are several factors potentially contributing to a lack of equivalency between LK and researcher-led observations. Discards, loss of catch at sea and secret or hidden landings inevitably create underestimates in fisheries landings observation data, but could feature in LK observations. Underestimates are potentially most prevalent for those catches most difficult or dangerous to bring aboard, especially in gears that are not suited to their capture, and for illegal or heavily regulated catches, which may be discarded or hidden for fear of prosecution. Furthermore, fishers often land catches at sites other than their home port, depending on local market conditions and demand for specific catches (Temple unpublished data). This may result in site-specific under- and over-representation of some catches from LK. Lastly, the migratory nature of some fisheries in this (Wanyonyi et al. Reference Wanyonyi, Wamukota, Mesaki, Guissamulo and Ochiewo2016) and other regions means that fishers may be active in other fishing grounds when activity from their home port is low. Greater consideration for, and disaggregation of, these and similar potential factors may help improve the equivalency of LK and researcher-led observations and/or improve the informed interpretation of their outputs relative to one another.
The efficacy of both LK and researcher-led observations in representing reality is another important consideration. For example, it is probable that the efficacy of researcher-led observations will vary with the overall level of observer competence (e.g., level of training provided), individual observer competence and the nature of the landing sites themselves (e.g., size, layout and level of formal organization). Similarly, researcher-led observation efficacy likely varies among components of the catch. For example, smaller specimens are perhaps less likely to be observed if they are mixed with bulk landings of similarly sized catch, and rare or infrequent catches may become underrepresented with only a small number of missed observations. Conversely, the efficacy of LK observations may be affected by survey design and biases in human memory recall. For example, the RBA questionnaire used in the present study derives catch and effort data from average monthly levels alongside months of occurrence, an approach that likely supresses the magnitude of monthly variability. Human recall is generally improved for events that are particularly unusual or emotive (e.g., unusually poor fishing conditions, catches of unusual size, volume, value or rarity) and/or that display prominent and consistent temporal trends (Matlin Reference Matlin2004, Hirst et al. Reference Hirst, Phelps, Buckner, Budson, Cuc and Gabrieli2009). Such events may be more easily recalled by fishers and may therefore be overrepresented relative to other less memorable events. As a result, LK observations of fisheries effort and catches may be partially obscured at the fishery level. High variability among fisher declarations, which was evident here, may also partially obscure catch and effort patterns at the fishery level (O’Donnell et al. Reference O’Donnell, Molloy and Vincent2012). Mobulid rays display traits that could potentially increase their memorability (e.g., unusual body form, large size, high value, distinct seasonality and relative rarity), and this might be expected to increase the reliability of LK observations, if it were the case. Agreement between LK and researcher-led observations for species that are not memorable to fishers might be expected to be lower, a potential effect that should to be considered in future sampling methodologies.
The current use and continued iterative refinement of both LK and researcher-led observation methods are ongoing challenges for researchers and managers of natural wildlife resource exploitation. Yet method comparison studies are uncommon and rarely consider bias and precision (e.g., Anadón et al. Reference Anadón, Giménez, Ballestar and Pérez2009, Rist et al. Reference Rist, Milner-Gulland, Cowlishaw and Rowcliffe2010, Daw et al. Reference Daw, Robinson and Graham2011). We believe that the concurrent use and thorough cross-examination of outputs from these methodologies will be valuable to future methodological developments and to current usage of method outputs, as well as support moves to integrate LK into mainstream research and management of natural resources (Stephenson et al. Reference Stephenson, Paul, Pastoors, Kraan, Holm and Wiber2016). Assessment of agreement, the identification of bias and quantification of precision allow for a greater understanding of the variable structure of the relationships among the methods. Thus, comparative studies can better facilitate the identification of method shortcomings or disparities and thus improve method refinement and contextualization. Most importantly, comparative studies stand to inform the appropriate use of LK, established and novel method outputs. This is a vital step in ensuring the appropriate application of method outputs to the sustainable management of wildlife resources and the livelihoods and wellbeing of those who are dependent upon them. The findings herein contribute to the wider discourse on how LK can help countries improve progress towards achieving the targets of the United Nations Sustainable Development Goals.
Acknowledgements
The authors thank Kenya Marine and Fisheries Research Institute, Conservation Centrée sur la Communauté (C3) Madagascar and Florida International University who, alongside the authors’ institutions, facilitated elements of this study. Particular thanks go to Y Salmin, N Wambiji, C Poonian, D Pandu, O Amir and J Kiszka. Furthermore, we thank all of the fisheries observers and interviewers involved in the collection and collation of data and the various fishers who agreed to be interviewed for this research.
Financial support
This work was supported by the Western Indian Ocean Marine Science Association (Grant Number MASMA/CP/2014/01).
Conflict of interest
None.
Ethical standards
All data used in this study were collected in line with national and institutional laws and requirements. Ethical approval for the study was sought, and granted, from both Newcastle University, UK, and the University of Dar es Salaam, United Republic of Tanzania, as appropriate. The RBA interviewees were informed of both the motivation and the intended use of the data collected, the anonymity of their responses, the right to decline answering any question and the right to end the interview at any stage were assured. Verbal consent was sought before the RBA was undertaken. The RBAs were not facilitated with either monetary or material motivation.