1 Introduction
The term ‘cloud robotics’ (CR) was first coined by Kuffner (Reference Kuffner2010). It refers to any robot or automation system that utilizes the cloud infrastructure for either data or code of its execution, that is, a system where all sensing, computation, and memory are not integrated into a single standalone system (Kehoe et al., Reference Kehoe, Patil, Abbeel and Goldberg2015).
Cloud robotic systems (CRS) appeared at the beginning of this decade, and their presence is increasing in both industrial utilization (Rahimi et al., Reference Rahimi, Shao, Veeraraghavan, Fumagalli, Nicho, Meyer, Edwards, Flannigan and Evans2017) and daily life use (Sharath et al., Reference Sharath, Srisha, Shashidhar and Bharadwaj2018). Hence, applications of CRS range from smart factory (Rahman et al., Reference Rahman, Jin, Cricenti, Rahman and Kulkarni2019) including teleoperation (Salmeron-Garcia et al., Reference Salmeron-Garcia, Inigo-Blasco and Cagigas-Muniz2015) to smart city (Ermacora et al., Reference Ermacora, Toma, Bona, Chiaberge, Silvagni, Gaspardone and Antonini2013), encompassing surveillance (Bruckner et al., Reference Bruckner, Picus, Velik, Herzner and Zucker2012), urban planning (Bruckner et al., Reference Bruckner, Picus, Velik, Herzner and Zucker2012), disaster management (Jangid & Sharma, Reference Jangid and Sharma2016), ambient-assisted living (Quintas et al., Reference Quintas, Menezes and Dias2011), life support (Kamei et al., Reference Kamei, Nishio, Hagita and Sato2012), and elderly care (Kamei et al., Reference Kamei, Zanlungo, Kanda, Horikawa, Miyashita and Hagita2017).
Although CR is still a nascent technology (Jordan et al., Reference Jordan, Haidegger, Kovacs, Felde and Rudas2013), a number of start-ups and spin-offs have been recently created to develop and commercialize CR targeting different application domains. For example, Rapyuta Robotics (Hunziker et al., Reference Hunziker, Gajamohan, Waibel and D’Andrea2013; Mohanarajah et al., Reference Mohanarajah, Hunziker, D’Andrea and Waibel2015), which is an Eidgenössische Technische Hochschule spin-off, builds cloud-connected, low-cost multi-robot system for security and inspection based on RoboEarth (Waibel et al., Reference Waibel, Beetz, Civera, D’Andrea, Elfring, Galvez-Lopez, Haussermann, Janssen, Montiel, Perzylo, Schiessle, Tenorth, Zweigle and van de Molengraft2011), (Riazuelo et al., Reference Riazuelo, Tenorth, Di Marco, Salas, Galvez-Lopez, Moesenlechner, Kunze, Beetz, Tardos, Montano and Martinez-Montiel2015). CloudMinds is a CR start-up for housekeeping and family services. SoftBank, which is a Tokyo-based phone and Internet service provider, has recently invested $100 million in the cloud robot Pepper created by Aldebaran Robotics. In June 2015, SoftBank Robotics announced that the first 1000 units of this robot were sold out in less than 1 minute (Guizzo, Reference Guizzo2011).
In particular, CRS are characterized by three levels of implementation (Zhang & Zhang, Reference Zhang and Zhang2019): (i) the infrastructure as a service (Du et al., Reference Du, Yang, Chen, Sun, Wang and Xu2011) to run, for example, the operating systems on the cloud; (ii) the platform as a service to share, for example, data (Tenorth et al., Reference Tenorth, Kamei, Satake, Miyashita and Hagita2013), system architectures (Miratabzadeh et al., Reference Miratabzadeh, Gallardo, Gamez, Haradi, Puthussery, Rad and Jamshidi2016), etc.; and (iii) the software as a service (Arumugam et al., Reference Arumugam, Enti, Bingbing, Xiaojun, Baskaran, Kong, Kumar, Meng and Kit2010) to allow, for example, the process and the communication of the data. Hence, CRS use the cloud for robotic systems to share their data about the context (Huang et al., Reference Huang, Lee and Lin2019), the environment (Wang et al., Reference Wang, Krishnamachari and Ayanian2015), the resources (Chen et al., Reference Chen, Li, Deng and Li2018), their formation (Turnbull & Samanta, Reference Turnbull and Samanta2013), their navigation (Hu et al., Reference Hu, Tay and Wen2012), or to perform tasks such as the simultaneous localization and mapping (SLAM) (Benavidez et al., Reference Benavidez, Muppidi, Rad, Prevost, Jamshidi and Brown2015), the grasping of objects (Zhang et al., Reference Zhang, Li, Nicho, Ripperger, Fumagalli and Veeraraghavan2019), leading to define path planning (Lam & Lam, Reference Lam and Lam2014) or robot control as services (Vick et al., Reference Vick, Vonasek, Penicka and Kruger2015), and more generally, robotic as a service (Doriya et al., Reference Doriya, Chakraborty and Nandi2012).
Thence, by studying the context of the CR field, its importance, and the need for standards that can support its growth, this paper brings a contribution in identifying and testing ontologies that aim to define and facilitate access to various types of knowledge representation and shared resources for the CRS.
The rest of the paper is structured as follows. Section 2 presents existing standard ontologies for the CR domain, along with a literature overview about relevant efforts in the CRS field. Section 3 describes a real-world case study providing an insight into the use of the presented ontologies to enhance information sharing in a CRS, while Section 4 concludes the work with reflections and future directions.
2 Ontologies for cloud robotic systems
2.1 Overview
In light of the need for standardization of the shared data among the CRS’ agents, the use of ontologies plays an important role (Kitamura & Mizoguchi, Reference Kitamura and Mizoguchi2003). Indeed, ontology specifies both formally and semantically the key concepts, properties, relationships, and axioms of a given domain (Olszewska & Allison, Reference Olszewska and Allison2018). In general, ontologies make the relevant knowledge about a domain explicit in a computer-interpretable format, allowing reasoning about that knowledge to infer new information. Some of the benefits of using ontology are thus the definition of a standard set of domain concepts along with their attributes and inter-relations, and the possibility for knowledge capture and reuse, facilitating systems specification, design and integration as well as accelerating research in the field (Olszewska et al., Reference Olszewska, Houghtaling, Goncalves, Fabiano, Haidegger, Carbonera, Patterson, Ragavan, Fiorini and Prestes2020).
Over the past, there have been several projects focusing on the use of ontologies for CR applications, in order to express the vocabulary and knowledge acquired by robots in specific context such as bioinformatics (Soldatova et al., Reference Soldatova, Clare, Sparkes and King2006), rehabilitation (Dogmus et al., Reference Dogmus, Erdem and Patoglu2015), or kit building (Balakirsky et al., Reference Balakirsky, Kootbally, Schlenoff, Kramer and Gupta2012). Indeed, through ontologies, robots are able to use a common vocabulary to represent their knowledge in a structured, unambiguous form and perform reasoning over these data (Muhayyuddin & Rosell, Reference Muhayyuddin and Rosell2015). However, the growing complexity of behaviors that robots are expected to present naturally entails, on one hand, the use of increasingly complex knowledge, calling for an ontological approach and, on the other hand, the need for multi-robot coordination and collaboration, requiring a standardized approach.
Thence, the remaining sections (Sections 2.2 and 2.3) are focused on two ontologies, namely the Ontology for Autonomous Systems (OASys) and the Robotic Cloud Ontology (ROCO), which are part of the IEEE standardization effort dedicated to Robot Task Representation (RTR) (P1872.1) and the Standard for Autonomous Robotics (AuR) (P1872.2), respectively. As explained in Section 3, these OASys and ROCO standard ontologies can be extended to the CR domain and applied in CRS.
2.2 Ontology for autonomous systems ontology
The OASys (Bermejo-Alonso & Sanz, Reference Bermejo-Alonso and Sanz2011) describes the domain of autonomous systems as software and semantic support for the conceptual modeling of autonomous system’s description (ASys Ontology) and engineering (ASys Engineering Ontology) (Figure 1) and could be used for CR. Indeed, OASys organizes its content in the form of different subontologies and packages. Subontologies address the intended use for autonomous systems’ description and engineering at different levels of abstraction, whereas packages within a subontology gather aspect-related ontological elements. Both subontologies and packages have been designed to allow future extensions and updates to OASys. The different subontologies and packages have been formalized using Unified Modeling Language (UML) (Olszewska et al., Reference Olszewska, Simpson and McCluskey2014).

Figure 1 OASys ontologies, subontologies, and packages
The ASys Ontology gathers the ontological constructs necessary to describe and characterize an autonomous system at different levels of abstraction, in two subontologies, as follows:
1. The System Subontology contains the elements necessary to define the structural and behavioral features of any system.
2. The AsysSubontology specializes the previous concepts for autonomous systems, consisting of several packages to address their structure, behavior, and function.
The ASys Engineering Ontology is dedicated to system’s engineering concepts. It comprises two subontologies to address at a different level of abstraction the ontological elements to describe the autonomous system’s engineering development process, as follows:
1. The System Engineering Subontology gathers the concepts related to an engineering process as general as possible, based on different metamodels, specifications, and glossaries used for software-based developments.
2. The ASys Engineering Subontology contains the specialization and additional ontological elements to describe an autonomous system’s generic engineering process, organized in different packages.
The OASys-driven Engineering Methodology (ODEM) guides the application of the OASys elements for the semantic modeling of an autonomous system considering the phases, the tasks, and the work products (as conceptual models and additional documents). It uses as guideline the ontological elements in the System Engineering and ASys Engineering Subontologies. ODEM also specifies the OASys packages to be used in each of the phases of the engineering process (Bermejo-Alonso & Sanz, Reference Bermejo-Alonso and Sanz2011).
2.3 Robotic cloud ontology
The ROCO is based on the robot architecture ontology (ROA) (Olszewska et al., Reference Olszewska2017). The ROA ontology, developed by the IEEE Robotics and Automation Society (RAS) AuR Study Group (Fiorini et al., Reference Fiorini, Bermejo-Alonso, Goncalves, Pignaton de Freitas, Olivares Alarcos, Olszewska, Prestes, Schlenoff, Ragavan, Redfield, Spencer and Li2017) as part of the IEEE-RAS ontology standard for AuR (Bermejo-Alonso et al., Reference Bermejo-Alonso, Chibani, Goncalves, Li, Jordan, Olivares, Olszewska, Prestes, Fiorini and Sanz2018), defines the main concepts and relations regarding robot architecture for autonomous systems and inherits from SUMO/CORA ontologies (Prestes et al., Reference Prestes, Carbonera, Fiorini, Jorge, Abel, Madhavan, Locoro, Goncalves, Barreto, Habib, Chibani, Gerard, Amirat and Schlenoff2013). In particular, essential concepts for effective intra-robot communication (Doriya et al., Reference Doriya, Mishra and Gupta2015) such as behavior, function, goal, and task have been defined in ROA (Olszewska et al., Reference Olszewska2017). Thence, ROCO ontological framework intends to apply the ROA ontology to CRS by extending ROA concepts to the CR domain (Figure 2), in order to enhance information sharing in CRS.

Figure 2 Excerpt of the cloud robotic ontology concepts implemented in OWL language
The ROCO ontology has been implemented in the web ontology language (OWL) (W3C OWL Working Group, 2009), which is well-established for knowledge-based applications (Olszewska et al., Reference Olszewska2017; Beetz et al., Reference Beetz, Bessler, Haidu, Pomarlan, Bozcuoglu and Bartels2018), using the Protégé tool and following the robotic ontological standard development life cycle (ROSADev) methodological approach (Olszewska et al., Reference Olszewska, Houghtaling, Goncalves, Haidegger, Fabiano, Carbonera, Fiorini and Prestes2018).
3 Cloud robotic case study
3.1 Cloud robotic context
The studied system is an interactive, heterogeneous, decentralized multi-robot system (Calzado et al., Reference Calzado, Lindsay, Chen, Samuels and Olszewska2018) that is composed of a set of unmanned aerial vehicles (UAVs) and unmanned ground vehicles (UGVs) that belong to different organizations, such as Regional Police, Federal Police, Firefighters, Civilian Defense, and Metropolitan Guard.
These organizations have their own UAVs and/or UGVs to support them individually in different application scenarios. These robots can share data inside their organizations through private cloud services. The robots used by these different organizations could have different characteristics, depending on the organizations’ needs. For instance, multi-rotor UAVs, such as quadcopters or hexacopters, can offer Metropolitan Guard surveillance of specific buildings or public areas, while UGVs from the Federal Police can access places, which are potentially under threat by terrorist attacks, in order to disarm bombs.
The convergence of efforts from different law enforcement organizations can better assist the population against the threats posed by criminals and outlaws. The previously mentioned UAVs and UGVs can form a robotic cloud (Mell & Grance, Reference Mell and Grance2011) to share data about the environment under concern, to consume data, to use resources to perform computation intensive tasks, and to offer their resources to other members of this cloud. As different organizations are sharing the same robotic cloud, it is possible to state that this is a CRS.
A service that the cloud can provide to the UAVs and UGVs composing this CRS is motion planning. As stated above, there are different types of UAVs and UGVs (e.g., fixed-wing, multi-rotors of different types, aerostats, ground robots with or without manipulators, etc.). Depending on the environment data that are uploaded to the cloud, different forms of event assistance services are needed in different areas. Whatever the required actions to be performed, obstacles may hinder the usage of given UAV or UGV to perform a given task or make one more appropriate than another. Upon the decision about which UAV or UGV should perform a given task, there is a need to execute its motion planning, in order the robot can move to the area in which it is needed. Moreover, it is also important to specify the tasks which have to be performed (Bruckner et al., Reference Bruckner, Picus, Velik, Herzner and Zucker2012).
For this heterogeneous system composed of different types of UAVs and UGVs with different capabilities, different sensors, and different actuators, semantic coherent definitions of the involved concepts have to be provided in order to ensure efficient communication in between the agents (Olszewska, Reference Olszewska2017). Indeed, the semantic coherence of these definitions is important so that the required tasks can be assigned to any UAV or UGV that may be able to perform them, regardless it specific type. In particular, the CR-based system, which owns the need for clear semantic processing of tasks allocation in context of this surveillance application (as described in Section 3.2), can benefit from a standard ontology (as illustrated in Sections 3.3–3.4).
3.2 Cloud robotic scenario
As a real-world example of this overall CRC system architecture, we consider that two UGVs (i.e., a wheeled robot with a surveillance camera and a gripper (UGV-1) and a wheeled robot with only a surveillance camera (UGV-2)) are used for surveillance by Neighborhood Patrol Division of the Metropolitan Guard, and that two UAVs (i.e., a conventional helicopter (UAV-1) and a quadrotor (UAV-2)) are used for patrolling operations by the Highway Patrol Division of the Regional Police. All these vehicles use cloud computing facilities to process data and/or computation intensive algorithms such as SLAM/cooperative SLAM, sampling-based planning, or planning under uncertainty, since it is much faster than relying on their own onboard computers.
On the other hand, all these UGVs and UAVs evolve in an environment, as illustrated in Figure 3, that is, with nine volumes of interest (VOIs). These VOIs consist of six areas of interest (AOIs), two highways (HWs), and a base station (BS). In particular, AOI-1 is a rural area, AOI-2 is an industrial zone, AOI-3 is a sport complex, AOI-4 is a residential area, AOI-5 is a service area, and AOI-6 represents a gas station and its surroundings.
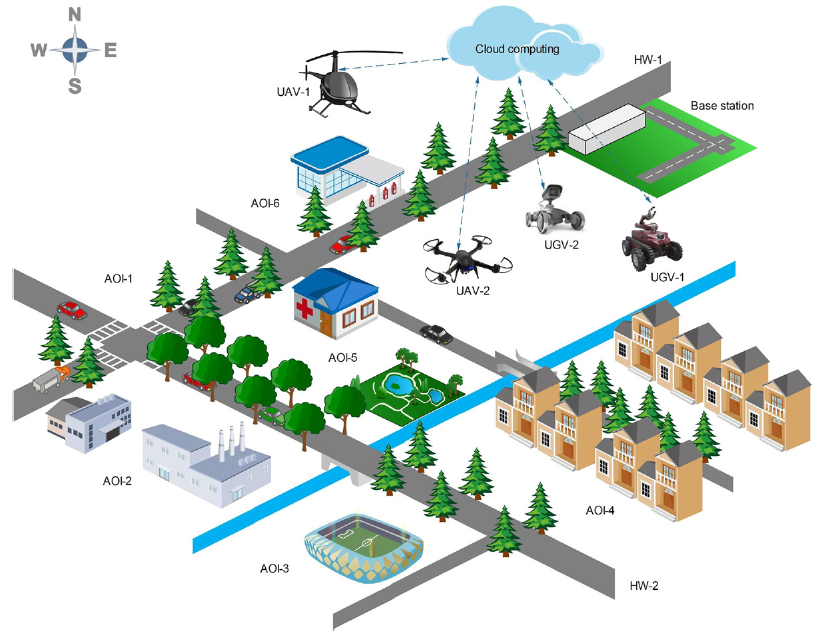
Figure 3 Cloud robotic scenario environment with unmanned aerial vehicles (UAVs) and unmanned ground vehicles (UGVs)
To perform daily routine surveillance and patrolling operations, it is required in the scenario to deploy these vehicles starting from the BS of the Regional Police and then to bring them back to this BS. It is assumed that the VOI of each vehicle is pre-assigned as follows: AOI-1, AOI-6, and HW-1 are assigned to UAV-1; AOI-4, AOI-5, and HW-2 are assigned to UAV-2; AOI-2 is assigned to UGV-1; and AOI-3 is assigned to UGV-2.
Part of this deployment mission is to plan the motion of each vehicle from the BS to a certain vantage point in the pre-assigned VOI and vice versa. In this planning problem, the goal is to determine a future course of actions/activities for an executing entity (UAV/UGV) to drive it from an initial state (BS) to a specified goal state (VOI). It is supposed that at the beginning of the scenario, all UAVs and UGVs are in the BS, and all AOIs and HWs are not under surveillance/patrolling. Besides, it is assumed that the CRS system must be able to provide ground robots with a safe manner to reach their destination AOIs, as they are not able to have the same overview as the UAVs. Indeed, UGV-1 has to go through AOI-5 and HW-2 to reach AOI-2, while UGV-2 has to go through AOI-4 and HW-2 to reach AOI-3.
Considering the cooperative manner a CRS operates, UGV-1 and UGV-2 can request to the cloud a service for clearance about these places that they have to go through, in order they are able to make a safe move towards their destination AOIs. Thence, this request for clearance triggers a query to all robots connected to the cloud, and thus, a request capability match takes place. This matching has to consider both the necessary capabilities related to surveillance areas and the situation or context. In this case, it consists in matches between what the robots are able to do and about where the information is required (i.e., AOI-4, AOI-5, and HW-2).
According to what is stated in the presented scenario, UAV-2 has the necessary capabilities to provide the requested information, and for this, it must behave in a way to perform the actions of moving to AOI-4, collecting an image of AOI-4, then moving to AOI-5, capturing an image of this area and next, moving to HW-2, and collecting an image of this place. Afterwards, it has to share within the cloud structure the requested data which will be used by the robots that demanded the clearance over the areas.
3.3 Ontology for autonomous systems ontology implementation
The case study presented in Section 3.2 is analyzed in this subsection focusing on the application of the proposed OASys ontological framework (Section 2.2) in a cloud-based robotic system that has the goal to provide law enforcement services (Section 3.1).
It is worth noting that ontological terms are written in this section as usual to ease the reading process, whereas they are named as a unique word capitalizing each word within it as made in the naming convention in OASys. Italics are used for the case study scenario components specified in Section 3.2.
Given the fact that the proposed case study is a multi-agent system scenario, it is possible to use multi-agent planning Language or MA-PDDL (multi-agent planning domain definition language) as extensions of the PDDL (McDermott et al., Reference McDermott, Ghallab, Howe, Knoblock, Ram, Veloso, Weld and Wilkins1998) in multi-agent scenarios. MA-PDDL (Kovacs, Reference Kovacs2012) provides the ability to represent different actions of different agents or different capabilities, different goals of the agents and actions with interacting effects between the agents such as cooperation, joint actions, and constructive synergy.
For the sake of simplicity, it is assumed that there are no cooperative behaviors/joint actions between the vehicles in the proposed scenario, and then, PDDL can be used. Objects, predicates, initial state, goal specification, and actions/operators are the main components of a PDDL planning task. Indeed, objects represent things in the world that interest us. Predicates are properties of objects that are of interest to be studied; they can be true or false. Initial state is the state of the world that the system starts in. Goal specification represents things that is desirable to be true, and finally actions/operators are ways of changing the state of the world or capabilities.
In the proposed scenario, it is possible to extract the following examples of these five components:
1. Objects: The two UAVs, the two UGVs, the nine VOIs. (At lower level of abstraction for each vehicle, it is possible to consider planning entity, planned/execution entity, monitoring entity, motion planning solver, and planning environments as objects.)
2. Predicates: Is VOI fully observable? Is VOI structured? Is VOI adversarial? Is VOI under surveillance by UAV/UGV? Is HW patrolled by UAV? Is UGV-2 within AOI-5?
3. Initial state: All UAVs and UGVs are in the BS. All AOI and HWs are not under surveillance/patrolling.
4. Goal specification: UAV-1 is in AOI-1/AOI-6/HW-1; UAV-2 is in AOI-2/AOI-3/HW-2; AGV-1 is within AOI-4, and AGV-2 is within AOI-5.
5. Actions and operators: Each vehicle can execute same/different motion plan shared via the cloud to move from the BS to the AOI/HWs and vice versa. It can avoid stationary and/or moving obstacles while executing the plan, or it can continuously monitor the execution of the plan and it can adapt/repair the plan to resolve conflicts, if any.
For example, we assume the plan execution case as follows:
1. Objects: VOI: AOI-1, AOI-2, AOI-3, AOI-4, AOI-5, AOI-6, HW-1, HW-2, and Base-Station Vehicles: UGV-1, UGV-2, UAV-1, UAV-2
In PDDL:
AOI-6,
1 (:objects AOI-1, AOI-2, AOI-3, AOI-4, AOI-5, AOI-6,
2) UGV-1, UGV-2, UAV-1, UAV-2)
2. Predicates: VOI(x) true iff x is a AOI/HW
Vehicle(x) true iff x is a UGV/UAV
at-Base-Veh(x) true iff x is a Base-Station and a Vehicle is in x
at-VOI-Vehicle(x,y) true iff x is a VOI and VOI Base-Station and a Vehicle y is in x
In PDDL:
(:predicates (VOI ?x) (Vehicle ?x)
(at-Base-Veh ?x) (at-AOI- UGV ?x)
(at-AOI- UAV ?x) (at-HW- UAV ?x))
3. Initial state: VOI(Base-Station) is true. Vehicle(UGV-1), Vehicle(UGV-2), Vehicle(UAV-1), Vehicle(UAV-2) are true. at-Base-Veh(UGV-1), at-Base-Veh(UGV-2), at-Base- Veh(UAV-1), at-Base-Veh(UAV-1) are true. Everything else is false.
In PDDL:
(:init (VOI Base-Station) (VOI AOI-1) (VOI AOI-2) (VOI AOI-3) (VOI AOI-4) (VOI AOI-5) (VOI AOI-6) (VOI HW-1) (VOI HW-2) (Vehicle UGV-1), (Vehicle UGV-2), (Vehicle UAV-1), (Vehicle UAV-2) (at-Base-Veh UGV-1), (at-Base-Veh UGV-2), (at-Base-Veh UAV-1), (at-Base-Veh UAV-2))
4. Goal specification: at-VOI-Vehicle(AOI-1, UAV-1), at-VOI-Vehicle(AOI-6, UAV-1), at-VOI-Vehicle(HW-1, UAV-1), at-VOI-Vehicle(AOI-2, UAV-2), at-VOI-Vehicle(AOI-3, UAV-2), at-VOI-Vehicle(HW-2, UAV-2), at-VOI-Vehicle(AOI-4, UGV-1), at-VOI-Vehicle(AOI-5, UGV-2). Everything else we do not care about.
In PDDL:
(:goal (and (at-VOI-Vehicle AOI-1 UAV-1)
(at-VOI-Vehicle AOI-1 UAV-1)
(at-VOI-Vehicle AOI-6 UAV-1)
(at-VOI-Vehicle HW-1 UAV-1)
(at-VOI-Vehicle AOI-2 UAV-2)
(at-VOI-Vehicle AOI-3 UAV-2)
(at-VOI-Vehicle HW-1 UAV-2)
(at-VOI-Vehicle AOI-4 UGV-1)
(at-VOI-Vehicle AOI-5 UGV-2)))
5. Action and operators:
Description: The vehicle can execute motion plan available on the cloud to move from the BS and between the AOI/HWs, can avoid stationary and/or moving obstacles, can monitor the execution of the plan, and can adapt/repair the plan to resolve conflicts. For the sake of simplicity, assume that a vehicle y moves from a to b.
Precondition: VOI(a), VOI(b), and at-VOI-Vehicle(a,y) are true.
Effect: at-VOI-Vehicle(b,y) becomes true. at-VOI-Vehicle(a,y) becomes false. Everything else does not change.
In PDDL:
(:action move :parameters (?a ?b)
:precondition (and (VOI ?a) (VOI ?b)
(at-VOI-Vehicle ?a))
:effect (and (at-VOI-Vehicle ?b)
(not (at-VOI-Vehicle ?a))))
Considering the OASys-driven ODEM, its analysis phase involves different tasks performed for the structural, behavioral, and functional analysis of the system. As such, the structural analysis task models the system structure and knowledge (Figure 4). The system modeling subtask models the structural elements in the system and their relationships, obtaining a structural model, based on the ontological elements in the system subontology. OASys allows to model the abstract knowledge to be used in the system. Later on, the motion planning process uses the instantiated knowledge for the particular system under specification.

Figure 4 Structural analysis task defined in ASys engineering subontology
In this sense, Figure 5 shows the structure model specializing OASys system subontology abstract knowledge classes (system, subsystem, and element) and their composition relationships using a UML class diagram notation. The bold horizontal lines distinguish the different level of abstraction, that is, any system concepts at the upper level, the CRC system concepts at the intermediate level as specialization through isA relationships, and the actual Objects in the Case Study as instances of the previous classes through instanceOf relationships (not all shown to avoid cluttering the diagram). This structure model is complemented with the topology models to show the topological and mereological relationships. These models allow representing the predicates specifying which specific vehicle surveys, patrols, or is within the limits of a concrete VOI.
The role played by this ontological-based structural model is twofold. Firstly, it allows the different elements in the CRC system to know about the different, involved vehicles and VOIs and the topological as well as mereological relationships among them for a further reasoning on the motion planning tasks. Secondly, the models can also be used as a semantic system specification for a Model-based Systems Engineering process.

Figure 5 Structure model specializing concepts for the case study
In a similar way, the knowledge modeling subtask obtains a knowledge model to be used by the system. In this case, the main knowledge to model is the planning-related concepts about initial state and the goal specification, in a goal structure model. Figure 6 shows how the abstract knowledge is conceptualized in OASys. As illustrated in this figure, concepts such as Goal, RootGoal, SubGoal, and LocalGoal are specialized for the CRC system at the intermediate level, while UML classes are used to specify generic vehicle goals. Later on, the actual objects for the case study become instances of the former classes, as shown in the UML object diagram, with attributes and operations to define the case study’s specific goals to be fulfilled by the vehicle (i.e., AGVs and UAVs) in relation to the VOI (i.e., AOI, HW, and BS). This semantic model constitutes the first kind of knowledge for the CRC system to make decisions during the motion planning reasoning. Other conceptual models using ODEM (Bermejo-Alonso et al., Reference Bermejo-Alonso, Hernandez and Sanz2016) can be obtained in a similar way in order to specify the system behavior in terms of the motion planning actions (e.g., avoid obstacles, resolve conflicts, etc.), as shown in different UML activity and sequence diagrams by specializing OASys ontological concepts and relationships.

Figure 6 Goal structure model as result of knowledge modeling task
Hence, the first qualitative results of OASys have been presented in Figures 4–6 in context of this CRS surveillance application. As a first attempt to characterize the CRS domain, it provides enough support to conceptualize this case study. Currently, OASys is under revision to be implemented using OWL and reasoning mechanism to enhance both the characterization of the robotic systems (Balakirsky et al., Reference Balakirsky, Schlenoff, Fiorini, Redfield, Barreto, Nakawala, Carbonera, Soldatova, Bermejo-Alonso, Maikore, Goncalves, De Momi, Sampath Kumar and Haidegger2017) and the metacontrol features (Hernandez et al., Reference Hernandez, Bermejo-Alonso and Sanz2018). In particular, OASys is considered for the standardization efforts under development by the IEEE P1872.1 working group focused on RTR.
3.4 Robotic cloud ontology implementation
The ROCO ontological framework introduced in Section 2.3 represents robots’ actions, functions, behaviors, and capabilities using the standard OWL language as demonstrated in context of the scenario described in Section 3.2.
The actual objects present in the case study are instances of the proposed CRS ontological concepts and are related by the defined axioms.
Particularly, the agents UGV1 (Figure 7) and UGV2 (Figure 8), which are both requiring clearance from UAV2 (Figure 9), are considered, since they navigate through the same VOIs; all these agents are being part of the same CRS, as implemented in OWL (Figure 10) and displayed in Figure 11. Therefore, UGV1 and UGV2 could use the CRS as a resource to request clearance. This has been implemented in OWL by means of the isRequestingClearance property, as illustrated in Figure 12.

Figure 7 Snapshot of the UGV1 instance of Agent. Yellow rows are automatically inferred by the reasoner. Best viewed in color

Figure 8 Snapshot of the UGV2 instance of Agent. Yellow rows are automatically inferred by the reasoner. Best viewed in color

Figure 9 Snapshot of the UAV2 instance of Agent. Yellow rows are automatically inferred by the reasoner. Best viewed in color

Figure 10 Snapshot of the CRS instances implemented in OWL

Figure 11 Snapshot of the CRS instance of CloudRobotic System. Yellow rows are automatically inferred by the reasoner. Best viewed in color

Figure 12 Snapshot of the isRequestingClearance property implemented in OWL
Hence, the first qualitative results of ROCO have been presented in Figures 7–15 in context of this CRS surveillance application. To sum up, the role played by this ontological-based CR model is twofold. Firstly, it allows the different elements in the CRS to know which agents (i.e., vehicles) are involved in and what are their positions (i.e., VOIs) as well as the topological and mereological relationships among them, leading to further reasoning on their motion planning (Figures 13–15). On the other hand, this approach is useful to establish a common shared vocabulary to enhance CRS agents’ communication. Thereupon, ROCO is considered for the standardization efforts under development by the IEEE P1872.2 working group focused on AuR.

Figure 13 Snapshot of the reasoning on the Action concept in context of the CRS case study

Figure 14 Snapshot of the reasoning on the Capability concept in context of the CRS case study

Figure 15 Snapshot of the reasoning on the Behavior concept in context of the CRS case study
3.5 Discussion
OASys is an ontology that comprises both autonomous systems description and its engineering process as mentioned in Section 2.2. The upper level regarding a general system is well founded, based on former standard and proved theories. Thence, the autonomous system level, both from a description and an engineering view, would benefit of the current standardization efforts by IEEE, for example, in its P1872.1 Task Representation Ontology project. Indeed, the action package in the ASys Subontology of OASys is an initial attempt to characterize goals, tasks, and functions in autonomous systems, which are aligned with the focus of the IEEE P1872.1 under-progress standard and which are useful for CRS.
The ROCO ontology in turn follows the METHONTOLOGY ontological methodology approach to characterize autonomous systems, and in particular to contribute to the IEEE P1872.2 ontology under development by the Autonomous Systems Working Group, as pointed out in Section 2.3. Thus, ROCO inherits from the autonomous ROA which was developed in the context of the IEEE P1782.2 Autonomous Robotic effort, while it has a greater focus on CR. Indeed, ROCO considers concepts related to behavior, function, task, etc. in order to specifically formalize the CR domain.
Hence, both ontologies contribute towards the current standardization efforts by the IEEE, regarding robotics and autonomous systems, and in particular CRS specific concepts and relations. It is worth noting that OASys was developed using a systems engineering language called UML, which is considered to be a lightweight language without an embedded reasoning mechanism. Consequently, the reasoning on OASys has to be processed externally, for example, on a PDDL platform as explained in Section 3.3. Unlike OASyS, the ROCO ontology has been directly developed in OWL, which is a computational logic-based language such that knowledge expressed in OWL can be exploited by computer programs and automated reasoners, for example, to verify the consistency of that knowledge or to make implicit knowledge explicit, as described in Section 3.4.
Therefore, OASys ontological engineering has served as a general systems approach and a common taxonomic basis for the IEEE P1872 ontologies in development, while the ROCO ontology has allowed to specialize the standardized ontologies to include CR-specific concepts and relations which automated reasoning can be directly performed on.
4 Conclusions
CRS alloy cloud computing and autonomous multi-robot systems. These technologies require an adequate understanding and a coherent sharing of the resources and data among the CRS agents in order to make possible an interoperable communication and efficient collaboration between the CRS stakeholders.
For this purpose, ontologies have been identified as a possible solution. Thence, this paper presents the current state of standard ontologies for the CR domain. Moreover, a case study showcases the implementation of the described ontologies, namely the OASys and the ROCO, into a real-world motion planning CRS scenario, highlighting the main features of these ontologies in the CR context and reporting the results obtained when using the defined ontologies to enhance the interoperability of CRS.
Future works aim to enrich the vocabulary developed so far in the existing standard ontologies and create further semantic extensions that will contribute to the ontological standard which is being developed by the IEEE Ontologies for Robotics and Automation (ORA) Working Group and its RTR and AuR subgroups.