Introduction
Rapid population growth, climate uncertainty and food insecurity are key challenges facing smallholder farmers in sub-Saharan Africa (Mpande and Adziwa, Reference Mpande and Adziwa2011; Kassie et al., Reference Kassie, Erenstein, Mwangi, Larovere, Setimela and Langyintuo2012). Population growth and rapid urbanization have substantially increased the demand for crop and livestock products (Homann and Van Rooyen, Reference Homann, Van Rooyen, Nambiro, Omare and Nkamleu2007; Subhadra, Reference Subhadra2007), and is projected to increase by as much as 70% by 2050 (Soussana, Reference Soussana2015). However, this demand might not be met because of the chronically poor crop and livestock production practices of smallholder farming systems in the region (Sánchez, Reference Sánchez2010; Umar et al., Reference Umar, Aune, Johnsen and Lungu2011; Nkomboni et al., Reference Nkomboni, Sisito, Van Rooyen, Homann-Kee Tui, Sikosana and Ndlovu2014).
Mixed crop–livestock production is the most practiced form of agriculture in smallholder farming communities of Zimbabwe (Mashingaidze et al., Reference Mashingaidze, Madakadze, Twomlow, Nyamangara and Hove2012). The actual choice of crop–livestock activity combinations in a mixed farming system is dependent on local socio-economic and agro-ecological conditions such as soil, rainfall and temperature (Davendra, Reference Davendra2002). Household dynamics, farmer ingenuity, input and output market prices, political stability and technological developments also influence the choice and components of mixed farming systems (Schiere and Kater, Reference Schiere and Kater2001; Kindu et al., Reference Kindu, Duncan, Valbuena, Gerard, Dagnachew, Mesfin, Gedion, Vanlauwe, van Asten and Blomme2014).
Mixed crop–livestock farming systems lead to diversification which improves farm productivity, minimize production and economic risks, and buffering against climate variability (Van Keulen and Schiere, Reference Van Keulen and Schiere2004). The livestock component acts as alternative security, savings and investment options in smallholder farming systems (Kurosaki, Reference Kurosaki1997). Crop–livestock integration has the potential to improve sustainable intensification of crop and livestock productivity (Sempore et al., Reference Sempore, Andrieu, Le, Nacro and Sedogo2016). Crop–livestock integration involves intensifying the interaction between crops and livestock in a single farming unit in order to improve the combined productivity of the individual components of the system and minimize use of external inputs (Mohammed-Saleem, Reference Mohammed-Saleem, Wilson, Ehui and Mack1995; Williams et al., Reference Williams, Hiernaux, Fernández-rivera and McCarthy1999). Crop–livestock integration could be one of the first steps to improve sustainability of smallholder mixed farming systems, which have been consistently plagued by low productivity and profitability (Wolmer, Reference Wolmer1997). It also minimizes crop production costs through use of manure and draught power. Use of crop residues improves livestock condition during drier seasons (Sempore et al., Reference Sempore, Andrieu, Le, Nacro and Sedogo2016). Smallholder farmers have always practised some form of mixed farming and the systems have been poorly integrated. Low integration has been attributed to increased land pressure, poor markets, limited ease of practice and low transfers between crops and livestock (Blackburn, Reference Blackburn1998). Such farming systems also demand more labor in manure handling, initial capital investment and have less economies of scale. They also potentially lead to conflicts, competition for resources and land degradation if poorly managed (Van Keulen and Schiere, Reference Van Keulen and Schiere2004). Large-scale commercial farmers do not use mixed farming systems due to the longer ‘gestation period’ of the benefits and due to specialization (Sempore et al., Reference Sempore, Andrieu, Le, Nacro and Sedogo2016).
Irrespective of their huge potential, mixed farming systems often suffer from recurrent low productivity due to competition for resources and the need to couple knowledge on both crop and livestock which, in most cases, is difficult for a farmer to do on their own (Blackburn, Reference Blackburn1998; Kindu et al., Reference Kindu, Duncan, Valbuena, Gerard, Dagnachew, Mesfin, Gedion, Vanlauwe, van Asten and Blomme2014). It can be argued that the smallholder farming systems have not benefitted from crop–livestock integration. The major challenge being lack of demonstrated knowledge and potential of crop–livestock integration in smallholder setups in understandable and measurable form such as monetary value. However, demonstration of the benefits of crop–livestock integration is often limited by the cost of on-farm demonstrations and experimentation. There is a need for a cost-effective method to demonstrate the benefits of crop–livestock integration in smallholder systems (Wolmer, Reference Wolmer1997).
The Food and Agriculture Organization (FAO) has described integrated farming systems as systems where crop and livestock are highly dependant on each other and products or by-products of each of the components are inputs of the other (FAO, 2001). Sempore et al. (Reference Sempore, Andrieu, Le, Nacro and Sedogo2016) similarly suggest that integration involves the transfer of products between crop and livestock within the same land area. This involves, for example, draught power for cultivation, fodder from crop residues and fodder crops and organic manure from livestock waste and crop residues (Sempore et al., Reference Sempore, Andrieu, Le, Nacro and Sedogo2016). According to Parsons et al. (Reference Parsons, Nicholson, Blake, Ketterings, Ramírez-aviles, Fox, Tedeschi and Cherney2011), this also involves feeding of animals with at least 10% of crop residues and maximization of the use of animal products in crop production and vice versa (Chipunza et al., Reference Chipunza, Mutibvu, Kashangura and Mbiriri2013). Wolmer (Reference Wolmer1997) highlights the potential to assess crop–livestock on the basis of the energy and nutrients interchanged between crop and livstock systems. Research on crop livestock integration in West Africa has led to an increase in the amount of crop residues fed to livestock (Sempore et al., Reference Sempore, Andrieu, Le, Nacro and Sedogo2016). Studies of crop–livestock integration in smallholder dairy farming and beef systems have indicated positive results, with the assertion that marketing is important for the uptake and enhanced impact of integrated crop–livestock integration strategies in smallholder systems (Gwiriri et al., Reference Gwiriri, Manyawu, Mashanda, Chakoma, Moyo, Chakoma, Sethaunyane, Imbayarwo-Chikosi, Dube and Maasdorp2016). Despite these studies, adoption of crop–livestock integration strategies is still low, which has been attributed to lack of clear demonstration of the benefits of appropriate crop–livestock integration. Low adoption has also been due to the assumption that smallholder farmers are a homogeneous group, hence blanket recommendation of crop–livestock integration strategies (Sempore et al., Reference Sempore, Andrieu, Le, Nacro and Sedogo2016).
The challenges facing smallholder farmers call for urgent multi-disciplinary strategies to increase the productivity and profitability options associated with integrated crop–livestock systems (Blackburn, 1995). Due to the different abilities of farmers to sustainably use technologies, categorizing farming households increases our understanding of the diversity and homogeneity of the smallholder farmer population. Use of farmer typology profiling is essential in disaggregating and understanding the diversity among smallholder farmers (Chikowo et al., Reference Chikowo, Zingore, Snapp and Johnston2014). This is a key in the development of banded policies and the development of corresponding solutions to their farming challenges (Tshoni, Reference Tshoni2015). Compared with the zoning technique, the typology approach provides a practical method of capturing farmer diversity and farming systems producing qualitative outputs for evaluation of developmental pathways and policy formulation. This reduces the tendency to offer blanket recommendations to challenges facing smallholder farmers and it increases the potential for the adoption of recommended interventions (Chikowo et al., Reference Chikowo, Zingore, Snapp and Johnston2014). In each case of crop–livestock integration, synergism can be further increased by adoption of enterprise-specific interventions that favor the performance of the system's component enterprises.
These assertions could be verified through use of traditional on-farm trials which have been widely used to provide agronomic solutions to challenges facing farmers (Maat, Reference Maat2011). The use of on-farm field trials is however usually expensive, time consuming and complex in most instances (Jones et al., Reference Jones, Hoogenboom, Porter, Boote, Batchelor, Hunt, Wilkens, Singh, Gijsman and Ritchie2003). The use of simulation models is relatively easier, less time consuming and practical in assessing crop–livestock integration options among smallholder farmers. Models like the Agricultural Production Simulator (APSIM) (Holzworth et al., Reference Holzworth, Huth, DeVoil, Zurcher, Herrmann, McLean, Chenu, van Oosterom, Snow, Murphy, Moore, Brown, Whish, Verrall, Fainges, Bell, Peake, Poulton, Hochman, Thorburn, Gaydon, Dalgliesh, Rodriguez, Cox, Chapman, Doherty, Teixeira, Sharp, Cichota, Vogeler, Li, Wang, Hammer, Robertson, Dimes, Whitbread, Hunt, van Rees, McClelland, Carberry, Hargreaves, MacLeod, McDonald, Harsdorf, Wedgwood and Keating2014), Integrated Analysis Tool (IAT) (Lisson et al., Reference Lisson, MacLeod, McDonald, Corfield, Pengelly, Wirajaswadi, Rahman, Bahar, Padjung, Razak, Puspadi, Dahlanuddin, Saenong, Panjaitan, Hadiawati, Ash and Brennan2010) and APSFARM have been extensively used in cropping systems (Masikati et al., Reference Masikati, Manschadi, Van Rooyen and Hargreaves2014), household and multi-disciplinary socio-economic modeling (de Voil et al., Reference de Voil, Rodriguez, Power and Rossing2009).
The current study sought to use simulation models to assess the effect of improved crop and livestock production technologies on crop and livestock productivity, profitability and integration in smallholder farming systems under sub humid conditions of Zimbabwe. We hypothesized that if smallholder farmers use improved agronomic and livestock husbandry practices, crop and livestock product yields will increase, resulting in higher household productivity, profitability and integration. Specific objectives were to (a) assess the prevailing levels of crop and livestock integration in Murehwa and Goromonzi districts, Mashonaland East province, Zimbabwe (Supplementary Fig. S1), (b) use APSIM and IAT modeling tools to assess the effect of improved productivity of crop and livestock enterprises; the contribution of crops to the livestock enterprise and vice versa; and on the overall household income generation.
Materials and methods
Study area
The study was undertaken within a larger ACIAR-funded research project ‘Integrating crop and livestock production for improved food security and livelihoods in rural Zimbabwe’ (ZimCLIFS). The project sought to improve food security through improved integration of crop and livestock production in Mashonaland (Murehwa and Goromonzi) and Matabeleland (Nkayi and Gwanda). This study is focused only on Murehwa and Goromonzi districts located in the sub-humid part of Zimbabwe.
On-farm experimentation with a range of crop–livestock integration practices was conducted in Murehwa and Goromonzi districts of Mashonaland East province in Zimbabwe. Murehwa is located at 17.65°S; 31.78°E at 1300 m above sea level (Supplementary Fig. S1). Goromonzi is located at 17.86°S; 31.22°E at 1462 m above sea level. Both districts are located in agro-ecological region II which covers 8% of ZimbabweFootnote 1 and receives an average rainfall ranging between 750 and 1000 mm per annum with 16–18 rainy pentads per season received between October and May (Mugandani et al., Reference Mugandani, Wuta, Makarau and Chipindu2012). The dominant soil type in both Goromonzi and Murehwa are low fertility sands derived from granitic parent material. There are however sporadic patches of fertile clay derived from dolerite parent material (Nyamapfene, Reference Nyamapfene1991). The research covered both Murehwa and Goromonzi districts which have similar agro-ecological conditions, thus there is similar agricultural productivity and socio-economic conditions. Similarly, it is assumed that there will be no significant differences in the crop and livestock productivity (Mugandani et al., Reference Mugandani, Wuta, Makarau and Chipindu2012).
Most of the households in the two districts practice mixed farming with maize (Zea mays L.) and cattle (Bos indicus L.) being the dominant crop and livestock species. Households also grow groundnuts (Arachis hypogaea L.), cowpeas (Vigna unguiculata L. var. Walp) and sweet potatoes [Ipomoea batatas (L.) Lam.]. Goats (Capra hircus L.) and traditional chickens also contribute to the livestock component of many smallholder farms (Mutenje et al., Reference Mutenje, Kassie, Gwara and Mujeyi2014). Some households in Murehwa and Goromonzi practice market gardening, and sell vegetables (Brassica spp.), tomatoes (Lycopersicon esculentum L.) and sweet potatoes at the Harare vegetable market (Mpande and Adziwa, Reference Mpande and Adziwa2011).
Under the local communal land tenure system, cattle are usually herded during the rainy season to avoid crop damages in the fields, and are confined to kraals near the homestead for protection during the night (Abunyewa and Karbo, Reference Abunyewa and Karbo2005; FAO, 2006). During the dry season, animals graze freely and are occasionally checked for diseases, injuries or losses. During such periods, cattle graze on crop residues left in the fields after harvesting and most of the manure that accumulates in the kraals is applied to the vegetable plots (Nzuma, Reference Nzuma2013). The bulk of the feed resource for smallholder cattle during the dry season is communal rangelands, which are poor in feed quality and quantity, thus negatively affecting productivity (Gwiriri et al., Reference Gwiriri, Manyawu, Mashanda, Chakoma, Moyo, Chakoma, Sethaunyane, Imbayarwo-Chikosi, Dube and Maasdorp2016).
Baseline survey and farmer classification
A total of 800 households were sampled from both Murehwa and Goromonzi districts of Mashonaland East province. A structured household questionnaire was administered to a statistical sample of ten household heads. The questionnaire collected data on household demography, asset ownership, crop and livestock dynamics, household economics and crop and livestock marketing information (Mutenje et al., Reference Mutenje, Kassie, Gwara and Mujeyi2014). The household survey data were subjected to principal component analysis (PCA). PCA is a tool for assessing diversity in a population. PCA identified the key patterns among the respondent farmers to classify them into different categories. PCA aggregates characteristics of farmers into different clusters based on the predominant characteristics of the farming systems. The following three farmer categories were identified: (1) old resource endowed (OR); (2) part time (PT); and (3) young, risk taking and enthusiastic (YRE) (Table 1). Chikowo et al. (Reference Chikowo, Zingore, Snapp and Johnston2014) categorized farmers similarly in their studies. During the project, one representative household that had adopted some form of crop–livestock integration practices was randomly selected for the case study from each of the ten ZimCLIFS project communities in Mashonaland East. This increases the chances of capturing all sources of diversity in the two districts. Household selection was conducted with assistance of the resident Agricultural Extension Officers (AEOs) in each project community. Selection criteria included willingness of the farmer to host on-farm trials, presence of both crop and livestock production activities on the farm and possession of unused land area on which to expand agricultural activities. The farmer representatives were then allocated into the relevant farmer categories obtained through PCA analysis (Table 1).
Table 1. Key characteristics of the dominant smallholder farmer categories in Murehwa and Goromonzi districts, Mashonaland East province, Zimbabwe

OR farmers are usually retired civil servants or former low-level private sector employees. These farmers possess greater land holdings, own more livestock, cultivate a variety of crops and generally have more accumulated financial resources. They also use more agricultural inputs but have less available household labor. PT farmers are mostly urban dwellers who have rural homesteads which they visit during the weekends and employ laborers who are mostly in charge of farming operations in their absence. These farmers have the least available land area and crop and livestock farming experience. YRE farmers are typically <40 years of age and are financially insecure, but have a genuine passion for farming and are willing to try new farming technologies (Table 1). Each category comprised of small-scale farmers with diverse characteristics, but the study focused on farmers with the most predominant characteristics, which was used to define each group. Using these farmer categories, a modeling-based case study approach was employed to assess the effect of improved crop–livestock integration on smallholder farms within the ZimCLIFS project communities.
The concept of crop–livestock integration
Expression of crop–livestock integration in physical terms such as kg ha−1, liters, livestock units (LU), labor hours and oxen days can be complex and confounding, hence the expression of integration in monetary values was adopted for the current study. Monetary value allows for easy comparison and understanding for smallholder farmers. Expression in monetary value aid in adoption of crop–livestock integration strategies as farmers are increasingly attracted by financial returns. Conceptually, integration can be expressed in monetary termsFootnote 2 as the ‘additional gross margin (GM)’Footnote 3 that is derived from the synergism of coupled crop–livestock production activities vs individual separate crop and livestock entities. In this study, crop–livestock integration was computed as the total monetary value of products interchanged between the crop and livestock components (Sempore et al., Reference Sempore, Andrieu, Le, Nacro and Sedogo2016). The monetary value of crop products such as fodder and crop residue was computed using actual production cost estimates from the farmers who produced the crop products. Similarly, the total monetary value of livestock products such as draught power and manure utilized or consumed by crops was computed. Current market values were used in assessing the value of the crop and livestock products and by-products.
Profitability assessment was conducted among each of the three farmer categories using the IAT (Lisson et al., Reference Lisson, MacLeod, McDonald, Corfield, Pengelly, Wirajaswadi, Rahman, Bahar, Padjung, Razak, Puspadi, Dahlanuddin, Saenong, Panjaitan, Hadiawati, Ash and Brennan2010). The model computed profitability as the difference between value of inputs and outputs. The study assumed that some profits from the enterprises will be utilized as the household income. It is also typical of households to utilize profits for financing household operations (Mutenje et al., Reference Mutenje, Kassie, Gwara and Mujeyi2014).
Crop–livestock integration scenarios
Several crop and livestock production scenarios were formulated for each farmer category that were judged by the authors to be technically feasible for improving crop–livestock integration for specific smallholders (Table 2). Each of the scenarios was formulated based on resource endowment, literacy levels and challenges in current crop–livestock production of the targeted groups. Resource endowed farmers were designed scenarios that need financial resources. With regards to semi-literate farmers, the scenarios were designed to mimic agricultural advice which came from AEOs. Scenarios for the young resource endowed farmers were designed to mimic advice from AEOs for rationale crop and livestock husbandry practices and their eagerness to undertake novel farming practices.
Table 2. Farmer category-specific crop–livestock improvement and integration scenarios for farmers in Murehwa and Goromonzi districts, Zimbabwe

Simulation models
The prospective production and financial implications of adopting the various crop and livestock integration strategies were explored using simulation modeling. Crop yields (grain, stover and forages) were projected using the APSIM (Holzworth et al., Reference Holzworth, Huth, DeVoil, Zurcher, Herrmann, McLean, Chenu, van Oosterom, Snow, Murphy, Moore, Brown, Whish, Verrall, Fainges, Bell, Peake, Poulton, Hochman, Thorburn, Gaydon, Dalgliesh, Rodriguez, Cox, Chapman, Doherty, Teixeira, Sharp, Cichota, Vogeler, Li, Wang, Hammer, Robertson, Dimes, Whitbread, Hunt, van Rees, McClelland, Carberry, Hargreaves, MacLeod, McDonald, Harsdorf, Wedgwood and Keating2014) and these data were then used to calibrate the IAT (Lisson et al., Reference Lisson, MacLeod, McDonald, Corfield, Pengelly, Wirajaswadi, Rahman, Bahar, Padjung, Razak, Puspadi, Dahlanuddin, Saenong, Panjaitan, Hadiawati, Ash and Brennan2010), a farm household-level farming systems performance evaluation model.
APSIM is a longstanding biophysical cropping systems model that simulates crop yields and biophysical processes through integrating crop, soil, climate and management parameter interactions (Keating et al., Reference Keating, Carberry, Hammer, Probert, Robertson, Holzworth, Huth and Hargreaves2003). The APSIM model has been extensively used in soil water balance (Verburg and Bond, Reference Verburg and Bond2003; Mupangwa and Jewitt, Reference Mupangwa and Jewitt2011), soil nutrient dynamics (Shamudzarira and Robertson, Reference Shamudzarira and Robertson2002; MacCarthy et al., Reference MacCarthy, Vlek, Bationo, Tabo and Fosu2010; Masikati et al., Reference Masikati, Manschadi, Van Rooyen and Hargreaves2014), climate change (Kandji et al., Reference Kandji, Verchot and Mackensen2006; Cooper et al., Reference Cooper, Dimes, Rao, Shapiro, Shiferaw and Twomlow2008; Dimes et al., Reference Dimes, Cooper, Rao, Humphreys and Bayot2008) and in mixed crop–livestock systems (Lisson et al., Reference Lisson, Rahman, MacLeod, McDonald, Ash, Pengelly, Brennan, Gross, Corfield, Saenong, Panjaitan, Wirajaswadi, Sutaryono and Bahar2001) research within the sub-Saharan region. APSIM is a biophysical crop model, hence it cannot simulate socio-economic aspects such as resource endowment. However, the model can indirectly account for resource endowment through fertilizer dynamics. Resource endowment will lead to increased availability of income to purchase mineral fertilizers. This is because farmers have highlighted that reduced input use is attributed to financial challenges (Thomas et al., Reference Thomas, Twyman, Osbahr and Hewitson2007). The model was therefore parameterized with high fertilizer application (Holzworth et al., Reference Holzworth, Huth, DeVoil, Zurcher, Herrmann, McLean, Chenu, van Oosterom, Snow, Murphy, Moore, Brown, Whish, Verrall, Fainges, Bell, Peake, Poulton, Hochman, Thorburn, Gaydon, Dalgliesh, Rodriguez, Cox, Chapman, Doherty, Teixeira, Sharp, Cichota, Vogeler, Li, Wang, Hammer, Robertson, Dimes, Whitbread, Hunt, van Rees, McClelland, Carberry, Hargreaves, MacLeod, McDonald, Harsdorf, Wedgwood and Keating2014). In this research, the APSIM model was calibrated for the climate, soil, crop variety and agronomic conditions of each of the cultivated crop types during the 2012/13 and 2014/15 cropping seasons. Calibration of the APSIM model indicated n-RMSE values for crop yields and stover of <30% across all crop types and farmers’ categories. Therefore, the APSIM model was assumed to predict yields accurately (Manuela et al., Reference Manuela, Soler and Paulo2007). In this research, the APSIM model was used to simulate grain yields and stover of all crops cultivated by the three farmer groups (Table 1).
The IAT employs a modular approach that combines crop and forage databases with an animal production simulation model and a household economic module to explore the resource and financial impacts of changes to crop and livestock production activities embedded within smallholder mixed crop–livestock farming systems. The model simulates enterprise, household and whole farm profitability, labor, food security based on crop yield, land area, household and labor dynamics, input and output prices. The model integrates the household financial and demographic dynamics, crop, labor, animals and pastures to evaluate their combined impact on household food security and profitability (Lisson et al., Reference Lisson, MacLeod, McDonald, Corfield, Pengelly, Wirajaswadi, Rahman, Bahar, Padjung, Razak, Puspadi, Dahlanuddin, Saenong, Panjaitan, Hadiawati, Ash and Brennan2010). The IAT model has been extensively used in crop livestock systems in Indonesia (Lisson et al., Reference Lisson, Rahman, MacLeod, McDonald, Ash, Pengelly, Brennan, Gross, Corfield, Saenong, Panjaitan, Wirajaswadi, Sutaryono and Bahar2001) and improved Bali cattle productivity (MacLeod et al., Reference MacLeod, McDonald, Lisson, Rahman, Oxley and Kulasiri1999). The IAT model was utilized to assess the profitability of the different maize and livestock enterprises among the different farming categories.
Crop management
The APSIM model was parameterized based on the crop management and agro-ecological condition data of Murehwa and Goromonzi districts. The model was calibrated for both the baseline and simulated crop management scenarios (Supplementary Table S1). For the baseline scenario, the crop management decisions were not uniform within and between the different smallholder farmer categories but overall they were lower than the recommended. Farmers applied relatively low fertilizer levels and had low plant populations. For the simulated scenarios, it was assumed that the fields were insect pest and disease free.
Statistical analysis
GenStat 14.1 (VSN 2002) was used to perform a Residual Maximum Likelihood (REML) assessment of whether crop grain and stover yields differ significantly across the different scenarios, farmer categories and crop types. Grain and stover yields were the main response variables while farmer categories and crop types were the fixed effects. The REML approach was used because the data were unbalanced due to farmers having cultivated different crop types. Means of grain and stover yields of different crops were separated through the Least Significant Difference (LSD) test (P ⩽ 0.05).
Results
Initial crop–livestock integration on smallholder farms
The initial assessment showed that OR farmers had the highest level of crop–livestock integration with a total monetary value of $3981 yr−1. The PT and YRE farmers had relatively lower integration with monetary values of $1487 yr−1 and $2872 yr−1, respectively (Table 3). At least 50% of the crop–livestock integration balance was derived from crops to livestock as opposed to livestock to crops. It can be noted that the contribution of crops to livestock varied with farmer category with the OR farmers having the highest contribution of the three classes. Y RE farmers had a relatively lower contribution of US$1832 yr−1 but PT farmers had the lowest contribution of crops to livestock. The contribution of crops to livestock among the OR farmers was dominated by cowpea, lablab (Lablab purpureus L.) and velvet bean (Mucuna pruriens L.) and the lowest individual crop to livestock contribution was from maize at $299 yr−1. However, maize had the highest crop to livestock contribution at $436 yr−1 among PT farmers. While maize had the least crop to livestock contribution of $200 yr−1, groundnuts had the greatest component of crop to livestock contribution of US$767 yr−1 in the YRE farmers (Table 3).
Table 3. Monetary value of the contribution of crops–livestock and livestock–crops under the baseline scenario (US$ yr−1) in Murehwa and Goromonzi districts, Mashonaland East province, Zimbabwe

As observed with crop contribution to livestock, initial livestock contribution to crops was variable between the classes, but with some degree of similarity between the OR and YRE farmers. PT farmers had the least livestock to crop contribution compared with OR and YRE. Manure contributed at least 30% of the livestock to crop contribution, contributing 38% among OR farmers and an equivalent of 76% among PT and YRE farmers, although notably the actual annual contribution value in YRE (US$800) was almost double that for the PT farmers (US$480). However, OR had the highest draught power usage and PT farmers the least (Table 3).
Simulated individual crop and livestock enterprise profitability
The baseline survey indicated that the OR farmers had a net profit of at least $5500 yr−1 with the bulk of it being contributed by cattle, maize and cowpea (Table 4). The lowest GMs were derived from sorghum and common beans with <$50 yr−1. After simulating future crop yields and profitability based on the agronomic and livestock improvement scenarios, overall profitability increased to at least $15,200 yr−1 for OR farmers. Maize, groundnuts and cattle had the highest simulated individual GMs. Sorghum, common beans, cowpea and chickens had the lowest GM and contributed the least to the total profit with each component contributing <$800 yr−1. The highest increase in profitability was derived from groundnuts, common beans and goats. For the PT farmers, the baseline survey indicated a net profit of $1510 yr−1. The largest contribution was from chickens and ducks. PT farmers experienced losses in cowpea and had no gains in groundnuts and goats. Crop and livestock production improvement options increased net profit by over 280%, up to $4250 yr−1. There was increased income from maize and cattle, while ducks and chickens remained unchanged (Table 4).
Table 4. Baseline and simulated crop and livestock contributions to income ($) in all farmer types from Goromonzi and Murehwa districts, Zimbabwe
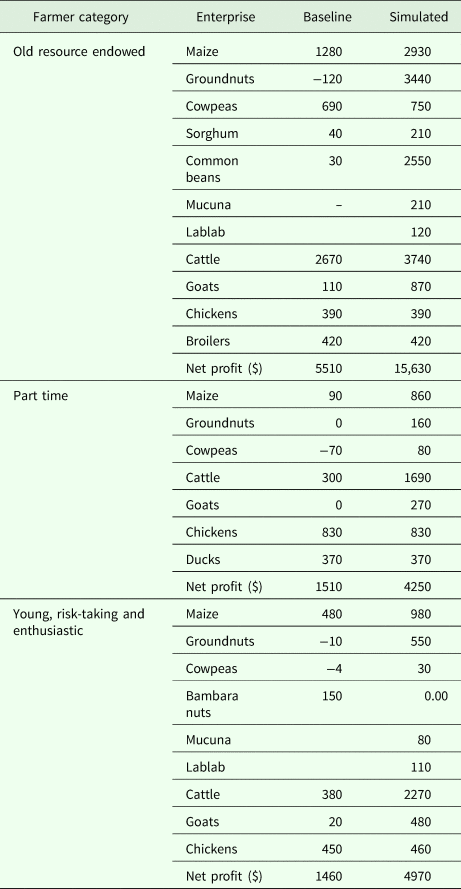
A negative figure indicates that the enterprise experienced a net loss and a positive figure indicates that the enterprise experienced a profit.
The YRE farmer baseline survey results indicated a net profit of approximately $1460 yr−1 with the bulk of it coming from maize, chickens, cattle and bambaranuts. They however experienced losses in cowpea. Upon imposition of crop and livestock improvement scenarios, YRE farmers achieved a 325% increase in net profit, attaining at least $4750 yr−1. Groundnuts, cowpea, cattle and goats enterprises had the greatest improvement in profitability (Table 4).
Simulated crop–livestock integration
After applying the crop and livestock improvement scenarios with the calibrated APSIM and IAT models, there was a projected overall increase in the contribution of crops to livestock in all farmer categories. The OR farmers realized at least a 140% increase in crop contribution to livestock, while the PT and YRE farmers, respectively, realized a 130 and 100% increase in crop contributions toward livestock. Legume crops made a higher crop contribution to livestock than cereals (Table 5).
Table 5. Total monetary value of the contribution of crops to livestock under improved options among smallholder farmers Murehwa and Goromonzi districts, Mashonaland East province, Zimbabwe
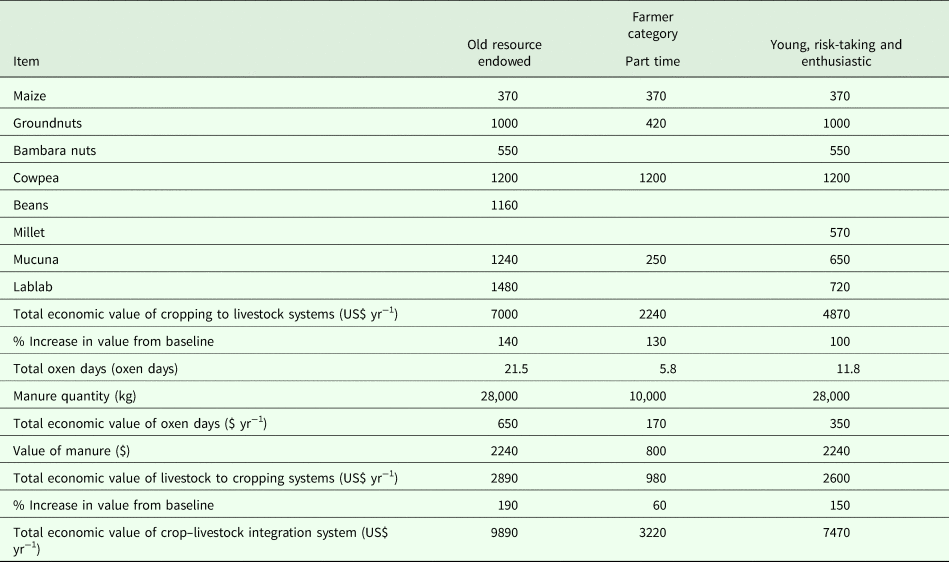
Under the improved livestock management scenarios applied to all farmer categories, there was an increase in the contribution of livestock to crops of 190% (US$2890 yr−1), 55% (US$980 yr−1) and 150% (US$2600 yr−1), respectively, for the OR, PT and YRE farmers. The economic value of oxen days (draught) also increased considerably compared with the baseline scenario. It is noted that the contribution value of crops to livestock was much higher than the reverse, with crop to livestock contribution being approximately 313% higher than livestock to crop contribution for the OR farmer category.
Discussion
Before attempting to evaluate crop–livestock integration, the study assessed the degree of diversity among the small-scale farmers in the Murehwa and Goromonzi districts. Generally, farmers currently experience relatively poor productivity in their farming systems.
Despite the wide array of recommendations for improving productivity, the uptake of the recommendations has been poor and the effectiveness is highly variable. Options to increase productivity differ among the different individual farmers. Twomlow et al. (Reference Twomlow, Urolov, Jenrich and Oldrieve2008) highlight the use of blanket recommendations ignoring the underlying socio-economic and cultural aspects as the leading cause of poor adoption. This therefore justifies the need for assessment of diversity among farmers prior to proposing recommendations. There is therefore a need for proposing recommendations that correspond to the farmer's socio-economic characteristics. The current study realized significant diversity among the different small-scale farmers in the form of old and resource endowed (OR), part-time (PT) and young and resource endowed (YRE) farmers. There is a significant variation of the socio-economic characteristics among the different farmer categories. This therefore justifies the use of different crop–livestock integration strategies in different farmer categories to improve effectiveness of uptake of the different crop–livestock integration recommendations.
Crop–livestock integration varied among the different farmer categories. Integration was greater in OR followed by YRE and PT farmers. High levels of integration are attributed to many factors including farming experience and the use of livestock manure. The greater crop–livestock integration among OR farmers can largely be attributed to the high level of experience of at least 30 years in both crop and livestock production technologies. Due to greater experience smallholder farmers acquire and perfect skills to increase productivity while minimizing external input. This was in sharp contrast to the relatively low farming experience of 10 and 17 years for the PT and YRE smallholder farmers, respectively (Table 1). That is, the OR farmers had greater know-how in terms of increasing the efficiency and productivity of their farming systems, especially the different systems’ components (Sombilla et al., Reference Sombilla, Balasubramanian, Bell, Papademetriou, Dent and Herath2000). PT farmers have alternate sources of income, hence they are not motivated to invest in crop–livestock integration strategies to improve productivity. The extra labor and knowhow needs may demotivate PT farmers from cultivating forage for livestock feeding for sell. A higher initial level of crop–livestock integration was evident with OR farmers, their crops contributing more income than livestock. The use of legume crops through grain and residues fed to livestock contributed at least 90% of the total crop value, although the yields are evidently low, which is a general characteristic of many smallholder farming systems. This can be attributed to the higher market value and quantity of legume grain and residue relative to cereals. For instance, legumes have a high grain content of at least 20–40% of their total dry weight compared with <10–12% in cereals (Shewry and Halford, Reference Shewry and Halford2015). Besides the physiological aspect that proteins require more energy of at least 50,000 kJ mol−1 compared with the 2868 kJ mol−1 required for carbohydrate production (Luley-Goedl and Nidetzky, Reference Luley-Goedl and Nidetzky2010), the production costs of legumes are higher than for cereals, which translated to the higher value of contribution of crops to livestock.
Livestock manure utilized in crop fields and draught power for crop cultivation and household transport were the most significant livestock-to-crop contributions across all three farmer types. The highest values for livestock contribution to crops was recorded for the OR farmers, and is attributed to the greater number of livestock holding in the OR farmer category, averaging 12.85 LU and 0.42 goat units (GU) compared with 5.45 and 10.27 LU among the PT and YRE farmers, respectively. In addition, most of the OR farmers were at least 58 years old, are retired government employees who once had relatively high-income jobs and had more disposable income and savings invested in cattle. The greater LUs also enabled OR farmers to be better positioned to increase crop livestock integration, through provision of manure to crops. On the other hand, the PT farmers were relatively young and <50 years of age, hence have less disposable income with which to acquire livestock (Parkinson, Reference Parkinson2009). On the contrary, the YRE farmers were <39 years of age, but were noted to be shrewd and full-time farmers, and this may explain their relatively higher livestock numbers.
The lowest level of integration was observed for the PT farmer category who had a crop-to-livestock and livestock-to-crop contribution of $860 and $630 yr−1, respectively (Table 3). These farmers allocate less time to farming and hence attain lower crop yields and less income. The low LU associated with these farmers might also be attributed to poor livestock management reflected in the low manure use and value of draught power.
Optimization of crop yields
Despite having greater crop diversity, crop yields for the OR farmers were generally low, with legumes having the lowest yields. Similarly, among the PT and YRE farmers, legumes had the least yields compared with other crop types. This is attributed to poor agronomic practices on smallholder farms especially in legumes. Legumes are often seeded late and at low plant populations with little or no fertilizer or manure applied. In addition, OR farmers were observed to use retained legume seed due to relatively high legume seed prices (up to $5 kg−1) compared with maize seed prices (~$2 kg−1) (Akibode and Maredia, Reference Akibode and Maredia2011). Farmers across all three categories generally perceive legumes as secondary crops, hence the amount of investment in them is low (Giller et al., Reference Giller, Murwira, Dhliwayo, Mafongoya and Mpepereki2011) resulting in low legume yields obtained in the current study compared with yields obtained in commercial systems.
The low crop yields for the OR farmer category could also be attributed to the limited availability of household labor (about two aged adults per household) that is characteristic of this category. Labor shortages are more critical during the peak labor demand periods where high weed pressure in multiple crops will lead to significant yield penalties for the OR farmer category. The effect of a labor shortage is less relevant for the YRE farmers category who have greater available household labor of eight persons, allowing for faster and timeous weed control.
PT farmers spend most of their time engaged in off-farm activities, as they only cultivate two crops compared with the OR and YRE farmers who cultivate multiple crops. This resulted in less time and labor allocated to on-farm activities, which was exacerbated by the limited land area of ~2.3 ha. Off-farm activities like cutting firewood, fishing and industrial jobs by PT farmers result in increased available capital to purchase inputs like fertilizers. This resulted in increased fertilizer use of at least 200 kg ha−1 basal dressing and 300 kg ha−1 top dressing among part-time farmers which complies with the generally recommended fertilizer rates (Seed Co., 2010).
The YRE farmers had relatively higher legume yields due to the use of relatively small quantities of basal fertilizer in some of the legume crops. This promoted better legume growth and development leading to higher yields. The risk taking, ingenuity and inquisitive mentality and attitude of this farmer type often leads them to applying fertilizers in legumes. This also enables them to cultivate a variety of crops despite their limited financial resources. However, use of retained legume seed, late sowing and poor soil fertility management are among the leading causes of poor legume yields in the smallholder farming sector (Giller et al., Reference Giller, Murwira, Dhliwayo, Mafongoya and Mpepereki2011). Legume yields are rarely above 500 kg ha−1 under smallholder farming. Improved agronomic practices through the use of better hybrid varieties at correct plant population can increase yields to commercial levels (Seed Co., 2010). This is reflected in the simulated improved crop yields across all three farmer types with the OR and YRE farmers recording the higher yield increases.
Livestock manure
Despite their higher livestock holdings, the OR farmers used the least amount of manure (<5 t ha−1 yr−1) compared with the PT and YRE farmers. While the greater number of cattle would imply that the OR farmers have high available quantities of manure to use, manure collection, transport and application require access to labor. The reduced household labor associated with the OR farmers may well account for the limited use and application of manure in their fields. High manure usage by the YRE farmers’ category might be attributed to the lack of disposable cash to purchase mineral fertilizer, hence management to improve soil fertility was mainly through manure use.
Simulated maximum utilization of livestock manure would reduce mineral fertilizer use across all three farmer types. Based on their livestock numbers, the OR farmers can apply at least 32 tonnes of manure which is sufficient to fertilize a hectare and improve maize productivity. In contrast, the PT farmers who have 5.45 LU can only apply a maximum of 14 tonnes of manure which is insufficient to fertilize a hectare of crop. Lack of mineral fertilizer subsidies in Zimbabwe as a result of macro-economic challenges also translates to a higher premium being placed on manure which, in turn, makes it an expensive commodity to purchase off-farm (Wolmer, Reference Wolmer1997). Farmers are therefore motivated to pen livestock during the night to enable them to collect more manure.
Crop residue use on smallholder farms
There is competition for stover in mixed smallholder farming systems. Livestock consume crop residues during the dry season, but crop residues are also applied as mulch in the field. The competition is exacerbated by the fact that crop residues have always been in short supply due to poor crop residue productivity in smallholder farming systems. As a result, the baseline use of crop residues for livestock production was <30% across the three farmer types. This is less than the recommended threshold of at least 30% crop residue cover. In smallholder systems, residues are utilized as mulch, in compost preparation, burnt or blown away by wind. This corresponds with the findings by Russo (Reference Russo, Dzowela, Said, Wendem-Agenehu and Kategile1988) where observation of local practices revealed that some farmers burn their crop residues after harvest or utilize them to making compost rather than keep for livestock feed. There is therefore a deficit in crop residues for use as either supplementary stock feed or mulch in smallholder farming systems. The bulkiness of whole maize crop plant culminates in substantial transport costs for off-field processing, hence maize is normally processed in the field. As a result, residues remain in the field and some are consumed by cattle in unfenced fields. Maize crop residues are the most common source of alternative livestock feed during the dry season in smallholder farming systems. Maize crop residues, however, have poor nutritional value with a crude protein content of 5.1% CP (Lazzarini et al., Reference Lazzarini, Sampaio, Detmann, Souza, Paulino and Oliveira2009). It can be argued that maize crop residues have better value when utilized as mulch, as opposed to stock feed. Crop and forage legumes are potential alternatives to maize crop residues as they have better nutritive value. This therefore increases the contribution of crops to livestock, thus improving integration.
Cattle normally feed on veld grass with a low crude protein content of 4% CP whose quantity and quality reduce during the dry season. The low baseline land area traditionally allocated to cultivating legumes reduced the amount of legume residue available for livestock. Improvements in agronomic practices and efficient use of residues could increase grain and biomass yields, thus increasing the amount of legume residues available for livestock feeding (Giller et al., Reference Giller, Murwira, Dhliwayo, Mafongoya and Mpepereki2011).
Forage and fodder legumes have a relatively high grain and residue CP content of at least 17%. It has been asserted by Gwanzura et al. (Reference Gwanzura, Ngambi and Norris2012) that velvet bean and lablab, low fiber legumes which have a crude protein content range of 16–19%, can be utilized to supplement low-quality roughages such as grass and maize crop residue resulting in improved ruminant productivity. Under the scenarios in which all available crop residues were used for feed, the level of livestock productivity increased (Table 1). OR farmers had access to the highest quantities of forage. However, limited availability of land and time resources of the PT farmers reduced the benefits that they could obtain from improved agronomic practices as the projected yield increases were minimal.
Profitability of crop and livestock production enterprises
The relatively low profits of $1510 yr−1 in the PT farmer type category before adoption of improved crop–livestock production technologies can be attributed to the reduced profits from maize and goat enterprises, and was worsened by losses in groundnut production (Table 4). The relatively high contribution to profits associated with chickens and cattle reinforce the fact that livestock may still perform relatively well despite limited management as typified by no use of supplementary feed and the free ranging system of grazing that is associated with labor-constrained households typical of PT farmers. This is also typical of OR and YRE farmers who receive at least 50% of income from chickens and cattle.
The higher projected profits that could be derived from the adoption of improved maize growing practices across all farmer types contrast with national trends in Zimbabwe which show recurrent maize losses. This can be attributed to the use of recommended agronomic farming practices across all farmer types. Specifically in PT and YRE farmers, this prospective gain can be attributed to the increased basal and top dressing fertilizer use to the recommended levels of at least 300 and 200 kg ha−1, respectively, compared with reduced fertilizer application (Seed Co., 2010). In contrast, the low profits associated with legume crops across all three farmer types could be due to the low projected grain and biomass yields. Low legume yields may be associated with the outlook of many farmers who view them as minor crops which can be planted late, require no mineral fertilizer, can be grown from retained seed and are planted in marginal fields (Giller et al., Reference Giller, Murwira, Dhliwayo, Mafongoya and Mpepereki2011).
The projected level of profitability from the application of improved crop and livestock management practices increased across all three farmer types (Table 4). As was similar for the baseline scenarios, livestock enterprises generated the highest profits while legumes made a greater contribution to household income compared with cereals. This can be attributed to the high legume prices on the market. Crop–livestock integration can also be driven by population growth, policy developments and market forces (Wolmer, Reference Wolmer1997). High producer prices for legumes relative to cereals increase the farmer's opportunity for profitably producing food and forage legumes which ultimately improves the scope for crop–livestock integration on smallholder farms.
Resultant crop–livestock integration
All farmer types experienced an increase in crop–livestock integration of 190, 60 and 150% for the OR, PT and YRE farmers, respectively, over the baseline. This can be attributed to improved crop and animal productivity across all farmer types. With regards to the individual components, all farm types had at least a 100% increase in the contribution of crops-to-livestock. This was mainly attributed to the increase in crop production where both grain and residue yields increased by at least 20% across all farmer types. The available land area and increased productivity of the different crops under the OR as opposed to the other farmer types led to the highest crop-to-livestock contribution of at least 190% in this category (Table 5).
Subsequently, OR farmers had the greatest increase in livestock-to-crops integration due to the hiring of extra labor to collect, carry and spread manure. The pattern was similar to the YRE farmers. For the latter farmer category, the increased integration was attributed to the increased manure use which was due to the increased awareness on the value of manure use in crop production. Farmers with more cattle will derive the most benefit from applying manure as a hectare of cropland ideally requires at least 30 t ha−1 of manure which requires at least 12 LU per year to produce (Chastain and Camberato, Reference Chastain, Camberato and Adams2004). The actual amount of manure produced is dependent on the diet, method of feeding and collection. For example, while 1 LU of cattle produces at least 7000 kg yr−1 manure, the recovery rate of manure from animals that are kraaled overnight is only 30–40% or 2500 kg of manure (Nzuma, Reference Nzuma2013). PT farmers who have <5.45 LU (Table 1) will thus produce about 6000 kg yr−1 of manure which may support less than 0.5 ha of cropland, resulting in lower productivity than the other two categories.
Conclusions
Current levels of crop–livestock integration are generally lower relative to the potential across smallholder farmer types in the Murehwa and Goromonzi districts of Zimbabwe. Specifically, individual and combined crop and livestock productivity is generally low across all the smallholder farmer types considered. Despite being low, crop–livestock integration is relatively higher for full-time farmers and lower for part-time farmers practicing mixed farming. Contribution of crops to livestock is greater as opposed to livestock contribution to crops, mainly due to the value of fodder and crop residues consumed by livestock being greater than the value of manure and draught power utilized in crop production. However, potential crop and livestock productivity and integration improvement strategies vary with farmer socio-economic status. Simulation modeling has indicated that improved crop agronomy and livestock husbandry can potentially increase both individual component and combined crop and livestock productivity for smallholder farming systems. System efficiency and profitability can potentially increase as a result. However, trade-offs in labor and soil fertility should be considered. Adoption of crop–livestock integration can potentially increase system value and income by at least 100% in specific smallholder systems, with access to land area and livestock holding influencing system outcomes. Integration is potentially improved in OR farmers through improved efficiency in crop and livestock productivity. This also includes increased labor availability, supplementary cattle feeding and use of cattle manure. PT farmers potentially improve integration through supplementary goat and cattle feeding and selling of small ruminants like goats. Among other options, inexperienced YRE farmers need improved access to crop and livestock extension to increase productivity and integration. While indications from the current study were based on modeled theoretical proposals for crop–livestock improvement and integration, there is a scope in assessing the practicability and feasibility of these results using on-farm trials in smallholder systems. The study therefore highlights that the first step toward improved crop–livestock integration in smallholder farming systems is increased component productivity through increased crop or livestock husbandry. Maximum use of by-products from either component in smallholder farms moves the systems toward an improved crop–livestock integrated state.
Author ORCIDs
Siyabusa Mkuhlani http://orcid.org/0000-0001-7872-2036
Supplementary material
The supplementary material for this article can be found at https://doi.org/10.1017/S1742170518000558.
Acknowledgements
The authors would like to acknowledge the research received from the Australian Centre for International Research through ACIAR through the project CSE/2010/022 ‘Integrating crop and livestock production for improved food security and livelihoods in rural Zimbabwe’ (ZimCLIFS).