INTRODUCTION
The debate over the effect of incidental and explicit learning conditions has been one of the central subjects in the field of Second Language Acquisition (SLA) (Ishikawa, Reference Ishikawa2019; Leow, Reference Leow2019; Rebuschat, Reference Rebuschat2015). Studies have generally showed an advantage for explicit over implicit learning conditions (for meta-analyses see, e.g., Goo et al., Reference Goo, Granena, Yilmaz, Novella and Rebuschat2015; Norris & Ortega, Reference Norris and Ortega2000; Spada & Tomita, Reference Spada and Tomita2010; for a different view see Ellis et al., Reference Ellis, Loewen, Elder, Erlam, Philp and Reinders2009); however, recent meta-analytical research (e.g., Kang et al., Reference Kang, Sok and Han2018) and psycholinguistic research (e.g., Morgan-Short et al., Reference Morgan-Short, Steinhauer, Sanz and Ullman2012) have enriched, challenged, or even reversed the conclusions of previous studies, thereby keeping the debate alive.
One of the key issues in the debate is whether linguistic complexity interacts with learning conditions (e.g., Andringa et al., Reference Andringa, de Glopper and Hacquebord2011; DeKeyser, Reference DeKeyser1995, Reference DeKeyser, Doughty and Williams1998, Reference DeKeyser2016; Housen et al., Reference Housen, Pierrard, Van Daele, Housen and Pierrard2005; Reber et al., Reference Reber, Kassin, Lewis and Cantor1980; Tagarelli et al., Reference Tagarelli, Ruiz, Moreno Vega and Rebuschat2016; see Spada & Tomita, Reference Spada and Tomita2010 for a meta-analysis). So far, a few studies have incorporated both explicit and implicit conditions and targeted both simple and complex structures, and their findings are inconclusive. Some report that the two conditions are equally effective regardless of linguistic complexity (e.g., Housen et al., Reference Housen, Pierrard, Van Daele, Housen and Pierrard2005); others find that while explicit instruction leads to more gains than implicit/incidental exposure in the learning of simple rules, the two conditions do not produce statistically different results when the learning target is complex (e.g., DeKeyser, Reference DeKeyser1995, Reference DeKeyser, Doughty and Williams1998; Williams & Evans, Reference Williams, Evans, Doughty and Williams1998). Still others point at an advantage of explicit instruction over implicit/incidental exposure regardless of complexity (e.g., Robinson, Reference Robinson1996; Tagarelli et al., Reference Tagarelli, Ruiz, Moreno Vega and Rebuschat2016; see Spada & Tomita, Reference Spada and Tomita2010 for a meta-analysis). Finally, very few studies revealed that other factors may also come into play, such as the type of the target structures (de Graaff, Reference de Graaff1997), the salience of the target structures (Tagarelli et al., Reference Tagarelli, Ruiz, Moreno Vega and Rebuschat2016), and learners’ first language (L1) background (e.g., Andringa et al., Reference Andringa, de Glopper and Hacquebord2011).
The inconclusiveness of the findings is partly attributable to the complex nature of the definition of linguistic complexity (Bulté & Housen, Reference Bulté, Housen, Housen, Kuiken and Vedder2012; Housen et al., Reference Housen, Kuiken, Vedder, Housen, Kuiken and Vedder2012; Housen & Simoens, Reference Housen and Simoens2016; Hulstijn & de Graaff, Reference Hulstijn and de Graaff1994). Hulstijn and de Graaff (Reference Hulstijn and de Graaff1994), arguably the first systematic investigation into this subject, proposed that linguistic complexity should be primarily determined by functional complexity, or the “number (and/or the type) of criteria to be applied to arrive at the correct form” (p. 103). Housen et al. (Reference Housen, Kuiken, Vedder, Housen, Kuiken and Vedder2012, p. 4) provided a more encompassing definition that includes the intrinsic “formal” or “semantic-functional” properties of second language (L2) elements and the properties of the “(sub-)systems” of the L2 elements. Importantly, there is a gap between the objective, feature-related complexity of the structures in the contexts of explicit instruction and the structures perceived and abstracted by learners in the incidental condition. DeKeyser (Reference DeKeyser, Doughty and Williams1998, p. 44), for example, pointed out that average learners are unlikely to induce implicitly any but the most semantically transparent and formally simple rules. Rebuschat (Reference Rebuschat2008, experiment 3) also reported that under the incidental condition, a complex rule may be partially and inaccurately perceived as a simple rule in learners’ interlanguage system, resulting in (apparent) learning gains. Some researchers have hence called for further studies on the exact nature and the relative weight of the various factors that contribute to objective, feature-related complexity and subjective, learner-related difficultyFootnote 1 (e.g., Housen & Simoens, Reference Housen and Simoens2016).
Among the few experimental studies of the relation between linguistic complexity and learning condition, Tagarelli et al. (Reference Tagarelli, Ruiz, Moreno Vega and Rebuschat2016) is the first to offer initial evidence that cognitive salience impacted the learning of one complex syntactic rule but not that of the other. The researchers used a semiartificial language composed of German syntax and English lexicon, a system developed by Rebuschat (Reference Rebuschat2008) and subsequently used in multiple recent studies (e.g., Kim & Godfroid, Reference Kim and Godfroid2019; Miller & Godfroid, Reference Miller and Godfroid2020; Rebuschat & Williams, Reference Rebuschat and Williams2012). Fifty-one native speakers of English with no background in German were randomly assigned to the incidental group or the instructed group. During the training phase, the participants in both groups were exposed to 120 sentences containing three patterns of varying linguistic complexity and were asked to judge the plausibility of the sentences and also orally repeat them. Before the plausibility and sentence repetition tasks, the participants in the instructed group were also presented with the verb-placement rules and were asked to write down two sentences that followed each rule. During the testing phase, both groups completed an untimed grammaticality judgment task (GJT) of 60 new sentences. For each sentence, participants were asked to judge the sentence’s grammaticality, indicate how confident they were in the accuracy of their judgment, and report the source of their judgment. The results showed that exposure condition was a significant predictor of two syntactic patterns (V2 and V2-VF), but not of the most complex structure (VF-V1). The debriefing questionnaire data showed that the VF-V1 rule was highly salient to many participants, which according to the researchers might have compensated for its complexity and led to better learning results than expected. The incidental group’s d’ scores for this structure approached significance (d’ = 0.28, p = .07), which contrasted with the same group’s performance for the V2-VF structure (d’ = 0.04, p > .05). Meanwhile, the VF-V1 rule was the only structure for which the learning outcomes were not predicted by exposure condition.
The interesting results reported by Tagarelli et al. (Reference Tagarelli, Ruiz, Moreno Vega and Rebuschat2016) suggested that in addition to linguistic complexity and learning condition, learner-related difficulty may also affect learning outcomes in more complicated ways than expected. Specifically, certain target structures (e.g., VF-V1 with its contiguous verb placement) may give rise to greater cognitive salience and less perceived difficulty, thus leveling out the advantage of explicit instruction.
Tagarelli et al.’s (Reference Tagarelli, Ruiz, Moreno Vega and Rebuschat2016) interesting results promoted us to explore another shared learner-related feature apart from cognitive salience, namely, learners’ L1 experience. Learners may be affected by their L1 syntax and perceive the difficulty of different L2 structures differently, which may moderateFootnote 2 the effect of linguistic complexity and exposure condition in semiartificial learning. As the following review will reveal, the use of lexicon from learners’ L1 in semiartificial grammar learning is a double-edged sword: on the one hand, it frees learners from the burden of learning new words while enabling researchers to control for exposure and simulate natural language processing, thereby giving studies in this paradigm an advantage over those in natural language instruction and artificial grammar learning; on the other hand, however, it could give rise to learners’ reliance on L1 syntactic knowledge, thus increasing the variability of results. It is therefore necessary to examine the generalizability of relevant findings in the semiartificial grammar learning paradigm by conducting partial/conceptual replications in diverse L1 contexts. In the context of this study, it is interesting to investigate whether Tagarelli and colleagues’ findings about exposure condition and linguistic complexity would be replicated in Chinese with a typologically different syntax. The present study contributes to this gap by using the same procedure as that of Tagarelli and colleagues but adopting the Chinese translations of the training and test stimuli, with a population of native speakers of Chinese.Footnote 3
In addition to recruiting L1-Chinese participants and translating the stimuli into Chinese, we also introduced another important change to the design of the initial study, namely, eliciting production data during the debriefing phase. As one anonymous reviewer pointed out, language production is barely investigated in L2 research that employs (semi)artificial languages. To our knowledge, Ruiz et al. (Reference Ruiz, Tagarelli and Rebuschat2018) is the only study so far that elicited sentence production data alongside grammaticality judgment data. In that study, the participants in both the incidental and intentional conditions predominantly produced simple sentences on the production task (incidental: 86%; intentional: 92%), and the two groups did not perform differently accuracy-wise in sentence production or grammaticality judgment. Importantly, the explicit condition that Ruiz and colleagues investigated was intentional rule-search while ours was instruction. By eliciting production data during the debriefing phase, we may compare our results with Ruiz and colleagues’, thereby offering new insights into the relation between language production and exposure condition, a rarely investigated area in previous (semi)artificial grammar learning research.
LITERATURE REVIEW
L1 TRANSFER IN SEMIARTIFICIAL GRAMMAR LEARNING
Abundant psycholinguistic and SLA research has investigated the crucial role of prior L1 knowledge in adult L2 learners’ perception of natural language instruction (e.g., Andringa et al., Reference Andringa, de Glopper and Hacquebord2011; Doughty & Williams, Reference Doughty, Williams, Doughty and Williams1998; Ellis, Reference Ellis2006; Kellerman, Reference Kellerman1978; VanPatten, Reference Van Patten and VanPatten2003). In the acquisition of L2 syntax, the whole of the L1 grammar constitutes the L2 initial state (Hartsuiker & Bernolet, Reference Hartsuiker and Bernolet2017; Neeleman & Weerman, Reference Neeleman and Weerman1997; Schwartz & Sprouse, Reference Schwartz, Sprouse, Hoekstra and Schwartz1994); after learner’s exposure to L2 input, L1 background affects the perceived difficulty of target structures (e.g., Andringa et al., Reference Andringa, de Glopper and Hacquebord2011; MacWhinney et al., Reference MacWhinney, Bates and Kliegl1984) and influences the sequence of L2 acquisition order (e.g., Della Putta, Reference Della Putta2016; Ellis, Reference Ellis2006). Studies also show that L1 syntactic knowledge continues to be activated in highly proficient late bilinguals’ processing of L2 constructions (Hwang et al., Reference Hwang, Shin and Hartsuiker2018; Luke et al., Reference Luke, Liu, Wai, Wan and Tan2002).
Despite the attention L1 transfer has received in the studies of natural language instruction, it remains understudied in semiartificial language learning (Hamrick & Sachs, Reference Hamrick and Sachs2018). As an alternative paradigm to artificial grammar learning, semiartificial language learning combines novel structures with the lexical information from the participants’ L1 (Williams, Reference Williams and Hinkel2005). It is argued that this paradigm can both control for exposure and engage participants in meaningful tasks, thereby preserving the strengths of artificial grammar learning while better simulating the cognitive processes in learning novel structures in natural languages (Tagarelli et al., Reference Tagarelli, Ruiz, Moreno Vega and Rebuschat2016; for an example of early artificial grammar learning research see Reber et al., Reference Reber, Kassin, Lewis and Cantor1980). However, as researchers pointed out, semiartificial language systems draw on multiple phonological/semantic cues from the participants’ L1, which may create challenges that have not been sufficiently addressed (Hamrick & Sachs, Reference Hamrick and Sachs2018) or lead to increased saliency that could influence and potentially alter the cognitive processes underlying natural language learning (e.g., Godfroid, Reference Godfroid2016; Leow, Reference Leow2019; Maie & DeKeyser, Reference Maie and DeKeyser2020).
So far two studies have addressed the possible impact of L1 background on the learning of semiartificial structures with different degrees of complexity. Using a semiartificial language (“Japlish”) that combines Japanese word order with English lexicon, Williams and Kuribara (Reference Williams and Kuribara2008) investigated how incidental exposure affects English native speakers’ learning of the SOV word order (the “canonical” order in Japlish) and of the “scrambled” sentence patterns (the “marked” order in Japlish). They found that (a) the no-exposure group showed an above-chance acceptance of the canonical SOV word order after very limited exposure; (b) the exposure group showed a statistical advantage over the no-exposure control on two trained sentence patterns (one canonical and the other scrambled) but did not show any advantage on other sentence patterns, be they trained or new, canonical, or scrambled (the exposure group even showed significantly lower acceptance rates on trained short scrambling), and (c) only a subset of the incidental exposure group showed signs of acquiring scrambling, but these participants did not perform above chance in rejecting structures that are grammatical in English syntax but illegitimate in Japlish. As a follow-up of Williams and Kuribara (Reference Williams and Kuribara2008), Williams (Reference Williams2010, experiment 1) repeated the procedures in the original study but doubled the amount of exposure. The results were largely consistent with those of Williams and Kuribara (Reference Williams and Kuribara2008), except that the learning gains for the trained scrambles were shown in the increased-exposure group only.
The results of the two studies show that L1 syntactic knowledge may play a vital role in the learning of semiartificial language structures. It should be noted, nevertheless, that neither Williams and Kuribara (Reference Williams and Kuribara2008) nor Williams (Reference Williams2010) incorporated explicit instruction; it is not clear whether learners’ L1 experience would moderate the intake of explicit instruction in the same way as it does that of incidental exposure. However, studies such as Tagarelli et al. (Reference Tagarelli, Ruiz, Moreno Vega and Rebuschat2016) that did compare explicit instruction with incidental exposure only included learners’ L1 experience as background information without addressing the possible role of L1 transfer in semiartificial grammar learning. By systematically changing the L1 background from English to a typologically different language—in our context, Chinese—we may investigate the generalizability of Tagarelli and colleagues’ findings about the relation between linguistic complexity, exposure condition, and learning outcomes, thereby shedding new lights on the neglected area of L1 transfer in semiartificial grammar learning.
In the following section, we first reanalyze the rules of the semiartificial language system and the stimuli used by Tagarelli et al. (Reference Tagarelli, Ruiz, Moreno Vega and Rebuschat2016) by comparing German, Chinese, and English word order. On the basis of the initial analysis, we predict the outcomes of this conceptual replication.
RULES AND STIMULI IN TAGARELLI et al. (Reference Tagarelli, Ruiz, Moreno Vega and Rebuschat2016) REVISITED: THE WORD ORDER ISSUE
The following are the three target rules and the sample sentences included in Tagarelli et al. (Reference Tagarelli, Ruiz, Moreno Vega and Rebuschat2016):
-
1. Simple: The finite verb is placed in the second phrasal position of main clauses that are not preceded by a subordinate clause (V2).
e.g., Yesterday scribbled David a long letter to his family.
Adjunct–Verb–Subject–Object–Adjunct
-
2. Complex 1: The finite verb is placed in the final position of all subordinate clauses (V2-VF).
e.g., In the afternoon acknowledged David that her children to England moved .
Adjunct–Verb 1–Subject 1–Subject 2–Adjunct–Verb2
-
3. Complex 2: When a subordinate clause precedes a main clause, the finite verb is placed in the first position of the main clause and the final position of the subordinate clause (VF-V1).
e.g., When his wife in the afternoon the office left , prepared Jim dinner for the entire family.
Subject 1–Adjunct–Object 1–Verb 1–Verb 2–Subject 2–Object 2–Adjunct
The German word order as exemplified in these example sentences departs from the typical Chinese and English word order in a number of ways. Drawing from the relevant findings of typological, corpus, and syntactic studies, we summarized the relevant features of German, English, and Chinese word order in S1 in the Supplementary Materials. On the basis of the literature-based analysis, we differentiated the grammaticality of major clause types of the training and test stimuli in German, Chinese and English as follows (Table 1):
TABLE 1. Grammaticality of sentence patterns in training and test stimuli in German/English/Chinese
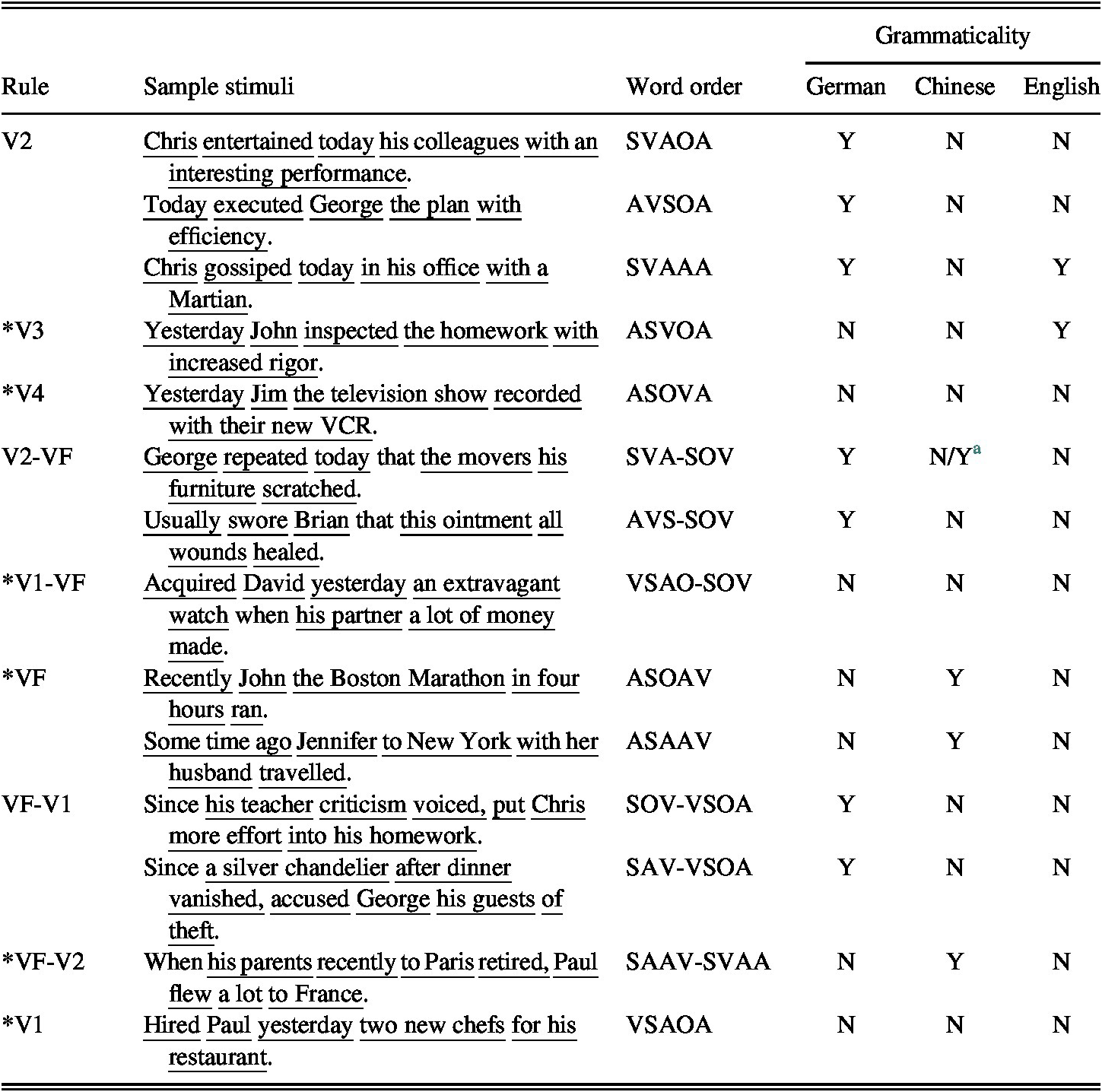
Note: S = Subject; V = Verb; O = Object; A = Adjunct.
a The word order of the main clause (VA) is ungrammatical in Chinese, but the lack of syntactic markers in Chinese and the aural mode make it possible for participants to misparse the adjunct as part of the subordinate clause, thereby making the stimuli sound grammatical in Chinese. From the initial analysis of the stimuli we may draw two observations. First, Chinese is the only language in the world that places the adjunct before the verb (Dryer & Gensler, Reference Dryer, Gensler, Dryer and Haspelmath2005), which results in its proximity to the VF part of the complex, less salient V2-VF rule. At the same time, as Chinese is also an SV language, many Chinese sentences have their verbs placed in the third position or even later (e.g., SAV; SAOV), which may make it more difficult for L1-Chinese learners to acquire the simple V2 rule. Second, the sentences grouped under the same rule (e.g., VF-V1, *VF-V2, and *V1) or even those grouped into the same sentence pattern (AVSOA and SVAAA under V2) may differ from each other in terms of their closeness to Chinese/English syntax, thus possibly leading to different perceived difficultyFootnote 4 for Chinese and English native speakers. These observations suggest that there would possibly be increased variability at the learner and item levels in the changed L1 background, which could be concealed by an analysis on the aggregated data (e.g., the mixed-effects repeated measures ANOVA that the original study adopted). It is therefore both important and necessary to contextualize the findings about group-level variation (i.e., the fixed effects) against random variability (i.e., the random effects) to gain a better understanding of the generalizability of such findings in the changed L1 background, specifically, L1-Chinese.
Based on this initial analysis, we put forward the following research questions:
RQ1: To what extent can Tagarelli et al.’s (Reference Tagarelli, Ruiz, Moreno Vega and Rebuschat2016) findings about the relation between exposure condition, linguistic complexity, and grammar learning be replicated and generalized with a different L1 background, specifically, L1-Chinese?
RQ2: How does the group-level variation based on exposure condition and linguistic complexity (the fixed effects) compare with the variability at individual learner and item levels (the random effects)?
Our predictions of the possible outcomes are as follows:
-
1a. Based on the findings of previous experimental and meta-analytical research (e.g., Andringa et al., Reference Andringa, de Glopper and Hacquebord2011; Goo et al., Reference Goo, Granena, Yilmaz, Novella and Rebuschat2015), we predict a main effect for exposure condition, with learners receiving explicit instruction outperforming those receiving incidental exposure;
-
1b. Based on the findings of previous typological, syntactic, psycholinguistic and corpus studies (e.g., Dryer & Gensler, Reference Dryer, Gensler, Dryer and Haspelmath2005; Rankin, Reference Rankin2014; Williams, Reference Williams2010), we predict a condition × complexity interaction with the L1-Chinese background, with L1-Chinese learners perceiving the simple V2 rule as more difficult and the complex V2-VF rule as less difficult; and
-
2. On the same basis, we predict a considerable variability at individual learner and item levels compared with the group-level variation based on exposure condition, linguistic complexity, and their interaction (if any).
METHOD
PARTICIPANTS
The data were collected at a public university in China. Participants were 84 Chinese native speakers with no background in German or any other V2 languages. The participants were randomly assigned to an incidental exposure condition (n = 41, 35 female, Mage = 19.10) or an instructed condition (n = 43, 41 female, Mage = 19.23). An a priori power analysis using G*Power 3.1 (Faul et al., Reference Faul, Erdfelder, Buchner and Lang2009) showed that the sample size is well-powered (for the power analysis see S2-1).
The mean age and gender distribution of our participants are similar to those of the participants in the original study (for further participant information see S2-2). Consistent with the original study, the participants in our study received course credit in return.
DESIGN
The study design, procedure, and instruments were exactly as reported by Tagarelli et al. (Reference Tagarelli, Ruiz, Moreno Vega and Rebuschat2016), except that (a) the training and test stimuli were translated into Chinese, and (b) we incorporated an additional question in the debriefing questionnaire to elicit the participants’ production data (see S4). As with the original study, the design included a session of artificial language training and testing and a session of cognitive tasks (the operationalization and the results of the cognitive tasks will be reported elsewhere). In the following, we will briefly describe the design, with special emphasis on the two aspects of the design in which our study differed from the original study.
Pretraining Instruction, Training, and Testing
In the three sessions of pretraining instruction, training, and testing, we adopted the same procedures as in the original study.
The pretraining instruction was administered to the instructed group only. During this session, the participants were told that the scrambled sentences they would hear followed three rules of word order. They were then presented visually with the three rules illustrated with two sentence examples, respectively. After each rule was presented, the participants were required to produce two sentences according to the rule, but they received no feedback. All the participants in the instructed group finished the pretraining session within 15 minutes.
During the training phase, both the incidental and the instructed groups were auditorily presented with the Chinese translations of the 120 stimuli used in Tagarelli et al. (Reference Tagarelli, Ruiz, Moreno Vega and Rebuschat2016) in random order. Neither group was told that there would be a testing phase. The participants in the incidental group were told that they were tested on their ability to judge the plausibility of sentences with scrambled word order to keep their attention away from the linguistic targets. In contrast, the participants in the instructed group were told to both judge the plausibility of the scrambled sentences and reflect over the rules they had learned.
The training stimuli were evenly distributed in plausibility and across the three rules, with 20 plausible and 20 implausible sentences for each syntactic pattern (V2; V2-VF; VF-V1). After hearing each sentence, participants were asked to indicate its plausibility by clicking on a “Plausible” or “Implausible” button on the screen and orally repeat the sentence. Feedback was immediately provided on each of the plausibility judgments. As with the original study, participants would have to process the entire auditory string before judging its plausibility. See the following stimuli for example:
Plausible:
小乔 重申了 在今天,搬运工 他的家具 划坏了。
George repeated today that the movers his furniture scratched.
Implausible:
小乔 切开了 在几个月前 菜谱 用桃子。
George laminated a few months ago the recipe cards with peaches.
During the GJT phase, the participants were either told (incidental group) or reminded (instructed group) that the “scrambled” word order of the sentences in the training phase followed a certain rule system, and that they should judge the grammaticality of the new sentences based on the ones they had heard in the training. Identical to the original study, participants first completed four practice trials (wherein no feedback was provided) and were then auditorily presented with 60 new sentences (30 grammatical and 30 ungrammatical) in random order. The testing set included 10 grammatical sentences for each rule (V2, V2-VF, and VF-V1) and 5 sentences for each ungrammatical pattern (*V3, *V4, *V1-VF, *VF, *VF-V2, and *V1). For each sentence, participants were asked to click on the appropriate buttons on the screen to judge the sentence’s grammaticality (grammatical/ungrammatical), indicate how confident they were in the accuracy of their judgment (not at all confident, somewhat confident, quite confident, and extremely confident), and report the source of their judgment (guess, intuition, memory, and rule).Footnote 5
Translation of the Training and Test Stimuli
We translated all the training and test stimuli into Chinese (see S9). Care was taken so that the translated stimuli follow the exact word order of the original stimuli. To prevent barriers to comprehension, all the common English names were substituted with common Chinese names and the lexical items that require certain culture-specific knowledge were replaced with general ones.Footnote 6 See the following list of examples:
V2: Chris entertained today his colleagues with an interesting performance. (SVAOA)
小李 招待了 在今天 他同事 一场演出。(SVAOA)
V2-VF: Paul speculated yesterday that the suspect from prison escaped . (SVA-SAV)
小罗 怀疑 在昨天,嫌疑犯 从监狱 逃脱了 。(SVA-SAV)
VF-V1: When his wife in the afternoon the office building left , prepared Jim dinner for the entire family. (SAOV-VSOA)
当 小金的妻子 在下午 办公楼 离开了 , 准备了 小金 晚餐 为全家人。(SAOV-VSOA)
The translations were proofread by two professional Chinese-L1 translators and one English-L1, Chinese-L2 bilingual, who agreed that every translated stimulus was accurate in terms of both lexical information and word order.
Debriefing
After all the participants completed the GJT, they were given a debriefing questionnaire in paper form. All participants completed the debriefing questionnaire within 20 minutes. In the questionnaire, we asked the participants to describe the rules they had inferred from the practice as Tagarelli et al. (Reference Tagarelli, Ruiz, Moreno Vega and Rebuschat2016) did; additionally, we also listed 10 expressions (see S4) and invited the participants to create as many grammatical sentences as they could with any number of these expressions, according to the rules they inferred from the training. The list of expressions included four NPs (Xiaoliang, Xiaoliang’s aunt, the oven, and bread), three verbs (fixed, bought, and said), two adjuncts (in a hurry and at eight last night), and one adverbial subordinator (since). The production data enabled us to triangulate the findings about the GJT data without demanding a high level of metalinguistic awareness on the part of the participants.
Cognitive Tasks
Following the original study, we arranged a separate session to test on the participants’ working memory (WM) and procedural learning ability. The specific operationalization and results of the cognitive tasks will be reported elsewhere.
OUTCOME MEASURES AND ANALYSIS
Following Tagarelli et al. (Reference Tagarelli, Ruiz, Moreno Vega and Rebuschat2016), our outcome measures included the accuracy rates and the d’ scores on the GJT (with the d’ scores controlling for response bias and reflecting the participants’ sensitivity), the subjective measures of awareness (i.e., source attributions and confidence ratings, to be reported elsewhere), and the outcomes of the cognitive tasks (which will be reported elsewhere). In addition to these measures, we also included the sentence production data to triangulate the findings about the GJT. The sentences were first manually coded by both researchers according to syntactic complexity and verb positioning. For instance, “Xiaoliang at eight last night repaired the oven” was coded V3, as it was a simple sentence with the verb appearing as the third element. “At eight last night Xiaoliang said Xiaoliang’s aunt the oven had repaired” was coded VFVF because it was a complex sentence with the verb in the main and subordinate clauses appearing as the last element. The two researchers then rated the grammaticality of each coded sequence based on the semiartificial grammar. The interrater reliability for both the coding and the grammaticality ratings was 100%.
Our RQ1 was whether Tagarelli and colleagues’ findings about exposure condition, linguistic complexity, and grammar learning would be replicated and generalized in a different L1 background, specifically, L1-Chinese. To address RQ1, we performed (a) a robust independent-samples t-test to compare the groups’ overall performance in the GJT,Footnote 7 and (b) a robust 2 × 3 between-within subjects ANOVA on trimmed means (20% trimming level) with Condition (Incidental vs. Instructed) as the between-subject variable and Complexity (Simple, Complex 1, and Complex 2) as the within-subject variable to examine the main effects and possible interactions.Footnote 8
Our RQ2 was how the individual-level variability in the raw data compared with the group-level variation based on condition and linguistic complexity. To address RQ2, we performed a mixed-effects modeling analysis to estimate both fixed and random effects (i.e., with a random slope and intercept for Subject and a random intercept for Item) based on the raw accuracy data and compared the results with those on the mean-based aggregated data.
The effect sizes of Cohen’s d were interpreted on the basis of Plonsky and Oswald (Reference Plonsky and Oswald2014), namely, d = 0.60, 1.00, and 1.40 corresponding to small, medium, and large effect sizes. The explanatory measure of effect size ξ was interpreted based on Mair and Wilcox (Reference Mair and Wilcox2020, p. 10), namely, ξ̂ = 0.10, 0.30, and 0.50 corresponding to small, medium, and large effect sizes.
RESULTS
RQ1: RELATIONSHIP BETWEEN EXPOSURE CONDITION AND SYNTACTIC COMPLEXITY
As with the original study, we adopted the outcome measures of accuracy rates and d’ scores. See Table 2 for descriptive statistics on the two outcome measures and Figure 1 for a graph of the mean differences on the d’ scores for group and complexity.
TABLE 2. Accuracy (%) and d’ scores on the GJT for each group overall and according to complexity

Note: Significance from chance: + p = .055; * p < .05; ** p < .01; *** p < .001.
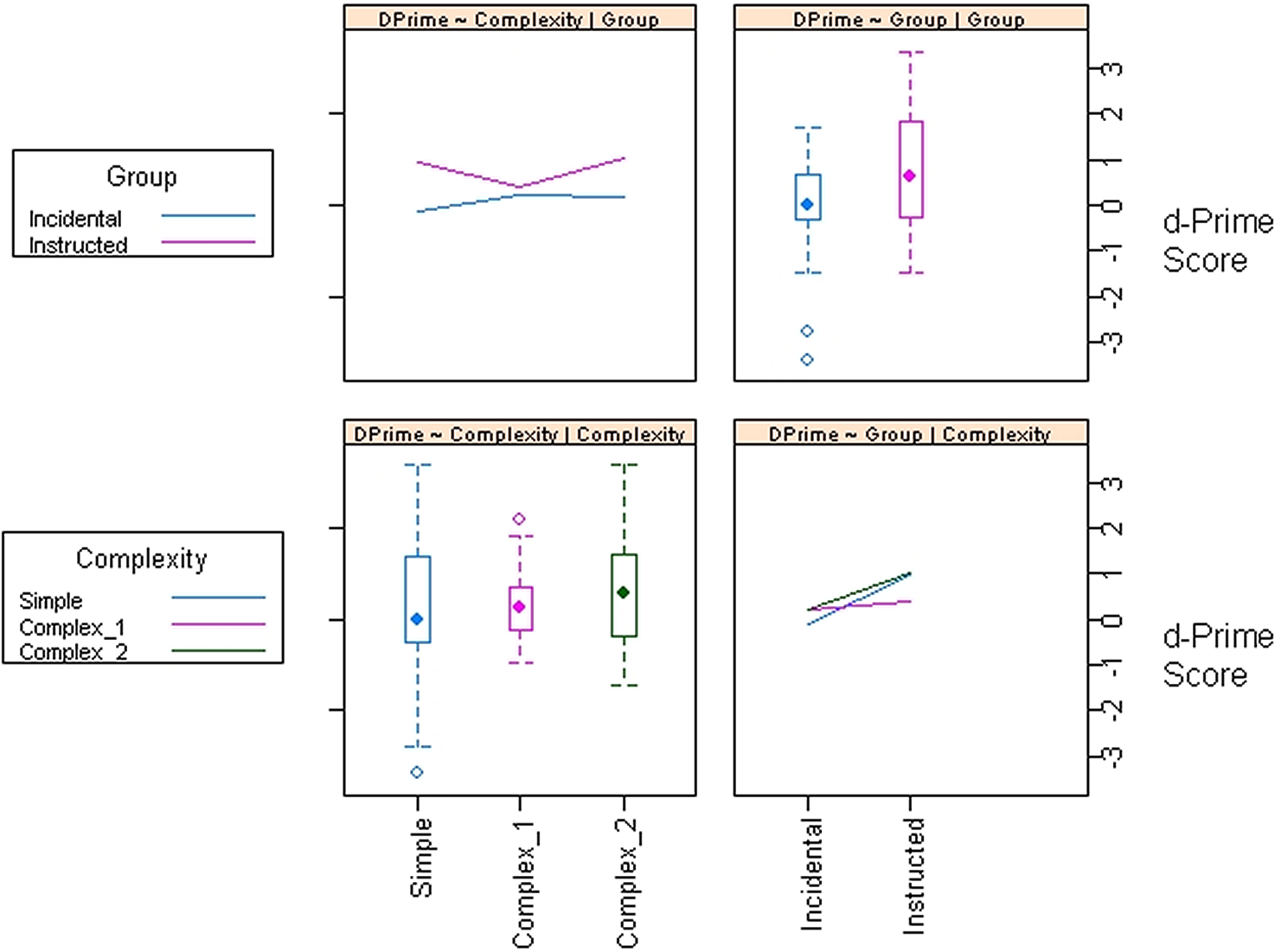
FIGURE 1. Effects of group and complexity on GJT performance.
As shown in Table 2, the 95% confidence intervals (CIs) for the incidental group’s overall d’ scores spanned 0 (0 indicating no discrimination ability), whereas those for the instructed group’s overall d’ scores did not. These results indicated that only the instructed condition performed above chance overall. Moreover, there was no overlapping in CIs between the two groups overall and for two of the three target structures, indicating an advantage of explicit instruction at large. A robust independent samples t-test on trimmed means (20% trimming level) showed that the instructed group performed statistically better than the incidental group overall, t(40.39) = 2.37, p = .023, with a large explanatory measure of effect size, ξ = 0.43 [0.08, 0.71]. The effect size of the between-group difference in overall GJT performance in our study (g[CI] = 0.87 [0.42, 1.32]) is consistent with the calculated effect size in Tagarelli et al. (Reference Tagarelli, Ruiz, Moreno Vega and Rebuschat2016) (g[CI] = 0.86 [0.28, 1.44]).
The structure-specific data in our study exhibited largely similar patterns to those in the original study regarding the role of exposure condition, but the different slopes of the two groups’ performance across the three structures (Figure 1) suggested a potential exposure condition × complexity interaction. To ascertain whether such an interaction existed, we performed a robust 2 × 3 between-within subjects ANOVA (20% trimming level) with Condition as the between-subject variable (Incidental vs. Instructed) and Complexity as the within-subject variable (Simple, Complex 1, and Complex 2). The results showed that the interaction between Condition and Complexity was statistically significant, F(2, 29.55) = 4.16, p = .026, and so was the main effect of Condition, F(1, 31.32) = 9.73, p = .004. The effect of Complexity was nonsignificant, F(2, 29.55) = 1.49, p = .242. We performed post hoc pairwise comparisons on trimmed means (20% trimming level), with the 95% CIs and p-values adjusted for multiple testing (p = .05/6 = .008). See Table 3 for the results:
TABLE 3. Post hoc pairwise comparisons on trimmed means (20% trimming level)

As shown in Table 3, before the p-value adjustment the instructed group outperformed the incidental group on both the Simple and Complex 2 structures, exhibiting a similar pattern as observed in the 95% CIs in Figure 1. We may also observe that compared with the instructed group, the incidental group scored significantly higher on the Complex 1 structure than on the Simple structure, which lent support to our prediction that the perceived difficulty of the structures would vary in the changed L1.
However, after the adjustment, the two groups differed significantly only on the Simple structure, with the instructed group outperforming the incidental, ψ̂ = −1.01 [−1.73, −0.30], p = .007. The difference between the incidental and instructed groups also remained statistically significant, ψ̂ = −1.97 [−3.00, −0.94], p < .001. These results supported our initial prediction about the interaction effect between condition and complexity.
We now summarize our findings about RQ1 in Table 4:
TABLE 4. Summary of findings on RQ1: Original study and replication contrasted

The findings of the GJT data analysis were both corroborated and enriched by an examination of the sentence production data. Six participants (incidental: 2, instructed: 4) left this part blank. The other participants produced a varied number of sentences from 1 or 2 sentences to as many as 16 sentences. See Table 5 for an overview of the sentence production data:
TABLE 5. Overview of the sentence-production data

a Patterns comprising 5+ instances are included as labeled types. For patterns with below five tokens, see S7-1.
b This pattern put the main clause before the subordinate clause.
From Table 5 we may draw the following observations. First, the instructed group exhibited substantially greater learning outcomes than the incidental group (compare the proportion of targetlike sentences produced by both groups), thus supporting Prediction 1a about the advantage of instruction over incidental exposure; second, the untarget-like sentences produced by the incidental group tended to place the verbs toward the end, suggesting the impact of L1 transfer on this group. Moreover, this group produced more ungrammatical V2 sentences (*V3: 23; *V4: 18) than grammatical ones (14), thus supporting Prediction 1b about the condition × complexity interaction. Third, the untarget-like sentences produced by the instructed group put the verbs in the first, second, or last places, all of which were contained in the rules taught to this group. These instances of rule overgeneralization indicated that the participants at the early stage of development failed to associate verb placement with syntactic complexity and clause sequence.
To sum up, our hypotheses regarding the effect of exposure condition, linguistic complexity, and the interaction between the two variables in the L1-Chinese background were supported.
RQ2: FIXED VS. RANDOM EFFECTS
So far we have explored the group-level patterns in the aggregated data following Tagarelli et al. (Reference Tagarelli, Ruiz, Moreno Vega and Rebuschat2016)’s methods of analysis. However, collapsing data could conceal the variability at individual learner and item levels. For the L1-Chinese learners in our study, the problem with random variability could be particularly prominent, as the test stimuli included ungrammatical sentence patterns (*V3, *V4, *VF, *VF-V2) that could be grammatical in Chinese due to the language’s preference of verb postponement. Given these particularities, it is both important and necessary to contextualize our discussions of the fixed effects (i.e., exposure condition, linguistic exposure, and their interaction) against a more accurate measurement of the random effects (i.e., variability at the individual learner and item levels). One method to realize this goal is applying mixed-effects modeling on raw, nested data, whose technique has been adopted by an increasing number of SLA studies (Dörnyei, Reference Dörnyei2009; Hui, Reference Hui2020; Mukarami, Reference Mukarami2016; Tao & Williams, Reference Tao and Williams2018; see Linck & Cunnings, Reference Linck and Cunnings2015 for the utility of the method in SLA research).
We ran binary mixed-effects logistic regressions using the lme4 package in R (Bates et al., Reference Bates, Maechler, Bolker and Walker2015). For the fixed effects we included Condition (Incidental and Instructed), Complexity (Simple, Complex 1, and Complex 2), and Grammaticality (Grammatical and Ungrammatical), with Grammaticality added because difficulty of GJTs may differ depending on whether the item sentences are grammatical or ungrammatical (e.g., R. Ellis, Reference Ellis2005).Footnote 9 For the random effects, we included random intercepts for items and random slopes and random intercepts for participants.Footnote 10 Regarding model selection, we mainly adopted James et al.’s (Reference James, Witten, Hastie and Tibshirani2013) method by building the unconditional model first (i.e., a model that included the random-effects variables only), gradually adding predictors that decreased the model’s Akaike information criterion (AIC) the most, and considering interaction terms only when the model already included the main effects constituting the interactions. The model comparisons are summarized in Table 6.
TABLE 6. Summary of binary mixed-effects logistic regressions model comparisons

As shown in Table 6, AIC categorically decreased until Model 5, and likelihood ratio tests suggested a steady improvement until Model 5. Considering that complexity may possibly improve the model after other variables are entered, we included complexity into the fixed-effects variables on the basis of Model 5. However, the addition of this parameter did not reduce the AIC scores significantly (Model 6).
To ensure the predictor variables were not collinear with each other, we diagnosed their corresponding VIF values that were obtained by the vif() function in the car package (Fox et al., Reference Fox, Weisberg, Price, Adler, Bates and Baud-Bovy2019). The results for Model 4 were acceptable (condition: vif = 1.00, grammaticality: vif = 1.00), while those for Model 5 were not (condition: vif = 1.85, grammaticality: vif = 3.41, grammaticality × condition: vif = 4.24).
Although Model 5 was a significant improvement on Model 4, we selected Model 4 as the final model. First, the decrease of AIC from Model 4 to Model 5 was fairly small (2.21) and the p value of the added parameter (.040) was not as low as the p values of other parameters; second, Model 4 is less complex than Model 5 and AIC tends to prefer more complex models in general (Held & Bové, Reference Held and Bové2014); and third, similar decisions were made by previous L2 research (e.g., Mukarami, Reference Mukarami2016).
See Table 7 for a summary of Model 4 (for the purpose of comparison, see S8 for a summary of Model 5).
TABLE 7. Summary of Model 4

a Both theoretical and observation-level (delta) values were reported for reproducibility.
From Table 7 we may draw the following observations. First, although Model 4 explained a medium proportion of the variance (theoretical: 28%; observation-level: 24%), most of the variance was attributable to random effects (theoretical: 23%; observation-level: 20%). The fixed effects (condition and grammaticality), significant predictors as they were, only accounted for a small proportion of the variance of the raw accuracy data (theoretical: 5%; observation level: 4%). Second, consistent with the findings about the aggregated data, linguistic complexity was not a significant predictor. Third, the analysis on the raw data differed from the group-level analysis in that the best-fit model did not include any interaction effect. Last but not least, the analysis on the raw data revealed that the by-learner random slope for grammaticality accounted for large variances of the participants’ accuracy rate on the logit scale (note the corresponding large SD in the model summary), indicating that the individual participants responded to grammatical(/ungrammatical) items very differently from each other. This finding is consistent with the wide dispersion of the individual d-prime scores in Figure 1 and points at the necessity to further investigate the random variability at individual participant (and item) levels.
With these observations we now summarize the findings about RQ2 in Table 8.
TABLE 8. Summary of findings about RQ2: original study and this study contrasted

SUMMARY OF FINDINGS AND DISCUSSION
Despite the extensive attention accorded to L1 transfer in natural language instruction, the factor is understudied in semiartificial language learning. In this study, we conceptually replicated Tagarelli et al. (Reference Tagarelli, Ruiz, Moreno Vega and Rebuschat2016) by recruiting L1-Chinese participants, translating the stimuli to Chinese, and eliciting language production data during debriefing, to investigate how L1 transfer moderates the interaction between linguistic complexity and exposure condition in semiartificial grammar learning. We also extended the initial study by using a binary mixed-effects logistic regression analysis to contextualize our findings about the fixed effects against the random effects. Our major findings are as follows: (a) in the changed L1 background explicit instruction continued to exhibit an advantage over incidental exposure overall and on the simple structure; (b) the analysis on the GJT data showed that linguistic complexity was not a significant predictor in the L1-Chinese background; (c) the analysis on sentence production data confirmed that the instructed group performed better than the incidental group, with negative L1 transfer affecting the incidental group more than the instructed group; (d) the mixed-effects modeling analysis supported the findings of the group-level analysis regarding the advantage of the explicit over the incidental condition and the nonsignificance of linguistic complexity; however, it did not find the condition × complexity interaction effect; and (e) the mixed-effects models revealed that the variation across individual participants and items accounted for a bigger portion of the outcome variance than the fixed effects combined.
Our interpretation of the results addresses three separate questions. First, explicit instruction still exhibited an overall advantage over incidental exposure even when the L1 background changed to a typologically different language. This finding lends support to the conclusion of the original study about the comparative efficacy of explicit instruction and corroborates with the findings of numerous experimental studies comparing the explicit with the incidental conditions (e.g., de Graaff, Reference de Graaff1997; Robinson, Reference Robinson1996) and meta-analytical research (e.g., Goo et al., Reference Goo, Granena, Yilmaz, Novella and Rebuschat2015; Spada & Tomita, Reference Spada and Tomita2010). Meanwhile, our results contrast with the findings of Morgan-Short et al. (Reference Morgan-Short, Steinhauer, Sanz and Ullman2012) and of Kang et al. (Reference Kang, Sok and Han2018). The contrast may be attributable to two factors, namely, the length and intensity of the treatment and the type of measures. First, the participants in our study were exposed to one training session consisting of 120 stimuli items before they took the judgment task. The limited length and intensity of exposure may have placed the incidental group at a disadvantage, for learning under implicit conditions is thought to take longer than under explicit conditions (N. C. Ellis, Reference Ellis2005). Second, researchers have argued that GJTs, even timed ones, tap into (automatized) explicit L2 knowledge rather than implicit L2 knowledge (e.g., Maie, Reference Maie2020; Suzuki, Reference Suzuki2017; Vafaae et al., Reference Vafaee, Suzuki and Kachinske2017) because they direct learners’ attention away from meaningful communication to formal accuracy (e.g., Suzuki & DeKeyser, Reference Suzuki and DeKeyser2017). Regarding the sentence production data, our form-focusing instruction about conforming to the rules of the language system may have heightened participants’ awareness, and as a result prompted the participants to use their explicit knowledge as well. Importantly, we did not include an analysis or discussion of the subjective measures of awareness in this study due to space limitations; a follow-up examination of these measures will undoubtedly offer valuable insights into the particular types of knowledge to which the different exposure conditions gave rise. We therefore call for more research that (a) investigates higher-proficiency learners and (b) includes measures of both explicit and implicit knowledge to present a fuller picture of the knowledge gained through the different conditions.
Second, our analysis of the group-level variation revealed no main effects of complexity, which departed from the findings of the original study in the L1-English context. The contrastive findings between our study and Tagarelli et al. (Reference Tagarelli, Ruiz, Moreno Vega and Rebuschat2016) help to determine the limits of generalizability of the conclusion of the original study about the role of complexity in L2 learning. As our analysis of sentence production data showed, L1 syntax transfer affected the incidental group more than the instructed group: on the one hand, the preference of Chinese syntax for postponed verb placement interfered with the incidental group’s intake of the V2 rule, leading to the group’s at-chance performance on this simple structure. On the other hand, some of the stimuli for the complex V2-VF sentence pattern are grammatical in Chinese, which could have contributed to the incidental group’s relatively high retention rate of this structure. These findings indicate that varying L1-L2 proximity could differentially affect the perceived L2 difficulty of simple/complex structures under different learning conditions, thereby rendering the effect of linguistic complexity less evident (for the instructed condition) or even offsetting the latter (for the incidental condition). This finding is corroborated by previous studies on natural language instruction that revealed the role of L1 background in perceived L2 difficulty (e.g., Della Putta, Reference Della Putta2016; González & Hernández, Reference González and Hernández2018). We hence call for more attention to the role of L1 transfer in discussions of the generalizability of findings regarding linguistic complexity, particularly in the field of semiartificial grammar learning.
Third, our analysis of the variability at individual learner and item levels confirmed the main effect of exposure condition, but negated the condition × complexity interaction found in the analysis on group-level variation. Moreover, the analysis revealed that the fixed effects, significant predictors as they were, accounted for a relatively small portion of the outcome variance compared with the random effects. These findings draw attention to the importance of accounting for learner- and item-level individual variation and point at the limitation of the group-level analysis methods adopted by the original study. On the basis of our results and echoing calls of researchers such as DeKeyser (Reference DeKeyser2012), we call for more interaction research and recommend that researchers compare the fixed effects with the random effects to contextualize the findings.
Importantly, although Tao and Williams (Reference Tao and Williams2018) also applied mixed-effects modeling to the analysis of raw data, they did it with a view to controlling the random effects without comparing the fixed effects with the random effects regarding the amount of variance of learning outcomes they explained (see Mukarami, Reference Mukarami2016 for a contrastive practice, namely, interpreting the random effects by plotting and summarizing). We hence call for more systematic investigations into the variability of the data at individual levels while controlling for random effects in discussions of the significance of the predictors.
CONCLUSION
In this study, we conceptually replicated and extended Tagarelli et al. (Reference Tagarelli, Ruiz, Moreno Vega and Rebuschat2016) and examined whether exposure condition affected the learnability of simple/complex structures in similar ways when the L1 background is changed to Chinese, a language typologically different from English (the L1 in the original study) and German (the target syntax). Our results showed that the effect of exposure condition remained a significant predictor of the outcome variance despite the changed L1 background, with explicit instruction resulting in better overall performance than incidental exposure. This lends support to the conclusion of the original study about the comparative efficacy of the explicit condition over the incidental one. Meanwhile, our results showed that the changed L1-L2 proximity could have interfered with the incidental group’s intake of the simple and complex structures, rendering the effect of complexity nonsignificant. This finding limits the generalizability of the conclusion of the original study about the effect of linguistic complexity. Last, the analysis on the raw data supplemented the group-level analysis in important ways and revealed that the fixed effects played a smaller part than the random effects, thus bringing attention to the importance of individual variation in discussions of fixed effects.
In this study, we did not include a control group. However, as Leow (Reference Leow2019) and Williams (Reference Williams2010) have pointed out, learners with no exposure do not necessarily perform at chance. Future research may expand on our findings by incorporating both L1-Chinese and L1-English control groups to further explore the role of learners’ L1 experience, thereby both redressing the limitations of reliance on chance performance and providing further evidence of the role of L1 transfer in semiartificial grammar learning. We also call for the adoption of measures of both implicit and explicit knowledge to present a fuller picture of the learning processes and outcomes under the incidental/explicit conditions.
Supplementary Materials
To view supplementary material for this article, please visit http://dx.doi.org/10.1017/S0272263120000686.