Patient-reported outcome measures (PROMs), defined as “standardized, validated questionnaires that are completed by patients to measure their perceptions of their own functional status and wellbeing” (Dawson, Doll, Fitzpatrick, Jenkinson, & Carr, Reference Dawson, Doll, Fitzpatrick, Jenkinson and Carr2010), are increasingly used in the clinical and the academic contexts (Dawson et al., Reference Dawson, Doll, Fitzpatrick, Jenkinson and Carr2010; Higginson & Carr, Reference Higginson and Carr2001). Within them, health-related quality of life (HRQL) measures are widespread, as they allow obtaining information, both on illness and recovery processes (Frendl and Ware, Reference Frendl and Ware2014).
Specifically, in the area of geriatrics and gerontology, perceived health is recurrently measured, because of its role as a predictor of survival as well as a sign of well-being (Sargent-Cox, Anstey, & Luszcz, Reference Sargent-Cox, Anstey and Luszcz2008). Indeed, studies have repeatedly found evidence on the relation between perceived health for elderly’s psychological life satisfaction and well-being. Yet in 1996, subjective health was found as the single best predictor of life satisfaction (Mannell & Dupuis, Reference Mannell, Dupuis and Birren1996). Recent evidence also highlights its importance for a successful aging (Gutiérrez, Tomás, Galiana, Sancho, & Cebrià, Reference Gutiérrez, Tomás, Galiana, Sancho and Cebrià2013).
When it comes to its assessment, a wide range of measures for health and perceived health have been developed (i.e., Carter & Walker, Reference Carter and Walker2014; Infurna, Gerstorf, & Zarit, Reference Infurna, Gerstorf and Zarit2011). Good examples of them are the Perceived Health Competence scale (PHCS) (Smith, Wallston, & Smith, Reference Smith, Wallston and Smith1995), a brief measure of capacity of effectively managing the health outcomes, or the use of single indicators (for example, Raina, Bonnett, Waltner-Toews, Woodward, & Abernathy, Reference Raina, Bonnett, Waltner-Toews, Woodward and Abernathy1999). But, with no doubt, the “SF” family of tools are the ones that appear most often in the scientific literature (Turner-Bowker, Bartley, & Ware, Reference Turner-Bowker, Bartley and Ware2002).
The Short Form–36 Health Survey Questionnaire (SF–36) (Ware, Snow, Kosinski, & Gandek, Reference Ware, Snow, Kosinski and Gandek1993) was the first one of the SF scales to be developed, and it is a short, generic health survey, yielding a profile with eight subdimensions including functional health, well-being, physical, mental health, and utility information (Ware & Sherbourne, Reference Ware and Sherbourne1992). It has been used to measure perceived health with several purposes, such as postoperative recovery, colorectal surgery, alcohol-dependent problems, arthritis, psoriasis, peripheral arterial disease or chronic obstructive pulmonary disease, among others (for a review, see Frendl & Ware, Reference Frendl and Ware2014). The 12-item form, the SF–12, is an adaptation of the lengthier SF–36. This is still a generic questionnaire, assessing two general dimensions: Physical Health (PCS) and Mental Health (MCS). SF–12 has also been used to assess self-reported health in different contexts, such as breast cancer survivors (Treanor & Donnelly, Reference Treanor and Donnelly2015), microsurgery (Patel et al., Reference Patel, Economides, Franklin, Sosin, Attinger and Ducic2014), or sports (Boykin, Patterson, Briggs, Dee, & Philippon, Reference Boykin, Patterson, Briggs, Dee and Philippon2013). More recently, a shorter form of the SF has been presented. The SF–8 Health Survey the most recent version of the SF health surveys. It has been designed to provide a HRQL profile, with only 8 items (Ware, Kosinski, Dewey, & Gandek, Reference Ware, Kosinski, Dewey and Gandek2001). It represents an advance in SF applications, as it achieves both brevity and comprehensiveness in population health surveys, and has been used in several populations, such as migraine sufferers (Turner-Bowker, Bayliss, Ware, & Kosinski, Reference Turner-Bowker, Bayliss, Ware and Kosinski2003), chronically ill (Lefante, Harmon, Ashby, Barnard, & Webber, Reference Lefante, Harmon, Ashby, Barnard and Webber2005), or prostate cancer patients (Sugimoto, Takegami, Suzukamo, Fukuhara, & Kakehi, Reference Sugimoto, Takegami, Suzukamo, Fukuhara and Kakehi2008).
SF–36, SF–12, and SF–8 have been amply used in elderly populations (i.e., Gregorio et al., Reference Gregorio, Brindisi, Kleppinger, Sullivan, Mangano, Bihuniak and Insogna2014; Maust et al., Reference Maust, Chen, Benson, Mavandadi, Streim, DiFilippo and Oslin2015; Naseer & Fagerström, Reference Naseer and Fagerström2015; Neubauer et al., Reference Neubauer, Krawany, Leitner, Karlbauer, Wagner and Plecko2012; Orive et al., Reference Orive, Aguirre, García-Gutiérrez, Las Hayas, Bilbao, González and Quintana2015). But, whereas there is plentiful evidence on SF–36 and SF–12’s psychometric properties, research on SF–8 behavior is scarce, and it only includes four studies: the one by the developers of the reduced version (Ware et al., Reference Ware, Kosinski, Dewey and Gandek2001); one that took place in Uganda (Roberts, Browne, Ocaka, Oyok, & Sondorp, Reference Roberts, Browne, Ocaka, Oyok and Sondorp2008); another one carried out in Japan (Tokuda et al., Reference Tokuda, Okubo, Ohde, Jacobs, Takahashi, Omata and Fukui2009), and a fourth one developed in Spain (Vallès et al., Reference Vallès, Guilera, Briones, Gomar, Canet and Alonso2010). Ware et al. (Reference Ware, Kosinski, Dewey and Gandek2001) explored the construct validity of the scale by means of a principal component analysis in a sample of the American population. They found evidence for a physical factor (items 1 to 5) and a mental factor (items 6 to 8), with the vitality item (number 5) loading highly on both dimensions. In Uganda, Roberts et al. (2008) also used principal component analysis and found similar conclusions. The vitality item did not load highly on the mental health component; it only loaded high in the physical dimension. Tokuda et al. (Reference Tokuda, Okubo, Ohde, Jacobs, Takahashi, Omata and Fukui2009) studied the SF–8 in a sample of Japanese general population. They analyzed psychometric properties of the scale with a statistical model, Item Response theory, with important advantages over the Classical Test theory. However, this statistical model is based on strong assumptions, among them the unidimensionality and local independence of all items within a single scale. In order to test for these assumptions, these authors apparently performed a confirmatory factor analysis and “one factor was retained with an eigenvalue of 4.65 and variance proportion of .58, and no other factors exceeded unity” (Tokuda et al., Reference Tokuda, Okubo, Ohde, Jacobs, Takahashi, Omata and Fukui2009, p. 570). However, neither goodness-of-fit indices nor model comparison with the repeatedly found two-factor structure were provided, and with this lack of information it is quite difficult to know if the Japanese version of the SF–8 unidimensionality is tenable. Finally, Vallès et al. (2010) also studied some psychometric characteristics of the Spanish version of the SF–8, specifically reliability, convergent validity with clinical variables, and differential validity with socio-demographics. Nonetheless, they took factorial validity for granted, assuming the two-factor structure with physical and mental dimensions. In other words, they gave no evidence for the number of dimensions underlying the SF–8 scores.
With this state of the art in mind, the aim of current research is to further analyze the psychometric properties of the Spanish version of the SF–8. This research tries to overcome some of the shortcomings of the aforementioned studies, such as their exploratory analyses, the lack of factorial validity or the strong untested assumptions. In order to accomplish this objective a double line of analyses was used: a combination of competitive Structural Equations models to establish factorial validity, and Item Response theory to analyze item psychometric characteristics and scale information.
Method
Design, participants and procedure
Research approach is a panel design of older adults attending long life learning programs of the University of Valencia. Surveys took place during the academic year of 2014–2015. Participants were asked to answer the survey in their classroom setting, in sessions of about 30 minutes. These sessions were carried on in the presence of trained interviewers. First wave of the longitudinal study was used for this work. Education Ethics Committee gave its approval and all those attending the program were asked to give their informed consent. All students of first grade were invited to participate, with a response rate of 78%. The final sample consisted of 593 people aged 60 years old or older. Their age ranged from a minimum of 60 to a maximum of 92 years old, with a mean age of 67.36 years (SD = 5.83). 67.6% were women, most of them were retired (78.4%), some declared to be unemployed (5.5%), 4.1% were currently working, and others (mostly housekeepers) were 12%. Regarding their marital status, 64.6% were married, 17.9% were widows or widowers, 10.9% were single, and 6.6% divorced.
Measures
The survey included both demographic information and scales on personality dimensions, attitudes, perceptions, and behaviors related to the aging process. In this particular study, only data from the Short Form–8 Health Survey Questionnaire (Ware et al., Reference Ware, Kosinski, Dewey and Gandek2001) was used.
The SF–8 measures the same eight health domains as the SF–36 Health Survey with only eight questions. It has been designed to monitor population health and large-scale outcome studies, as it can be completed in one to two minutes. This HRQL measure provides a general measure of physical and mental health status (Ware et al., Reference Ware, Kosinski, Dewey and Gandek2001).
Additional to the SF–8 some other variables were used. These variables have been found to be consistently related (nomological net) to perceived health in older people, specifically life satisfaction and social support. To measure life satisfaction, the Temporal Life Satisfaction Scale (TSLS; Pavot & Diener, Reference Pavot and Diener1993) was used. The TSLS has 15 items and is composed of the original five items assessing global life satisfaction in the Satisfaction with Life Scale (SWLS; Diener, Emmons, Larsen, & Griffin, Reference Diener, Emmons, Larsen and Griffin1985) reworded to measure past, present and future life satisfaction alpha for the scale was .91. The original scale was Likert-type with five points. The Spanish version of the Duke–UNC–11 Functional Social Support Questionnaire (Bellón, Delgado, Luna, & Lardelli, Reference Bellón, Delgado, Luna and Lardelli1996) was used to assess social support.
Statistical analyses
Psychometric properties of the SF–8 were analyzed via two different statistical models, Confirmatory Factor Analysis (CFA), a procedure based on Classical Test theory (CTT), and Item Response theory (IRT) analyses. Several CFAs based on previous results and/or content validity considerations were estimated. These models were:
-
a) Model 1, one-factor (health) model. This model is based, among others, on Tokuda et al. (Reference Tokuda, Okubo, Ohde, Jacobs, Takahashi, Omata and Fukui2009).
-
b) Model 2, two factors, physical and mental health, based on the exploratory results by Ware et al. (Reference Ware, Kosinski, Dewey and Gandek2001) and also the dimensionality of the SF–12. In this model Item 5 “How much energy did you have?” was included in the physical dimension.
-
c) Model 3, two factors, physical and mental health, based on the dimensionality of the SF–12. In this model Item 5 was included in the mental dimension.
-
d) Model 4, two factors, physical and mental health, based on the dimensionality of the SF–12. In this model Item 5 was included both in the physical and mental dimensions.
All CFA were estimated in Mplus with WLSMV (Weighted Least Square Mean and Variance corrected) in order to accommodate the non-normality and ordinal nature of the items. Missing data were considered via Full Information Maximum Likelihood. Model fit was evaluated using several statistics and indices, specifically, the chi-square, the CFI, TLI and RMSEA. The following criteria were used to determine good fit: CFI and TLI above .90 (better if above .95) and RMSEA below .08 (better if below .05) (Marsh, Hau, & Wen, Reference Marsh, Hau and Wen2004). Additionally, to overall fit indexes, the acceptability of the model was evaluated by the strength and interpretability of the parameter estimates and the absence of large and substantively meaningful modification indices. Given that there were several competitive models and that ad-hoc modifications of these models were plausible, a cross-validation study was performed. The overall sample was randomly split into two samples, one used to develop the models (development sample) and the other one (cross-validation sample) to confirm that the best fitting model which could have been capitalized on chance was, again, the best one.
IRT analyses were also conducted with Mplus using the Graded Response Model (GRM; Samejima, Reference Samejima, van der Linden W. and Hambleton1997). Specifically, one-parameter and two-parameter logistic models (1PL and 2PL) were estimated with maximum likelihood and robust corrections and their relative fit to the data assessed. The graded response models (1PL and 2PL) were estimated in Mplus with a logit link function. These models are “one of the most popular IRT models to address polytomous data” (Hambleton, van der Linden, & Wells, Reference Hambleton, van der Linden, Wells, Nering and Ostini2010). 1PL and 2PL models estimate two types of parameters for each item: discrimination (a) and difficulty (b). Discrimination parameter (a) determines the slope by which responses to the items change as a function of the level in the “ability” or latent construct measured. The 1PL model constrains all discrimination estimates to the same value, whereas the 2PL model freely estimates discrimination for each item. These slopes (discrimination) typically range from 0 to 3, and values above 1.0 are considered highly discriminant. Item difficulty (b) parameters determine how challenging each item is. Given that the SF–8 employs a five-points rating scale, there are four response thresholds for each indicator. These thresholds indicate the level of the latent variable at which an individual has 50% chance of score at or above a particular response category. The 1PL and 2PL model fit was compared in order to decide which one had the best relative fit. For comparison purposes the usual fit statistics and indices were used (Raykov & Marcoulides, Reference Raykov and Marcoulides2011): first information criteria were used, specifically the Akaike Information Criterion (AIC) and Bayes Information Criterion (BIC) as well as its adjusted version (ABIC); second, being the 1PL and 2PL nested models their Likelihood Ratio tests (LRT) may be used to calculate a deviance test, if the two LRT do not differ the more parsimonious (1PL) model is preferred.
Amount of measurement error was also estimated, both from CTT and IRT frameworks. With respect to the CTT, the internal consistency of the SF–8 was estimated via alpha and the composite reliability index (CRI) (Raykov, Reference Raykov2001, Reference Raykov2004), an index based on the confirmatory results that overcomes some of the shortcomings of alpha. Regarding the IRT framework, accuracy of measurement was estimated with information functions: item and total information curves were calculated. These curves represent the amount of information an indicator or a scale provides across various levels of the latent variable.
Results
Factorial validity
The four CFA models presented in the method section were first estimated in the development sample. Their goodness-of-fit indexes are shown in Table 1 (models 1 to 4 development sample). None of them fit the data reasonably well. Although models 2 and 3 had good CFIs and TLIs, the RMSEA estimates were inadequate. When factor loadings were studied, loading of Item 5 was recurrently low. In model 1 the estimate was .40. Models 2 and 3 posited the indicator in either the physical or the mental dimensions and nevertheless, the loading was still the lowest (.43 and .44, respectively). When Item 5 was cross-loaded on both dimensions in model 4, both loading were low (.29 and .15) and overall fit was not better than the more parsimonious models 2 and 3. Accordingly, two more CFAs were estimated, a one factor (health) solution removing Item 5, and a two-factor (physical and mental health) solution also removing Item 5. As seen in Table 1, only the two-factor solution (model 6 development sample) adequately represented the observed data. Once the different models were tested in the development sample, all the models were estimated and tested, again, in the cross-validation sample. Goodness-of-fit indices for the six models are presented in Table 1, and results are extremely similar to those in the development sample. Again, model 6 better fitted the data than any other model in the cross-validation sample.
Table 1. Set of Confirmatory Factor Analyses (CFA) in Both Samples, the Development and Cross-Validation Samples

Notes: Model 1: one factor of general health; Model 2: two factors (physical and mental health) including Item 5 as an indicator of physical health; Model 3: two factors (physical and mental health) including Item 5 as an indicator of mental health; Model 4: two factors (physical and mental health) including Item 5 as an indicator of both physical and mental health; Model 5: one factor of general health without Item 5; Model 6: two factors (physical and mental health) without Item 5.
Table 2 offers means and standard deviations for all the items in the SF–8, and the factor loadings of the best fitting solution (model 6) in both samples. All factor loadings were statistically significant (p < .01) and very large. The correlation between the physical and mental dimensions of health was .67, 95% CI [.61, .73].
Table 2. Item Content, Means, Standard Deviation and Factor Loadings of the SF–8
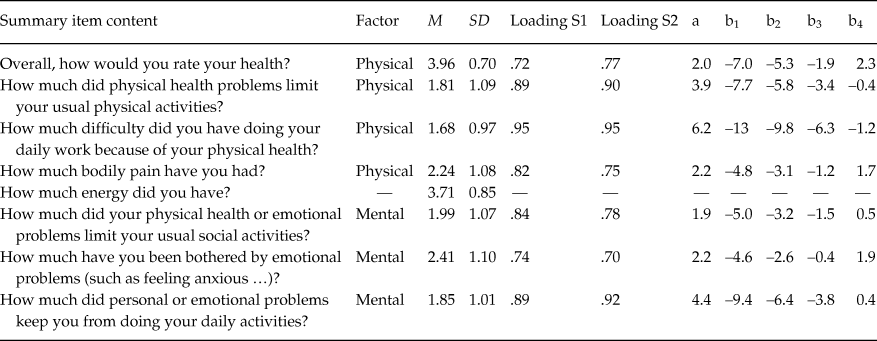
Notes: M = Mean; SD = Standard deviation; S1 = Development sample; S2 = Cross-validations sample.
Item response theory models
IRT models, and specifically the 1 and 2PL model estimated in this particular research, are based on quite strong assumptions. In particular, they assume unidimensionality and local independence. This IRT model permit more sophisticated estimation of item statistics, but it cannot test for these assumptions. The two graded response models, 1PL and 2PL, for the four items measuring physical health (Items 1 to 4) were estimated and their fit compared. On one hand, the fit indices and statistics of 1PL were: Likelihood Ratio Test (LRT) (601) = 332.77, p = 1, AIC = 4492.1, BIC = 4566.5, and ABIC = 4512.6. On the other hand, the 2PL model had these fit indices and statistics: LRT (598) = 257.9, p = 1, AIC = 4430.3, BIC = 4217.8, and ABIC = 4454.3. 2PL model had lower Information Criterion (the lower the better) and a chi-square difference test was also statistically significant (Δχ2= 74.87, Δdf = 3, p < .001), thus supporting the 2PL model against the simpler 1PL. Taking the estimates from the 2PL model, Table 2 shows the a and b parameters for all the items in the physical dimensions. The thresholds for the b parameters showed monotonicity, as expected. However, the low values in the thresholds showed that the items were quite easy. All the items measuring physical health had discrimination values well above 1.0, and accordingly they can be considered highly discriminant.
With respect to the mental health dimension, model fit for the 1PL model was: LRT (111) = 139.42, p = .035, AIC = 4156.9, BIC = 4213.7, and ABIC = 4172.4. The 2PL model for the mental health dimension had the following fit measures: LRT (109) = 118.4, p = .25, AIC = 4139.8, BIC = 4205.3, and ABIC = 4157.7. Again the information criteria favored the 2PL model. Additionally, a chi-square difference test also found that model 2 better fits the data: Δχ2 = 81, Δdf = 2, p < .001. Parameter estimates (a and b) are also shown in Table 2. As was the case with physical health, the thresholds for the b parameters showed monotonicity, as expected, and again the items were quite easy. Item discriminations for mental health were also higher than 1, and accordingly they can be considered highly discriminant. Item Characteristic Curves (ICCs) for both dimensions and all indicators are graphically shown in figure 1.

Figure 1. Item Characteristic Curves (ICCs) for the four indicators of Physical Health (a) and the three indicators of Mental Health (b).
Error of measurement
The amount of measurement error has also been studied via CTT and IRT estimates. The reliability estimates used from a CTT perspective have been the coefficient alpha and the CRI. Alpha and CRI were, respectively, .84 and .91 for Physical Health and .80 and .85 for Mental Health. Overall reliability, as measured by these estimates, was adequate. IRT estimates of reliability are the Item Information Curves and Test Information Function. Contrary to the CTT estimates, these measures do not give an average error of measurement across the scale of the latent variable, but different estimates across values of this scale. Information is shown in Figures 2 and 3, and it points that the SF–8 was more informative in the low levels (below average) of health.

Figure 2. Item Information Curves (IICs) for the four indicators of Physical Health (a) and the three indicators of Mental Health (b).

Figure 3. Item Information Curves (IICs) for the four indicators of Physical Health (a) and the three indicators of Mental Health (b).
Nomological validity
In order to give some evidence about the nomological validity of the perceived health dimensions in samples of older people, we have correlated physical and mental health with social support and life satisfaction (past, present and future). All the correlations are shown in Table 3. In general, as expected, the two dimensions of health had consistent and positive correlations both with social support and life satisfaction. Only the correlation between physical health and social support was non-significant.
Table 3. Correlations among the Two Dimensions of Health (Physical and Mental) and Life Satisfaction (Past, Present and Future) and Social Support

Note: ** = p < .01.
Discussion
The aim of this study was to analyze the psychometric properties of the Spanish version of the SF–8, overcoming previous limitations, such as the exploratory nature of the studies, the lack of factorial validity, or the strong untested assumptions. With this objective in mind, an integrative perspective for the analyses was adopted, including both the traditional analyses derived from the Classical Test theory, and the approximation coming from the Item Response theory.
Results regarding the first approximation took into account different structures for the SF–8. After testing for its adequacy, with evidence pointing out the lack of an appropriate fit, Item 5 (“How much energy did you have?”) was removed. This item had a particular bad behavior, with low factor loadings in every model tested. Problems with this item have been previously documented in the literature. Ware et al. (Reference Ware, Kosinski, Dewey and Gandek2001) were the first to note cross-loading problems for Item 5. Tokuda et al. (Reference Tokuda, Okubo, Ohde, Jacobs, Takahashi, Omata and Fukui2009), in turn, found in the Japanese version of the SF–8 that Item 5 had accuracy problems, with a low information function.
After removing Item 5, two additional models were calculated. This time, results of overall fit were clear and the two-factor solution was retained as the best representation of the data. Thus, this study points that two health factors, physical and mental health, underlay the Spanish version of the SF–8. Current evidence is in line with earlier studies. Both the original authors (Ware et al., Reference Ware, Kosinski, Dewey and Gandek2001) and the study of the Ugandan version (Roberts et al., Reference Roberts, Browne, Ocaka, Oyok and Sondorp2008), using exploratory factor analyses, pointed out a two-factor structure. On the contrary, Tokuda et al. (Reference Tokuda, Okubo, Ohde, Jacobs, Takahashi, Omata and Fukui2009) championed the unidimensional solution. Nevertheless, it should be borne in mind that none of previous studies did test and compare the possible one and two-factor solutions, and thus, this is the first time the two dimensions are defended over the general approach.
Once the dimensionality of the SF–8 was established, IRT models were estimated, with adequate fit for the physical and the mental health factors. In both cases, thresholds for the b parameters showed monotonicity and easiness of the items. This easiness of the items means that items only discriminate (are reliable) for low levels of health. This, in turn, points out that the scale is better suited for populations with poor health in any of the two domains covered, physical and mental.
Error measurement of the Spanish version of the SF–8 was studied. Traditional reliability indices showed appropriate estimates. Additionally, evidence pointed out high discrimination for all the items of the scale. Specifically, Item 3 of the physical health factor (“How much difficulty did you have doing your daily work because of your physical health?”) and Item 8 of the mental health factor (“How much did personal or emotional problems keep you from doing your daily activities?”) were the most discriminant. It is worthy to note that both items share the same characteristic: a specific reference to the influence of health, either physical or mental health, in the daily activities or daily work. It seems clear, then, that, at least in the Spanish elderly population, the one under study, health is primarily related to the development of daily life activities, or normal functional status. This is in line with an important corpus of geriatrics and gerontology literature, which has pointed out a positive, statistically significant relation between physical health and functional status (i.e., Gutiérrez et al., Reference Gutiérrez, Tomás, Galiana, Sancho and Cebrià2013; Hoeymans, Feskens, van den Bos, & Kromhout, Reference Hoeymans, Feskens, van den Bos and Kromhout1997). In fact, and taking into account that functional status has also been closely related to elderly’s life satisfaction and well-being (Deng, Hu, Wu, Dong, & Wu, Reference Deng, Hu, Wu, Dong and Wu2010; Gutiérrez et al., Reference Gutiérrez, Tomás, Galiana, Sancho and Cebrià2013), future studies considering if the traditional relation among elderly’s health and life satisfaction is not direct any more, but a relation mediated by the functional status, would be welcomed.
Finally, nomological validity was also studied. The two dimensions of health had consistent and positive correlations both with social support and life satisfaction, except for the correlation between physical health and social support, which resulted non-significant. This is in line with what Wallston, Alagna, DeVellis, and DeVellis pointed already in 1983, suggesting that evidence supporting a direct link between social support and physical health was more modest than previously claimed.
Taking into account the discussed results, a main, overall conclusion seems to be deduced of current research: The Spanish version of the SF–8 has, in general, adequate psychometric properties in this sample, being better represented by two dimensions of health, physical and mental health. It should be noted that from the SF–8, Item 5 did not function properly, up to the point that it had to be excluded from the analysis. Thus, we may be better off speaking about the SF–7. In addition, the sample under study is composed by people over the age of 60 attending a university life learning program. This may hinder the generalization of results to other populations, such as the general elder population. Further research would be needed, both in the elder and in the general adult population, to shed some light in SF–8 structure. Gathering evidence on patient-reported outcome measures is of crucial importance, as this type of measurement instruments are increasingly used both in academic and clinical arenas (Dawson et al., Reference Dawson, Doll, Fitzpatrick, Jenkinson and Carr2010; Higginson & Carr, Reference Higginson and Carr2001). It is specifically important for the overlooked SF–8, which has been understudied although being part of the most prevalent “SF” family (Turner-Bowker et al., Reference Turner-Bowker, Bartley and Ware2002), and its structure is still under controversy.