Introduction
Compared with conventional farming, organic farming (OF) is partially characterized by the absence of synthetic pesticides and fertilizers, and by practices that enhance ecosystem health (Finckh et al., Reference Finckh, Bruggen and Tamm2015). OF may generate important benefits on farm by improving soil physical properties, increasing biologically available soil organic matter and beneficial soil microbes, reducing disease potential and increasing plant health (Reeve et al., Reference Reeve, Hoagland, Villalba, Carr, Atucha, Cambardella, Davis and Delate2016). OF may provide off-farm benefits on human health, by promoting beneficial phytochemicals in fruits and vegetables (Reeve et al., Reference Reeve, Hoagland, Villalba, Carr, Atucha, Cambardella, Davis and Delate2016; Santos Neto et al., Reference Santos Neto, Schwan-Estrada, Sena, Jardinetti and Alencar2016), and on nearby growers, by increasing the services of beneficial insects (e.g., pollinators and natural enemies of pests). In addition, OF can improve food and nutritional security and contribute to poverty alleviation (Kremen and Miles, Reference Kremen and Miles2012; Leakey, Reference Leakey2014).
There is a growing interest among growers and consumers in organic production (OP) as an alternative to conventional production (CP). The global retail sales of organic food and drink products expanded from US$15.2 to 80 billion, and the worldwide market for organic food and drink products has grown at a much higher annual rate (10–15%) than for conventional products (2–4%) from 1999 to 2014 (Willer and Lernoud, Reference Willer and Lernoud2016).
Despite the fact that Brazil is the largest market for organic products in Latin America with US$930M (US$4 per capita) in retail sales in 2014 (Research Institute of Organic Agriculture-FiBL, 2016; Willer and Lernoud, Reference Willer and Lernoud2016), Brazil ranks only third in Latin America and twelfth in the world in agricultural land organically cultivated. The 11,650 registered organic producers in Brazil in 2015 were mostly small-scale family farmers (Dalcin et al., Reference Dalcin, Souza, Freitas, Padula and Dewes2014) and organically cultivated only 750,000 hectares, which is only 1.04% of the total agricultural land in Brazil (Meirelles, Reference Meirelles, Willer and Lernoud2016). Therefore, there appears to be ample opportunity to expand the domestic supply of organic products. One possible explanation for the current low domestic supply of organic products in Brazil is that OP may be less productive (Goklany et al., Reference Goklany, Mäder, Fließbach, Dubois, Gunst, Fried and Niggli2002; Badgley et al., Reference Badgley, Moghtader, Quintero, Zakem, Chappell, Viles-Vazquez, Samulon and Perfecto2007; Ponti et al., Reference Ponti, Rijk and Ittersum2012) and less profitable (Nemes, Reference Nemes2009) than CP, even though organic products command 20–30% higher prices than conventional products (Dalcin et al., Reference Dalcin, Souza, Freitas, Padula and Dewes2014). The objective of this study was to examine how OP and CP compare in economic (e.g., revenue, costs and profit per box of strawberry) and in productivity terms (i.e., boxes of strawberry per hectare of land), using farm-level observational data collected from randomized surveys of organic and conventional strawberry growers in a key producing area in Brazil, Brazlândia, Federal District (DF).
Two factors would seem to encourage conversion to strawberry OP in Brazlândia. First, strawberry growers there are small-scale growers who rely on considerable labor inputs. About 74% of them have less than 1 ha of strawberries and the largest one has only 6 ha of strawberries. In addition, it is widely known in the area that organic strawberries command a price premium of 44 and 28% higher than conventionally produced strawberries in years 2014 and 2015 (Seagri, 2015, 2016). Thus, it would seem that growers would be interested in exploring the possibility of converting to OP. But conversion to strawberry OP has been slow as only nine out of 188 strawberry growers in the production area of Brazlândia, DF were producing organically in 2014 (EMATER-DF, 2016).
This study is part of a larger study examining factors that could influence conversion, including psychological factors (Andow et al., Reference Andow, Resende Filho, Carneiro, Lorena, Sujii and Alves2017) and social capital (unpublished data). We applied in this study the nearest neighbor matching (NNM) estimator (Abadie and Imbens, Reference Abadie and Imbens2006, Reference Abadie and Imbens2011) to match growers on their propensity scores (PS) obtained from a probit selection model (Rosenbaum and Rubin, Reference Rosenbaum and Rubin1983). As results we estimated the average treatment effect for the treated (ATT) of organically producing strawberry on land productivity, revenue, costs, and profit per box. We also applied the endogenous switching regression (ESR) estimator (Heckman, Reference Heckman1976, Reference Heckman1978) to check the robustness of our results (Maddala, Reference Maddala1983; Dutoit, Reference Dutoit2007) as ESR accounts for potential selection bias caused by unobservable characteristics of growers (Kleemann and Abdulai, Reference Kleemann and Abdulai2013). The remainder of this study is structured as follows: The next section ‘Methods’ gives an overview of strawberry production in Brazil and Brazlândia and the methods used to collect the data, the conceptual framework for the NMM and ESR and the empirical methods employed to implement these analyses. The empirical results are presented and discussed in the section ‘Results and Discussion’. The final section provides concluding remarks and implications.
Methods
Strawberry production in Brazil
Strawberry production in Brazil is important in economic, social and environmental terms. Strawberry is broadly known and accepted by consumers (Antunes and Reisser Junior, Reference Antunes and Reisser Junior2008), and is also a profitable crop in Brazil (Antunes and Peres, Reference Antunes and Peres2013; Fagherazzi et al., Reference Fagherazzi, Grimaldi, Kretzschmar, Molina, Gonçalves, Antunes, Baruzzi and Rufato2017). In 2016, strawberry was cultivated on 4300 ha producing 155,440 t with a gross production value of R$1.2 billion (to facilitate comparison, the average daily exchange rate was 3.22 Brazilian Real (R$) per US$1 from July 27, 2016 to August 25, 2016 according to the Central Bank of Brazil at http://www4.bcb.gov.br/pec/taxas/port/ptaxnpesq.asp?id=txcotacao) (Fagherazzi et al., Reference Fagherazzi, Grimaldi, Kretzschmar, Molina, Gonçalves, Antunes, Baruzzi and Rufato2017).
In social terms, strawberry production is labor intensive (about four persons per ha), which creates jobs, and an important source of income for small-scale family farmers, who currently produce most of the strawberry in Brazil and in our study area, Brazlândia, DF (Lopes et al., Reference Lopes, Silva, Nascimento, Ramos, Pereira and Carneiro2005; EMATER-DF, 2016).
In environmental terms, the conversion to strawberry OP may have important consequences for environmental sustainability and food safety in Brazil. Strawberry CP, which accounts for 99% of the land used for strawberry production (Darolt, Reference Darolt2008), requires frequent applications of pesticides and intensive production practices to control arthropod pests and disease (de Carvalho, Reference de Carvalho2005), which result in negative effects on the environment and on the health of farm workers and consumers. The National Health Surveillance Agency (Anvisa) through its Programme of Pesticide Residue Analysis in Food has consistently found strawberry as one of the foods most contaminated with pesticide residues above the Maximum Residue Limit (MRL) allowed by Brazilian law (Anvisa, 2011; Oshita and Jardim, Reference Oshita and Jardim2012).
Study area and production system
Brazlândia is 475 km2 with 53,800 inhabitants, and has many rural communities, including Assentamento Betinho, Barreiro, Disterro, Bucanhão, Cascalheira, Chapadinha, Alexandre de Gusmão, Morada dos Pássaros, Rádiobras, Rodeador and Torre, where the strawberry farmers in our sample are located.
Brazlândia is the seventh largest producer of strawberries in Brazil and the largest one in the Central West region (EMATER-DF, 2017; Fagherazzi et al., Reference Fagherazzi, Grimaldi, Kretzschmar, Molina, Gonçalves, Antunes, Baruzzi and Rufato2017). The growers of Brazlândia produced 7400 t of strawberry valued at R$62M on 200 ha of land, which is about 5% of the land and production in Brazil in 2016. As it is only 45 km from the capital Brasília, a city with a population of 2.9 million people, its strawberry production fulfills from 70 to 100% of the strawberry consumed in Brasília depending on the month of the year (Lopes et al., Reference Lopes, Silva, Nascimento, Ramos, Pereira and Carneiro2005).
Strawberries in Brazlândia are mostly produced in soil in an open-field, annual hill system with plastic mulch under conventional or organic systems using drip or sprinkler irrigation or both (Lopes et al., Reference Lopes, Silva, Nascimento, Ramos, Pereira and Carneiro2005; Falcão et al., Reference Falcão, Lacerda, Carvalho Mendes, Leão and Carmo2013). There are growers who combine drip irrigation with at least 2 weeks of sprinkler irrigation to ‘wash’ the plants so to reduce mite eggs (Lopes et al., Reference Lopes, Silva, Nascimento, Ramos, Pereira and Carneiro2005; Henz, Reference Henz2010). Almost all production practices, including soil preparation, transplanting, mulching, runner removal, weed and pest control, harvesting, and packing are primarily done by hand without machinery. Therefore, conversion to organic practices seems to be feasible from a labor and technology perspective.
Strawberry growers in Brazlândia plant vegetatively propagated transplants imported either from other states/countries, produced by local nurseries, or produced by local growers (Lopes et al., Reference Lopes, Silva, Nascimento, Ramos, Pereira and Carneiro2005; Antunes and Peres, Reference Antunes and Peres2013). Plants are transplanted from March to April (dry season) on previously prepared beds that are 1–2 m wide, with 2–3 rows per bed at 30 cm plant spacing for a total of 60,000–72,000 plants per hectare. Beds are covered with plastic mulch (30 micron polyethylene canvas) 30–35 days after planting, which requires a four-person crew (Lopes et al., Reference Lopes, Silva, Nascimento, Ramos, Pereira and Carneiro2005). Strawberries are harvested from June through September with peak harvest in July and are almost entirely marketed as fresh fruit in local or regional domestic markets, in 300 g plastic containers packed in 4-container cardboard boxes (Lopes et al., Reference Lopes, Silva, Nascimento, Ramos, Pereira and Carneiro2005; Henz, Reference Henz2010).
Survey design and data
We designed a survey to investigate producer and farm characteristics, quantities and costs of inputs, and revenues associated with OP and CP of strawberry among strawberry farmers in Brazlândia, DF, Brazil. Prior to the final survey design, we met several times with extension personnel from Brazlândia and the central extension office in Brasília to identify and adjust questions on grower and farm characteristics, quantities and costs of inputs, and revenues from organic and conventional strawberry production in Brazlândia. These extension personnel have had many years of experience interacting with strawberry growers in the study area, and know many of them personally, including all of the organic strawberry growers in the area. We tested the draft survey with 12 strawberry growers in Brazlândia, and based on these results modified the questions to remove ambiguities. The survey design related to this study is provided in Table A1 in the Appendix, both in the original Portuguese and translated into English.
With the assistance of the Brazlândia extension office, we identified all 188 strawberry growers in the area. These growers had been classified by area of strawberry production and production system. The production system was an open-field, annual hill system but under conventional or organic production. The organic growers (The organic producers in the sample are all certified according to the Brazilian legislation for organic agriculture in place that consists of the Law number 10,831 for Organic Farming from 23rd December of 2003, and two Normative Instructions from the Ministry of Agriculture (IN17/2014 from June 17, 2014, and IN46/2011 from October 6, 2011) that cover crop production, animal husbandry, food processing and handling and labeling. This legislation states that all farms selling or labeling products as organic should be certified as such. Agriculture that meets OP standards, but is not subject to organic inspection, certification and labeling is referred to as ‘non-certified organic agriculture’ as distinguished from ‘certified organic agriculture.’) were previously identified by the Brazlândia extension office, and were supplemented by two additional farmers who we identified during the interview process. In a sister study (Andow et al., Reference Andow, Resende Filho, Carneiro, Lorena, Sujii and Alves2017), we separated conventional growers into two subgroups, transitional (A grower is considered transitional if he/she has a high diversity of crop production and has used at least two other agroecological practices, such as relying on biological control, using living windbreaks or organic fertilizers (Carneiro, Reference Carneiro2014).) and conventional, but found no difference between these subgroups. As our preliminary analysis also found no differences, the two subgroups were combined in this study.
We classified growers by area as ≤1 ha (small-scale), >1 and ≤2 ha (medium scale) and >2 ha (large-scale). We included all organic and large-scale conventional growers in our sample and randomly sampled 51.5% of medium-scale and 55.5% of small-scale conventional growers. If a producer was no longer in strawberry production, we selected a random replacement, when possible. Only nine growers refused to answer the survey, and they were replaced when possible, for an 85.2% response rate, which makes non-response bias probably absent. The data were collected between May and July, 2015 through face-to-face interviews by one person (DRL). The final sample size was 86 growers (Table 1).
Table 1. Number of growers contacted, sampled and interviewed and response rate.

1 12 growers were involved in the test sample, and thus could not be included in the final data, and 13 growers were either no longer producing strawberry, moved from Brazlândia or a relative who managed the farm had been interviewed.
2 2 organic growers were dropped of the sample because their data showed inconsistencies.
Notes: The data were collected between May and July, 2015 through face-to-face interviews by one person (DRL).
Impact evaluation strategies and techniques
Estimation of the impact of conversion to OP on outcome variables of growers based on nonexperimental observational data is not simple. The counterfactual outcome variables for organic growers in case they had chosen to produce conventionally are unobservable, which creates a missing data problem. In an experimental study, this problem would not exist because growers would be randomly assigned to produce conventionally (control group) or organically (treatment group). However, this was not possible in this study.
In observational studies like this one, wherein the assignment of a producer to produce conventionally or organically is not random, growers decide themselves given the information they have. Thus, organic and conventional growers may be systematically different on the basis of their observable and unobservable characteristics. To correct for these potential selection biases in estimating the impact of conversion to OP on outcome variables of growers, we use the propensity score matching (PSM) estimator as proposed by Rosenbaum and Rubin (Reference Rosenbaum and Rubin1983) and ESR estimator brought into the modern literature by Heckman (Reference Heckman1976, Reference Heckman1978).
The Roy–Rubin model based on potential outcomes (Roy, Reference Roy1951; Rubin, Reference Rubin1974) is the standard approach to this problem of inference in an observational study of individuals. The Roy–Rubin model relies on three main pillars (individuals, treatment and potential outcomes) and on the assumption that each individual has a well defined outcome for each treatment level (Caliendo and Kopeinig, Reference Caliendo and Kopeinig2008). Thus, let potential outcomes y 0i and y 1i be realizations of random variables y 0 and y 1 where the subscript zero denotes CP and subscript 1 denotes OP for individual i = 1, …, n, such that individual treatment effects, τi, is calculated as:

But in reality, for an organic grower i, only y 1i is observed and, for a conventional grower i, only y 0i is observed, as counterfactual outcomes are unobservable. The impossibility of observing both potential outcomes for the same individual at the same time constitutes the fundamental problem of causal inference in observational studies (Holland, Reference Holland1986) that ultimately is a missing data problem.
This study uses propensity score estimation and matching to overcome this missing data problem by matching the nearest organic and conventional growers according to the propensity score (Amare et al., Reference Amare, Asfaw and Shiferaw2012) to impute the missing potential outcome for each individual in the sample (Uematsu and Mishra, Reference Uematsu and Mishra2012).
We estimated the ATT growers as we are interested in the effect of the conversion to organic on outcomes of growers who might convert (Heckman, Reference Heckman1997) as:

where t receives 1 if the grower is treated, otherwise zero.
The first assumption underlying matching estimators is the conditional independence (CI) assumption, which is also known by several other names, including ignorability (Wooldridge, Reference Wooldridge2010), selection on observables (Fitzgerald et al., Reference Fitzgerald, Gottschalk and Moffitt1998) or unconfoundedness (Rosenbaum and Rubin, Reference Rosenbaum and Rubin1983; Imbens, Reference Imbens2004). A problem, however, is that CI is not a directly testable assumption (Uematsu and Mishra, Reference Uematsu and Mishra2012).
The CI assumption says that after accounting for covariates in vector X, which is the set of characteristics used in propensity matching, the choice to convert or not can be taken as independent of the potential outcomes (y 0, y 1), which implies E(y 1|t) = E(y 0|t) = 0 (mean independence of outcomes from treatment). In other words, any remaining difference in the outcome variable y after matching can be solely attributed to organically or conventionally producing (Imbens, Reference Imbens2004), which can then be taken as purely random among matched observations (Becker and Ichino, Reference Becker and Ichino2002). Therefore, under the CI assumption, as E(y 1|X,t) = E(y 1|X) and E(y 0|X,t) = E(y 0|X), ATT may be obtained from matched observations as:

The second assumption underlying matching estimators is the overlap assumption. The overlap assumption means that each grower in the sample must have a positive probability of being assigned to producing organically so that for each possible X in the population, 0 < Pr(t = 1|X) < 1. Rosenbaum and Rubin (Reference Rosenbaum and Rubin1983) call the combination of the CI and overlap assumptions strong ignorability. As there is no specification test for the overlap assumption, we checked for violation of this by looking at the estimated probability densities to see if they have too much mass around 0 or 1 (Busso et al., Reference Busso, DiNardo and McCrary2014), but did not find evidence of violation.
The third assumption underlying matching estimators is that the observations are independent and identically distributed (i.i.d.), by which the outcome and treatment status of each individual are unrelated to the outcome and treatment status of all the other individuals in the sample. This assumption is fulfilled for this study because we used stratified random sampling.
Matching growers based on X might be difficult when the set of covariates is large (Amare et al., Reference Amare, Asfaw and Shiferaw2012) because of the problem of high dimensionality. However, Rosenbaum and Rubin (Reference Rosenbaum and Rubin1983) showed that if it is sufficient to estimate effects by adjusting for a vector of covariates, X, then one can use the estimated probability of treatment conditioned on X, $\widehat{{\rm p}}_i = \widehat{{{\rm pr}}}(t = 1 \vert{\bf X}_i)$ to match growers.
To avoid the curse of dimensionality, we follow Rosenbaum and Rubin (Reference Rosenbaum and Rubin1983) and instead of matching on each of the covariates in X, we match along $\widehat{{\rm p}}_i$, which is a single index variable that summarizes the covariates in X. In so doing, we use the NNM estimator derived by Abadie and Imbens (Reference Abadie and Imbens2006, Reference Abadie and Imbens2011) to match growers based on PS that we call the propensity score matching (PSM) estimator or PSM/NNM estimator. The NNM estimator based on the PSM estimator selects the individuals who are the closest matches to impute the missing potential outcome for each individual in the sample using the PS conditional on the vector of covariates Xi,
$\widehat{{\rm p}}_i$. In other words, the PSM estimator matches samples based on a single continuous covariate,
$\widehat{{\rm p}}_i$, such that the difference between the observed outcome and the imputed potential outcome is an estimate of the individual-level treatment effect. More recently, Abadie and Imbens (Reference Abadie and Imbens2016) derived a method to estimate the standard errors of the NNM estimator based on PS that we used in this study.
First step of the PSM estimator
The first step of the PSM estimator consists in estimating the probabilities that growers choose OP conditional on the vector of observable covariates, $\widehat{{\rm p}}_i({\bf X})$. We used a probit selection model to obtain those estimates as we assume the grower i decision to produce organically or conventionally is driven by the stochastic process (4):

where t i is a binary variable that receives 1 if grower i were to choose to produce organically, which means $t_i^* \gt 0$, and that receives 0 if grower i were to choose to produce conventionally, which means
$t_i^* \le 0$, where
$t_i^* \;{\rm \equiv}\; {\bf X}_i{\rm ^{\prime}\beta \;+ \;} {\rm \epsilon} _i$ is a latent variable (non observable), Xi is the vector of observed covariates for grower i, β is a vector of parameters and εi is the realization of an i.i.d. normal error ε.
The maximum likelihood estimate for β, which is $\widetilde{{\rm \beta}} $ = arg max lnL(
$\widetilde{{\rm \beta}} $), is obtained by finding
$\widetilde{{\rm \beta}} $ that maximizes the log-likelihood function of the probit model:

where Φ(.) is the cumulative normal distribution, i ∈ S denotes a grower i who belongs to set S of growers who have chosen to produce organically, and $i \notin S$ denotes a grower i who does not belong to set S and therefore produces conventionally.
The conditional probability of grower i choosing OP over CP is estimated as:

Following Uematsu and Mishra (Reference Uematsu and Mishra2012), we used the first step of the PSM estimator to analyze how the covariates in vector X determined the conditional covariate probabilities of choosing to produce strawberry organically.
To eliminate the potential observable sample selection bias, it is important to carefully choose the covariates in vector X. They should be observable characteristics of growers that truly condition the probability that a producer would choose OP over CP, so that conditional probabilities can be estimated by Equation (6) according to the probit selection model.
The covariates we used in the probit model were selected based on empirical findings in the literature (Burton et al., Reference Burton, Rigby and Young2003; Uematsu and Mishra, Reference Uematsu and Mishra2012) and their values were directly obtained or calculated based on growers’ answers to related questions in Table A1 in the Appendix. To represent observable individual characteristics, we used the binary covariate male that receives 1 if a grower is male, and 0 otherwise, educ and educ 2 that are the number and squared number of years of formal education a producer declared, age 2 that is the squared age of a producer, p34 and p342 that are years and squared years a producer declared s/he has been farming strawberry.
We also included covariates related to farm characteristics in the probit selection model. The first of them was the binary variable emater that receives 1 if a producer declared s/he has used services provided by EMATER (Empresa de Assistência Técnica e Extensão Rural), which is the primary rural extension institution in Brazil, and 0 otherwise. The other two covariates were related to farm characteristics. We used area, which is the total area in hectares that a producer declared to have used to produce strawberry in year 2014, and the covariate p42, which receives values 1, 2, … ,7 according to the category of debt ratio or total debts divided by total assets in years 2013/14, such that, 0–15% (=1), 15–30% (=2), 30–45% (=3), 45–60% (=4), 60–75% (=5), 75–95% (=6) and 90–100% (=7). Thus, the higher the value of p42, the higher is the debt ratio in years 2013/14.
Second step of the PSM estimator
In the second step, the PSM estimator uses the NNM estimator developed by Abadie and Imbens (Reference Abadie and Imbens2006, Reference Abadie and Imbens2011) to generate the set of nearest neighbor indices, Ω(i), for each observation i = 1, … , n, according to Equation (7):

where m i is the number of elements in the set Ω(i).
We use the teffects psmatch command in Stata (StataCorp, 2015) to execute the entire PSM estimator. Within this command, the number m such that m i ≥ m can be chosen so that the number of matches for each grower i is at least m growers from the opposite treatment group (t = 1 − t i). If m is small then unmatched observations may be discarded in estimating ATT but if m is larger more observations are used, which may compromise the quality of match (Uematsu and Mishra, Reference Uematsu and Mishra2012). Thus, we considered m i = 1,…,5 in our analyses to check for the consistency of the PSM estimates.
Third step of the PSM estimator
In the third step, the PSM predicts potential outcomes $\widehat{y}_{1i}$ and
$\widehat{y}_{0i}$ for each grower i = 1,…,n, according to Equation (8):

where t i is 0 if the grower i had chosen CP and 1 if s/he had chosen OP, Ω(i) is the set of growers j = 1, …, m i from the opposite treatment group of grower i and matched by NNM to grower i.
Finally, ATT is estimated according to Equation (9):

ESR estimator
Despite the fact that PSM corrects for observable bias by comparing the difference between the outcome variables of organic and conventional growers with similar observable characteristics, it does not correct for potential selection bias caused by unobservable characteristics of growers. Moreover, if there is selection bias based on unobservable characteristics, the CI assumption is violated.
We follow Amare et al. (Reference Amare, Asfaw and Shiferaw2012) and complement the PSM/NNE analysis with the ESR estimator brought into the modern literature by Heckman (Reference Heckman1976, Reference Heckman1978) to check the consistency of the results (Maddala, Reference Maddala1983; Dutoit, Reference Dutoit2007). We apply the ESR estimator using the etregress command in Stata (StataCorp, 2015).
The ESR estimator offers less flexibility than the PSM estimator because more structure must be imposed on the model when CI is not assumed. We use the ESR estimator that assumes a linear model for potential outcomes and a probit model for treatment assignment, such that:

where δ0 is the intercept, δ is the parameter whose value is the ATT, εi is the error unrelated to treatment that is used to model potential outcomes, Xi is the vector of covariates that are unrelated to the error term u i that is used to model treatment assignment, and the errors εi and u i are bivariate normal with mean zero and covariance matrix given by:

where σ2 is the variance of εi, the variance of u i is normalized as 1 so that the covariance of εi and u i is ρσ.
We estimated the ESR model by full maximum likelihood based on the likelihood function given by Maddala (Reference Maddala1983, p. 122), and conducted a Wald test of the null hypothesis of H 0: ρ = 0; to test if the correlation between the treatment assignment errors and the outcome errors is zero. We take the non-rejection of the null as evidence in favor of the (CI) assumption.
Results and Discussion
Table 2 provides the definitions of the outcome variables used in this study and the mean values for the entire sample, organic strawberry growers and conventional strawberry growers.
Table 2. Definitions of outcome variables, mean values and mean comparison tests.

1 The differences in means are obtained by subtracting means for organic strawberry growers from those for conventional ones. t-test is used to perform bilateral hypothesis tests of H0: difference in means is zero.
Notes: A box has 1.2 kg of strawberries and all values are in Brazilian Real, R$; productivity is the number of boxes per hectare; tcb is the total production cost per box; revenueb is the total revenue per box; nrb is the net revenue per box that is (revenueb – tcb); lcb is the labor cost per box; trcb is the transplant cost per box; fcb is the fertilizer cost per box; pcb is the pesticide cost per box; occb is the cost of other chemicals per box; icb is the irrigation cost per box.
***, **, and * indicates statistical significance at 1, 5 and 10%.
The average daily exchange rate was 3.22 Brazilian Real (R$) per US$1 from July 27, 2016 to August 25, 2016 according to the Central Bank of Brazil at http://www4.bcb.gov.br/pec/taxas/port/ptaxnpesq.asp?id=txcotacao.
The last column in Table 2 shows t or z statistics that compare means of the organic and conventional growers. By these tests, we observed that the revenue per box (revenueb), the net revenue per box (nrb), the labor cost per box (lcb), and the irrigation cost per box (icb) were significantly higher for organic growers than for conventional growers. For all remaining outcome variables, we found no significant difference between the two groups of growers. However, simply comparing the mean value of outcome variables for the treated (organic growers) and untreated (conventional growers) groups as in Table 2 is a poor solution to the problem of unobservable counterfactual outcomes. This approach badly estimates the effect of treatment (to produce organically) because of potential selection bias on observable and unobservable characteristics of growers.
Probit selection model estimation
Table 3 summarizes the definitions of covariates in vector X and provides their mean values for the entire sample, organic growers and conventional growers.
Table 3. Definitions of covariates in vector X, mean values and mean comparison tests for conventional and organic strawberry growers.

1 The differences in means are obtained by subtracting means for organic strawberry growers from those conventional ones, and t-test is used to compare the differences for continuous variables. The z asymptotic test on the equality of proportions is used to compare the differences for category variables and z-score is reported.
Notes: organic receives 1 if organic, 0 otherwise; male receives 1 if male, 0 otherwise; educ is years of formal education; educ 2 is squared educ; age is the grower age in years; age 2 is squared age; p34 is the number of years the grower has been producing strawberry; p34 2 is squared p34; emater receives 1 if the grower has used the services provided by Emater; 0 otherwise; area it the total area cultivated with strawberry as hectares; p42 denotes the category of debt ratio or the total debts divided by the total assets in years 2014/15 such that: 0–15% receives 1, 15–30% receives 2, 30–45% receives 3, 45–60% receives 4, 60–75% receives 5, 75–95% receives 6 and 90–100% receives 7.
***, **, and * indicates statistical significance at 1, 5 and 10%.
The last column in Table 3 shows t or z statistics that compare means of the organic and conventional strawberry growers for covariates in vector X. These tests show the two groups were different only with respect to the covariates age, age 2, and area, which may indicate that the potential selection bias caused by observable characteristics of growers was low.
The propensity score estimates obtained by the probit selection model had a mean of 0.0817, which is very close to the rate observed in the sample, and a standard deviation of 0.183. Table 4 shows the probit selection model estimates.
Table 4. Estimates of the probit selection model as first step of the Propensity Score Matching (PSM) estimator.

1 P-values for the z-test is calculated based on robust standard errors (Huber/White/sandwich estimator).
2 The numbers reported in the ‘Marginal Effects’ column are average predicted probabilities.
Notes: See footnote Table 3 for definition of covariates.
***, **, and * indicates statistical significance at 1, 5 and 10%.
The probit model shows significance according to the overall Wald test, and a good fit (Table 4). Except for the constant and covariates male and emater (A grower is considered transitional if he/she has a high diversity of crop production and has used at least two other agroecological practices, such as relying on biological control, using living windbreaks or organic fertilizers (Carneiro, Reference Carneiro2014).), all other covariates in the model were statistically significant, and we briefly review the results for these as follows.
More formal education makes growers more likely to produce strawberry organically if they have studied less than 5.6 years (0.801/(2 × 0.072) = 5.6 years), otherwise the effect is the opposite direction. However, as 54.7% of growers in the sample declared that they had more than 5.6 years of formal education, the marginal effect of education on the average probability of producing strawberry organically was not significant.
Uematsu and Mishra (Reference Uematsu and Mishra2012) found in the USA that more educated growers had a higher probability of being certified organic. In their sample, organic growers had about one more year of education than conventional growers and the average level of education of growers was 13.62 years, which is almost twice what was observed for strawberry growers in Brazlândia. As organic and conventional strawberry growers in Brazlândia have the same low average level (6.3 years) of formal education and we found no significant marginal effect of formal education on the probability of producing strawberry organically, perhaps an effect of education requires a higher average education level than that observed in Brazlândia.
Older growers were more likely to produce strawberries organically. But the marginal effect of age indicated that the average probability of producing organic strawberries increased by only 0.01% for each additional year of life, which is not economically meaningful.
More experience on the production of strawberries makes growers less likely to produce strawberry organically if they have been farming strawberry for less than 13.5 years (0.352/(2 × 0.013) = 13.5 years). Otherwise, an inverse relationship is expressed. However, as 31.4% of growers have been farming strawberry for longer than 13.5 years, the marginal effect of experience on the average probability of producing strawberry organically was not significant.
The greater the area allocated to strawberry production, the less likely was organic strawberry production. For each additional hectare allocated to produce strawberry, the average probability of producing organically decreased by 39.7%.
Finally, the higher was the debt ratio of a producer, the more likely was organic strawberry production. In fact, for each step to a higher category of debt ratio, the average probability of producing strawberries organically increased by 6.6%.
PSM/NNM estimates of the ATT
Table 5 shows the ATT of producing strawberries organically on productivity (productivity), revenue per box (revenueb), net revenue per box (nrb) and total production cost per box (The total production cost of strawberry was calculated as the sum of the labor cost, transplant cost, fertilizer cost, pesticide cost, other chemicals cost, irrigation cost and postharvest cost according to answers to associated questions in the survey as shown in Table A1 in Appendix.) (tcb). We also examined the main components of total production cost per box, which accounted for 70.2% of the tcb of growers in the sample: labor cost per box (lcb) as R$ per box (31.2% of tcb), transplant cost per box (trcb) as R$ per box (17.8% of tcb), fertilizer cost per box (fcb) as R$ per box (17.8% of tcb), pesticide cost per box(pcb) as R$ per box (10.4% of tcb) and irrigation cost per box(icb) as R$ per box (7.9% of tcb).
Table 5. Propensity score matching/nearest neighbor matching (PSM/NNM) estimates of the average treatment effect for the treated (ATT).
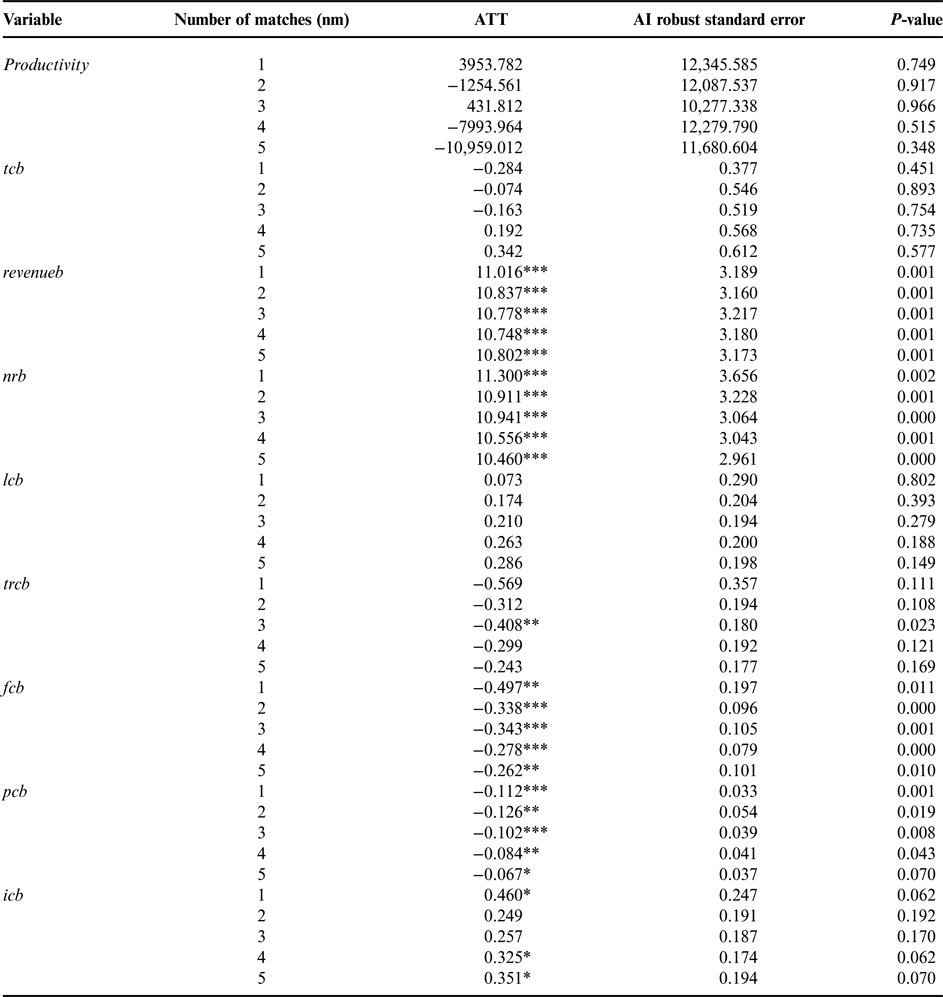
Notes: A box has 1.2 kg of strawberries and all values are in Brazilian Real (R$); productivity is the number of boxes per hectare; tcb is the total production cost per box; revenueb is the total revenue per box; nrb is the net revenue per box that is (revenueb – tcb); lcb is the labor cost per box; trcb is the transplant cost per box; fcb is the fertilizer cost per box; pcb is the pesticide cost per box; occb is the cost of other chemicals per box; icb is the irrigation cost per box.
***, **, and * indicates statistical significance at 1, 5 and 10%. A box contains 1.2 kg of strawberries.
The average daily exchange rate was 3.22 Brazilian Real (R$) per US$1 from July 27, 2016 to August 25, 2016 according to the Central Bank of Brazil at http://www4.bcb.gov.br/pec/taxas/port/ptaxnpesq.asp?id=txcotacao.
The ATT estimates for the main components of total production cost per box in Table 5 show that the fertilizer cost per box and pesticide cost per box were less for OP for all m i, but the irrigation cost per box (icb) was more for OP for m i = 1, 4 and 5. Thus, as organic growers do not use pesticide, they may substitute irrigation to ‘wash’ the plants so to reduce mite eggs or to improve plant health to resist pests.
The ATT estimates for productivity and tcb changed signs for m i = 1, 2, …, 5, but in no case could we reject the hypothesis that ATT was zero (Table 5). Despite the cost component differences, the total production cost per box did not differ between organic and conventional growers. The higher irrigation cost and other costs in OP, while not significantly different, may have partially offset the cost reductions associated with fertilizer and pesticides. In other words, producing strawberries organically had similar total production cost per box and productivity as CP. These findings are in line with the findings of Oelofse et al. (Reference Oelofse, Høgh-Jensen, Abreu, Almeida, Hui, Sultan and Neergaard2010), but contrary to the hypothesis that yields are less under adoption of organic practices (Goklany et al., Reference Goklany, Mäder, Fließbach, Dubois, Gunst, Fried and Niggli2002; Badgley et al., Reference Badgley, Moghtader, Quintero, Zakem, Chappell, Viles-Vazquez, Samulon and Perfecto2007).
In contrast, the ATT estimates for revenueb and nrb were positive for everym i = 1, 2, …, 5, and we rejected the null hypothesis that ATT was zero. Thus, organic strawberry production had higher revenue per box and higher net revenue per box than CP. The higher revenue per box was due to the significant price premium for organic strawberries. As the nrb estimates were very close to the revenueb estimates and there was no difference in tcb, we conclude that the price premium for organic strawberries was the main reason for the higher profit per box for organic strawberry production.
Despite the higher profit per box for organic strawberry production, we observed only 4.8% of farmers had converted to OP. Thus, there may be other economic and non-economic factors that can drive or inhibit conversion that could be missed in comparative profitability studies such as ours (Burton et al., Reference Burton, Rigby and Young2003).
A grower in our sample, who has produced strawberry for more than 20 years, started to convert to the OP in 2001, and supplies organic strawberries to big supermarket chains, such as Pão de Açúcar, Extra and Big Box, reported that ‘it took me about five years to fully convert to the OP of strawberries. The change was difficult, as I had to give up 70% of my income to convert, fully restoring my income only five years later. Since then, however, the great demand and the excellence of the fruit I offer made my business steadily grow’. Indeed, as it takes time for the land to readjust to an organic system as well as for the farmer to adjust to the organic practices, it is likely that there are adjustment costs in the process of conversion to OP (Chase, Reference Chase2009). These costs were likely not incorporated in the cost estimates they reported, as they have produced strawberry organically for more than 11 years in average.
In addition, our estimates of production did not characterize aspects of strawberry traits, which are sometimes considered important for OP. Larger berry size, enhanced strawberry flavor and reduced contamination by pesticides may be important characteristics that affect this price premium (Lin et al., Reference Lin, Smith and Huang2008). Clarifying the reasons for the price premium is an important area of future investigation as these would affect equilibrium (price and quantity) in the market of organic strawberries under widespread adoption of OP (Nemes, Reference Nemes2009).
Regarding non-economic factors that can drive or inhibit conversion, Oelofse et al. (Reference Oelofse, Høgh-Jensen, Abreu, Almeida, Hui, Sultan and Neergaard2010), based on two cases from China and one from Brazil, found that conversion was largely facilitated when farmers belonged to a cooperative organization and had external technical advice about production, certification and marketing. Andow et al. (Reference Andow, Resende Filho, Carneiro, Lorena, Sujii and Alves2017) examined psychological barriers to conversion to strawberry OP for the same sample of growers as studied here, and found that for interested growers, conversion might be enhanced by addressing the social/financial status that conversion could provide, and their perceived lack of control over informational and physical resources needed for conversion. For disinterested growers, subjective social norms and lack of perceived behavioral control were the major barriers.
ESR estimates of the ATT
Table 6 shows the ESR estimates.
Table 6. Endogenous switching regression (ESR) estimates of the average treatment effect for the treated (ATT).

1 All covariates (male educ educ2 age2 p34 p342 emater area p42) in the PSM estimation were also used in the ESR estimation.
2 Only covariates educ2 p34 p342 p42 emater area were used in the ESR estimation.
3 Only covariates educ educ2 age2 p34 p342 area p42 were used in the ESR estimation.
4 Only covariates male educ educ2 age2 p34 p342 emater were used in the ESR estimation.
Notes: See footnote Table 5 for definition of variables.
***, **, and * indicates statistical significance at 1, 5 and 10%.
The ESR estimates in Table 6 led to the same conclusions from the PSM/NNM estimates, except that none of the major cost components were significant. Specifically, the ATTs for fertilizer cost per box (fcb), pesticide cost per box (pcb) and irrigation cost per box (icb) estimated by the ESR were not significant.
As the null hypothesis that there was no correlation between the treatment assignment errors and outcomes errors was not rejected by the Wald test (Table 6), the CI assumption was not violated. In this case, the reduced model structure afforded by the PSM/NNE estimates may have enabled finer discernment of the differences between the major cost components of organic and conventional strawberry production.
Conclusion
We found that the gender of a grower and if the grower used technical assistance from EMATER had no effect on the probability the grower chooses to produce strawberry organically. In addition, growers were more likely to produce strawberry organically if they were older, used smaller areas for the production of strawberry and were more indebted. If a grower had less than 5.6 years of education and more than 13.5 years of experience in strawberry production, more education and experience made him/her more likely to produce strawberry organically. The opposite was true for growers with more than 5.6 years of education and less than 13.5 years of experience. Thus, we expect that the growers’ probabilities of conversion for strawberry OP will remain about the same over time in the study area. One way around this problem would be for EMATER to assume a positive role in the conversion decisions and processes of growers. This could be accomplished if EMATER develops a more unified and concerted effort to build the technical and methodological capacity to support conversion to OP. This should be treated as a public policy that could reduce or eliminate the role of positive externalities in strawberry production that would occur in the study area if market were left to its own devices.
We found that producing strawberry organically had no effect on productivity and total production cost per box, but had a positive effect on profit per box due to the price premium for organic strawberry. Although there is no disincentive and a significant profit-based incentive to convert to the OP, this has been insufficient as we observed only 4.8% of farmers had converted to OP. There may be other economic factors such as adjustment costs to conversion, and non-economic factors such as psychological factors (Andow et al., Reference Andow, Resende Filho, Carneiro, Lorena, Sujii and Alves2017) and social capital that may be barriers to conversion. Those factors should be investigated in future studies.
Acknowledgements
We thank the Editor and two anonymous Reviewers for their assistance and very helpful comments. We thank Débora Pires Paula, whose help in revising the survey, translating it into Portuguese, and co-coordinating the survey process was essential to the completion of this work. We thank Ayslan Barbosa Moreno for helping us to critically evaluate the survey responses on economic aspect of the strawberry production. We thank the University of Minnesota for a Global Spotlight Faculty Seed Grant, which funded this work. The first and fifth authors thank CNPq-Brazil for their research fellowships. All remaining errors are the authors’.
Appendix
Table A1. Questions included in the survey.
