1. Introduction
Corpus tools, either web-based (e.g. Collins COBUILD Corpus, WebCorp, WebCollocate, Mark Davies’ Brigham Young Corpora, Corpus of Contemporary American English (COCA)) or stand-alone (e.g. WordSmith Tools, AntConc), have been explored by many researchers and teachers to examine their educational efficacy in language learning. Corpus exploration tools are particularly useful for learners to examine lexico-grammatical patterns from authentic texts; for example, finding correct word combinations (Chen, Reference Chen2011; Daskalovska, Reference Daskalovska2015; Vyatkina, Reference Vyatkina2016; Yoon, Reference Yoon2008), understanding the subtle meaning of certain verbs that lack direct first-language equivalents (Chan & Liou, Reference Chan and Liou2005), and identifying common word-choice errors in writing (Chambers & O’Sullivan, Reference Chambers and O’Sullivan2004; Wu, Franken & Witten, Reference Wu, Franken and Witten2009). Johns (Reference Johns1991) described this approach as “data-driven learning” (DDL). To understand and evaluate the effectiveness of DDL in collocation learning, many researchers design learning activities focused on preselected words or collocation patterns such as verb + noun, adjective + noun, and verb + preposition (Chan & Liou, Reference Chan and Liou2005; Chen, Reference Chen2011; Daskalovska, Reference Daskalovska2015; Vyatkina, Reference Vyatkina2016; Wu et al., Reference Wu, Franken and Witten2009; Yoon, Reference Yoon2008). The activities, normally taking place in computer labs during class time, come in a variety of forms, from multiple-choice, gap-fills and sentence construction, to the correction of word-choice errors marked by instructors. Students’ learning outcomes from test scores or written learner texts, their retrospective perceptions, and user experiences gathered from surveys and interviews constitute the empirical data that researchers have examined and analyzed to report their findings (Boulton & Cobb, Reference Boulton and Cobb2017; Chambers, Reference Chambers, Hidalgo, Quereda and Santana2007; Charles, Reference Charles2014). With the advancement of DDL research and practice, many researchers have recognized the need to investigate how corpus tools are actually used by learners in the long term to enhance our understanding of the efficacy of corpus use in language learning and teaching (Chambers, Reference Chambers, Hidalgo, Quereda and Santana2007; Charles, Reference Charles2014; Crosthwaite, Wong & Cheung, Reference Crosthwaite, Wong and Cheung2019; Hafner & Candlin, Reference Hafner and Candlin2007; Horst, Cobb & Nicolae, Reference Horst, Cobb and Nicolae2005; Johns, Reference Johns, Wichmann, Fligelstone, McEnery and Knowles1997; Kennedy & Miceli, Reference Kennedy and Miceli2017; Pérez-Paredes, Sánchez-Tornel, Alcaraz Calero & Jiménez, Reference Pérez-Paredes, Sánchez-Tornel, Alcaraz Calero and Jiménez2011).
Corpus queries that are recorded by students manually or automatically in computer logs have been used by some researchers to track learners’ actual interactions with corpus tools. Analyzing corpus queries has allowed researchers to verify students’ participation in related activities, the corpus functions they preferred, and the different purposes and approaches to corpus use (Cobb, Reference Cobb1997; Gaskell & Cobb, Reference Gaskell and Cobb2004; Hafner & Candlin, Reference Hafner and Candlin2007). However, although many researchers have called for use of tracking data to gain in-depth insights into what students actually do during DDL, research that capitalizes on user query analysis is still limited.
This paper presents a user query data study that examines language learners’ interactions with a corpus-based consultation tool to characterize their look-up patterns. Our data are large scale in terms of the number of queries and the number of users who come from diverse demographic and geographic backgrounds. The paper also presents multidimensional analyses of learner query data, which, to the best of our knowledge, have not been explored in the DDL literature.
2. Use of corpus queries in previous DDL research
User queries that contain learners’ actual interactions with a corpus have been a popular data source for providing researchers with insights into learners’ corpus use. There are two types of query logs: those manually recorded by learners and those automatically generated by computers. With manual query logs, learners keep a detailed record of their corpus consultation activities, such as the purpose of a look-up (e.g. finding a second-language (L2) equivalent, confirming a hunch, finding a suitable collocate, choosing the best alternative), the items (e.g. words/phrases) they used to formulate a query, whether the results were helpful or not, the exact tools and resources they have used within a corpus-based system, and the problems they have encountered in utilizing such systems (Frankenberg-Garcia, Reference Frankenberg-Garcia2005; Yoon, Reference Yoon2008). This information helps researchers to establish learners’ general look-up goals and habits to identify the usefulness of language resources and the difficulties encountered when using them (Frankenberg-Garcia, Reference Frankenberg-Garcia2005). Despite their obvious benefits, manual logs require students to remember their search queries while depending on learners’ willingness to record their every move and perceived learning needs. As a result, research that employs self-reported logs are typically on a small scale and limited by the number of participants (less than 20 students), the time duration (within 10–15 weeks), and the amount of log data that can be collected (less than several hundred loop-ups) (Frankenberg-Garcia, Reference Frankenberg-Garcia2005; Yoon, Reference Yoon2008).
Few DDL researchers have incorporated computer-generated logs as complementary data to support their findings. Typical log data include user account name or ID given by the teachers or researchers, date and time accessed (e.g. timestamp), search query (e.g. words or phrases that users type in or click), and so on. The size of log data depends on the number of learners who have used the system, how often they have used it, and the time duration recorded. In earlier studies, computer logs provided reliable verification of students’ engagement with corpora (Chan & Liou, Reference Chan and Liou2005; Cobb, Reference Cobb1997; Gaskell, & Cobb, Reference Gaskell and Cobb2004; Hafner & Candlin, Reference Hafner and Candlin2007), or indicated changes in patterns of corpus use with particular language activities (Cobb, Reference Cobb1997). Data such as user account name, timestamp, and search frequency are easily and accurately captured by computers, allowing researchers to establish a correlation between corpus use and learning outcome. For example, Cobb (Reference Cobb1997) investigated whether the time students spent on concordance lines correlated to the test score gain they achieved. In a similar study based on collocation loop-up frequency recorded in logs, Chan and Liou (Reference Chan and Liou2005) concluded that the scores of items taught via concordancing were significantly higher than those that were not taught using a concordancer. With the help of log data, Hafner and Candlin (Reference Hafner and Candlin2007) observed how their students’ corpus use changed over the course of one and a half years by way of modeling full documents in writing composition. Park and Kinginger (Reference Park and Kinginger2010) made a novel use of corpus search queries by linking them to screen recordings, along with learners’ oral and written reflections on their writing to support the authors’ interpretation of the L2 writer’s composition process. Follow-on research by Park (Reference Park2012) included more student log data that plotted students’ queries and subsequent language use in their essays to determine whether corpus querying would result in having a positive, a negative, or no effect on essay quality.
DDL studies that have used computer-generated logs as the main data source for investigation are relatively limited in number. We have identified only two in the literature. Pérez-Paredes et al. (Reference Pérez-Paredes, Sánchez-Tornel, Alcaraz Calero and Jiménez2011) examined learners’ (37) interactions with a web-based version of the British Nation Corpus (BNC) while doing six form-based activities with and without guided consultation. They looked at the number (171) of BNC searches the students made and the words, wildcard, and part-of-speech (POS) tags used in each search, resulting in the recommendation that skills and guidance are necessary when teachers employ corpora in the classroom. Crosthwaite et al.’s (Reference Crosthwaite, Wong and Cheung2019) study on characterizing students’ corpus query and usage patterns was carried out on a larger scale in terms of the number of participants (327) and the volume of log data collected (11,000 individual corpus queries). Computer log data helps reveal, in great detail, how students have made use of corpus tool facilities such as query functions and query filters. It also helps in identifying the problems that students encounter in formulating queries by way of analyzing errors in the search syntax (e.g. the misuse of wildcards or POS tags).
In research that involves the analyses of logs, search queries are used as direct evidence for identifying what students were trying to find out. Studies that analyze query data of DDL systems recorded as computer logs differ from those studies that analyze manually recorded data where students are asked to keep written logs of their use of DDL systems and to specify the purpose of their queries alongside the words they searched for (Frankenberg-Garcia, Reference Frankenberg-Garcia2005; Yoon, Reference Yoon2008). To interpret query words recorded in computer logs, however, researchers commonly employ query word categorization schemes to generalize the purpose of corpus queries. For example, Hafner and Candlin (Reference Hafner and Candlin2007) have categorized query words into topic or language-related words (e.g. defence and counterclaim) to indicate students’ use of a corpus. Utilization of a corpus may, for instance, be at the full-text document level for looking at domain-specific knowledge or at the sentence or phrase level for looking at specific patterns of language. Park and Kinginger (Reference Park and Kinginger2010: 32) linked query words to the cognitive process of learning as “each query expresses an immediate need.” They identified three broad categories of learner needs after conducting an analysis of their query words – syntactic, lexical, and morphological.
Our literature review demonstrates that despite the great potential that computer logs offer DDL researchers for understanding learners’ actual interaction with corpus tools, there is still a noticeable deficit in research that conducts in-depth and large-scale analyses on computer-generated logs that can assist with characterizing learner behaviors and usage patterns.
3. Research questions
We used a free open-source collocation consultation tool called FlaxCLS to collect user query data. The computer logs contain about 1.5 million collocation look-up queries sent to a system from more than 140 countries over a period of two years (from November 2017 to November 2019). The study attempts to identify collocation look-up patterns with user query data and address the following three research questions:
-
1. How do learners interact with the FlaxCLS while looking up collocations?
-
2. What are the lexico-grammatical characteristics of learners’ query terms?
-
3. What are the characteristics of learners’ preferred lexico-grammatical collocations?
4. The collocation consultation tool: FlaxCLS
FlaxCLS houses collocations that contain language sequences from two to five continuous words using 14 different collocation patterns (see Table 10 for some patterns and Wu, Li, Witten & Yu, Reference Wu, Li, Witten and Yu2016, for a detailed description). The FlaxCLS design team has extended some of these collocation types to include more constituent parts that were deemed to be beneficial for learners. For example, the noun part of a verb + noun collocation can contain a complex noun phrase including one or more nouns coupled with modifiers or prepositions: examples are take complete control of, battle for control of. To look up collocations, the user simply types in the word(s) of interest, as shown in Figure 1 where the word control has been entered in the search box and collocations associated with control (either used as a noun or a verb) are returned.
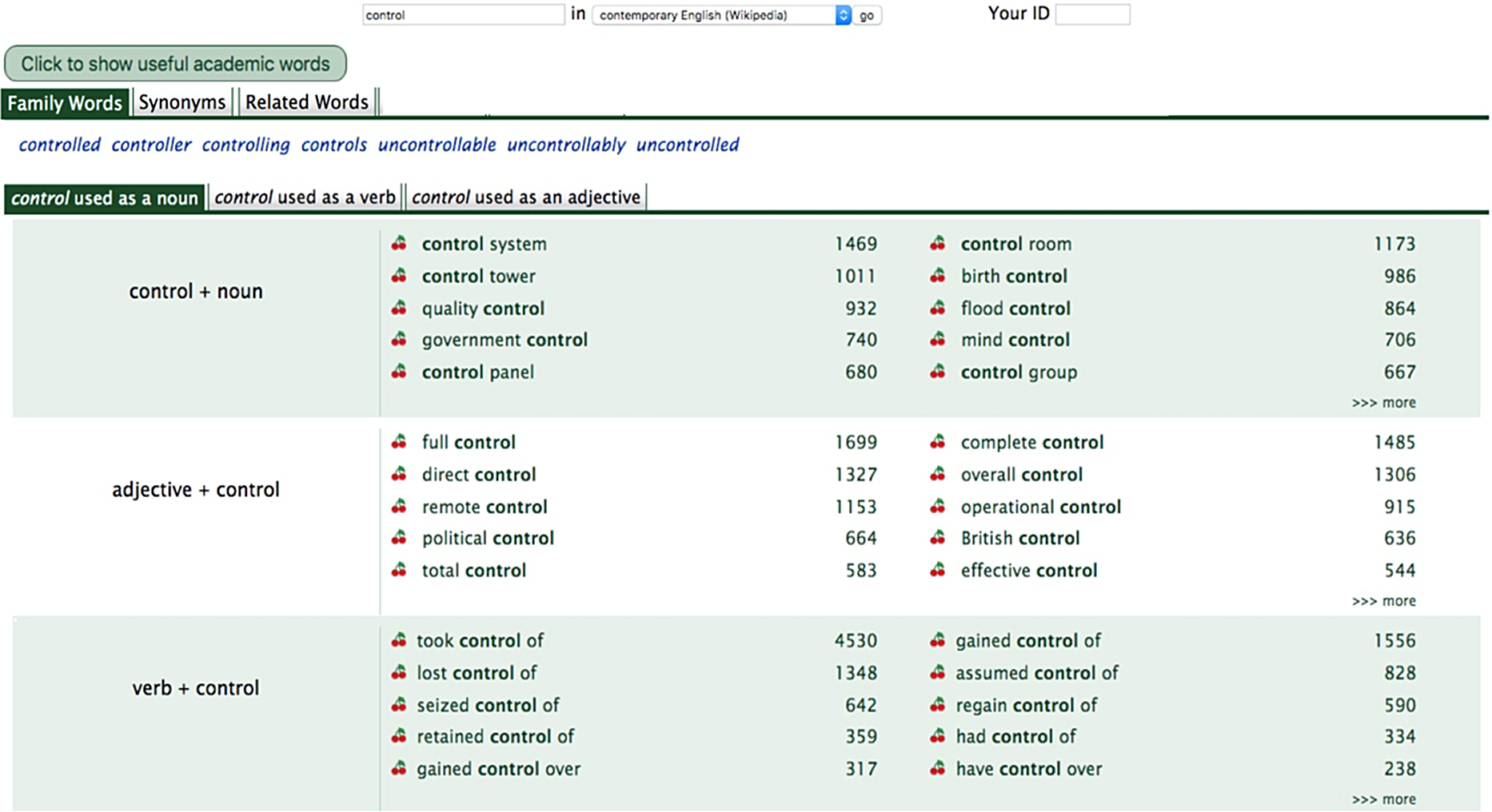
Figure 1. Family Words, Synonyms, Related Words, and collocations associated with the word control
Note. The word control does not have antonyms in our database.
If the user types in more than one word (i.e. a multi-word query), FlaxCLS retrieves collocations containing all the constituent parts, irrespective of word order and intervening words. For example, searching for complete control yields the expansions shown in Figure 2.

Figure 2. Collocations containing both words: complete and control
FlaxCLS provides four query formation aids, similar to search suggestions with relevant feedback: Family Words, Synonyms, Antonyms, and Related Words (see Wu, Fitzgerald, Yu & Witten, Reference Wu, Fitzgerald, Yu and Witten2019, for a detailed description). In Figure 1, the family words (controlled, controller, controlling, controls, uncontrollable, uncontrollably, uncontrolled) of the word control are given as a query aid and the end user can click one of these to retrieve its collocations.
Collocations shown in Figure 1 are hyperlinked whereby the user can click to retrieve either extended collocations or sample sentences. Figure 3 shows four interactions users can make with FlaxCLS.

Figure 3. User interactions with FlaxCLS
-
Interaction 1 (retrieving collocations). The user types a query term (e.g. control) or selects a word from among the search suggestions (i.e. family words, synonyms, antonyms, or related words) to retrieve collocations. The FlaxCLS returns collocations and displays them, as shown in Picture 1, Figure 3.
-
Interaction 2 (viewing extended collocations). The user clicks on a hyperlinked collocation (e.g. gain control over) to view extended collocations, as shown in Picture 2, Figure 3.
-
Interaction 3 (viewing more collocations). The user clicks the “more” button to retrieve more collocations (e.g. won control of), as shown in Picture 3, Figure 3.
-
Interaction 4 (viewing sample sentences). The user clicks on a hyperlinked collocation (e.g. gain control over) to view example sentences of collocations in real-world contexts of communication (e.g. The Republic of Venice had gained control over much of the trade …), as shown in Picture 4, Figure 3.
At any point in the interactions, the user can opt to leave the system and not proceed any further. To illustrate, the user could enter a search term, view the resulting collocations retrieved by the FlaxCLS server, and then depart the system.
User interactions shown in Figure 3 are recorded as query entries in computer log files at the backend of the FlaxCLS server. The next section will identify the kinds of data query entries collected and how they have been processed and analyzed.
5. Data processing and analysis
Since FlaxCLS’s launch in 2015, millions of user interactions (e.g. the word(s) a unique user has entered, the query formation aids a unique user has chosen, and the hyperlinks a unique user has clicked) have been recorded in computer logs. Query data collected for this study spans a period of two years (from November 2017 to November 2019). A computer program was written to process the log files and extract query entries that can then be analyzed using three methods, which will be discussed in section 5.2. First, we will look at the format and content of query entries.
5.1 User query entries and interactions
The following are three query entry examples (E1, E2, and E3):
-
E1. [138.37.177.233] [2018-10-23 06:11:10]
[s=CollocationQuery&query=control&dbName=Wikipedia&from=wf]
-
E2. [138.37.177.233] [2018-10-23 06:11:16]
[s=ExtendedCollocations&collocation=gained control over&collocationType=vn&dbName=Wikipedia]
-
E3. [138.37.177.233] [2018-10-23 06:11:19]
[s=SampleTexts&collocation=gained control over&collocationType=vn &dbName=Wikipedia]
Entries are divided by square brackets into three parts (e.g. E1). An IP address (138.37.177.233) makes up the first part from which the query was sent. The IP address is mapped to a geographic region. In this case, the IP address (138.37.177.233) is located as being in the United Kingdom. User queries can then be grouped into different geographic regions using this information. A timestamp (2018-10-23 06:11:10) makes up the second part that indicates when a query arrived at the FlaxCLS. Query parameters (s=CollocationQuery … s1.from=wf) provide further details for decoding a user interaction. The three recorded entries above detail the resulting sequence of interactions from a learner based in London, England:
-
In E1, the user clicked the word control (query=control) on the “family words” panel (from=wf) and chose the Wikipedia corpus (dbName=Wikipedia).
-
In E2, after 6 seconds, the user selected the extended collocation gained control over with the collocation type verb + noun (collocationType=vn).
-
In E3, after 3 seconds, the user selected a sample sentence showing gained control over in context with the collocation type verb + noun (collocationType=vn).
Analyzing user interactions encoded in query entries allows us to examine user query behaviors and to identify look-up patterns that would be of interest and use to language teachers and DDL researchers. The next section will introduce the data analysis methods used in the study.
5.2 Multidimensional analyses of learner query data
Query data can be analyzed in many different ways for different purposes. We developed three methods based on the information that can be gleaned from learner query data and the pedagogical value that such analyses could potentially contribute to collocation teaching research and practice. They are:
-
1. look-up interaction analysis, to investigate how learners formulate query terms and how they follow hyperlinks in seeking collocations for their needs,
-
2. query term analysis, to study the characteristics of the words or phrases that learners entered to look up collocations,
-
3. collocation analysis, to examine the characteristics of learners’ preferred collocations.
These three analyses correspond to the research questions proposed in the introduction section of this paper. This section explains each method of analysis, and the next section will present the results of the analyses.
5.2.1 Look-up interaction analysis
An interaction, recorded as a query entry (e.g. E1, E2, or E3), is an action that the learner takes when looking up collocations in the FlaxCLS. Interactions include entering a query word or clicking a collocation hyperlink on the web page, as shown in Figure 3. The look-up interaction analysis looks at two aspects: how learners formulate a query term and what types of hyperlinks they have clicked.
As shown in Figure 3, the first task the learner is faced with when using FlaxCLS is to enter a query term. Some learners already know which word/words they are looking for, whereas others require assistance. FlaxCLS users formulate a query term in the following ways: typing in a single word (with the help of Word Autocomplete); typing in two or more words; and clicking one of the query formation aids (Family Words, Synonyms, Antonyms, Related Words). Examining how learners formulate query terms not only helps us assess the usefulness of query functions, such as the multi-word query facility and the query formation aids (Figure 1), but also provides invaluable insights for making further improvements to the design of the system.
Learners enter a query term to retrieve collocations (Interaction 1 in Figure 3) and click a hyperlink to view extended collocations (Interaction 2 in Figure 3), to view more collocations (Interaction 3 in Figure 3), or to view sentence samples (Interaction 4 in Figure 3). Analysis of click frequency and types of clicks provides a quantitative measurement of user engagement with FlaxCLS, as certain types of clicks (i.e. viewing extended collocations and viewing sentence samples) indicate learners’ particular attention to certain collocations in FlaxCLS.
5.2.2 Query term analysis
We define a query term as a string of characters (that make up a single word or multiple words) formulated by the learner to retrieve collocations; that is, the word(s) the learner enters or selects from one of the four query formation aids (e.g. Family Words, Synonyms, Antonyms, Related Words). The analysis helps us better understand the characteristics of words whose associated collocations are of great interest to learners; in particular, we seek answers to the following research sub-questions:
-
Q1. Are learners interested in collocations of common or less common words?
-
Q2. What type of words do learners frequently enter to look up collocations?
-
Q3. Are learners interested in the collocations of academic words?
Query term categorization is a common approach in analyzing web user behavior for using search engines or for visiting a website. In traditional web query analysis, query terms are typically classified by topics pertaining to those such as sexual, social, educational, sports, news, and so on (Jansen, Spink & Saracevic, Reference Jansen, Spink and Saracevic2000; Li, Zheng & Dai, Reference Li, Zheng and Dai2005; Ross & Wolfram, Reference Ross and Wolfram2000). However, this approach is not useful for a DDL study because our users seek language patterns, not websites, for information. Instead, we categorize query terms using four schemes:
-
1. Length: whether a query term is a single-word or a multi-word query.
-
2. Frequency band: whether a query word is in the top 1,000, 2,000, or 5,000 most frequent word list.
-
3. Word type: whether a query word is a noun, verb, or adjective, etc.
-
4. Academic-ness: whether a query word is an academic word.
Categorization involves two steps. In Step 1, the number of words in a query term is counted to extract queries containing only one word (single-word queries) and queries containing more than one word (multi-word queries). Step 2 divides single-word queries into different groups, described as follows.
First, single-word queries are assigned to four frequency bands (the top 1,000, 2,000, 5,000, and 5,000 words and above) according to a freely available top 5,000 words/lemma word list generated from the 450-million-word COCA. Footnote 1 Figure 4 shows a snippet of the top 30 words/lemmas, along with their ranks, POS tags, and rate of frequency according to the list. In Figure 4, the word the is the most frequent word (number 1 in rank), its POS is a (article), and it occurs 22,038,615 times in the corpus. Second, single-word queries are grouped by word type (e.g. noun, verb, adjective, etc.) based on the POS tags (a, v, j, r, etc., in Figure 4) associated with each word. Third, Coxhead’s (Reference Coxhead2000) Academic Word List is used to group single-word queries into academic and non-academic words.

Figure 4. The top 30 words/lemmas in the COCA
Note. a = article; v = verb; j = adjective; p = pronoun; d = determiner; i = preposition; t = to; c = conjunction; x = auxiliary word.
5.2.3 Collocation analysis
As shown in Figure 1, FlaxCLS presents collocations by syntactic pattern such as verb + noun, adjective + noun, and noun + noun, where collocations are made up of two to five continuous words in sequence. Collocation analysis examines the collocation hyperlinks the learner has clicked while navigating FlaxCLS. A hyperlink click is recorded as a query entry when the learner:
-
1. selects a hyperlinked collocation, gained control over, to view extended collocations (Interaction 2 in Figure 3), or
-
2. clicks on the “more” button to retrieve more collocations, won control of (Interaction 3 in Figure 3), or
-
3. selects a hyperlinked collocation, gained control over, to view sample sentences (Interaction 4 in Figure 3).
This analysis attempts to identify the characteristics of collocations that learners are most interested in and to answer the following three research sub-questions:
Q1. What are the most frequently queried collocation patterns: verb + noun, adjective + noun, or something else?
Q2. Do learners prefer collocations that have more constituent parts?
Q3. What kind of collocations do learners select to view sample sentences?
We did not use collocations returned by multi-word queries in this analysis because multiple-word query logs do not contain collocation pattern information.
6. Results
The first part of this section provides general information about the query entries we collected over the two-year period (from November 2017 to November 2019) before presenting the analysis results. Just over 1.5 million query entries were recorded from 140 countries, with a daily average of 2,000 queries. The top 10 countries and their corresponding percentages are shown in Table 1. A far smaller percentage of queries were made by learners based in 57 countries, which are grouped as “other” in Table 1. Roughly two thirds of queries were made by learners based in five major English-speaking countries: the United Kingdom (26.1%), New Zealand (18.7%), Australia (16.6%), Canada (5.7%), and the United States (3.5%). China (7.3%) is at the top of the list among all of the non-English-speaking countries, followed by Vietnam (3.8%), South Korea (1.5%), and Myanmar (1.3%).
Table 1. Geographic distribution of FlaxCLS users

6.1 How do learners interact with FlaxCLS while looking up collocations?
This section looks at the results of the look-up interaction analyses. Table 2 provides statistics on how learners formulate a query term and how the query formation aids are utilized. Single-word queries make up 92.8% and most (86.56%) were typed in by learners. Multi-word queries make up only a small percentage (7.2%). The most popular query formation aid used was Family Words (4.5%), followed by Synonyms (1.6%), Antonyms (0.08%), and Related Words (0.06%).
Table 2. Statistics on how learners formulate query terms

Once initial collocation results are displayed after entering a query term, learners can take further actions by clicking a hyperlink to view extended collocations (Interaction 2 in Figure 3), view more collocations (Interaction 3 in Figure 3), or view sentence samples (Interaction 4 in Figure 3). Table 3 shows the statistics for the type of interactions the learners have made with FlaxCLS. A majority of FlaxCLS users progressed only to the first step (entering a query term) of interactions to retrieve collocations (65.8%), meaning that no further interactions took place. One possible explanation could be that they had already found what they were looking for. Viewing extended collocations (16.3%), viewing sample sentences (8.2%), and viewing more collocations (6.7%) made up one third of combined interactions, indicating that a moderately high percentage of learners were engaged in the exploration of alternative collocations in addition to the original query term they had entered at the first step of their interaction with the system, and wanted to know how to use these additional collocations in sentences.
Table 3. Statistics of types of collocation hyperlinks clicks

6.2 What are the characteristics of query terms?
This section examines the characteristics of the query words that learners entered while looking up collocations. Query terms make up 47,600 unique single words and 34,500 unique multiple words. Table 4 presents the top 30 single-word queries and their respective frequencies. The top five words – research, impact, benefit, knowledge, influence – were searched for more than 1,800 times over a period of two years, more than five times per day.
Table 4. Top 30 single-word queries and their frequencies in FlaxCLS

Multi-word queries were made up of two to nine words, with an average of 2.3 words per query. Learners tended to include articles and prepositions in their multi-word searches. Out of 34,500 multi-word queries, 15% are phrasal verbs (point out, roll out, lead to, focus on, find out, carry out, figure out) based on an online dictionary. We reviewed the top 100 two- to five-word queries and discovered that discourse markers (in addition, in order to, according to, in terms of, as a result) and phrases (it could be said that, have an impact on, play an important role) commonly employed in academic writing were also prevalent. Table 5 shows the top 30 multi-word queries and their respective frequencies. Excluding market opportunity, liberal beliefs, grasp the opportunity, and negotiation subject, phrasal verbs and discourse markers make up the balance of the top 30 multi-word queries.
Table 5. Top 30 multi-word queries and their frequencies in FlaxCLS

We further categorized single-word queries using three schemes – frequency band, word class, and academic-ness – to answer the following three questions.
-
Q1. Are learners interested in collocations of common or less common words?
As shown in Table 6, single-word queries are comprised of roughly two thirds (65.7%) of those words in the top 5,000 word list and one third (34.3%) that are not. This suggests that learners are more likely to look up collocations of common words. Among the top 5,000 words, the 1 to 1,000 (24.7%) and 2,000 to 5,000 (24.5%) word-frequency bands are the most popular, followed by the 1,000 to 2,000 (16.5%) band. The number of unique-query words in the top 5,000 words paints an interesting picture. About 84% of the top 5,000 words have been queried by FlaxCLS users, with 88.2% in the 1 to 1,000, 84.7% in the 1,000 to 2,000, and 82.2% in the 2,000 to 5,000 word-frequency bands respectively.
Table 6. Statistics of single-query and unique-query words in each frequency band

In light of learners’ particular interest in querying common words, we undertook a close examination of query words in the 1 to 1,000 band. Table 7 shows that most of the query words are in the 500 to 1,000 (12.4%) band, followed by the 200 to 500 (9.1%) band. Surprisingly, there is still a small yet noticeable percentage of query terms (3.2%) from the 1 to 200 band of which we would normally assume learners are already familiar with understanding and using.
Table 7. Statistics of query words and unique-query words in each frequency band in the 1 to 1,000 band

Table 8 shows that the 1 to 200 band is comprised of a number of so-called de-lexicalized verbs (make, way, take, come, get, become) that the research suggests should be met, acquired, and recorded in collocations learning (Lewis, Reference Lewis2008). It also contains a number of shell nouns (problem, question, system) that are pervasive components of academic writing (Aktas & Cortes, Reference Aktas and Cortes2008).
Table 8. Listing of the top 30 single-word queries in the 1 to 200 frequency band

Q2. What type of words do learners frequently enter to look up collocations?
To answer this question, the single-query words are grouped according to their POS tags provided in the top 5,000 word list (Figure 4). Note that for words like support, which can be a verb or a noun, its most frequent word type – verb, in this case – is used in our analysis because it is impossible to decide word type without context. Table 9 provides query word types, examples with their frequency in brackets, and percentage in the log data set. More than half (55.1%) of query words are nouns, followed by verbs (24.2%), adjectives (15.5%), and adverbs (3.0%). There is a small percentage (2.2%) grouped under “others” that includes prepositions, conjunctions, determiners, pronouns, articles, etc. Discourse markers (however, later, moreover, thus, furthermore, hence) are the most popular adverbs. Function words such as despite (372), because (358), beyond (314), due (251), while (196), but (189), and and (153) have a surprisingly high-query frequency. Similar behavior has also been reported in research by Crosthwaite et al. (Reference Crosthwaite, Wong and Cheung2019) where words like my, our, will, and may occurred in the top 30 most frequent query terms.
Table 9. Query word types, examples, and percentage

-
Q3. Are learners interested in the collocations of academic words?
To examine the academic-ness of query words, single-word queries in the top 5,000 words were divided into academic and non-academic groups using Coxhead’s (Reference Coxhead2000) Academic Word List. The results show that 39% of query words are in Coxhead’s word list, which suggests that learners are indeed very interested in the collocations of academic words.
6.3 What are the characteristics of learners’ preferred collocations?
This section reports findings on the characteristics of FlaxCLS users’ preferred collocations.
Q1. What are the most frequently queried collocation patterns?
Table 10 shows the collocation patterns that learners selected, along with examples, and the percentage from the log data set. Adjective + noun (31.9%), verb + noun (24.4%), and noun + noun (11.3%) are the top three most popular collocation patterns. Collocation patterns containing prepositions, noun + preposition + noun (9.4%) with 6.2% in noun + of + noun, verb + preposition + noun (5.1%), adjective + preposition + noun (2.1%), which make up 16.6% in total, are also popular choices among learners. The “others” category (2.5%) covers four other collocation patterns whose percentages fall below 2%.
Table 10. Collocation patterns, examples, and percentage
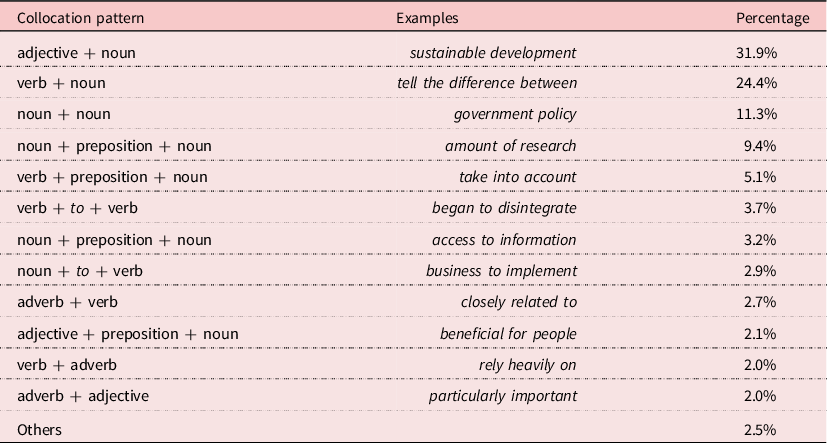
Q2. Do learners prefer collocations that have more constituent parts?
To answer this question, we examined collocations where learners progressed through FlaxCLS to look at their sample sentences based on the assumption that these collocation samples in context are most likely to be taken away by learners. Table 11 shows the distribution of the collocation length (the number of words in a collocation) and what percentage of sentence samples were viewed.
Table 11. Collocation length and viewed sentence sample percentage

FlaxCLS users viewed the sentence samples of 95,131 collocations over a period of two years. That is, for every 10 queries that learners have made in FlaxCLS there is one progressed query for viewing sample sentences. The results show that 58.1% (38.6% + 16.2% + 3.3%) of collocations for which learners viewed sample sentences contained more than two words (including articles, prepositions, etc.), suggesting that FlaxCLS users are in favor of learning longer collocations.
-
Q3. What kind of collocations do learners select to view sample sentences?
The type of collocations for which learners viewed sample sentences occurred most frequently in band 1 to 1,000. There are no sharp peaks or drops among the four bands, as indicated in Figure 5, with only a slightly higher and lower percentage in the 1 to 1,000 and the 1,000 to 2,000 bands respectively. This again confirms learners’ preferences for looking up the collocations of common words.

Figure 5. The frequency bands and viewed sentence sample percentage
7. Discussion and conclusion
We analyzed 1.5 million query entries recorded in computer logs over a period of two years to identify learners’ collocation look-up patterns in a corpus-based consultation system. First, users’ interactions with the system were examined to find out how they formulated search queries and how they followed hyperlinks to seek collocations for their learning needs. The results show that the majority of users entered query terms by themselves and only looked at the first results page without clicking through to more collocations, extended collocations, or sample sentences. Prior DDL research confirms similar behavior where learners tend to perform relatively simple searches (Pérez-Paredes et al., Reference Pérez-Paredes, Sánchez-Tornel, Alcaraz Calero and Jiménez2011; Yoon, Reference Yoon2008). Similar behavior is reflected in web search engine user query analyses – where the user types in a word, expects the system to provide what they want in an instant, and rarely reads beyond the first page of results (Xie, Yu & Cen, Reference Xie, Yu and Cen2012). The underutilization of query formation aids (Family Words, Synonyms, Antonyms, Related Words) may be due to users’ unfamiliarity with those concepts or the perception by users that the aids are not providing satisfactory results.
Second, we adopted three categorization schemes – frequency band, word class, academic-ness – to investigate the characteristics of query words users entered. With 67.5% of query words coming from the top 5,000 word list, the data suggest that learners are more likely to look up collocations for words they have already learned. These results confirm observations by Frankenberg-Garcia (Reference Frankenberg-Garcia2005) and Yoon (Reference Yoon2008) whereby students often looked up familiar words with the goal of checking or extending their existing knowledge of familiar words (e.g. finding novel ways of using them). FlaxCLS users’ interest in de-lexicalized words such as make, thing, way, get, take, and put, which carry little or no meaning in themselves, indicates that teaching and learning the collocates of high-frequency de-lexicalized words is a far more productive way for learners to spend their time and energy than studying unusual and new words, as supported by Lewis (Reference Lewis2008). We propose that a compilation of the most frequent query words would be of great value to language teachers and researchers for understanding which words students are interested in or are having difficulty with in terms of learning and employing collocations.
That almost 80% of query words are nouns (55.1%) and verbs (24.2%) is not surprising when we consider their “substantial” linguistic roles in sentence construction. In direct contrast, function words such as prepositions, determiners, and pronouns make up 2.2% of query words. This finding points to issues with DDL systems used by learners, suggesting that more guidance is required to help students make good word choices when querying DDL systems in order to achieve a positive user experience. Searching for function words alone, for example, would quite possibly lead to overwhelming and/or unsuccessful results. Such unsatisfactory user experiences in a first encounter with a corpus consultation tool could lead to some learners becoming reluctant to further engage with DDL systems.
The dominance of academic words (39%) in query words is an interesting aspect. It suggests that the user base of FlaxCLS is academically oriented students in universities or colleges, which is in line with Tribble’s (Reference Tribble, Leńko-Szymańska and Boulton2015) survey findings where nearly 80% of respondents were working in higher education. A relatively lower percentage of academic word queries by users in non-English-speaking countries may indicate that the concept of academic words and the use of academic word lists in teaching and learning are not yet widespread in non-English-speaking countries. These findings with respect to user preferences for searching academic English language has prompted us to develop a large dedicated academic collocations database with linguistic data harvested from metadata and full-text content from over 135 million texts such as journal articles, with divisions into different disciplines (e.g. arts and humanities, social sciences, physical sciences, and life sciences). The present study draws on the Wikipedia corpus, which is the default querying corpus in FlaxCLS; however, the system can just as easily draw on this new academic collocations database.
Upon further inspection of query terms, a relatively low usage (7.8%) and misuse of the multi-word query facility in FlaxCLS was revealed. This may indicate only a partial use of this functionality perhaps due to unsatisfactory query results or perhaps due to not understanding the multi-word search function. Another explanation for the low usage of the multi-word query function is that the FlaxCLS does not support queries for function words such as in, of, as, and up, instead rendering them obsolete in the collocation retrieval process. The large amount of discourse markers (in addition, in order to, according to, in terms of, as a result) entered as query terms by users may imply differences in interpretation of what constitutes a collocation. These results, again, highlight the importance of helping learners understand the strengths and limitations of corpus consultation tools so that they can develop search strategies that make the most of what DDL systems can offer.
Last but not least, with regard to the characteristics of collocations that learners preferred, all 14 collocation patterns that FlaxCLS houses were utilized by learners and some proved to be more frequently queried than others. The popularity of adjective + noun, verb + noun, and noun + noun collocations reflects the dominance of these three types of patterns, which also feature prominently in collocation dictionaries and textbooks, and coincide with the recommendations made by researchers. For example, Liu (Reference Liu2002) identified that the majority (87%) of their students’ word-choice errors were verb + noun miscollocations and particularly the misuse of verb collocates. Nesselhauf (Reference Nesselhauf2003) suggested that adjective + noun collocations are most common, but that this pairing in parts of speech is of particular difficulty for advanced learners of English to acquire. One interesting tendency for developing language proficiency (Dechert, Reference Dechert, Dechert, Möhle and Raupach1984) is that learners were in favor of collocations that contained articles and prepositions and were more than two words long, as these served as “points of fixation” or “islands of reliability.” Explicitly, learners in the Dechert study demonstrated a predilection for noun + preposition + noun (noun + of + noun, in particular) and adjective + preposition + noun patterns that have been acknowledged as essential for achieving grammatical complexity and textual density in academic writing (Biber, Gray & Poonpon, Reference Biber, Gray and Poonpon2011; Halliday, Reference Halliday, Halliday and Martin1993). However, further studies have shown that these same patterns are consistently underutilized in English for academic purposes students’ writing (Lu, Reference Lu2011; Parkinson & Musgrave, Reference Parkinson and Musgrave2014).
In conclusion, the fine-grained analyses provided in this paper offer valuable data-driven insights into corpus use that would be difficult to gain from small and short-term studies that solely rely on observation or self-reports. Nevertheless, our data analysis approach has its weaknesses. Our data are derived from observable artifacts of what the learners actually did: when learners searched and for how long, what word(s) they searched for, which querying facilities they used (Family Words, Synonyms, Antonyms, Related Words), whether they viewed example sentences of a collocation, and so on. We know much less about learners’ motivations for using the system, and whether they are satisfied with their experience of using the system, and the results returned to them by the system in response to their queries. Apart from information pertaining to their geographic regions, we are none the wiser about how learners may have employed their search results for language learning or for real-world language application purposes, if at all – which collocations were taken away and whether or not they were used and how. Future studies would benefit from linking tracking data to individual learners, and other techniques (i.e. traditional DDL research methods), to provide a more complete picture of learners’ successes and struggles in becoming “research workers” or “language detectives to explore language data themselves” (Johns, Reference Johns, Wichmann, Fligelstone, McEnery and Knowles1997: 101). Subsequently, such mixed methods of research can inform the design and development of training materials, and the redesign of corpus-based software or web services for cultivating long-term habitual behavior in corpus consultation by learners (Chan & Liou Reference Chan and Liou2005; Crosthwaite et al., Reference Crosthwaite, Wong and Cheung2019; Hafner & Candlin, Reference Hafner and Candlin2007; Pérez-Paredes et al., Reference Pérez-Paredes, Sánchez-Tornel, Alcaraz Calero and Jiménez2011; Wu et al., Reference Wu, Fitzgerald, Yu and Witten2019).
Although our research has shown possible ways for utilizing computer log data and has demonstrated the value of these approaches in the DDL literature, we acknowledge that collecting and processing log data is not always easy and more often impractical for DDL researchers and teachers who do not have the in-house tools nor the technical support (Kennedy & Miceli, Reference Kennedy and Miceli2017). FlaxCLS currently provides facilities for linking log data to individual users if the user enters a unique ID while using FlaxCLS, whereby data can be made available to researchers and teachers upon request. Finally, we would like to initiate a call for research methodologies and technologies that ensure log data is easily accessible to stakeholders who wish to take full advantage of the affordances that automated methods for tracking learner queries and interactions with DDL systems can provide as we have presented in this paper.
Ethical statement
FlaxCLS is free to use and it does not require users to log in or register when looking up collocations. The data analysis was conducted with anonymous user query data recorded in computer logs from which individual users cannot be identified.
About the authors
Shaoqun Wu is a senior lecturer at the Faculty of Computing and Mathematical Sciences, University of Waikato, New Zealand. Her research interests include computer-assisted language learning, data-driven learning, mobile language learning, supporting language learning in MOOCs, and text mining.
Alannah Fitzgerald is postdoctoral research fellow at the University of Waikato in New Zealand. She is an open education practitioner and researcher working across formal and non-formal higher education. Her research interests include computer-assisted language learning, data-driven learning, English for specific and academic purposes, MOOCs, open education, and self-regulated learning.
Alex Yu is a senior lecturer at the Centre for Business, Information Technology and Enterprise at Waikato Institute of Technology, New Zealand. His research interests include computer-assisted language learning, MOOCs, mobile language learning, and data mining.
Zexuan Chen is an associate professor of foreign studies at the Southern Medical University, China. She is also a PhD student in educational technology at the School of Information Technology in Education, South China Normal University. Her research interests lie in technology-enhanced language teaching and learning, blended learning, corpus-assisted L2 writing, etc.
Author ORCIDs
Shaoqun Wu, https://orcid.org/0000-0001-9566-005X
Alannah Fitzgerald, https://orcid.org/0000-0003-0392-2740
Alex Yu, https://orcid.org/0000-0001-6068-4721
Zexuan Chen, https://orcid.org/0000-0002-2310-5126