1. Introduction
According to Vygotsky’s (Reference Vygotsky1978) sociocultural theory, object-oriented learning is mediated by tools or artifacts in conjunction with scaffolding and support provided by teachers or other capable peers. Textbooks and dictionaries are traditionally employed to support English as a foreign language (EFL) students in their writing. Over the past few decades, digital technologies such as spell check, grammar check, electronic translators, and, more recently, machine translation (MT) have helped students to autonomously analyze and revise their written work. However, the value of such digital technologies is not always readily acknowledged by language teachers, and often for good reason. The use of MT, for example, has long been stigmatized among teachers and students alike due to its inaccuracies (Lee, Reference Lee2020). Further concerns indicate that the use of MT may contribute to student disengagement from the cognitively demanding processes of writing in a foreign language (Crossely, Reference Crossely2018; Im, Reference Im2017). Although these concerns are certainly valid, it is important to carefully examine the potential of today’s MT technology as a language learning tool without being biased by its historical inaccuracies.
The accuracy of MT technology is continuously improving. Although typically in gradual increments, such improvements are occasionally marked as a result of new technological developments. One such notable leap occurred in 2016 when Google introduced neural machine translation (NMT), a technology that quickly surpassed its predecessor, statistical machine translation (SMT), in terms of accuracy (Lewis-Kraus, Reference Lewis-Kraus2016; Sun, Reference Sun2017). Educators must not overlook the significance of this relatively recent shift in MT technology, particularly because studies show that its use is already widespread among students (i.e. Alhaisoni & Alhaysony, Reference Alhaisoni and Alhaysony2017; Briggs, Reference Briggs2018). To date, NMT provides students with access to instant translations of the learners’ first language (referred to hereafter as L1), which can be remarkably accurate depending on the nature of the user’s input.
Second language (referred to hereafter as L2) writing is a particular domain in which MT can effectively mediate student learning in the university EFL context. As one of the most challenging areas to master for EFL students, aspiring writers generally require extensive amounts of teacher instruction and feedback to steadily improve (Ferris, Reference Ferris2011; Ferris & Roberts, Reference Ferris and Roberts2001). The underlying reason for this assertion is that when working independently, students with limited English proficiency levels can feel cognitively overwhelmed by the mere prospect of beginning the L2 writing process. Students with advanced English proficiency levels, conversely, may express ideas with relative ease but struggle to engage in the higher-order thinking processes that are required to effectively analyze and revise their work. Although frequent and persistent support and feedback are generally required by the teacher to help students navigate through such challenges, teacher availability is commonly constrained by large class sizes and limited instructional hours in general (Ferris, Liu & Rabie, Reference Ferris, Liu and Rabie2011). Furthermore, with the demands of the workforce looming in their futures, university students often lack the time to work step by step through the long and arduous processes of becoming proficient English writers.
Therefore, students need to learn the effective use of MT to address these issues and effectively mediate the L2 composition processes. In other words, MT cannot remain the proverbial elephant in the EFL room. Evidence has indicated that when used strategically, MT outputs can serve as a valuable point of comparison with student-produced L2 production and help them improve their written production (Lee, Reference Lee2020; Tsai, Reference Tsai2019). As the accuracy of MT continues to improve, its value as a point of comparison with students’ original L2 texts is also bound to continuously improve.
2. Literature review
2.1 From statistical machine translation to neural machine translation
In any MT-related discussion, acknowledging that the technology has undergone a paradigmatic shift in recent years is important. SMT, the long-dominant MT technology that functions by rapidly analyzing millions of parallel bilingual texts, was replaced by NMT in 2016 (Lewis-Kraus, Reference Lewis-Kraus2016; Sun, Reference Sun2017). In contrast with SMT, which analyzes small numbers of words at a time, NMT analyzes entire sentences (Ducar & Schocket, Reference Ducar and Schocket2018). In other words, the fluency of NMT outputs is generally better than that of SMT outputs. NMT is a relatively recent technological development. Thus, recognizing that the vast majority of past MT studies can be attributed to the use of SMT is important. NMT is a distinctly different translation technology than SMT, which was famously known for its inaccuracies concerning grammatical structure, poor lexical selection, and occasional production of incomprehensible sentences (Bahri & Mahadi, Reference Bahri and Mahadi2016).
Although NMT produces more accurate outputs than its predecessor, it continues to have its imperfections and error tendencies. Recent studies have consistently indicated that NMT has difficulties producing accurate outputs for inputs that contain long sentences, ambiguous sentence structures, and low-frequency lexis (Bowker & Ciro, Reference Bowker and Ciro2019; Koehn & Knowles, Reference Koehn, Knowles, Luong, Birch, Neubig and Finch2017). However, even such error tendencies cannot be regarded as static. This is because, as Ducar and Schocket (Reference Ducar and Schocket2018) argued, NMT is capable of learning and is continually improving its ability to translate idioms, proper nouns, and less commonly used words. The authors noted that pragmatic and discourse aspects, such as the level of formality and cultural expectations, are a few domains that remain beyond its capabilities.
2.2 Machine translation as a language learning tool
Despite findings suggesting that MT use is widespread among EFL students (Briggs, Reference Briggs2018), studies indicate that teachers are hesitant to introduce this technology to L2 classrooms (Alhaisoni & Alhaysony, Reference Alhaisoni and Alhaysony2017; Van Praag & Sanchez, Reference Van Praag and Sanchez2015). In part, this can be attributed to the fact that relying on L1 as a basis for L2 composition is contradictory to historically established pedagogical norms. L2 composition is generally taught via a process-based approach through which learners are encouraged to engage in the recursive process of pre-writing, composing multiple drafts, and extensive revision based on teacher or peer feedback (Ferris & Hedgcock, Reference Ferris and Hedgcock2011). The use of L1 is typically discouraged throughout the L2 writing process because of the negative transfer that can result from cross-linguistic differences between languages (Göpferich, Reference Göpferich, Schwieter and Ferreira2017). However, numerous studies have found pedagogical benefits of L1 use in L2 writing. It plays an encouraging role for compensatory and ideational purposes (Kim & Yoon, Reference Kim and Yoon2014), helps students generate ideas and plan and monitor the L2 writing process (Wang & Wen, Reference Wang and Wen2002), and leads to better performance in L2 writing (Kim, Reference Kim2011; Stapa & Majid, Reference Stapa and Majid2012).
Another reason that L2 teachers tend to hold a negative view of using MT is that it encourages the use of translation in L2 writing. Translation, in contrast with L2 process writing, can be defined as “the act of transferring the linguistic entities from one language in to their equivalents in to another language” (Osman, Reference Osman2017: para. 1). Although there are discrepancies among studies, advantages of translation in L2 writing have been found for particular student ability levels, task types, and teacher interventions. Studies indicate that translation, and particularly mental translation, occurs naturally in the L2 writing process and helps to promote L2 reading and writing skills, reduce cognitive load, and enhance learner autonomy (Kim, Reference Kim2011; Liu, Reference Liu2009). Additionally, research has shown translation to be the most frequently used L2 writing strategy among Asian EFL students, particularly low-proficiency students (Kim, Reference Kim2011; Kim & Yoon, Reference Kim and Yoon2014; Wang & Wen, Reference Wang and Wen2002).
Teachers’ reluctance is also often related to their doubts about the pedagogical effectiveness of MT. However, studies show that MT can be of benefit in diverse areas of L2 classrooms. From the L2 writing perspective, MT can support students’ efforts to autonomously analyze and revise their written work (Baraniello et al., Reference Baraniello, Degano, Laura, Zahonero, Naldi and Petroni2016). Studies have confirmed that using MT during the L2 writing process helps students to enhance the overall quality of their writing (Kol, Schcolnik & Spector-Cohen, Reference Kol, Schcolnik and Spector-Cohen2018; O’Neill, Reference O’Neill2016; Stapleton & Kin, Reference Stapleton and Kin2019), focus more on content (Kim, Reference Kim2011; O’Neill, Reference O’Neill2016), and produce more lexically accurate and complex texts (Fredholm, Reference Fredholm2019) with fewer orthographic errors (O’Brien, Simard & Goulet, Reference O’Brien, Simard, Goulet, Moorkens, Castilho, Gaspari and Doherty2018; Shadiev, Sun & Huang, Reference Shadiev, Sun and Huang2019). Even in the relative infancy of MT technology, its outputs were found to benefit beginner writers in terms of improving the quality, quantity, and fluency of L2 writing (Garcia & Peña, Reference Garcia and Peña2011). As the accuracy of MT continues to improve, the potential value of MT outputs improves as a point of comparison with learner-produced, human translations. Furthermore, it can provide students with a source of individualized feedback, which they can compare with their L2 translations to help them draw inferences, paraphrase, and make revisions throughout the editing process (Lee, Reference Lee2020). Similarly, research indicates that students are capable of strategically using MT to aid in the decoding of unfamiliar vocabulary, general reading comprehension, and writing composition (Alhaisoni & Alhaysony, Reference Alhaisoni and Alhaysony2017).
MT also has specific benefits pertaining to the cognitive and affective domains of language learning. From the cognitive perspective, using L1 as an input, the cognitive load associated with L2 language production can be largely reduced and can prevent students from feeling cognitively overwhelmed and disengaged from the writing process (Bahri & Mahadi, Reference Bahri and Mahadi2016; Garcia & Peña, Reference Garcia and Peña2011; Niño, Reference Niño2008; Van Praag & Sanchez, Reference Van Praag and Sanchez2015). This, in turn, from the affective perspective, can create a non-threatening L2 learning environment (Niño, Reference Niño2008), reduce student language anxiety, and increase their confidence and motivation (Bahri & Mahadi, Reference Bahri and Mahadi2016; Niño, Reference Niño2008). Writing or revising with MT, from the metacognitive perspective, can increase students’ linguistic awareness (Lee, Reference Lee2020), increase higher-order thinking skills (Yang & Wang, Reference Yang and Wang2019), and promote independent learning strategies and self-directed learning (Bernadini, Reference Bernardini2016; Garcia & Peña, Reference Garcia and Peña2011).
2.3 Machine translation and error correction in L2 writing
Writing is a complex cognitive process that requires various skills at multiple stages and demands considerable cognitive awareness. L2 writers must focus on many details during writing (i.e. language, content, organization, and mechanics) and are limited by cognitive capacities such as attention and working memory (Waller & Papi, Reference Waller and Papi2017). Thus, L2 writers are prone to producing more linguistic errors compared with L1 writers (Goulet, Simard, Parra Escartín & O’Brien, Reference Goulet, Simard, Parra Escartín and O’Brien2017). Therefore, revision is important for L2 writing, because without it, producing high-quality writing may be difficult for L2 students. Corrective feedback can be considered a key catalyst for making effective revisions, as the majority of studies have confirmed that students receiving corrective feedback outperform those who do not (Ferris, Reference Ferris2010, Reference Ferris2011; Sheen, Reference Sheen2007). As such, effectively engaging in the processes of error correction throughout the revision process is of primary interest to students and instructors (Han & Hyland, Reference Han and Hyland2015).
Although error correction is an essential aspect of the L2 revision process, numerous studies have indicated that students’ abilities to utilize such feedback differ according to their L2 ability levels. For example, Sheen (Reference Sheen2007) focused on the relationship between language aptitude and learning outcome. He provided evidence that students with high levels of language aptitude benefited more from corrective feedback than those with low aptitude levels. The reason underlying this mechanism is because students were more likely to use such feedback to increase metalinguistic awareness. Similar studies have consistently revealed that students with high L2 proficiencies produce higher quality revisions with the aid of large amounts of prior knowledge than those with low proficiency levels (Lee, Reference Lee2014; Qi & Lapkin, Reference Qi and Lapkin2001).
Based on the research pertaining to the ways that students of various ability levels cope with corrective feedback, students of diverse L2 ability levels are expected to utilize MT outputs in different ways. This point is evidenced by a study that revealed that EFL students with low proficiency levels often lack the capability to select better options in their writing (Pae, Reference Pae2008). Similarly, Fredholm (Reference Fredholm2015) determined that although MT may have the potential to provide students with viable alternatives to their L2 texts, only students with high proficiency levels effectively used its outputs to improve their L2 writing. Additional studies have suggested that using MT is more effective and appropriate for intermediate or advanced levels of learners than that for beginners (Bahri and Mahadi, Reference Bahri and Mahadi2016; Lee, Reference Lee2020; Tsai, Reference Tsai2019). Particularly in Lee’s (Reference Lee2020) study, higher-level EFL students significantly reduced lexical and grammatical errors in their revisions with the help of MT, whereas students of low proficiency levels were frequently unable to select better alternatives provided by MT due to their lack of L2 knowledge and confidence.
Students can use MT outputs to mediate the revision of their written work via two methods. The most commonly employed technique is “post-editing,” a process by which humans edit MT outputs “in order to correct any errors and make the text sound more natural” (Bowker & Ciro, Reference Bowker and Ciro2019: 76). Another approach, as indicated by Correa (Reference Correa2014), is “pre-editing” at the L1 input level. In pre-editing, a writer carefully checks the source text for spelling mistakes, correct use of punctuation, and potential ambiguity within sentences to ensure the production of additional accurate MT outputs. Throughout such editing processes, students are afforded the opportunity to better recognize the possibility that multiple forms and expressions can accurately be applied throughout the translation process (Baraniello et al., Reference Baraniello, Degano, Laura, Zahonero, Naldi and Petroni2016). In other words, MT can be used in diverse ways to support students’ efforts to compose accurate L2 writing. In pre-editing and post-editing, MT provides students with an output that they can then compare with their L2 translations.
Despite its apparent value as an L2 learning tool, studies that have focused on the accuracy of MT and the effectiveness of using MT to support the L2 revision process are rare in the EFL context. As MT is rapidly improving in terms of accuracy, up-to-date research on the use of MT as an error analysis tool is required. Understanding the strengths and weaknesses of MT is imperative to maximize its effectiveness as a pedagogical tool in L2 writing classrooms. Therefore, this study addresses the following issues:
-
1. Did using MT help Korean EFL students correct errors during revision? If so, which error types were reduced the most?
-
2. Were differences noted between error frequency groupings regarding the number of errors in the original versions and the number of changes, corrections, and new errors in the revised texts?
3. Methodology
The participants of the study comprised 58 (male = 36, female = 22) Korean college students with diverse majors and enrolled in general English courses. Except for six sophomore students, the participants were freshmen. The first language of all participants was Korean, and their English proficiencies ranged from low-intermediate to high-intermediate. The students first wrote essays (half a page) in Korean (source text) and translated it into English (L2 text). The students were permitted to use other additional resources such as dictionaries and could utilize the spell checker in MS Word. As the last step, the participants translated the text again using Google Translate (MT output). They were tasked with comparing their L2 texts with the MT outputs, examining the texts for differences, detecting errors, and revising the texts (revised text).
The students were directed to produce an L1 source text as a starting point for this assignment for three reasons. First, it ensured that all students regardless of proficiency level would be able to independently engage in the revision of their L2 writing. Second, it served as a control that “maximized” the students’ use of MT. In all cases, students used MT to produce a complete translation of their Korean source text. Lastly, the provision of an L1 source text allowed the researchers to make clear comparisons of the students’ work and to detect the sources of their errors. The writing task was conducted as a take-home assignment at the beginning of the semester.
Two trained evaluators performed an error analysis of the students’ L2 and revised texts. In the study, T-unit analysis was used as it is the most widely used in error analysis (Andujar, Reference Andujar2016). In addition, as multiple errors were frequently found in a single T-unit that would skew the results, the error ratio per word was also counted to provide a more robust analysis. Particularly when error analyses were conducted in diverse areas not limited to lexical and grammatical errors but also including orthographic errors, previous studies, and particularly MT studies, commonly analyzed the total number of errors (i.e. Fredholm, Reference Fredholm2015; Niño, Reference Niño2008; O’Brien et al., Reference O’Brien, Simard, Goulet, Moorkens, Castilho, Gaspari and Doherty2018) or errors per number of words (i.e. Lee, Reference Lee2020; Tsai, Reference Tsai2019). The units of analysis in this study were errors and changes that students produced at any level of symbol, word, phrase, or clause/sentence. The researchers first established error types based on the literature (Ferris, Reference Ferris2011; Goulet et al., Reference Goulet, Simard, Parra Escartín and O’Brien2017; Kim, Reference Kim2019). Then, error types that distinctively and repetitively appeared in the Korean students’ L2 texts were finalized through the reiterative processes of error analysis. This step resulted in 12 distinctive categories, namely Noun Plurals, Verb Tense, Verb Form, Subject–Verb Agreement, Articles, Prepositions, Insertions/Deletions, Word Order, Substitutions, Incomprehensibility, Fragments, and Punctuation, as displayed in Table 1.
Table 1. Error types
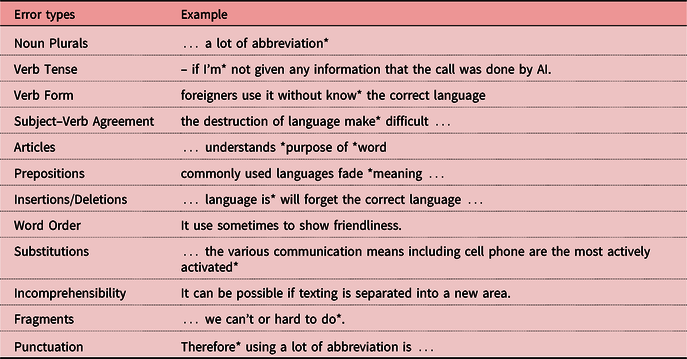
Additional details are provided to clarify the criteria for categorizing the respective error types. Insertions/Deletions refer to errors in which words or phrases were incorrectly added to or omitted from students’ composition. Substitutions refer to the incorrect choice of words. Insertions/Deletions and Substitutions pertaining specifically to the use of articles and prepositions were categorized into their respective categories. Incomprehensibility refers to phrasing in which the evaluator cannot grasp the author’s intended meaning.
With respect to the revised texts, the researchers conducted a complex multistep analysis. First, changes were categorized in terms of level: symbol, word, phrase, and sentence/clause. No changes were made at the paragraph level as the students were instructed to edit rather than replace L2 texts. Then, the errors that occurred in the revised texts were compared to those in the L2 texts and were evaluated according to whether the errors were corrected (positive change), remained incorrect (neutral change), or new errors were added to the revised text (negative change). Finally, the final errors were categorized into 12 error types.
During analysis, each evaluator carefully examined the L2 samples, categorized the errors, cross-examined each other’s analysis, discussed discrepancies between them, drew consensus, and continued to apply the agreed-upon procedures to analyze the subsequent samples. Through continuous dialogues during analysis, the researchers were able to identify and eliminate the vast majority of discrepancies. The inter-coder reliability of the final analysis after several rounds of cross-checking reached 93%.
All errors found in the L2 and revised texts were recorded in terms of error types, and multiple steps of quantitative analyses were conducted. First, descriptive statistics were employed to determine the frequencies and means of each error type. Second, t-tests were conducted to verify whether any significant differences were observed for each error type as well as the total number of errors in the original L2 and revised texts. Next, the students were categorized into three groups based on the frequency of errors in the original versions. Groups 1 (N = 19), 2 (N = 23), and 3 (N = 16) comprised students who produced between 1 and 10, between 11 and 20, and over 20 errors per 100 words, respectively. ANOVA was employed to compare the results, such as the number of errors, changes, corrections, and new errors, as noted in the students’ texts among the grammar proficiency groups. Finally, regression was conducted to determine whether the number of changes made by students influenced the final number of errors in the revised texts.
4. Results
The average word count of the students’ original L2 texts was 206.67, and the errors that occurred within such L2 texts were analyzed in terms of the 12 error types. Table 2 presents the results according to the frequency of errors, where Insertions/Deletions was the most frequently occurring error type, followed by Noun Plurals and Substitutions. The least frequently occurring error type was Fragments, followed by Word Order and Incomprehensibility. The average number of overall errors occurring in the L2 texts was 15.14 per 100 written words.
Table 2. Descriptive statistics and t-test

Results revealed that the word count increased (M = 219.67) in the revised texts, whereas the number of errors per 100 words was reduced in all areas of the revised texts. The results also show that the error-free T-units of the revised drafts significantly increased (p < 0.001) in comparison to the original L2 texts. Table 3 reveals that the students made 7.02 changes per 100 words on average in the revised texts. Regarding the level of change, students made changes most frequently at the word level, followed by the changes at the phrase, clause, and symbol levels.
Table 3. Level changes

a Per 100 words.
As shown in Table 4, the students made positive changes (error correction) most frequently, followed by neutral changes (no change) and negative changes (addition of new errors). In summary, the results indicated that students improved their revised texts by 2.67 errors per 100 words. Taking a close look, the results provide evidence that MT most frequently helped students to make corrections concerning errors in Articles, Prepositions, Noun Plurals, and Substitutions. The mean of the total frequency of errors in the revised texts was 12.48 per 100 words in comparison with 15.14 in the L2 texts. The results of the t-test confirmed that the differences between the two versions were significant for all areas except for Fragments and Incomprehensibility (Table 2).
Table 4. Positive and negative changes per 100 words

Based on the number of errors produced in the original L2 texts, the students were categorized into three distinct groups. The mean numbers of errors for Groups 1, 2, and 3 were 7.26 (SD = 2.314), 13.80 (SD = 2.5253), and 26.44 (SD = 4.46), respectively. Table 5 provides further descriptive statistics for the groups. With a few exceptions, the groups predictably tended to produce increasing amounts of errors in each category. Group 1 most frequently made errors in Articles, Insertions/Deletions, and Substitutions. In comparison, Group 2 most frequently made errors in Insertions/Deletions followed by Noun Plurals and Substitutions. Group 3 most commonly made errors in Noun Plurals, closely followed by Insertions/Deletions and Punctuation.
Table 5. Details of error frequency per group

The results of ANOVA in Table 6 reveal that significant differences exist among the groups with respect to each error type except for Articles and Prepositions. However, no significant differences were noted between groups for the overall number of corrections, new errors, or the overall number of changes in their revised texts.
Table 6. Differences among groups
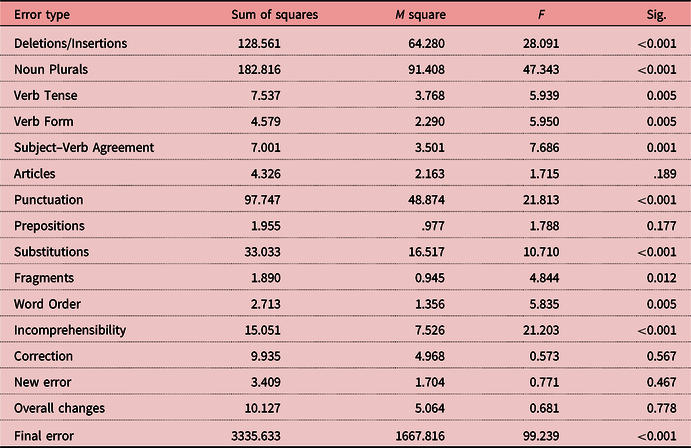
Table 7 reveals the regression analysis results and indicates that the numbers of changes were positively correlated with the reduction in errors in the revised texts (R 2 = −0.357, p = 0.006). The result confirmed that the more changes the students made, the more the errors were corrected. The overall change for the groups varied from 7.40, 7.14, and 6.36 corrections/100 words for Groups 1, 2, and 3, respectively (Table 5). However, the groups produced increasingly frequent errors in the L2 texts (i.e. 7.26, 13.80, and 26.44 errors/100 words, respectively). Thus the differences in the ratios at which corrections were made are worth noting. In the final draft, Group 1 successfully corrected 28.9% of their original errors, Group 2 corrected 23.2%, and Group 3 corrected only 10.0%. These findings suggest that students who committed fewer errors were able to correct a large proportion of their errors than those who more frequently committed errors.
Table 7. Relation between the number of changes and error reductions

5. Discussion
Results revealed that the errors in the revised texts decreased for all error types, and t-tests confirmed that the number of error corrections between the two versions was statistically significant in all but two of the 12 categories. Concerning such changes, the largest improvements were noted for Articles and Prepositions. The most frequent error types were largely attributed to the distinctive differences between the two languages. For example, the Korean language lacks definite and indefinite articles. In English, prepositions precede the relevant noun phrase, whereas the opposite is true for the Korean language (Song, Reference Song2006). Therefore, consistent with the findings of Lee (Reference Lee2014), the two error types were found to persist even among Korean university students who have lived in English-speaking countries. Prepositions have been identified as an uncorrectable error type (Bitchener, Young & Cameron, Reference Bitchener, Young and Cameron2005) and the most difficult area for Korean students to address (Back, Reference Back2011). In other words, students are unlikely to detect such errors independently without direct feedback. The results of this study indicate that the challenge of correcting Articles and Prepositions was consistent among the error frequency groups because no statistically significant differences were revealed.
The next most frequently occurring error types were Noun Plurals and Substitutions. Although the Korean language uses nouns in plural form, they are rarely used in speaking or writing. Thus, Korean students are prone to omitting plurals when writing in English. Nonetheless, previous studies have revealed that learners can revise such errors and improve in terms of Articles, Prepositions, and Noun Plurals when provided with sufficient and adequate corrective feedback (Back, Reference Back2011; Nassaji, Reference Nassaji2011; Sheen, Reference Sheen2007). The results of this study are consistent with such findings, as the students significantly reduced these types of errors while making minimum negative changes. The students significantly reduced the frequency of errors in Substitutions in meaning and form errors (see the examples that follow). Their successes with making such changes may be attributed to evidence suggesting that NMT has become capable of understanding context, and is increasingly able to select words appropriate to that particular context (Ducar & Schocket, Reference Ducar and Schocket2018).
-
Example of meaning errors in Substitutions
-
[L2 text] using improper language in a public seat → [revised text] using improper language in a public place
-
Example of form errors in Substitutions
-
[L2 text] lose of mother tongue → [revised text] the loss of mother tongue
Although the overall changes were predominantly positive, the results revealed that the students also frequently made neutral or negative changes for the error category of Insertions/Deletions. Among the various errors in this category, two subtypes of errors often remained uncorrected. The first one is the redundant copular verb “be,” as indicated in the following examples:
-
1. … abbreviations are make sense (neutral change)
-
2. [L2 text] It is a factor that is harmful to our language. → [revised text] It is a factor that is hinders to our language. (negative change)
As the examples demonstrate, Korean students with low-level English proficiency often use the redundant copular verb “be,” attributable to the intralingual interference between the language pair (Lee, Reference Lee2014). That is, in Korean, the verb equivalent to the English “be” is often attached to another verb without changing its meaning or grammatical function, which may result in the redundant usage of “be” in English. Another frequent form of the Insertions/Deletions error that persisted in the revised versions were missing pronouns. In particular, pronouns in the object position were frequently omitted. An example of this error is demonstrated in the following example of a student composition “People seem to use it frequently,” where the italicized “it” was deleted. This type of error is also derived from the interference of L1, with the feature of the Korean language as a pro-drop language (Kim, Reference Kim2019). This suggests that the students’ habits of omitting pronouns in Korean seem to frequently transfer to their L2 writing. Both types of errors in the Insertions/Deletions category may be developmental errors, which might improve in the later stages of learning. However, this aspect is frequently plateaued or fossilized and, accordingly, difficult for students with low proficiency levels to improve upon. This finding is corroborated by Niño (Reference Niño2008), who claimed that compared with lexical errors, grammatical errors are more complicated to fix. In this study, Insertions/Deletions errors are classified as grammatical errors rather than lexical errors, hence such errors were increasingly difficult to correct.
The current study revealed that the use of MT helped students to correct a significant number of errors in Articles, Prepositions, Insertions/Deletions, and Substitutions. Studies have confirmed that errors in these areas can improve with corrective feedback (Back, Reference Back2011; Sheen, Reference Sheen2007). As MT has reached a high level of accuracy in articles, prepositions, pronouns, and verbs (Ducar & Schocket, Reference Ducar and Schocket2018; Fredholm, Reference Fredholm2015), its outputs in this study served as corrective feedback that helped the students to revise their errors. Kim (Reference Kim2011) argues that, even when having adequate L2 knowledge, Korean college students often cannot apply their knowledge in L2 writing and translation tasks. She found that students usually benefit from translation tasks and improve lexical and grammatical accuracy in their L2 writing. This is because translation tasks help them to activate their L2 linguistic knowledge that they acquired through translation exercises in secondary school and ultimately helped them to make connections between their knowledge and L2 writing. The results of this study are consistent with Lee’s (Reference Lee2020) findings in terms of providing evidence that the process of translation, error detection, and correction via the use of MT raised the students’ linguistic awareness and helped them enhance lexico-grammatical accuracy in revisions.
In contrast, the Fragments and Incomprehensibility categories revealed no significant differences between the L2 and revised texts. This result may be due to two possible reasons. First, the occurrence of the two error types was scarce, a condition that greatly reduces the likelihood of statistical differences. Second, Fragments and Incomprehensibility can be attributed to errors associated with the lack of basic grammatical knowledge about sentence structure. This point is evident in this study, where Table 5 indicates that students with limited writing proficiency levels predictably tend to commit these types of errors frequently. Notably, Group 3 produced errors in these categories more frequently (0.09, 0.25, and 0.55 errors/100 words for Fragments and 0.00, 0.09, and 1.19 errors/100 words for Incomprehensibility for Groups 1, 2, and 3, respectively). Ferris and Roberts (Reference Ferris and Roberts2001) claimed that various linguistic categories, such as lexical, syntactic, and morphological, are acquired through progressively complex stages, and sentence structures are considered the most difficult category. Hence, making accurate error corrections for Fragments and Incomprehensibility, which require linguistic knowledge about sentence structure, can be an extremely challenging task for students with limited proficiency levels. Furthermore, addressing complex errors at the sentence and clause levels can quickly lead to cognitive overload for students with low proficiency levels (Crossely, Reference Crossely2018; Im, Reference Im2017). In other words, these students are prone to giving up on correcting such complex, global errors while remaining focused on addressing minor errors, such as Articles and Prepositions, which require relatively less cognitive resources.
Importantly, the results of this study revealed that students who less frequently committed errors in their L2 texts were able to proportionately make more corrections than the students in the groups that committed errors relatively more frequently. A few explanations for this result are possible. First, as numerous prior studies have revealed (Bitchener et al., Reference Bitchener, Young and Cameron2005; Fredholm, Reference Fredholm2015; Lee, Reference Lee2014; Qi & Lapkin, Reference Qi and Lapkin2001; Sheen, Reference Sheen2007), students with high proficiency levels engage better in higher-order thinking processes, which are required to effectively revise their work. In this study, this point extends to the use of MT and students’ ability to notice key differences between their L2 texts and MT outputs and to use that information to effectively detect and treat errors in their L2 composition. A second explanation lies in the students’ lack of confidence. Even when MT offered other accurate alternatives, students often chose not to adopt these in their revisions. According to Lee (Reference Lee2014), this tendency was ascribed to the lack of confidence among students, and particularly among low-level students, regarding the certainty of whether their changes will be correct. Although the lack of confidence can potentially account for the students’ non-adoption of the MT outputs in this study, further investigation should be conducted to confirm this point.
Based on the results of the study, numerous pedagogical implications can be inferred. First, in English as a second language contexts such as Korea, most students’ lives and work will be predominantly conducted in their L1. Accordingly, learning to use MT and to simultaneously develop an awareness of cross-linguistic differences between their L1 and L2 is likely to be of great value. Specifically, this study shows that MT helps to mediate the L2 editing process by providing students with the unique opportunity to gain instant access to text, which can facilitate the comparison, analysis, and replacement of their L2 texts. Although it was beyond the scope of the current paper, it is recommended that the results of such MT assignments be used by the teacher to draw student attention toward common issues of negative transfer and to provide additional practice through which students can learn to correct their errors with greater confidence and efficiency (Bahri & Mahadi, Reference Bahri and Mahadi2016; Goodwin-Jones, Reference Goodwin-Jones2015; Kim, Reference Kim2019; Tsai, Reference Tsai2019).
In addition to the potential benefits associated with MT use, teachers should carefully consider several caveats. For example, students with limited L2 writing proficiency levels are likely to notice that the quality of the MT outputs is superior to that of their writing. Accordingly, those students may be tempted to depend on those outputs rather than engage in the cognitively demanding processes associated with L2 composition. Although this possibility is commonly cited by teachers as a reason for prohibiting students from using MT (Van Praag & Sanchez, Reference Van Praag and Sanchez2015), increasing student accountability by requiring them to provide multiple versions of their work (i.e. source text, L2 text, and revised text) can help to reduce student dependency on MT outputs. Moreover, it has been proposed that beginner and intermediate EFL learners have increased language anxiety toward lexico-grammatical errors (Lee, Reference Lee2014), a condition that largely limits their attention only to linguistic features. As such, other important features of writing such as content, organization, and writing fluency are often ignored. MT outputs can mitigate this situation and help learners focus their cognitive resources on ideas and content.
To facilitate students’ use of MT outputs, teachers should take several specific measures. First, teachers can inform students of the lexical and grammatical areas that are considered strengths or weaknesses of the current MT. For instance, average-level EFL Korean students may benefit from using MT to aid in the correction of article- and preposition-related errors. However, they should exercise caution when using MT to translate culturally charged expressions or polysemic words (Ducar & Schocket, Reference Ducar and Schocket2018; Kim, Reference Kim2019). Second, although beyond the scope of this paper, students are likely to produce accurate revisions by encouraging the use of MT in conjunction with other existing and emerging spell check and grammar check technologies. Third, in the effort to produce grammatically coherent sentences, students can also be instructed to use pre-editing strategies by rephrasing the L1 input to produce alternative L2 outputs (Correa, Reference Correa2014). Finally, students will likely increase their ability to use the tool effectively when provided with additional scaffolding and support for MT-related tasks (Kim, Reference Kim2019; Tsai, Reference Tsai2019). For example, teachers can provide students with sets of L1 inputs and practice tasks designed to draw student attention toward correcting specific error types.
This study investigated the ways in which MT mediated EFL students’ revision process and facilitated L2 writing by reducing lexico-grammatical errors. The result, however, is limited to the specific language pair between Korean and English. Different pairs of languages may lead to diverse results, as MT accuracy varies depending on language pairs (Goulet et al., Reference Goulet, Simard, Parra Escartín and O’Brien2017; Shadiev et al., Reference Shadiev, Sun and Huang2019). Moreover, students across mother languages are prone to making idiosyncratic lexical and grammatical errors. Also, as MT advances, its accuracy will improve accordingly. Therefore, updated research is consistently required in this field. Longitudinal studies on using MT will also be imperative to enhancing the understanding of the long-term mediating influence of MT on L2 writing. Furthermore, research is required to identify the type of pedagogical activities that may be most beneficial to L2 writing concerning the use of MT.
6. Conclusion
Although accuracy may not be the single most important criterion for overall L2 writing development, it cannot and should not be overlooked. This point is supported by previous studies that have verified the positive effects of language-focused correction on L2 writing (Bruton, Reference Bruton2009; Ferris, Reference Ferris2011) and noted that improved grammar is a characteristic of enhanced text quality (Min, Reference Min2006). However, teacher feedback often remains limited in L2 writing classrooms, and students lack opportunities to receive individualized corrections (Ferris, Liu & Rabie, Reference Ferris, Liu and Rabie2011). Considering this reality alongside the findings of this study, MT can effectively serve as an alternative tool for mediating students’ revision process and facilitating accurate L2 writing.
This study has revealed the benefits of using MT outputs in facilitating accurate L2 writing. Notably, however, the error corrections in the students’ revised texts do not necessarily lead to significant improvements in subsequent L2 writing or language learning (Truscott, Reference Truscott2009). In particular, prior studies have argued that covering one linguistic feature may require numerous writing tasks (Bruton, Reference Bruton2009; Sheen, Reference Sheen2007). Hence, rather than an isolated assignment in which students use MT to make corrections and produce a single revised text, teachers should carefully track the students’ L2 writing development throughout the course and use the results of their writing to address specific challenges and limitations of making error corrections.
In conclusion, MT should be regarded as an additional language learning tool that can support students in their efforts to produce accurate L2 writing. MT should not be regarded as a replacement of the traditional language learning classroom, nor should language learners be prohibited from taking advantage of its beneficial features. Language teachers should readily acknowledge its accuracy and existence, especially considering that the quality of its outputs now surpasses the ability of many EFL learners. By strategically incorporating MT into the language learning classroom, its affordances can effectively be leveraged for the benefit and future use of students who, without it, may fail to become engaged in the L2 writing process.
Ethical statement
There are no conflicts of interest in this paper. All participation in the study was voluntary and informed consent was provided prior to commencement of the assignment. The anonymity of the participants was maintained throughout the study.
About the authors
Sangmin-Michelle Lee is a professor of global communication at Kyung Hee University in Korea. She earned her PhD from the Pennsylvania State University in language education. She has published papers on language learning in a technology-enhanced learning environment, machine translation, L2 writing, and digital creativity.
Neil Briggs earned his PhD at Kyung Hee University in Korea. He spent the past decade working in a variety of tertiary institutes in Korea. He has published papers on a variety of topics such as digital learning technologies, machine translation, autonomous learning, and assessment.
Author ORCIDs
Sangmin-Michelle Lee, http://orcid.org/0000-0002-7686-3537
Neil Briggs, http://orcid.org/0000-0002-7070-2214