Introduction
Research in depression has progressed rapidly over the past four decades (Gotlib and Hammen, Reference Gotlib and Hammen2015; Frodl, Reference Frodl2016), yet depression remains the leading cause of disability (Kessler and Bromet, Reference Kessler and Bromet2013; World Health Organization, 2017), and current treatments are often unsuccessful (Holmes et al., Reference Holmes, Craske and Graybiel2014). This discrepancy has been attributed to breaks in the ‘translational pipeline’ from basic science to clinical practice (Read, Reference Read1992) and from effective treatments to dissemination (Kilbourne et al., Reference Kilbourne, Williams, Bauer and Arean2012). Indeed, low funding for translational efforts (Insel, Reference Insel2012), few instances of basic results yielding successful phase 2 trials (Insel, Reference Insel2012), and problems with dissemination in clinical practice (Weersing et al., Reference Weersing, Rozenman and Gonzalez2009; Eisenberg and Druss, Reference Eisenberg and Druss2015) are well known. One possible cause of these breaks may be suboptimal communication across the domains of stakeholders (henceforth, we will refer to ‘stakeholders’ as individuals with shared concerns; these concerns will be referred to as ‘domains’) necessary for translation including researchers, patients, providers, and industry. Here, we consider mental health subdisciplines (e.g. neuroscience, psychology) to form networks connected by pipelines that have the potential to break (Holmes et al., Reference Holmes, Craske and Graybiel2014), and map these networks using graph theory. To reveal these networks, we examined depression-relevant terms in two linguistic corpora (Google News and academic papers in Web of Science). Google News was used to capture how the public understands depression. The Web of Science corpus was analyzed by disciplines, to examine whether there are some academic disciplines that might aid translation but are poorly included in the translational pipeline. If disciplines are not talking about depression in the same ways or referencing the same literature, their work may not be integrated, preventing unified insights for the wider community. Terms cited in the Web of Science publications were also analyzed to indicate how academics understand depression. The goal was to see whether the same stakeholder domains emerge from public (Google News) and academic sources, establishing convergence on the nature of likely breaks in the translational pipeline. We asked the following five questions to identify ‘breaks’ in the pipelines between stakeholder domains in order to generate ideas for how to fix them.
Q1) What is the overall structure of the depression knowledge network(s)?
We considered that pipelines could be broken if terms associated with depression were used in isolation, and did not form a coherent body of research/discussion. We therefore used graph theory to examine whether terms and citations were generally used similarly or in context with each other (henceforth, ‘connected’), making a single network (‘giant component’ in graph theory terminology). We also considered two ways in which terms and citations, if generally connected, were organized to naturally promote or inhibit inter-stakeholder communication. A first translation-promoting organization would be if terms were organized in ‘small worlds’, consisting of small groups of interconnected terms, and for which key terms or ‘hubs’ in the small groups were connected across domains, yielding an organization in which there are a small number of connections, and hence easy translations, from any term to any other term (Humphries and Gurney, Reference Humphries and Gurney2008). The second would be if terms formed a well-connected, simple structure that again, allowed easy traversal across the network (low ‘fractal dimensionality’). The alternative is that the domain is more fragmented, suggesting fundamental difficulties in inter-stakeholder communication, or complex, yielding difficulty figuring out how to improve communication because the paths between domains are not short or obvious.
Q2) Are there identifiable stakeholder domains, and how well connected are they?
A second way the pipeline could be ‘broken’ is that groups of terms or citations are specific to stakeholder domains, suggesting that groups of stakeholders may not have the same language or concerns. Thus, we examined whether terms and citations clustered in interpretable stakeholder-relevant domains (‘modules’, in graph theory terminology) and whether those domains appeared to use terms similarly, or enough in conjunction with terms from other domains, that stakeholders from one domain could relate to writings about another.
Q3) Are there specific terms which are important to stakeholders in one domain but are not connected to other domains?
A third way the pipeline could be broken is that domain-critical terms are not well connected; that is, the way terms in one domain are used share little conceptually with how terms are used in another, yielding potentially disparate literatures – proverbial blind men feeling different parts of an elephant. We considered whether terms or citations critical to a module have few connections to the remaining network, decreasing chances that stakeholders that care about one domain would care about advances in another.
Q4) Are there hints, from network structure, how to improve communication between stakeholder knowledge domains?
We examined whether there were critical terms within networks that could be considered ‘targets’ for maximally impactive cross-domain translational efforts.
Q5) Are there depression relevant terms that are not typically considered?
Increasingly depression is recognized as being broader than academic/industry definitions (Daughtry and Kunkel, Reference Daughtry and Kunkel1993). For example, cognitive and functional deficits last after depression severity scores have nominally remitted (Nebes et al., Reference Nebes, Pollock, Houck, Butters, Mulsant, Zmuda and Reynolds2003; Kennedy et al., Reference Kennedy, Foy, Sherazi, McDonough and McKeon2007). This has led some to think that what industry and academia consider to be depression is not consistent with how the phenomenon is viewed by patients (Angst and Merikangas, Reference Angst and Merikangas1997), which would represent a clear break in the translational pipeline. The Google News corpus allows an initial test of whether there are other terms which the public discusses in the context of depression (terms rated by Google as ‘similar’ to the term depression) that may be important to recognize as integral to depression.
Search 1. Google News
Methods
Initial seed terms
Terms relevant to depression were obtained by searching the corpus for terms that were similar to both ‘depression’ and a few other fragments used similarly in depression (‘depress’, ‘anhedon’, ‘dysthym’) and mental health treatment (‘mental_’, ‘psychol’, ‘psychia’, ‘therapy’, ‘patient’) (GS and AC generated this list). Irrelevant terms such as ‘fundamentally’ (contains ‘mental’) were removed (full term list is available in online Supplementary 1a).
Corpus, word similarity weight assignment, and network parameters
The Google News corpus included ~1 billion words, with 200 000 words and phrases in common dictionaries and coded for content (https://code.google.com/archive/p/word2vec/#Pre-trained_word_and_phrase_vectors). Online Supplementary 1b describes our network parameters in reproducible detail. Briefly, we used an algorithm that derived features of words based on their co-occurrences (Mikolov et al., Reference Mikolov, Chen, Corrado and Dean2013), and derived their similarities based on how closely these features aligned. We considered highly similar terms to be ‘connected’ (similarity scores>mean similarity in the network − 2 s.d.; see online Supplementary 7 for advantages and disadvantages of considering terms as either connected or not, rather than using continuous values). To answer Questions 1 (network structure) and 2 (domain identification), we used industry standard network software (van Eck and Waltman, Reference van Eck and Waltman2010) (http://www.vosviewer.com) to view the networks and derive ‘modules’ or clusters of well-connected terms. To quantify our answers to Question 1, we calculated standard graph theory parameters to describe the complexity of the network, including ‘small-worldness’ representing whether arbitrary terms are linked through a small number of connections (Humphries and Gurney, Reference Humphries and Gurney2008) via (Konganti et al., Reference Konganti, Wang, Yang and Cai2013), and ‘Hausdorff dimensionality’ (Hausdorff, Reference Hausdorff2001) a measure of fractal dimensionality to describe the internal complexity of the network; low numbers reflect a ‘simple’ organization without extraordinary differences in traversing between close v. far terms whereas high numbers could suggest a ‘complex’ organization in which getting from one place in the network to another conceptually could be an arduous or unlikely journey.
To answer Questions 3 (poorly connected terms) and 4 (opportunities for better communication), we calculated a number of standard network indices (Konganti et al., Reference Konganti, Wang, Yang and Cai2013) addressing whether terms are central to a domain, including ‘degree centrality’ – the number of connections a term has to other terms ‘clustering coefficient’ – how much a term is part of a small group of tightly connected terms that form a ‘clique’ (may represent a domain used by a small group of people that is not well connected to the rest of the depression world), and ‘local average connectivity’ – how well, on average, the terms to which a term is connected are, themselves, connected to the network (average degree centrality of a term's connections). This metric represents how important a term's neighborhood is to the network. For Question 3, we suggest that terms low (mean − 1 s.d.) on all of these indices are especially likely breaks in the translational pipeline (henceforth ‘orphans’) and terms low on any of these indices are ‘at risk’ for becoming orphans, i.e. translational breaks. We considered terms connected only within their own module to be at risk for being ‘lost in translation’ between domains and operationalized this quantity as the ratio of between-module to between + within module connections.
To answer Question 4 regarding what terms might help rescue the translational pipeline, we searched for terms that communicated between modules. We calculated ‘betweenness centrality’, an index of the term's influence over the network. Terms that were high (mean + 1 s.d.) on any property and had at least the mean number of connections were considered ‘influential’ and worthy of consideration for improving communication across the translational pipeline. The ‘brokering coefficient’ reflects the extent to which a term connects terms not otherwise connected in the network (Cai et al., Reference Cai, Borenstein and Petrov2010). Items were ‘brokering influential’ if they were ‘influential’ and also high (mean + 1 s.d.) in brokering. Terms with high numbers of between-module connections were considered potential targets for remediating communication between stakeholder domains [hence ‘communicative’ or ‘bridge’ terms (Cramer et al., Reference Cramer, Waldorp, van der Maas and Borsboom2010)].
To address Question 5 (terms relevant to depression), we examined words strongly associated with depression in the corpus regardless of whether they met our criteria.
Results
Q1) What is the structure of the depression network(s)?
There was one giant component connecting most (75/108) terms with 247 connections (mean of 6.59 per term) (Fig. 1). The network appeared to have small-world properties [small-world-ness index = 3.22, exceeding the traditional cutoff of 3.0 (Humphries and Gurney, Reference Humphries and Gurney2008)] and a simple structure (Hausdorff dimensionality, 1.29), suggesting that overall network properties were not responsible for translational gaps. Most terms had fewer than 10 connections, suggesting fairly sparse connectivity across the network [M(s.d.) degree centrality = 4.63(5.11); 25th percentile = 0.50; 75th percentile = 7.00; sorted histogram is shown in online Supplementary Fig. S3-2].

Fig. 1. Google News corpus search. Similarity network of all searched terms with layout and clustering obtained using VOSviewer. Shaded boxes show terms with strongest between-module connection frequencies (communicating terms; Z between module connections) along with the mean and standard deviation of the ‘lost in translation’ index (ratio of the number of between to within + between connections) for all terms as a function of module (figures with all terms and term names are shown in online Supplementary Figs S3-1 and S3-2).
Q2) Are there identifiable stake-holder domains?
We detected three modules: (1) antidepressants and disorders, (2) patients and providers, and (3) academic disciplines and psychotherapies (Fig. 1; replicated with an alternative clustering approach in online Supplementary 2). Links between modules were sparse with the ratio of between- to within + between module connections consistently below 0.1 (Lost in Translation indices in the gray boxes in the figure; indices for each term are shown in online Supplementary Fig. S3-1). Terms in the patients and providers module had links to the academics and psychotherapies module through provider (‘psychiatrist’) and general mental health (‘mental illness’) terms. Terms in the academic domain had links to ‘patients and providers’ but almost none to ‘antidepressants and disorders’.
Q3) Are there specific terms that are important to stakeholders in one domain but not connected to other domains?
Multiple terms had no strong connections to any term in the giant component (online Supplementary Fig. S3-1; Supplementary S3-1 and S3-2). ‘Orphan’ terms included ‘hypnotherapy’, ‘neuroses/neurosis’, ‘occupational therapy’, ‘psychiatric hospital’, and ‘psychotic depression’. ‘At risk’ terms included ‘electroconvulsive therapy/electroshock therapy’, ‘neuropsychiatric’, ‘neuropsychological’, and ‘psychotherapy’. Specific therapies were not part of the giant component (e.g. herbal therapy) or had few connections (e.g. behavior therapy). Discipline (e.g. ‘clinical psychology’), disorder (‘unipolar depression’), and medication-related terms were moderately connected. Best-connected terms included broad labels such as ‘psychological’ and ‘psychiatric’.
Q4) Are there hints, from network structure, how to improve communication between stakeholder domains?
Multiple terms within the giant network appeared ‘influential’, i.e. could be used in documents intended for multiple stakeholder groups (high bridging centrality; online Supplementary Table S3-1). ‘Depression’ had high scores on both centrality measures. ‘Developmental psychology’, ‘dysthymia’, ‘mental disorders’, ‘psychiatric’, ‘psychiatry’, and ‘tricyclic antidepressants’ also qualified, and none were ‘at risk’. No terms were ‘brokering influential’, i.e. likely to rescue the ‘orphan’ terms. Some terms did have high between-stake-holder connections (Fig. 1, gray boxes), yielding potential targets for improving translational work. Online Supplementary 4 reports network statistics on additional a priori terms.
Q5) Are there depression relevant terms that are not typically considered?
Table 1 shows the 50 words most strongly associated with depression in Google News and their level of similarity. Depression shared most features with ‘bipolar disorder’ and ‘mental illness’. Additional words included symptoms often discounted (‘insomnia’) or rarely discussed for depression (‘hypochondriasis’) and not diagnostic features (‘migraines’) or rarely diagnostic (‘premenstrual syndrome’).
Table 1. Most similar words to depression. Specific words considered in the discussion are highlighted
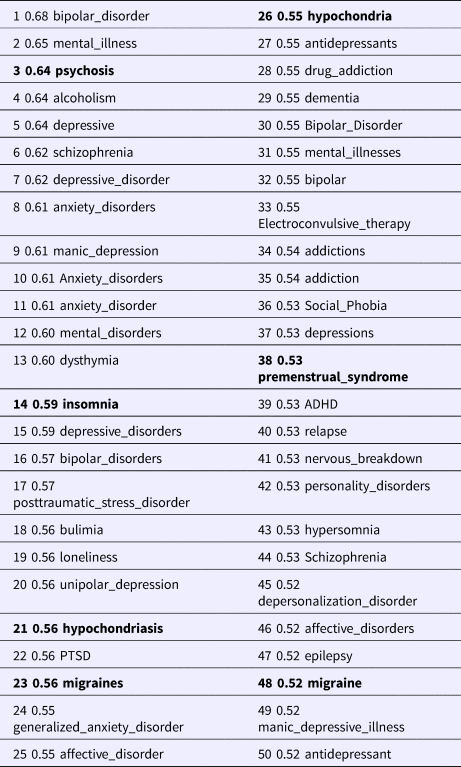
Discussion
Rather than a ‘translational pipeline’ from theory to clinical practice network analyses suggest a multidimensional translational network associated with depression, suggesting multi-pronged, interdisciplinary approaches may be useful in addressing observed ‘breaks’. Within that network there were separate domains associated with disorders/pharmaceuticals, patients and providers, and academic pursuits as shown by the detection of three modules and few terms that connected them. This is similar to findings by Duan et al. (Reference Duan, Wu and Long2015), in which research foci for disorder-labels clustered differently from work on biological variables. If these separations reflect clinical reality, it could suggest that patients, special interests (drug companies and insurance companies), and academics, have different interests and appear in different linguistic contexts. Efforts aimed at integration may involve academic authors writing summaries of results for patients and providers, disseminated through popular media. Using terms that bridge modules (Fig. 1, gray boxes) may be helpful.
The notion of a broken ‘translational pathway’ from neuroscience to clinical trials was not supported, as neuroscience seems as connected as other terms. However, there is support for breakdowns in getting academically or industry derived interventions discussed in manners or contexts that are accessible to patients. Developmental psychology and psychiatry are candidates to bridge these worlds. It may be helpful to integrate terms considered distant from the mainstream of depression (e.g. ‘hypnotherapy’ and ‘aromatherapy’) with the same communication venues as used by the detected modules, e.g. publishing in peer reviewed journals for academic integration or formally examining associations of pharmaceutical interventions with alternative treatments for integration with special interests.
The purview of depression may be broader than typically recognized. Results from Q5 analyses support examining associations between depression and rarely considered features (e.g. migraines and insomnia), and integrating such features more formally in canonical considerations for depression. For example, often neglected diagnostic features (e.g. insomnia) may be particularly important. Similarly, migraines command enough literature from popular sources, epidemiological findings, and mechanistic models (Lantéri-Minet et al., Reference Lantéri-Minet, Radat, Chautard and Lucas2005; Llesuy, Reference Llesuy and Kanner2012; Jancin, Reference Jancin2013; Baksa et al., Reference Baksa, Gonda and Juhasz2017; Zarcone and Corbetta, Reference Zarcone and Corbetta2017; Breslau et al., Reference Breslau, Lipton, Stewart, Schultz and Welch2003), to suggest utility of a stronger clinical focus, academic projects, and pharmaceutical targets.
Search 2. Web of Science
Methods
Terms
We chose 71 academic disciplines from the Web of Science (webofscience.com) (online Supplementary Table S5-1) to represent ‘Mental Health Science’. We chose the following search terms to capture depression: ‘anhedonia’, ‘sad mood’, ‘dysthymia’, ‘dysthymic’, ‘depression’, ‘depressed’, ‘depressive’, and ‘mood disorder’. We applied filtering (online Supplementary Table S5-2) to capture only articles that had depression as a mental health problem. With filtering, searching for depression terms in abstracts and titles of Web of Science publications of Mental Health Science journals yielded ~120 000 publications between 2007 and 2016. We extracted noun phrases using natural language processing techniques (Van Eck and Waltman, Reference Van Eck and Waltman2011).
Computation of network indices
We used citations to compute connection strengths between subdisciplines, representing how often subdisciplines cited one another. Connection strengths for noun phrases were based on their rate of co-occurrence within the titles and abstracts of selected publications. We used software and procedures described in the methods for Search 1 and online Supplementary 1b to visualize the network, derive sub-domains (‘modules’), and compute relevant network indices.
Results for discipline-wise search
Q1) What is the structure of the disciplines?
The search revealed depression-related publications in all 71 disciplines, from 57 publications in philosophy to 72 200 in psychiatry. The domain structure had 2043 ‘strong’ connections (mean of 28 per discipline). The network was characterized by one giant component with hubs for psychiatry, neurosciences, clinical neurology, pharmacology, psychology – multidisciplinary, and medicine (Fig. 2). The network did not have small-world properties [small-worldness index = 1.01 which is below the traditional cutoff of 3.0 (Humphries and Gurney, Reference Humphries and Gurney2008)] and was moderately complex (Hausdorff dimensionality = 1.59). Thus, at least among academic publications, the hypothesis of fundamental obstacles to translation in the network structure could be supported. There were many well-connected disciplines [M(s.d.) degree centrality = 28.76(18.31); 25th percentile = 12.50; 75th percentile = 42.75; linear increase in degree centrality shown in online Supplementary Fig. S6-2], suggesting there are avenues for communication.

Fig. 2. Subdiscipline citation search. Network of subdisciplines with layout and clustering obtained using VOSviewer. Shaded boxes show terms with strongest between-module connection frequencies (communicating disciplines; Z between module connections) along with the mean and standard deviation of the ‘lost in translation’ index (ratio of the number of between to within + between connections) for all terms as a function of module (figures with all citation names are shown in online Supplementary Figs S6-1 and S6-2).
Q2) Are there identifiable stake-holder domains?
The network had three distinct modules we have labeled (1) psychological disciplines (developmental, social, and clinical), (2) biological disciplines (e.g. neurosciences, clinical neurology, and psychiatry), and (3) general medicine (e.g. anesthesiology and obstetrics-gynecology). Disciplines were moderately connected across modules with the ratio of between- to within + between module connections consistently in the range of 0.2–0.5 (Lost in Translation indices in the gray boxes in the figure; disciplines are listed in online Supplementary Fig. S6-1).
Q3) Are there specific disciplines which are important to stakeholders in one domain but are not connected to other domains?
Results suggested that there were breaks in communication between domains. The most central disciplines were psychiatry and clinical psychology (Fig. 2). Disciplines such as religion, anthropology, social issues, and ethnic studies were not central to the network and were poorly connected even within their own modules (online Supplementary Figs S6-1 and S6-2). Biological aspects of psychology were particularly poorly connected to other modules. The humanities and multiple psychology subdisciplines had at least one low connectivity index, putting them ‘at risk’ for poorly interfacing with the rest of the network (online Supplementary 3-1).
Q4) Are there hints of how to improve communication between stakeholder domains?
Disciplines associated with development were well connected between the general medical and psychology modules (shaded boxes in Fig. 2 showing the top five terms linking between modules). Disciplines associated with neuroscience and neurology had strong connections with the medical and biological modules. Because the ‘at risk’ disciplines from the previous analyses were from the most disparate parts of the network, their connections had ‘influence’ over bringing disciplines outside the usual depression disciplines into the network.
Discussion of the discipline-wise search
We examined the extent to which the gap between science and practice is associated with the level of integration in how depression is considered in research. A wide range of subdisciplines formed a complex network without clearly communicating sub-domains, suggesting possible systemic difficulties in translation. A schism between psychology and psychiatry/neuroscience was borne out empirically. Depression in the context of general medicine derived from a separate literature than psychological or psychiatric/neuroscience literature. The lack of contact between all aspects of humanities and the rest of the network could mean their insights are not well integrated into the depression literature. Alternately, as these disciplines often publish outside the sphere of Web of Science, results could reflect sampling bias. That said, the analysis of Q4 suggested that there were intuitive ways for disciplines in one sub-domain to make contact with disciplines in another. For example, clinical and developmental/pediatric issues are candidates for psychology to make strong contact with both the general medical and biological literatures.
Results for noun phrase search
Q1) What is the structure of the domain?
The domain structure consisted of 2591 noun phrases with 1 605 728 connections (mean of 619 per noun phrase) (Fig. 3; zoomed details are given in online Supplementary 8). The network was characterized by a giant component with hubs for questionnaires, health, trials, efficacy, mechanisms, functions, roles, and behavior. The network did not have small-world properties [small-worldness index = 1.37 which is below the traditional cutoff of 3.0 (Humphries and Gurney, Reference Humphries and Gurney2008)] and was complex (Hausdorff dimensionality = 1.8). Thus, at least among noun phrases in academic publications, the hypothesis of fundamental obstacles to translation in the network structure could be supported.

Fig. 3. Noun phrase co-occurrences. Co-Occurrence network of noun phrases with layout and clustering obtained using VOSviewer. Shaded boxes show noun phrases with strongest between-module connection frequencies (communicating noun phrases; Z between module connections) along with the mean and standard deviation of the ‘lost in translation’ index (ratio of the number of between to within + between connections) for all terms as a function of module (figures with all citations are shown in online Supplementary Figs S8-2 and S8-3).
Q2) Are there distinct sub-domains?
Three detected modules resembled those of the Google News search: patients and providers (‘mother’, ‘child’, ‘care’, ‘health’), industry considerations (‘trial’, ‘efficacy’, ‘effectiveness’, ‘antidepressant’, ‘drug’), and academic considerations (‘mechanism’, ‘receptor’, ‘brain’, ‘cortisol’, ‘exposure’).
Q3) Are there specific noun phrases that are important to stakeholders in one domain but are not connected to other domains?
Results suggested there were breaks in communication between domains. Some noun phrases appeared in the context of nearly every other noun phrase and some appeared rarely with others (Fig. 3) yielding a relatively well connected network [M(s.d.) degree centrality = 581.08(435.93); 25th percentile = 286.25; 75th percentile = 719.75; sorted histogram is shown in online Supplementary Fig. S8-3]. Connectivity between modules varied considerably with the ratio of between module to between + within module connections from the patients and providers, and academic disciplines network being in the range of 0.1–0.2 and connections from the industry module being in the range of 0.4–0.45 (Lost in Translation indices in the gray boxes in Fig. 3; all connections are shown in online Supplementary Fig. S8-2). Table 2 highlights the most and least strongly connected terms. Weakly connected terms often involved a specific type of stimulus (e.g. ‘elevated plus maze test’), specific populations (e.g. ‘Turkish version’), or technical language (‘sequenced treatment alternatives’). The best-connected terms were common words expected across various contexts (‘activity’, ‘function’) along with terms intuitively relevant to depression (‘drug’, ‘mental health’) and central to depression (‘prevalence’, ‘efficacy’).
Table 2. Least and most well-connected noun phrases

Q4) Are there hints of how to improve communication between stakeholder domains?
The shaded boxes in Fig. 3 show the top 10 noun phrases that connect one module to another.
Discussion of noun-phrase-wise search
The noun-phrase domain structure replicated the Google News domain structure, suggesting that the lay press and academic literature may exhibit similar understandings of depression and its sub-domains, with gaps between academic, patient/provider, and special interest communities. The complex non-small-world structure of the network could suggest that the sheer breadth and disconnected nature of how depression is discussed could present obstacles to translation. That being said, candidate mechanisms were observed; the presence of so many terms outside the network that seem specialized to small audiences (‘dna methylation’, ‘Cochrane central register’) could speak against the use of jargon in trying to communicate about depression in a way that maximizes translational potential. As many noun phrases had few connections, it could suggest that much of the information in the depression literature is not well integrated. Candidates for maximally improving communication across modules are generic terms (‘stress’, ‘mechanism’, and ‘behavior’) by academics whereas more specific targets were present for other domains. ‘Efficacy’ allows pharmaceutical considerations to be accessed by academia and patients/providers. Patients/providers’ topics most easily accessed by academics include ‘child’, ‘adolescent’, ‘health’, and ‘prevalence’.
General discussion
We examined the extent to which the gap between science and practice is associated with the level of integration in how depression is considered in research and stakeholder-relevant documents. There were separable groups or modules of information that appear together but do not strongly reference each other (Fig. 4). In both Google News and academic searches, depression-relevant terms fell into categories associated with patients/providers, academics, and special interests (pharmaceuticals and insurance). The academic disciplines formed a single loosely connected network with the humanities removed from the rest of the depression literature and with separations between psychology, biology/neuroscience, and medicine.

Fig. 4. Conceptual domain and discipline-wise integration of primary results across the three searches.
Analyses of network structure and complexity suggest that the lay literature may conform to a simple, small-world structure amenable to translational discussion, whereas academia is complex and does not conform to a small-world organization, suggesting that there may be systemic obstacles to translational communication. Whether this needs changing is unclear; it could suggest the utility of a change in how academics speak, emphasizing connections of specific academic work to the larger depression literature. The lay/academic distinction could also suggest that the lay literature does not capture the complexity of the academic literature, again suggesting that stronger translation from academia could be useful.
The most noticeable absence of connections, or ‘fractures’, occurred along lines associated with canonical translational pipelines from academics to industry to community implementations. Previous guidelines from other disciplines for understanding translational pipeline problems (Krieger, Reference Krieger2011; Morris et al., Reference Morris, Wooding and Grant2011; Institute of Medicine and Board on the Health of Select Populations, 2013; Williams, Reference Williams2015) and for facilitating translation along this pipeline (Tsuji and Tsutani, Reference Tsuji and Tsutani2008; Alving et al., Reference Alving, Dai and Chan2012; Oborn, Reference Oborn2012; Wehling, Reference Wehling2015) may help address these issues. In addition, successful instances of translation from research to clinic (e.g. the Veterans Affairs’ dissemination of evidence-based psychotherapies), can illustrate concrete steps in implementing a multilevel, collaborative approach (Karlin and Cross, Reference Karlin and Cross2014).
Explanations and possible remedies for terminological and disciplinary fragmentation
There are multiple explanations for possible fractures between domains, which would suggest different approaches to increasing translation.
Language differences
Fractures may be because lay and academic communities do not use the same terminology or are not phrasing their work to be accessible to other communities. For example, academics or pharmaceuticals might write about treatments as odds ratios above and beyond placebo controls, whereas a patient might express their concerns as chances they get better. Similarly, interventions may not be phrased for patients or providers in a way that makes their connections with the broader network apparent. Themes popular in cognitive neuroscience involve decreasing reactivity v. increasing the ability to control reactions. Phrased to a patient as ‘would you like us to help you to control reactions when you have them?’ might encourage patients to make contact with the scientific rationale. To formally address language differences, if disciplines ‘seed’ their work with key terms that link to other disciplines, it could lead to strategic taking-notice by intended stakeholders (see shaded boxes in Figs 1–3 for example). Computational graph theoretic methods of viral marketing could be useful in this regard (Domingos and Richardson, Reference Domingos and Richardson2001; Kempe et al., Reference Kempe, Kleinberg and Tardos2003). It may also be helpful to explicitly try to use more domain general language or language adopted by other disciplines so they can relate.
Content differences
If stakeholder goals do not match across domains, progress in one domain (e.g. neuroscience) may not match the concerns of stakeholders in another domain (e.g. patients). Potential obstacles to interdisciplinary goal alignment may be revealed by disciplines not strongly connected in the network analysis, e.g. ethnic studies and anthropology highlight the role of ethnicity (Torres, Reference Torres2010) and ethnic identity (Ai et al., Reference Ai, Nicdao, Appel and Lee2015) in depression. These perspectives (Kleinman and Good, Reference Kleinman and Good1985) can be integrated with psychological perspectives (Hagmayer and Engelmann, Reference Hagmayer and Engelmann2014). Psychology relies on group statistics whereas anthropology tends toward idiographic qualitative analyses, and cognitive neuroscience applies reductionist simplifications in computational models. Such tendencies may hinder cross-disciplinary and cross-stakeholder adoption.
To address content differences, work that targets commonalities and shared interests across fields could be useful. For example, bringing geneticists together with life stress researchers explicated the way nature interacts with nurture (Caspi et al., Reference Caspi, Sugden, Moffitt, Taylor, Craig, Harrington, McClay, Mill, Martin, Braithwaite and Poulton2003), and a collaboration between an evolutionary biologist and psychiatrist resulted in the notion that depression is an evolved adaptation that works through rumination to resolve complex social problems (Andrews and Thomson, Reference Andrews and Thomson2009). One example of a shared topic of interest is side effects. Online Supplementary 9 shows different associations with ‘side effect’. Primary industry associations are with ‘agents’, ‘effectiveness’, ‘remission’, and specific drug type, whereas patient/provider associations are with ‘adherence’, ‘care’, and ‘child’, and academics with ‘concentration’, ‘target’, ‘mechanism’, and ‘brain’. Academic books may further increase translational value by inviting contributions from patient or industry perspectives. If pharmaceutical companies primarily communicate through advertising, academics through journals, and patients through newsletters and blogs, insights from one domain might not translate to another. It could be helpful for centralized bodies to solicit and act on information across domains, disciplines, and stakeholders. For example, Japan allows both academics and patients to petition for new drug approvals (Tsuji and Tsutani, Reference Tsuji and Tsutani2008).
Knowledge differences
People have varying levels of familiarity with different disciplines. Academia is filled with domain experts, special interests hire for expertise in one area, and providers have little incentive to learn about treatments other than those in which they are trained. Higher education may promote narrow disciplinary dispositions among students. However, ‘the scale and complexity of today's biomedical research problems increasingly demand that scientists move beyond the confines of their own discipline and explore new organizational models of team science’ (Zerhouni, Reference Zerhouni2003: p. 64; Casadevall and Fang, Reference Casadevall and Fang2014).
If differences reflect unfamiliarity across disciplines, increasing interdisciplinary and inter-community collaboration could help. It also may be useful to encourage trainees to learn more about other disciplines, e.g. to become part of wider health care teams (Lemieux-Charles and McGuire, Reference Lemieux-Charles and McGuire2006). Increased interdisciplinary education in mental health sciences (encompassing behavioral, neuroscience, clinical, and other approaches) (Holmes et al., Reference Holmes, Craske and Graybiel2014) could facilitate this path, especially by encompassing training in both how to collaborate across disciplines and specializations in mental health science itself, such as societal mental health science that includes disciplines such as clinical psychology, epidemiology, social work and health economics or individual interventions in mental health science including clinical psychology, psychiatry, pharmacology, computer science, and neuroscience.
Funding specialization
Fractures may occur because there is little financial incentive for integrating across gaps. Funding agencies play a crucial role in realizing interdisciplinary research (To thwart disease, apply now, 2008; Lyall et al., Reference Lyall, Bruce, Marsden and Meagher2013). Funding agencies could incentivize interdisciplinarity by dedicated interdisciplinary programs or financing of interdisciplinary proposals. NIH funding is highly weighted toward biological approaches, with little incentive to combine with even psychosocial approaches. Conflict of interest regulations have made the prospect of collaborations between academics and industry scary, and patient groups have limited funds.
A potential way to address funding specialization is the creation of funding mechanisms that require or encourage involvement of multiple stakeholders, e.g. necessitating patient/provider buy-in and industry commitments before basic science is undertaken. There are hints of such programs. NIH Conte Centers require integration of animal, basic human, and clinical trials research, but do not make contact with patients/providers or industry. NIH SBIRs support collaborations between industry and academia, but rarely involve large industry (e.g. major pharmaceuticals or insurance agencies). In Europe, the Framework Programmes encourage integrated interdisciplinary problem-oriented approaches. In the Netherlands, funding for groups that involve both academics and patients and cross-disciplinary projects (ZON-MW) is prioritized, but the review process is conducted within disciplines. The Dutch National Research Agenda that integrates priorities across science, industry, and the public also strongly advocates collaborative research on health issues and collaboration of academia with industry.
Limitations
The current study has limitations. As a computational (linguistic) analysis it involved no actual people, and thus cannot be considered descriptive of human phenomena except for by remote proxies. The terms were subjectively, not exhaustively generated. We have proposed a model, but have not empirically tested whether the breaks in translation we have derived from graph theory actually cause research to not make it from the lab to the clinic. Failure of translation is a notorious problem across disciplines, and the primary problems may be more generic than ones highlighted here.
Summary and moving forward
This paper has identified gaps in lay and academic literatures associated with depression, suggested mechanisms that might drive these gaps and proposed potential solutions. The authors represent an interdisciplinary group that worked together to identify why depression research does not translate to the clinic. We intend to take a number of steps including (1) crowdsourcing goals for projects of multiple constituencies and disciplines and (2) contacting funding agencies to promote supporting interdisciplinary research. We believe the way forward is together and that we can address the gap between lab and clinic.
Supplementary material
The supplementary material for this article can be found at https://doi.org/10.1017/S003329171800363X.
Author ORCIDs
Greg J Siegle, Marlene Strege 0000-0002-1420-8975, 0000-0002-4105-7279.
Acknowledgements
The authors thank the Netherlands Institute for Advanced Studies (NIAS) for supporting our joint work on this manuscript.
Financial support
This work was supported by the Netherlands Institute for Advanced Studies.
Conflict of interest
GJS is the unpaid chair of the scientific advisory board for Apollo Neuroscience, Inc., a company devoted to commercializing insights from academic studies.