Introduction
Autism spectrum disorder (ASD) and schizophrenia are debilitating neurodevelopmental disorders characterized by wide-ranging impairments in cognition (Cohen and Volkmar, Reference Cohen and Volkmar1997; Rapoport et al., Reference Rapoport, Addington and Frangou2005). Although extensive similarities in cognitive profiles have been reported in both disorders (Couture et al., Reference Couture, Penn, Losh, Adolphs, Hurley and Piven2010; Eack et al., Reference Eack, Bahorik, McKnight, Hogarty, Greenwald, Newhill, Phillips, Keshavan and Minshew2013a), most studies have investigated social cognition rather than nonsocial cognition (Fett et al., Reference Fett, Shergill and Krabbendam2015). Given that nonsocial cognition is an important predictor of functioning for both disorders (Fett et al., Reference Fett, Viechtbauer, Dominguez, Penn, van Os and Krabbendam2011; Kraper et al., Reference Kraper, Kenworthy, Popal, Martin and Wallace2017), evaluating nonsocial cognition across ASD and schizophrenia is critical for adapting common and unique therapeutic strategies to improve functioning across the autism-schizophrenia spectrum.
Treatment comparisons across ASD and schizophrenia have been constrained by a lack of validated cognitive batteries for evaluating treatment outcomes in both disorders. On one hand, a standard cognitive battery for ASD adults has yet to be established, to our knowledge, despite emerging evidence that cognitive remediation treatments improve general cognition not only in schizophrenia (d = 0.45) (Wykes et al., Reference Wykes, Huddy, Cellard, McGurk and Czobor2011) but also in ASD (d = 1.40) (Eack et al., Reference Eack, Greenwald, Hogarty, Bahorik, Litschge, Mazefsky and Minshew2013b). ASD adults show substantial variability in performance on neuropsychological batteries, with performance ranging from normative levels to marked impairments on par with deficits observed in schizophrenia (Mesholam-Gately et al., Reference Mesholam-Gately, Giuliano, Goff, Faraone and Seidman2009). Numerous investigations indicate that, compared to normal controls, ASD adults demonstrate poorer performance in processing speed (David et al., Reference David, Gawronski, Santos, Huff, Lehnhardt, Newen and Vogeley2008; Holdnack et al., Reference Holdnack, Goldstein and Drozdick2011; Fried et al., Reference Fried, Joshi, Bhide, Pope, Galdo, Koster, Chan, Faraone and Biederman2016), attention/vigilance (Ambery et al., Reference Ambery, Russell, Perry, Morris and Murphy2006; Fried et al., Reference Fried, Joshi, Bhide, Pope, Galdo, Koster, Chan, Faraone and Biederman2016), verbal fluency (Ambery et al., Reference Ambery, Russell, Perry, Morris and Murphy2006; Bramham et al., Reference Bramham, Ambery, Young, Morris, Russell, Xenitidis, Asherson and Murphy2009), working memory (Holdnack et al., Reference Holdnack, Goldstein and Drozdick2011; Fried et al., Reference Fried, Joshi, Bhide, Pope, Galdo, Koster, Chan, Faraone and Biederman2016), verbal learning/memory (Sumiyoshi et al., Reference Sumiyoshi, Kawakubo, Suga, Sumiyoshi and Kasai2011), visual learning/memory (Ambery et al., Reference Ambery, Russell, Perry, Morris and Murphy2006), and reasoning/problem solving (Ambery et al., Reference Ambery, Russell, Perry, Morris and Murphy2006; Bramham et al., Reference Bramham, Ambery, Young, Morris, Russell, Xenitidis, Asherson and Murphy2009; Altgassen et al., Reference Altgassen, Koban and Kliegel2012; Torralva et al., Reference Torralva, Gleichgerrcht, Roca, Ibanez, Marenco, Rattazzi and Manes2013; Wilson et al., Reference Wilson, Happe, Wheelwright, Ecker, Lombardo, Johnston, Daly, Murphy, Spain, Lai, Chakrabarti, Sauter, Consortium, Baron-Cohen and Murphy2014; Fried et al., Reference Fried, Joshi, Bhide, Pope, Galdo, Koster, Chan, Faraone and Biederman2016; Otsuka et al., Reference Otsuka, Uono, Yoshimura, Zhao and Toichi2017), consistent with leading theories of ASD as a disorder of complex information processing (Minshew and Goldstein, Reference Minshew and Goldstein1998). On the other hand, the development and widespread implementation of a cognitive battery, the Measurement and Treatment Research to Improve Cognition in Schizophrenia (MATRICS) Consensus Cognitive Battery (MCCB; Nuechterlein et al., Reference Nuechterlein, Green, Kern, Baade, Barch, Cohen, Essock, Fenton, Frese, Gold, Goldberg, Heaton, Keefe, Kraemer, Mesholam-Gately, Seidman, Stover, Weinberger, Young, Zalcman and Marder2008) has enabled standardized treatment evaluation of cognitive outcomes in schizophrenia. With its measures overlapping considerably with domains showing impairments in ASD adults, MCCB encompasses the most salient cognitive performance deficits in schizophrenia and ASD, making it an ideal candidate to assess cognition across both disorders.
As far as we are aware, only one study to date has used the MCCB to compare cognition across ASD and schizophrenia. This study, using an early subset of this sample found no differences in MCCB domain scores (Eack et al., Reference Eack, Bahorik, McKnight, Hogarty, Greenwald, Newhill, Phillips, Keshavan and Minshew2013a). To our knowledge, six other studies have compared cognitive functioning in ASD and schizophrenia using broader neuropsychological batteries, such as the Wechsler Adult Intelligence Scale (WAIS; Wechsler, Reference Wechsler1997). These studies have reported mixed findings across cognitive domains, with ASD generally demonstrating better performance compared to schizophrenia in domains for which ASD and schizophrenia show differences (Bölte et al., Reference Bölte, Rudolf and Poustka2002; Goldstein et al., Reference Goldstein, Minshew, Allen and Seaton2002; Murphy, Reference Murphy2003; de Boer et al., Reference de Boer, Spek and Lobbestael2014; Marinopoulou et al., Reference Marinopoulou, Lugnegård, Hallerbäck, Gillberg and Billstedt2016; Mançe Çalişir et al., Reference Mançe Çalişir, Atbaşoğlu, Devrımcı Özgüven and Ölmez2018). Overall, most studies comparing cognition across ASD and schizophrenia have found some domains to be similarly impaired and some domains where ASD may demonstrate better performance than schizophrenia.
To compare cognitive impairments across ASD and schizophrenia, it is necessary to establish that both groups share common relationships within and among the underlying cognitive domains that are being assessed by the measures in a cognitive battery. In other words, schizophrenia and ASD should respond to the measures' underlying cognitive domains in the same way. This ensures the validity of the measures and of their relationships with other constructs. Where this assumption is not tested, group mean differences in a measure may not reflect actual group differences in the underlying cognitive domain. MCCB's applicability for ASD can established by demonstrating that MCCB performance is attributable to a set of cognitive domains (latent constructs or factors) in the population for which it was initially developed and validated (Nuechterlein et al., Reference Nuechterlein, Green, Kern, Baade, Barch, Cohen, Essock, Fenton, Frese, Gold, Goldberg, Heaton, Keefe, Kraemer, Mesholam-Gately, Seidman, Stover, Weinberger, Young, Zalcman and Marder2008), schizophrenia. Confirming a transdiagnostically stable set of relationships within and among measures and cognitive domains (a factor structure) provides evidence that MCCB is one and the same assessment in schizophrenia and ASD.
To date, three studies have examined the factor structure of the English-language MCCB in schizophrenia using confirmatory factor analysis (CFA) (Burton et al., Reference Burton, Vella, Harvey, Patterson, Heaton and Twamley2013; Harvey et al., Reference Harvey, Raykov, Twamley, Vella, Heaton and Patterson2013; Lo et al., Reference Lo, Szuhany, Kredlow, Wolfe, Mueser and McGurk2016). In contrast to exploratory factor analysis (EFA), which assumes no prior knowledge about relationships among measures and factors, CFA is used to empirically test a hypothesized factor structure and thus imposes a priori constraints on these relationships (Kline, Reference Kline2015). MCCB and measures of functional capacity have been found to load onto a single factor in individuals with schizophrenia or schizoaffective disorder (Harvey et al., Reference Harvey, Raykov, Twamley, Vella, Heaton and Patterson2013). In more heterogeneous samples of individuals with schizophrenia-spectrum disorders, a three-factor model comprising processing speed, attention/working memory, and learning has shown better fit than a one-factor model of general cognition (Burton et al., Reference Burton, Vella, Harvey, Patterson, Heaton and Twamley2013; Lo et al., Reference Lo, Szuhany, Kredlow, Wolfe, Mueser and McGurk2016). Two studies have further reported testing a forced six-factor structure for MCCB (Burton et al., Reference Burton, Vella, Harvey, Patterson, Heaton and Twamley2013) and a Korean-language battery of equivalent measures (Noh et al., Reference Noh, Kim, Hong, Kim, Nam, Lee and Yoon2010); however, this factor structure is highly unstable due to the inclusion of factors that are informed by only one measure. Finally, no models demonstrated adequate fit in CFA conducted based on EFA models for the Norwegian-language MCCB (Mohn et al., Reference Mohn, Lystad, Ueland, Falkum and Rund2017). Overall, these studies suggest that one-factor and three-factor structures may best represent MCCB performance in schizophrenia, although a definitive solution has yet to be replicated.
Whether MCCB can be validly compared across schizophrenia and ASD remains to be examined. More fundamentally, it is still unclear which cognitive domains are assessed by MCCB in ASD, as, to our knowledge, no studies have yet investigated MCCB's factor structure in ASD. This suggests the utility of a multigroup CFA approach, which we describe next, to examine whether MCCB measures the same cognitive domains across schizophrenia and ASD.
Multigroup CFA can establish the extent to which our theories correctly predict how the measures are capturing cognitive abilities similarly, or invariantly, across groups (Byrne et al., Reference Byrne, Shavelson and Muthén1989; Meredith, Reference Meredith1993), by testing the hypothesis that variance between groups is not supported. Establishing the measures’ validity starts with establishing configural invariance (Dimitrov, Reference Dimitrov2010), which suggests that the nature of cognitive abilities as assessed by these cognitive measures is similar across groups. Here, the number of cognitive measures, the number of cognitive abilities (i.e. factors), and which cognitive measures are related to which cognitive abilities can be specified to confirm that this configuration does not differ significantly across groups.
Once configural invariance is established, measurement invariance can also be established, which suggests that the measures reflect their respective cognitive abilities with similar strengths and have similar average scores for each group (Byrne et al., Reference Byrne, Shavelson and Muthén1989; Meredith, Reference Meredith1993; Dimitrov, Reference Dimitrov2010). Here, the correlations between cognitive measures and cognitive abilities (i.e. factor loadings) are confirmed to not differ significantly across groups, and the average scores for cognitive measures for a given level of a cognitive ability are confirmed to not differ significantly across groups.
In addition, structural invariance can be established, which suggests that the cognitive abilities, as assessed by these cognitive measures, have similarly strong relationships to each other and have similar levels of variability across groups (Byrne et al., Reference Byrne, Shavelson and Muthén1989; Meredith, Reference Meredith1993; Dimitrov, Reference Dimitrov2010). Here, the correlations among cognitive abilities are confirmed to not differ significantly across groups, and the degree of heterogeneity in cognitive abilities are also confirmed to not differ significantly across groups.
Thus, drawing upon the multigroup CFA approach in a large, well-characterized sample of adult outpatients with schizophrenia and verbal ASD adults to establish MCCB's validity in both schizophrenia and ASD, the aims of this study are threefold:
(1) Confirm MCCB's transdiagnostic configural invariance by establishing that schizophrenia and ASD show similar patterns of MCCB performance such that the same subsets of MCCB measures inform the same underlying cognitive domains.
(2) Confirm MCCB's transdiagnostic measurement invariance by establishing that schizophrenia and ASD show similar relationships among MCCB measures and their cognitive domains and furthermore show similar average scores for a given domain score.
(3) Confirm MCCB's transdiagnostic structural invariance by establishing that schizophrenia and ASD show similar levels of variability for each cognitive domain and similar relationships among domains.
Methods
Sample
The sample was comprised of English-fluent, community-residing outpatients participating in ongoing studies of Cognitive Enhancement Therapy (Hogarty et al., Reference Hogarty, Flesher, Ulrich, Carter, Greenwald, Pogue-Geile, Kechavan, Cooley, DiBarry, Garrett, Parepally and Zoretich2004; Eack et al., Reference Eack, Greenwald, Hogarty, Bahorik, Litschge, Mazefsky and Minshew2013b). Participants in the schizophrenia group (N = 100; 78 with schizophrenia and 22 with schizoaffective disorder) met the following inclusion criteria: were aged 18 to 60; met criteria for schizophrenia or schizoaffective disorder according to the Structured Clinical Interview for DSM-IV Disorders (First et al., Reference First, Spitzer, Gibbon and Williams1995); were concurrently treated with antipsychotic medication; had an IQ ⩾ 80 estimated using the Quick Test (Ammons and Ammons, Reference Ammons and Ammons1962) or Wechsler Abbreviated Scale of Intelligence-Second Edition, two-subtest version (WASI-II; Wechsler, Reference Wechsler2011); and demonstrated significant cognitive and social disability on the Cognitive Styles and Social Cognition Eligibility Interview (CSSCEI; Hogarty et al., Reference Hogarty, Flesher, Ulrich, Carter, Greenwald, Pogue-Geile, Kechavan, Cooley, DiBarry, Garrett, Parepally and Zoretich2004). Schizophrenia participants were generally in the early course of the illness (illness duration = 3.83 years, s.d. = 2.30) and none were diagnosed with ASD. Participants in the ASD group (N = 113) met the following inclusion criteria: were aged 16 to 45; met criteria for autism or ASD according to the Autism Diagnostic Observation Schedule (Lord et al., Reference Lord, Risi, Lambrecht, Cook, Leventhal, DiLavore, Pickles and Rutter2000) or Autism Diagnostic Interview (Lord et al., Reference Lord, Rutter and Le Couteur1994); had an IQ ⩾ 80 estimated using the WASI-II (Wechsler, Reference Wechsler1999); had not been abusing substances within the past 3 months prior to study enrollment; did not have a history or concurrent diagnosis of psychotic disorder according to clinical records; and demonstrated significant cognitive and social disability on the CSSCEI (Hogarty et al., Reference Hogarty, Flesher, Ulrich, Carter, Greenwald, Pogue-Geile, Kechavan, Cooley, DiBarry, Garrett, Parepally and Zoretich2004).
Measures
MCCB comprises ten tests of seven cognitive abilities (Nuechterlein et al., Reference Nuechterlein, Green, Kern, Baade, Barch, Cohen, Essock, Fenton, Frese, Gold, Goldberg, Heaton, Keefe, Kraemer, Mesholam-Gately, Seidman, Stover, Weinberger, Young, Zalcman and Marder2008): (1) Speed of Processing: Trail Making Test, Part A (TMT); Brief Assessment of Cognition in Schizophrenia, Symbol Coding subtest (BACS Symbol Coding); Category Fluency, Animal Naming (CF); (2) Attention/Vigilance: Continuous Performance Test, Identical Pairs (CPT); (3) Working Memory: Wechsler Memory Scale, Third Edition, Spatial Span subtest (WMS-III Spatial Span); Letter-Number Span test (LNS); (4) Verbal Learning: Hopkins Verbal Learning Test–Revised (HVLT); (5) Visual Learning: Brief Visuospatial Memory Test–Revised (BVMT); (6) Reasoning and Problem Solving: Neuropsychological Assessment Battery, Mazes subtest (NAB Mazes); and (7) Social Cognition: Mayer-Salovey-Caruso Emotional Intelligence Test (MSCEIT), Emotional Management task. Each test demonstrates adequate test-retest reliability and validity, high practicality and tolerability ratings, brief administration times, minimal practice effects, and substantial covariation with functional outcomes (Nuechterlein et al., Reference Nuechterlein, Green, Kern, Baade, Barch, Cohen, Essock, Fenton, Frese, Gold, Goldberg, Heaton, Keefe, Kraemer, Mesholam-Gately, Seidman, Stover, Weinberger, Young, Zalcman and Marder2008). Consistent with previous studies examining MCCB's factor structure (Burton et al., Reference Burton, Vella, Harvey, Patterson, Heaton and Twamley2013; Lo et al., Reference Lo, Szuhany, Kredlow, Wolfe, Mueser and McGurk2016), all measures were included except for MSCEIT, given that this social cognitive measure differs significantly from other nonsocial cognitive measures in the battery.
Procedures
Participants were recruited from support groups, community agencies, community mental health centers, clinics, colleges and universities, online advertisements, prior studies, and local advocacy groups in the Pittsburgh region. Participants underwent diagnostic interview administered by research staff who were trained and supervised by a study psychologist or expert diagnostician. After determining eligibility and prior to commencing treatment, participants were administered the IQ assessment and MCCB by trained psychometrists supervised by a study psychologist. Studies were reviewed and approved annually by the University of Pittsburgh Institutional Review Board, and all participants provided written informed consent to participate.
Analyses
Data were complete for all cognitive measures, and only TMT required transformation due to non-normality: a raw TMT score of 200 in the ASD group was winsorized to the next poorest score in the ASD group (80) whereas TMT scores of 105 and 110 in the schizophrenia group were winsorized to the next poorest score in the schizophrenia group (62). Raw scores for each measure were then standardized to the mean of the total sample via z-score transformation. Skew and kurtosis of each measure within groups were acceptable [absolute skewness ⩽2.0, kurtosis ⩽7.0 (West et al., Reference West, Finch, Curran and Hoyle1995)], suggesting that factor analysis was appropriate.
Standardized scores for each measure were then subjected to multigroup CFA in Mplus, version 8 (Muthén and Muthén, Reference Muthén and Muthén2010) using maximum-likelihood estimation. CFA tests whether the hypothesized relationships among measures can be captured by latent constructs (factors) which cannot be directly measured, as indicated by the fit of a hypothesized factor structure to the observed performance on the measures. CFA of MCCB captures factors assessed by a set of nine measures rather than a comprehensive factor structure of cognition; the resultant factors may differ from but do not contradict conceptualizations of MCCB's nine nonsocial measures as assessments of six nonsocial cognitive abilities.
Multigroup CFA compares the factor structures of two or more groups by estimating models where specific parameters of the factor structures are constrained to equivalence (invariance) across groups (Dimitrov, Reference Dimitrov2010). The fit of nested models with increasingly restrictive constraints is evaluated using the Comparative Fit Index (CFI) comparison. The CFI comparison was chosen over the chi-square difference test given its robustness to model complexity and sample size; a difference in CFI ⩽ 0.01 indicates that constraining the parameters maintains model fit, retaining the null hypothesis of invariance (Cheung and Rensvold, Reference Cheung and Rensvold2002). The following fit indices were used to determine the extent to which each model appropriately captures the data: CFI, Tucker-Lewis Index (TLI), Root Mean Squared Residual of Approximation (RMSEA), and Standardized Root Mean Square Residual (SRMR), with adequate fit indicated when CFI and TLI ⩾ 0.90 and good fit indicated when CFI and TLI ⩾ 0.95, RMSEA ⩽ 0.06, and SRMR ⩽ 0.08 (Hu and Bentler, Reference Hu and Bentler1999).
The transdiagnostic validity of a set of measures can be established by confirming configural, measurement, and structural validity of its factor structure across groups (Dimitrov, Reference Dimitrov2010; Milfont and Fischer, Reference Milfont and Fischer2010; Van de Schoot et al., Reference Van de Schoot, Lugtig and Hox2012). First, configural invariance establishes that the same subsets of measures relate to the same cognitive domains across groups, as the factor structure's configuration is likely to be transdiagnostically equivalent. Next, measurement invariance establishes that the measures can be compared transdiagnostically, as (1) each factor loading, representing the strength and directionality of the relationship between each measure and its respective domain, is likely to be transdiagnostically equivalent, and (2) each intercept, representing the average score of each measure that produces a score of zero in its respective domain, is likely to be transdiagnostically equivalent. Finally, structural invariance establishes that the domains can be compared transdiagnostically, as the variance of each factor, representing the variability in performance on a cognitive domain, and its correlations with other factors, representing its relationships with other domains, are likely to be transdiagnostically equivalent.
Results
Demographic and cognitive characteristics of the sample
As shown in Table 1, schizophrenia and ASD groups both consisted of young adults aged approximately 24 years, were largely male, Caucasian, and had attended college. Across groups, participants were similar in age, sex, race and IQ. A greater proportion of schizophrenia participants than ASD participants had attended college; however, consistent with the substantial deficit in functioning, only 15% of the schizophrenia participants had received a college degree whereas 29% of ASD participants had received a college degree. All schizophrenia participants were taking antipsychotic medication, with an average daily chlorpromazine equivalent dosage of 452 mg (s.d. = 352 mg), and 43% had a history of substance abuse or dependence. The two groups showed T-scores for measures that were largely within overlapping ranges, with schizophrenia showing lower scores than ASD for measures of Processing Speed (CF), Attention/Vigilance (CPT), and Verbal Learning (HVLT), and showing higher scores than ASD for one measure of Attention/Vigilance (WMS-III Spatial Span).
Table 1. Demographic and performance characteristics across groups

BACS Symbol Coding, Brief Assessment of Cognition in Schizophrenia, Symbol Coding subtest; BVMT, Brief Visuospatial Memory Test-Revised; CF, Category Fluency; CPT, Continuous Performance Test – Identical Pairs; HVLT, Hopkins Verbal Learning Test – Revised; LNS, Letter Number Span; NAB Mazes, Neuropsychological Assessment Battery, Mazes subtest; TMT, Trail Making Test, Part A; WMS-III Spatial Span, Wechsler Memory Scale – Third Edition, Spatial Span subtest.
Note. Results of one-way Type III ANOVAs are presented for age, IQ, cognitive domains and standardized cognitive measures, whereas chi-square tests are reported for sex (% male), race (% Caucasian), and education (% attended college).
a For the schizophrenia group, 59% had attended college but did not have a college degree, and 15% of the schizophrenia group had received a college degree. For the autism group, 40% had attended college but did not have a college degree, and 29% had received a college degree.
Cognitive domains and measures are presented as T-scores, which have normative distributions centered around a mean of 50 and standard deviation of 10.
Establishing configural invariance
We began our evaluation of MCCB's transdiagnostic validity by first examining MCCB's factor structure within and across participants with schizophrenia and ASD. This is necessary to ensure that the same factor structure likely captures the configuration of measures and domains transdiagnostically. Invariance test results and fit for all models (M1–M8) are summarized in Table 2.
Table 2. Fit indexes across models of configural, measurement, and structural invariance

First, we replicated MCCB's factor structure in schizophrenia (Fig. 1a; online Supplemental Results and Table S1). The three-factor model with correlated residuals established in schizophrenia (M1) demonstrated excellent fit in ASD (M2), requiring no further modifications. In ASD, this model fit significantly better than the three-factor model without correlated residuals (CFI = 0.948, TLI = 0.921, RMSEA = 0.078, SRMR = 0.054) and the one-factor model (CFI = 0.844, TLI = 0.793, RMSEA = 0.126, SRMR = 0.072). Standardized factor loadings for all measures in this model were moderate to high in schizophrenia (range = 0.305–0.786) and in ASD (range = 0.554–0.824), as were correlations among factors (schizophrenia range = 0.444–0.760; ASD range = 0.586–0.776) (Table 3). Thus, within disorders, each measure captured a substantial amount of variance in its respective cognitive domain, and each domain also showed substantial covariation with other domains.

Fig. 1. Path diagram depicting the configural model. Bolded items represent transdiagnostic equivalence of parameters across schizophrenia and ASD. (a) Hypothesized factors and measures in three-factor model. (b) Configural invariance establishes invariant configurations of relationships among measures and factors across schizophrenia and ASD, as in model M3. (c) Metric invariance establishes invariant factor loadings across schizophrenia and ASD, as in model M4. (d) Scalar invariance establishes invariant means for measures (except for NAB and WMS) across schizophrenia and ASD, as in model M6. (e) Structural invariance establishes invariant factor variances across schizophrenia and ASD, as in model M7. (f) Structural invariance further establishes invariant factor covariances across schizophrenia and ASD, as in model M8.
Table 3. Standardized factor loadings and covariances for the configural model across groups
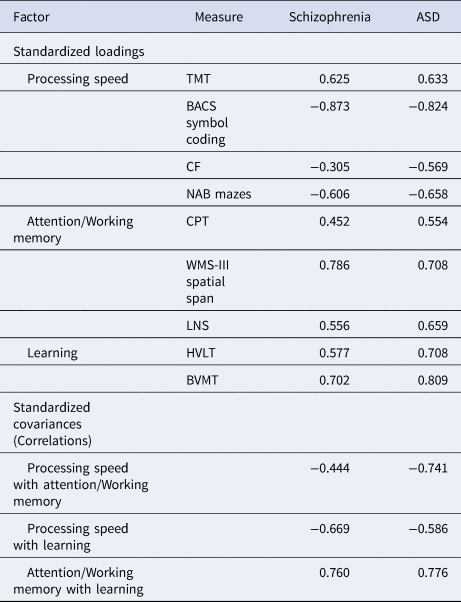
BACS Symbol Coding, Brief Assessment of Cognition in Schizophrenia, Symbol Coding subtest; BVMT, Brief Visuospatial Memory Test-Revised; CF, Category Fluency; CPT, Continuous Performance Test-Identical Pairs; HVLT, Hopkins Verbal Learning Test – Revised; LNS, Letter Number Span; NAB Mazes, Neuropsychological Assessment Battery, Mazes subtest; TMT, Trail Making Test, Part A; WMS-III Spatial Span, Wechsler Memory Scale – Third Edition, Spatial Span subtest.
Note. All parameters are significant at p < 0.001.
We then sought to establish whether the measures informed the same domains across disorders. The three-factor model demonstrated good fit across schizophrenia and ASD (M3), supporting MCCB's transdiagnostic configural invariance (Fig. 1b). Because MCCB measures assess three cognitive domains in both groups, each informed by the same subset of measures, the factor structure for MCCB can be compared transdiagnostically.
Establishing measurement invariance
Next, we examined whether the relationships among the measures and domains were likely to be transdiagnostically invariant in two steps: (1) metric invariance of the correlations among measures and domains, and (2) scalar invariance of the measures’ means for a given domain score. The first step of establishing measurement invariance was complete when constraining the structure's factor loadings to equivalence across groups maintained the model fit (Fig. 1c). The loading of a designated reference measure was constrained to 1 for each factor (TMT, CPT, and HVLT). This model (M4) retained good fit, confirming that a difference in a cognitive domain score is associated with the same differences in the values of its contributing measures in both groups.
For the second step of establishing measurement invariance, given that constraining all intercepts to equality in schizophrenia and ASD significantly reduced model fit (M5), partial scalar invariance was established by constraining as many intercepts to equivalence as possible while maintaining the model's fit (Fig. 1d; online Supplemental Table S2) (Milfont and Fischer, Reference Milfont and Fischer2010). Specifically, in this model (M6), beyond the reference measures for each factor, the means for BACS Symbol Coding and NAB Mazes may be transdiagnostically invariant for a given score in Processing Speed, the mean for LNS may be transdiagnostically invariant for Attention/Working Memory, and the mean for BVMT may be transdiagnostically invariant for Learning. Given the small proportion of non-invariant intercepts and that the invariant intercepts were not uniformly higher in one group than the other (Chen, Reference Chen2008), bias in comparing means across groups is unlikely to be substantial. Overall, this model confirms that a given cognitive domain score is associated with similar values of its contributing measures in both groups.
The above analyses together demonstrate that the majority of factor loadings and intercepts are likely to be invariant across groups for each factor. Thus, both groups show similar relationships among measures and domains and similar mean values for measures, supporting MCCB's transdiagnostic measurement invariance. Thus, scores for MCCB measures can be compared transdiagnostically.
Establishing structural invariance
Finally, we examined whether the cognitive domains are likely to be transdiagnostically invariant. Constraining all factor variances to equality across schizophrenia and ASD maintained model fit (M7; Figure 1e), as did constraining factor covariances to equality across groups (M8; Figure 1f). These results confirm that both groups show similar variability in each cognitive domain and similar relationships among cognitive domains, supporting MCCB's transdiagnostic structural invariance. Thus, scores for MCCB cognitive domains can be compared transdiagnostically.
Discussion
Replicating the results of prior studies in heterogenous samples of schizophrenia-spectrum disorders (Burton et al., Reference Burton, Vella, Harvey, Patterson, Heaton and Twamley2013; Lo et al., Reference Lo, Szuhany, Kredlow, Wolfe, Mueser and McGurk2016), this study demonstrated that MCCB is represented by a three-factor structure in schizophrenia consisting of processing speed, attention/working memory, and learning. Furthermore, this study is the first to extend these findings across neurodevelopmental diagnoses, confirming that MCCB comparisons are likely to be valid across schizophrenia and ASD.
Configural invariance
Our findings indicate that MCCB measures inform the same three cognitive domains across schizophrenia and ASD. These cognitive domains capture different levels of cognitive processing, from lower-order, basic processing speed and mid-level attention/working memory, to higher-order, complex cognitive abilities involved in learning. Despite the three-factor model showing significantly better fit than the one-factor model in schizophrenia in our study and previous literature (Burton et al., Reference Burton, Vella, Harvey, Patterson, Heaton and Twamley2013; Lo et al., Reference Lo, Szuhany, Kredlow, Wolfe, Mueser and McGurk2016), the large correlations among factors and the substantial improvements in model fit after allowing residuals to be correlated across factors is consistent with the generalized cognitive deficit model of schizophrenia (Dickinson et al., Reference Dickinson, Ragland, Gold and Gur2008).
Our three-factor model replicates prior models in the literature with minor modifications. Although no adjustments were necessary to obtain good model fit for the three-factor model by Burton et al., (Reference Burton, Vella, Harvey, Patterson, Heaton and Twamley2013), in this study, three pairs of residuals were allowed to correlate, two of which were overlapping with the five pairs of correlated residuals in the three-factor model presented by Lo et al., (Reference Lo, Szuhany, Kredlow, Wolfe, Mueser and McGurk2016): NAB Mazes and CPT, and NAB Mazes and WMS-III Spatial Span. The factor structure of the Norwegian-language MCCB has been examined in a large sample comparable to the size of our schizophrenia sample (Mohn et al., Reference Mohn, Lystad, Ueland, Falkum and Rund2017), yet the Norwegian-language MCCB sample comprised schizophrenia-spectrum disorders, which is a broader diagnostic definition than the definition used in the current study. Furthermore, the analytical approach taken in the Norwegian-language MCCB study was atheoretical in using EFA rather than theorized cognitive domains to inform and test models in CFA. Although none of the factor structures showed even adequate fit for the Norwegian-language MCCB even after optimization (Mohn et al., Reference Mohn, Lystad, Ueland, Falkum and Rund2017), the three-factor model showed identical configural models to the three-factor models established in other studies (Burton et al., Reference Burton, Vella, Harvey, Patterson, Heaton and Twamley2013; Lo et al., Reference Lo, Szuhany, Kredlow, Wolfe, Mueser and McGurk2016) and the current study, except for spatial span additionally loading onto the learning factor. Ultimately, though levels of cognitive variation and covariation assessed by MCCB differ across schizophrenia samples, MCCB is represented by three correlated cognitive domains in schizophrenia.
To our knowledge, MCCB's factor structure has not previously been examined in ASD. Given that the three-factor model with correlated residuals fit significantly better than the one-factor model, our findings indicate MCCB performance may also be characterized by deficits in moderately correlated domains rather than one general cognitive domain in ASD without intellectual disability. Although most studies of nonsocial cognition in ASD have focused on specific cognitive domains and/or IQ (Charman et al., Reference Charman, Jones, Pickles, Simonoff, Baird and Happé2011; Magiati et al., Reference Magiati, Tay and Howlin2014), our study argues for more diverse assessments of multiple cognitive domains to parse the heterogeneity of cognitive profiles in ASD. More broadly, our findings support the utility of drawing upon conceptualizations and implementations of cognitive assessment from schizophrenia to inform our understanding of cognitive assessment in ASD.
Measurement invariance
MCCB measures shared the same relationships with the cognitive domains and largely the same patterns of mean scores for the cognitive domains across schizophrenia and ASD.
The strength and directionality of the relationships was likely to be transdiagnostically invariant for all measures, indicating that the measures are equally important to informing their respective domains across groups. Across groups, the processing speed domain shared the most overlap with pencil-and-paper measures. Furthermore, the attention/working memory domain shared the most overlap with spatial span (block stimuli) compared to the continuous performance test or letter-number span (symbol representations). The learning domain shared more overlap with visuospatial compared to verbal learning.
The means of the measures were likely to be transdiagnostically invariant except for category fluency, continuous performance, and spatial span, suggesting that, holding performance on other MCCB tests constant, schizophrenia and ASD showed similar mean levels of performance on these three measures. This is consistent with previous research showing poorer verbal comprehension (related to category fluency) and better visuospatial planning (related to spatial span) in verbal ASD adults compared to controls, a pattern of cognitive performance found in a subset of schizophrenia adults (Goldstein et al., Reference Goldstein, Minshew, Allen and Seaton2002).
Structural invariance
MCCB domains shared the same amount of variability and the same relationships with each other across schizophrenia and ASD. This suggests that, for these three domains corresponding to different hierarchical levels of cognitive processing, their variability and their overlap are likely to be transdiagnostically invariant. Ultimately, schizophrenia and ASD adults show performance deficits in the same cognitive domains, extending previous literature demonstrating similar cognitive deficits in schizophrenia and ASD (Goldstein et al., Reference Goldstein, Minshew, Allen and Seaton2002).
Considerations
This study is the first to validate a nonsocial cognitive battery across schizophrenia and ASD. The large, community-based sample is particularly well-suited for examining this question given the narrow diagnostic criteria defining both groups, the wide range of cognitive performance levels that were assessed, and the similarity of the groups for key characteristics that may impact cognition, such as age and intelligence. Despite these strengths, certain limitations remain. Schizophrenia participants were generally in the early course of their illness, which may limit the generalizability of these findings in individuals with chronic schizophrenia. Given the restriction of the ASD group to verbal adults, these findings may not generalize to adults with non-verbal ASD or comorbid intellectual disability. Although intelligence was similar across groups, education levels differed between groups. Given that the sample only included participants who did not meet criteria for intellectual disability, the majority of participants had attained some college education, which is a somewhat high level of educational attainment. Thus, these findings may not generalize to adults with schizophrenia who do not have comorbid intellectual disability. Furthermore, substance abuse problems were found only in the schizophrenia group. Despite the dissimilarities in education level and substance abuse across groups, most of the characteristics of MCCB's factor structure were likely to be transdiagnostically invariant, supporting MCCB's generalizability across schizophrenia and ASD.
Applications
To compare cognitive domain performance across schizophrenia and ASD for individuals who have completed the entire MCCB, each measure's factor loading (Table 3) can be multiplied by the measure's raw score. The sum of these weighted raw scores across the contributing measures yields the cognitive domain factor score.
Implications
In light of current movements to characterize transdiagnostic features of psychiatric and developmental disorders (Insel et al., Reference Insel, Cuthbert, Garvey, Heinssen, Pine, Quinn, Sanislow and Wang2010), our findings indicate that MCCB measurement of nonsocial cognition can be extended from schizophrenia to ASD. Our recent report of large cognitive improvements in ASD adults following Cognitive Enhancement Therapy (Eack et al., Reference Eack, Greenwald, Hogarty, Bahorik, Litschge, Mazefsky and Minshew2013b) is among the promising initial studies (Miyajima et al., Reference Miyajima, Omiya, Yamashita, Miyata, Yambe, Matsui and Denda2016; Okuda et al., Reference Okuda, Asano, Numata, Hirano, Yamamoto, Tanaka, Matsuzawa, Shimizu, Iyo and Nakazato2017) to reveal that cognition in ASD adults can indeed be improved using therapeutic approaches that have a proven track record of improving cognition in schizophrenia adults (Wykes et al., Reference Wykes, Huddy, Cellard, McGurk and Czobor2011). Just as MCCB's adoption has vastly improved the comparability of cognitive outcomes across randomized control trials of schizophrenia treatments (Keefe et al., Reference Keefe, Davis, Harvey, Atkins, Haig, Hagino, Marder, Hilt and Umbricht2017), we hope that MCCB's adoption for treatment evaluation in ASD adults will advance the adaptation, development, and evaluation of evidence-based treatments for this underserved population.
Author ORCIDs
Susan S. Kuo, 0000-0001-9754-2970
Supplementary material
The supplementary material for this article can be found at https://doi.org/10.1017/S0033291719001582
Financial support
This work was supported by the Canadian Institutes of Health Research (S.S.K., Doctoral Foreign Study Award) and National Institutes of Health (M.S.K. and S.M.E., MH-92440; S.M.E., MH-8585, MH-95783, RR-24154, and DA-30763); Autism Speaks (S.M.E., 5703); and the Department of Defense (S.M.E., AR100344).
Conflict of interest
None.
Ethical standards
The authors assert that all procedures contributing to this work comply with the ethical standards of the relevant national and institutional committees on human experimentation and with the Helsinki Declaration of 1975, as revised in 2008.