Acute pain is an often unavoidable side-effect of medical procedures, such as treatment for burns (Tsirigotou, Reference Tsirigotou1993; Norman and Judkins, Reference Norman and Judkins2004), cancer (van den Beuken-van Everdingen et al., Reference van den Beuken-van Everdingen, Hochstenbach, Joosten, Tjan-Heijnen and Janssen2016), or dental (Pak and White, Reference Pak and White2011; da Costa et al., Reference da Costa, do Ribeiro and Cabral2012); surgery (Sommer et al., Reference Sommer, de Rijke, van Kleef, Kessels, Peters, Geurts, Gramke and Marcus2008), or intensive care procedures (Barr et al., Reference Barr, Fraser, Puntillo, Ely, Gélinas, Dasta, Davidson, Devlin, Kress, Joffe, Coursin, Herr, Tung, Robinson, Fontaine, Ramsay, Riker, Sessler, Pun, Skrobik and Jaeschke2013). Inappropriate management of acute pain is accompanied by protracted hospitalization (Chan et al., Reference Chan, Blyth, Nairn and Fransen2013), short-and long-term costs (Torrati et al., Reference Torrati, Rossi, Ferreira, Dalri, de Carvalho and dos Santos Barbeira2000) and represents a risk factor for developing chronic or persistent pain (Turk and Okifuji, Reference Turk and Okifuji2002; Breivik et al., Reference Breivik, Eisenberg and O'Brien2013).
Psychological interventions for acute pain management, such as relaxation (Seers and Carroll, Reference Seers and Carroll1998) or distraction (Kleiber and Harper, Reference Kleiber and Harper1999) attempt to disrupt the process of allocating attentional resources to pain. However, not all patients are equally capable of making use of these techniques and effectively regulating their attention (Seers and Carroll, Reference Seers and Carroll1998), particularly in situations of increased pain salience and in the absence of goal directed motivation (Verhoeven et al., Reference Verhoeven, Crombez, Eccleston, Van Ryckeghem, Morley and Van Damme2010). Though psychological interventions are generally effective in adults, particularly in some contexts like burn wound care (Scheffler et al., Reference Scheffler, Koranyi, Meissner, Strauß and Rosendahl2017), their effectiveness is more limited in children and adolescents (Uman et al., Reference Uman, Chambers, McGrath and Kisely2008), who are particularly ill-equipped to modulate pain attention, probably due to lower inhibition and working memory abilities (Verhoeven et al., Reference Verhoeven, Dick, Eccleston, Goubert and Crombez2014).
Virtual reality (VR) technology is a promising development for enhancing the effectiveness of traditional interventions, such as distraction or relaxation, for acute pain. An immersive (Brooks, Reference Brooks1999) and multi-sensorial experience (Gallace et al., Reference Gallace, Ngo, Sulaitis, Spence, Ghinea, Andres and Gulliver2012), achieved through a combination of technologies (i.e. head-mounted displays, vibro-tactile gloves, individualized sounds, and gesture-sensing joysticks), along with the possibility of active exploration could facilitate the shift of attention away from the painful stimuli or the experience of pain, aiding effective distraction and reshaped pain perception (Gold et al., Reference Gold, Belmont and Thomas2007; Piskorz and Czub, Reference Piskorz and Czub2014). The technology could be effectively exported in medical care settings (Li et al., Reference Li, Yu, Shi, Shi, Tian, Yang, Wang and Jiang2017) as a potentially cost-effective tool (Malloy and Milling, Reference Malloy and Milling2010), particularly since recent user-friendly developments (e.g. smaller headsets, intuitive controllers) do not require special training and could easily be used by medical providers (e.g. nurses). Moreover, some newer VR technologies like the multimodal device (MMD) are especially designed for medical settings with younger patients. The technology uses a hand-held screen as an alternative to HMD or video glasses to protect the visual functions of children under 7 years of age. Programs on it include tailored stories and interactive games that prepare children for undergoing medical procedures or are used for distraction during painful ones.
Single trials of VR-based interventions for acute pain are accruing, with both encouraging (Schmitt et al., Reference Schmitt, Hoffman, Blough, Patterson, Jensen, Soltani, Carrougher, Nakamura and Sharar2011; Gold and Mahrer, Reference Gold and Mahrer2018) and mixed results (Wint et al., Reference Wint, Eshelman, Steele and Guzzetta2002; Walker et al., Reference Walker, Kallingal, Musser, Folen, Stetz and Clark2014). One meta-analysis (Scheffler et al., Reference Scheffler, Koranyi, Meissner, Strauß and Rosendahl2017) of non-pharmacological treatments in general for adults undergoing burn care reported large effects for distraction interventions, particularly when these used VR, but the number of studies in this subgroup was small and outcomes of pain intensity, affective and cognitive components were combined. Another meta-analysis (Chan et al., Reference Chan, Foster, Sambell and Leong2018) examined VR-based treatments for painful clinical interventions and reported a moderate ES of 0.49 for maximum self-rated pain. Yet several clinically and theoretically important aspects were not investigated. Trials often also include additional measures of pain intensity (e.g. pain threshold), as well other pain-related outcomes (e.g. distress) and involve other assessors beside participant themselves. Moreover, the timepoint of pain assessment is subject to a clinically important distinction between real-time assessments ‘during’, and retrospective evaluations ‘after’, medical procedures. Comparisons between VR and other active treatments were not examined, though these could indicate whether observed effects are specific to VR or rather attributable to non-specific factors like novelty. Several potential moderators of clinical or theoretical importance were not examined. VR-enhanced interventions might be particularly effective for young participants, less able to engage in standard distraction. An important theoretical question is whether VR enhances the effectiveness of regular distraction. Possible moderating effects could result from concomitant analgesic use or the type of VR system employed. Finally, publication bias, as well potential adverse effects were not previously examined.
Hence, our goal was to assess the efficacy and safety of VR-based psychological interventions for pain associated to medical procedures, expanding on the issues identified above.
Method
Data sources and searches
We searched the National Library of Medicine via PubMed, Embase, PsycInfo and Cochrane Library databases through June 17th 2018, using the following keywords: ‘virtual reality’, ‘game’, ‘interface’, ‘immersion’, ‘virtual reality exposure therapy’, ‘pain’, ‘burn’, ‘wound’, and ‘injuries’ (complete search strings in online Supplementary Appendix 1). We also searched the references of previous narrative and systematic reviews.
Study selection
Eligible studies were: (1) randomized controlled trials (RCTs), in (2) patients of any age undergoing a painful procedure delivered in a medical setting comparing (3) a VR-based psychological intervention (4) with treatment as usual, (e.g. analgesics alone, standard distraction) or an active comparator devised by investigators (psychological, pharmacological), (5) reporting any pain outcomes, (6) published in peer-reviewed journals. VR interventions could be stand-alone or combined with another intervention (e.g., pain medication), provided the same ancillary intervention was also administered to the control group. Both crossover and parallel designs were eligible. No language restrictions were used.
We excluded the dissertations and conferences abstracts. For multiple reports of the same trial, we used the most complete one. One researcher screened all abstracts and flagged potentially eligible studies (RG). These were then retrieved full-text and independently assessed by two independent researchers (RG, LF). Disagreements were resolved by discussion with a third author (IC).
Data extraction
The primary outcome of interest was pain intensity (i.e. mean pain intensity, pain threshold and worst pain), measured by Visual Analogue Scale (VAS) or other clinical rating scales (e.g. Graphical Rating Scale/GRS, Faces Pain Scale), assessed real-time (i.e. during the medical procedure) or retrospectively (i.e. after the procedure). For outcomes assessed by more observers (e.g. child, parent, nurse), we extracted data for each. Secondary outcomes were cognitive (e.g. time spent thinking about pain and worry), and affective components of pain (e.g. pain unpleasantness, anxiety and distress) as assessed by VAS, GRS or other clinical rating scales (e.g. Symptom Distress Scale; Face, Leg, Activity, Cry, Consolability Scale).
For each included trial, we extracted information about: (a) study design (i.e. parallel, crossover); (b) medical procedure (e.g. dressing change, physical therapy); (c) condition requiring medical procedure (e.g. burn, cancer, dental treatment); (d) age group (i.e. children, adults or mixed); (e) recruitment (i.e. clinical, community); (f) VR-based intervention (e.g. distraction, psycho-education); (g) TAU or active comparator condition; (h) numbers of patients randomized in the treatment groups; (i) number of sessions; (j) concomitant analgesic use, and, if present the class of drugs (e.g. opioids, nonsteroidal anti-inflammatory drugs/NSAIDs, local); (k) VR system (e.g. head-mounted display/HMD, video glasses) and the number of interactive components (e.g. visual feedback, sound, navigation); (l) assessment of presence and immersion, if present; (m) adverse effects associated with VR; (n) number of drop-outs in the treatment groups; (p) VR program developer trial investigator (yes/no); (o) country of provenience. One reviewer (RG) extracted descriptive and outcome data. Another reviewer (IC) independently checked one third of the trials.
Risk of bias (Rob) assessment
RoB was assessed with the revised Cochrane Collaboration tool (Higgins et al., Reference Higgins, Sterne, Savović, Page, Hróbjartsson, Boutron, Reeves and Eldridge2016), separately for parallel and crossover designs, using templates with incorporated decision algorithms (available at: http://www.riskofbias.info/welcome/rob-2-0-tool). We evaluated sources of bias in five domains: (a) the adequate generation of the allocation sequence, (b) deviations from intended interventions (including blinding of participants and research personnel), (c) handling of incomplete outcome data, (d) measurement of the outcome (blinding of outcome assessors) and (e) selection of the reported results. For deviations from intended interventions, we rated studies as low risk of bias if the investigators described any valid method of blinding participants or research personnel, or if they specifically mentioned attempting to control for possible deviations. We rated studies as low risk for missing outcome data if all randomized participants were included in the analysis, the authors specified there were no or less than 5% missing data, or intent-to-treat analyses were used (Higgins et al., Reference Higgins, Sterne, Savović, Page, Hróbjartsson, Boutron, Reeves and Eldridge2016). For blinding of assessors, self-report assessments were considered high risk (Higgins et al., Reference Higgins, Sterne, Savović, Page, Hróbjartsson, Boutron, Reeves and Eldridge2016). In crossover trials, we also evaluated potential carry-over effects, by weighing whether the time elapsed between the successive interventions was sufficient to prevent carry-over. For selective outcome reporting, we extracted trial registration numbers in the paper and, if available, published protocols or other secondary reports. We also computed an overall RoB score for each study by awarding 1 point for each bias source rated as low risk, for use in subsequent sensitivity analyses. Ratings were independently done by three researchers (RG, LF, and IC) and disagreements were discussed and resolved.
Data synthesis and analysis
Meta-analyses
We used the software packages Comprehensive Meta-Analysis (CMA v. 2.2.064) for computing study-level effect estimates and Stata SE 14.0 (STATA Corp., Inc., College Station, TX) packages Admetan (Fisher, Reference Fisher2015, Reference Fisher2019) for pooling, Metabias (Harbord et al., Reference Harbord, Harris and Sterne2009) for testing small study effects and Confunnel (Palmer et al., Reference Palmer, Sutton, Peters and Moreno2008) for visualization. Effect sizes (ESs) were calculated as standardized mean difference (SMD) for each comparison, transformed in the adjusted Hedges' g (Hedges and Olkin, Reference Hedges and Olkin1985) to correct for the small sample size of most studies. In parallel designs, SMDs represent the difference between the means of the VR and the control group at each timepoint (real-time, retrospective), divided by the pooled standard deviations (s.d.) of the two groups, with positive values indicating superiority of VR-based interventions. When means and standard deviations were not reported, we computed the SMD from alternative statistics (Borenstein et al., Reference Borenstein, Hedges, Higgins and Rothstein2009), such as t values or p values from independent group comparisons at the time-point of interest, and sample sizes.
In crossover designs, we primarily relied on individual participant means in each period and derived SMDs by computing within-participant mean differences, corresponding standard errors (s.e.) for the differences, and the correlation between intervention and control (Elbourne et al., Reference Elbourne, Altman, Higgins, Curtin, Worthington and Vail2002). When individual participants means were not available, we computed the SMD from the within-subject mean differences, s.d. of differences and sample size, paired-sample t values or p values (Elbourne et al., Reference Elbourne, Altman, Higgins, Curtin, Worthington and Vail2002; Borenstein et al., Reference Borenstein, Hedges, Higgins and Rothstein2009).
If no usable information was available, authors were contacted. If a trial employed more comparison arms from the same category, only data for one of the eligible arms was used (i.e. the most similar to the other included trials). In the case of multiple VR intervention groups, we computed and averaged separate ESs for each comparison with a control group. If an outcome (e.g. pain intensity) was assessed by more observers (e.g. self-report, others), we computed ESs both separately and across all assessors. To facilitate the clinical interpretation, we also report absolute benefits as numbers-needed-to-be-treated (NNT), the number of patients that have to be treated in order to generate one additional positive outcome (Laupacis et al., Reference Laupacis, Sackett and Roberts1988), computed with the Kraemer and Kupfer formula (Kraemer and Kupfer, Reference Kraemer and Kupfer2006).
We aggregated individual ESs separately for: pain intensity as sensory component of pain, measured real-time and, respectively, retrospectively; time spent thinking about pain and worry as cognitive components of pain; and pain unpleasantness, anxiety, distress as affective components of pain. Comparisons against TAU or other active competitors were aggregated separately.
We pooled studies with a random-effect model. Based on previous systematic reviews and the particularities of the population and setting, we expected most studies to use small samples. Therefore, we used the Paule and Mandel estimator (Paule and Mandel, Reference Paule and Mandel1982) for between-study variance (τ 2), as recommended by a recent review of estimation methods (Veroniki et al., Reference Veroniki, Jackson, Viechtbauer, Bender, Bowden, Knapp, Kuss, Higgins, Langan and Salanti2016). We also applied the Hartung-Knapp-Sidik-Jonkman (HKSJ) variance correction (Hartung and Knapp, Reference Hartung and Knapp2001; Sidik and Jonkman, Reference Sidik and Jonkman2002), with truncation of correction factor at 1, recommended for random-effects meta-analysis with few studies (Röver et al., Reference Röver, Knapp and Friede2015). We evaluated statistical heterogeneity with the I 2 statistic, which shows the percentage of total variation across studies due to heterogeneity. Values across 25%, 50% and 75% suggest low, moderate and high heterogeneity (Higgins et al., Reference Higgins, Thompson, Deeks and Altman2003). We used the Q-profile (QP) method for constructing the confidence intervals around heterogeneity estimates, shown to be adequate in terms of coverage probabilities even in small samples (Viechtbauer, Reference Viechtbauer2007). We also report predictive intervals (PI) (Higgins et al., Reference Higgins, Thompson and Spiegelhalter2009), as the confidence interval of the approximate predictive distribution of future trial, considering heterogeneity.
Sensitivity and subgroup analyses
As we expected high heterogeneity and few studies with low RoB across domains, we computed two additional meta-analysis models: the Henmi-Copas approximate exact distribution, which produces a confidence interval for the pooled effect robust to publication bias (Henmi and Copas, Reference Henmi and Copas2010), and the Quality Effects model, which integrates study quality into pooled estimate, favoring (i.e. assigning larger weights) both larger and better trials (Doi et al., Reference Doi, Barendregt, Khan, Thalib and Williams2015). We used the overall RoB score as a proxy for study quality.
We also conducted sensitivity analyses: (i) excluding outliers (no overlap between the 95% CIs of the pooled ES with those of single trials); (ii) excluding trials using the new technology MMD; (iii) excluding comparisons where the control group received no treatment; (iv) for burns dressing change; (v) for children participants; (vi) separately for outcome assessors (self-report, other-reports); (vii) separately by design (parallel, crossover); (viii) for trials with at least 20 participants randomized/arm; (ix) excluding trials characterized by their authors as pilot or feasibility studies.
We had initially planned several of the sensitivity analysis as subgroup analysis, but realized the number of studies was too small. Using a rule of at least 10 studies per characteristic modelled (Higgins and Green, Reference Higgins and Green2011, sec. 9.6.5.1) and expecting at least one comparison with over 20 studies, we retained two characteristics: one of practical relevance- use concomitant analgesic use (present v. absent), and one of theoretical relevance- use of standard distraction in the control group, in the case this group received an intervention (Y/N). The correlation between the two characteristics was computed (Cramér's V for nominal data) to reduce the risk of confounding (Higgins and Green, Reference Higgins and Green2011, sec. 9.6.5.6). The statistical significance threshold for subgroup analysis was set at 0.025, Bonferroni corrected for multiple comparisons.
Small study effects and publication bias
We visually examined funnel plot asymmetry and constructed contour enhanced funnel plots (Peters et al., Reference Peters, Sutton, Jones, Abrams and Rushton2008), with contour lines indicating regions where the test of treatment effects was significant for various statistical significance levels. For comparisons with at least 10 ESs, we also conducted Eggers' test of the intercept (Egger et al., Reference Egger, Davey Smith, Schneider and Minder1997). We also addressed publication bias in sensitivity analysis with the Henmi-Copas estimate.
Results
Study selection
We identified 3381 records and screened 1943 after removal of duplicates (Fig. 1 and online Supplementary Appendix 1). We retrieved full-texts of 68 reports and further selected a total of 36 RCTs for inclusion. Figure 1 represents the flowchart of the inclusion process following the PRISMA guidelines (Moher et al., Reference Moher, Liberati, Tetzlaff and Altman2009). For 12 RCTs, data was insufficient for ES calculation and authors were contacted, with a second reminder if necessary. Data for ES calculation were retrieved in 3/12 cases (Bentsen et al., Reference Bentsen, Svensson and Wenzel2001; Maani et al., Reference Maani, Hoffman, Morrow, Maiers, Gaylord, McGhee and DeSocio2011; Schmitt et al., Reference Schmitt, Hoffman, Blough, Patterson, Jensen, Soltani, Carrougher, Nakamura and Sharar2011). In total, 27 included trials had sufficient information for ES calculation, and were included in the meta-analysis.
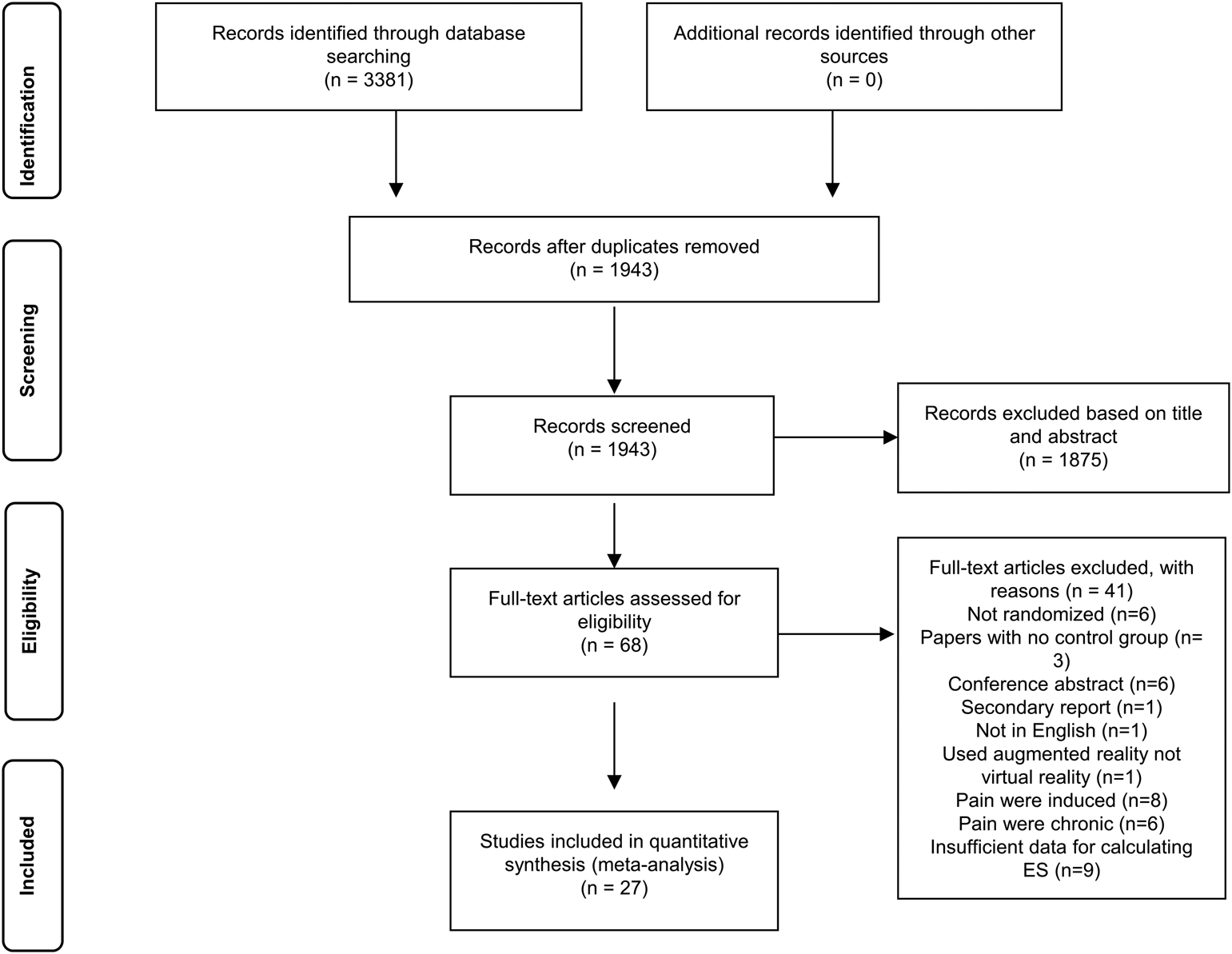
Fig. 1. PRISMA flow-diagram of the study selection process.
Characteristics of included studies
1452 patients were treated (659 with VR-based interventions and 793 with TAU or another active intervention) (Table 1 and online Supplementary Table S1). The average number of randomized participants in the VR arm was 25, and the average number of drop-outs was less than 1. Five trials had 10 or less participants per arm. Fourteen trials had parallel design and thirteen had crossover design (AB|BA format). Most trials were focused on burns, for new or chronic wounds, either for dressing change (12), or physical therapy (5), with Total Burn Surface Area (TBSA) ranging from 1% to 15%. Five studies were conducted for pain and distress related with needle procedures (e.g. during intravenous (IV) port access placement or phlebotomy), two with dental treatment and another two with chemotherapy. Most participants were recruited from clinical settings (26). Thirteen studies targeted children and youth, ten, adults and four, mixed samples. All but one of the VR-based interventions used distraction. Twenty-seven trials had a TAU comparison: no treatment (5), analgesics alone (16), distraction (2), analgesics plus distraction (4). Additionally, four trials also included an active comparator arm, designed for the purpose of the trial (e.g. external cold and vibration group (Gerçeker et al., Reference Gerçeker, Binay, Bilsin, Kahraman and Yılmaz2018) or video game group (Gershon et al., Reference Gershon, Zimand, Pickering, Rothbaum and Hodges2004)). Interventions ranged from one to five sessions and were all conducted individually. In eighteen studies, all participants received concomitant analgesics, most frequently Oxycodone opioids. The most used VR system was HMD (15), followed by video glasses (8) and MMD (3). The VR developer was also an investigator in twelve trials.
Table 1. Characteristics of included studies

a CO, crossover; P, parallel.
b Restorative Tx., dental restorations owing to primary cavities; Dress. Ch., dressing change; IV Placement, Intravenous (IV) placement; IV chemotherapy, Intravenous chemotherapy.
c Cond, condition; Tx, treatment; TBSA, total body surface area; MRI, magnetic resonance imaging; CT, computed tomography; FDC, first dressing change; Cancer Tx., cancer treatment.
d Age gr, age group; child, children.
e Clin, participants recruited from clinical settings; comm, participants recruited from community settings.
f Nrand VR, number of participants randomized to the VR intervention.
g Nrand control, number of participants randomized to the control intervention.
h Interv., Intervention received by the experimental group; VR-Distr., distraction in VR; VR-RLX, relaxation in VR; VR-Distr. + analg., Distraction in VR plus analgesics.
i Control, intervention received by the control group; No Tx., no treatment received by the control group; Analg., analgesic only; Analg. + distraction, analgesic + distraction.
j N ses, number of sessions.
k Unpleas, unpleasantness; Time think, time thinking about pain; VAS, visual analog scale; FPS-R, FACES Pain Scale Revised; FLACC, Face, Legs, Activity, Cry, Consolability scale; WB-FACES, Wong-Baker FACES; GRS, Graphic Rating Scale; DFS, Dental Fear Survey; CHEOPS, Children's Hospital of Eastern Ontario Pain Scale; NRP, Numeric Rating Scale; APPT-WGRS, The Adolescent Pediatric Pain Tool – Word Graphic Rating Scale; NPRS, Numeric Pain Rating Scale; SAI, State-Anxiety Inventory for Adults; SDS, Symptom Distress Scale; VAT, Visual Analog Thermometer.
l Opioids, morphine and derivate (e.g. codeine, oxycodone); Opioids + NSAIDs, morphine and derivate + Nonsteroidal anti-inflammatory drugs; Local, cream or spray with local administration (e.g. Lidocaine, ELMA cream).
m Prov, provenience; IR, Iran; DK, Denmark; Au, Australia; ES, Spain; US, United States; CN, China; TR, Turkey; NL, Netherlands.
n Trials defined by their authors as pilot or feasibility studies.
Risk of bias in the included studies
Most of the included studies were rated as having some concerns or high risk of bias for both parallel and crossover (marked with * in online Supplementary Fig. S1) designs (Fig. 2 and online Supplementary Fig. S1). Random sequence generation was rated as some concerns in 13 trials and high risk in 8 trials. For deviations from intended interventions, 13 studies were rated as some concerns, and 8 studies as high risk. All studies were rated as low risk for missing outcome data. All studies used self-report measures. For bias due to selective reporting, based on the trial report and available protocols, 6 trials were rated at high risk and 21 as having some concerns. Only 3 trials were registered (Schmitt et al., Reference Schmitt, Hoffman, Blough, Patterson, Jensen, Soltani, Carrougher, Nakamura and Sharar2011; Brown et al., Reference Brown, Kimble, Rodger, Ware and Cuttle2014; JahaniShoorab et al., Reference JahaniShoorab, Ebrahimzadeh Zagami, Nahvi, Mazluom, Golmakani, Talebi and Pabarja2015), all retrospectively. Only two trials (Miller et al., Reference Miller, Rodger, Kipping and Kimble2011; Jeffs et al., Reference Jeffs, Dorman, Brown, Files, Graves, Kirk, Meredith-Neve, Sanders, White and Swearingen2014) could be rated as low RoB on at least 3 domains.

Fig. 2. Risk of bias graph: review authors' judgments about each risk of bias item presented as percentages across all included studies.
VR-based interventions v. TAU
Pain intensity (primary outcome)
Real-time: Nine RCTs (7 parallel) resulted into a Hedges' g of 0.95 (95% CI 0.32–1.57), NNT = 2.00, with high heterogeneity (I 2 = 86%; 95% CI 65–96) (Table 2, Figs 3 and 4). Sensitivity analyses indicated smaller effects with the Henmi-Copas model, g = 0.77, 95% CI 0.22–1.33, and larger with the Quality Effects model, g = 1.13, 95% CI 0.66–1.60, with heterogeneity remaining high (I 2 = 79%). The effect was reduced in a sensitivity analysis excluding outliers (n = 1), g = 0.74 (95% CI 0.25–1.24), I 2 = 74% (95% CI 20–94) and when only self-report was considered (n = 5), g = 0.65 (95% CI 0.32–0.98), I 2 = 0% (95% CI 0–82). Analyses restricted to children participants, for burns' dressing change, or in parallel designs yielded similar estimations. Six trials with 20 or more participants randomized per arm resulted into a similarly large g of 1.11 (95% CI 0.07–2.15), I 2 = 90% (95% CI 72–98). Owing to the high heterogeneity, all PIs, except for self-reported pain, included 0.
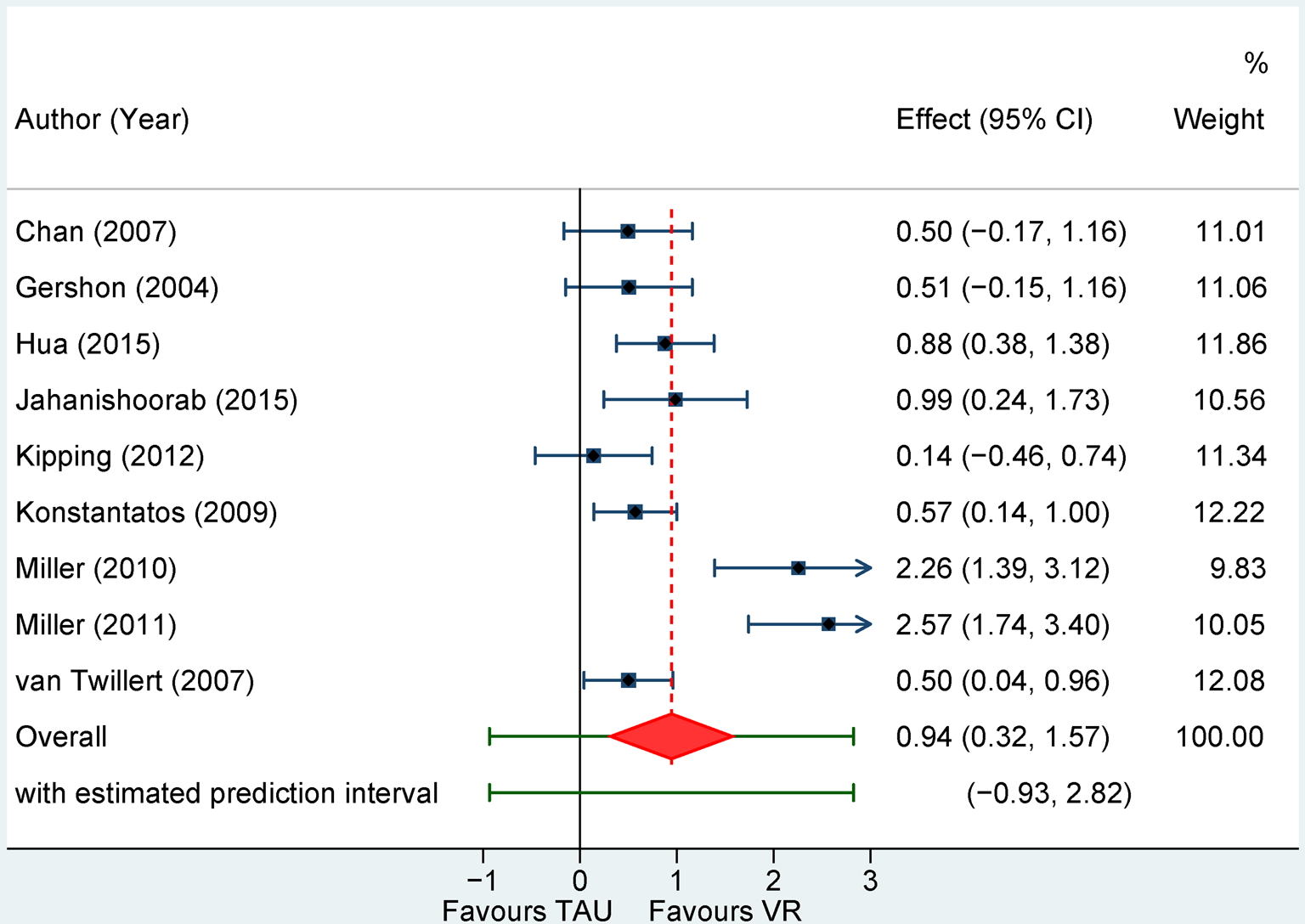
Fig. 3. Forest plot of standardized mean differences for pain intensity assessed real-time for VR-based interventions v. treatment as usual.
NOTE: Weights are form random-effects model
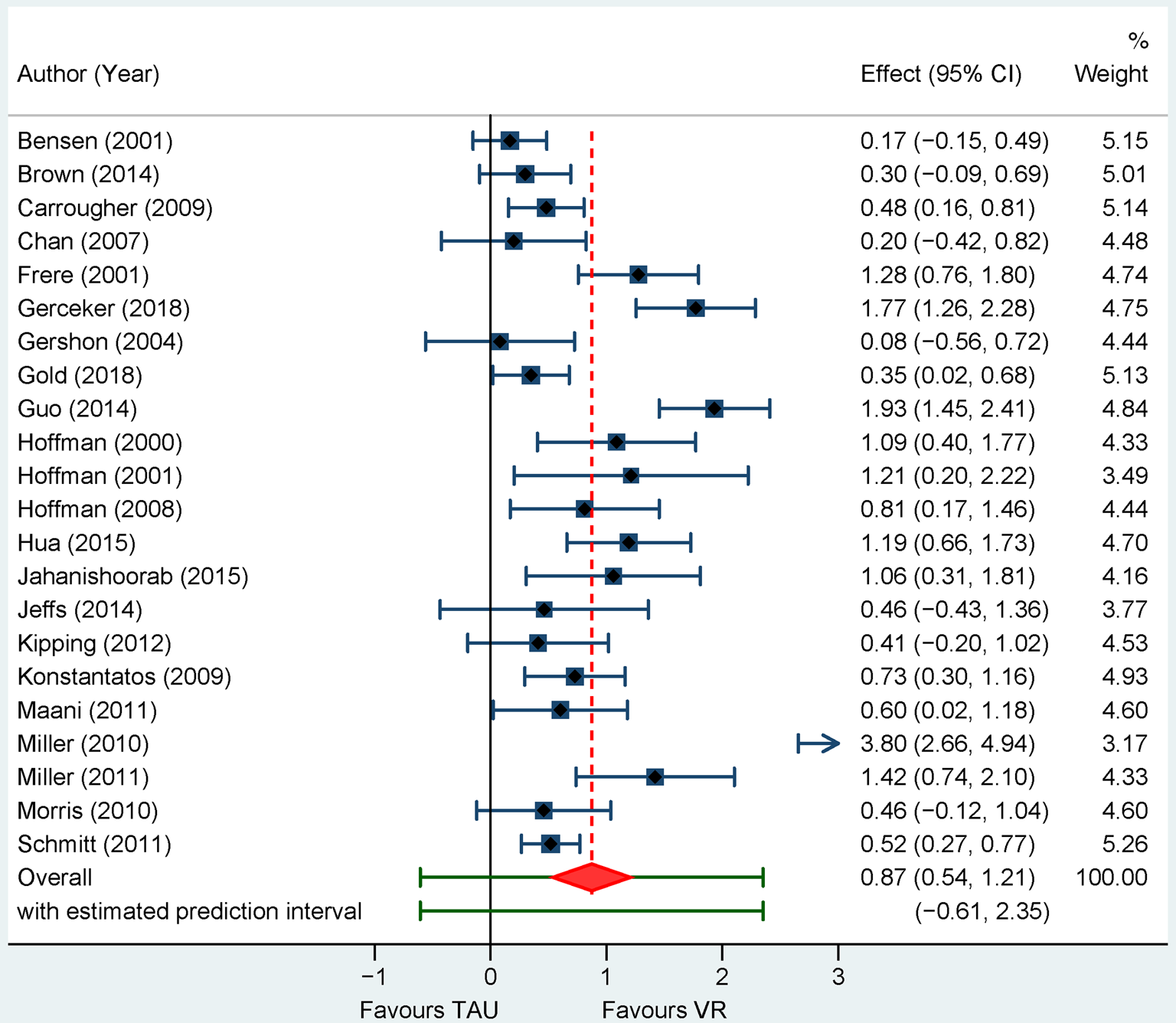
Fig. 4. Forest plot of standardized mean differences for pain intensity assessed retrospectively for VR-based interventions v. treatment as usual.
NOTE: Weights are form random-effects model
Table 2. VR-based interventions compared with treatment as usual (TAU)
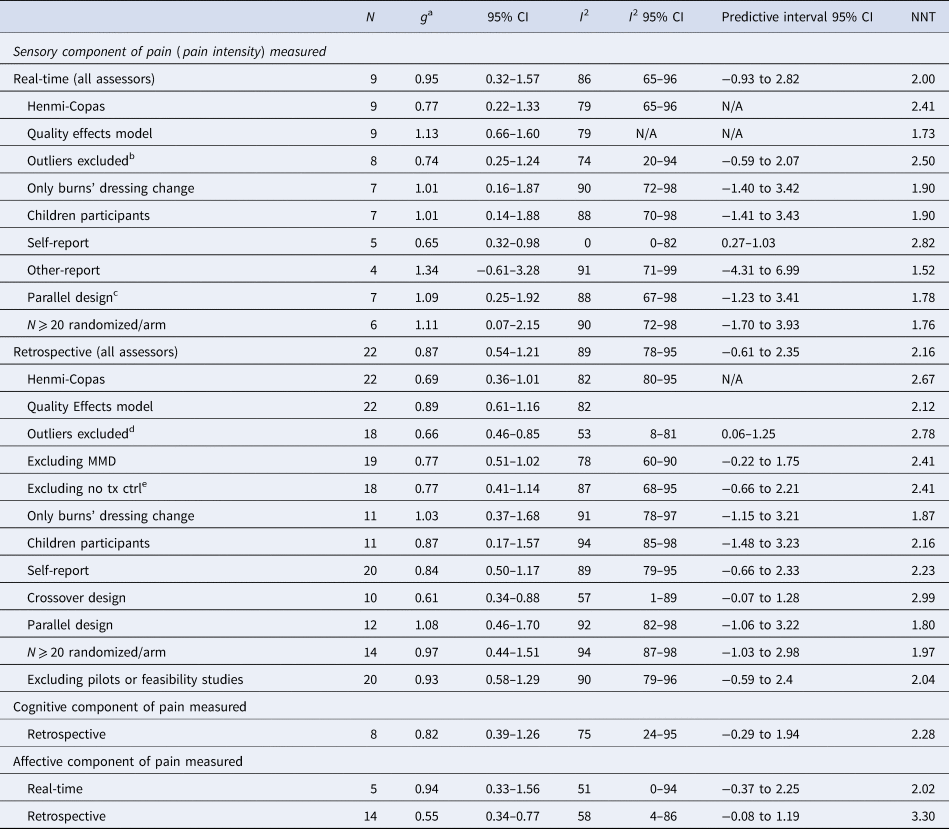
N, number of studies; NNT, numbers needed to treat; Child, children; Dress Ch, dressing change; Phys, Physical; Tx, Therapy; Ctrl, control; Conc, concomitent; Analg, analgesic; Distr, distraction; VR, Virtual Reality; HMD, Head-Mounted Display; VG, Video Glasses; MMD, Multi-modal device; RoB, risk of bias; N/A, not available
a All results are reported with Hedges' g, using a random effects model, positive effect indicates superiority of the experimental group over control group (significant results are marked with italic).
b Miller et al. (Reference Miller, Rodger, Kipping and Kimble2011).
c The two crossover studies were both identified by the authors as pilot or feasibility studies.
d Bentsen et al. (Reference Bentsen, Svensson and Wenzel2001); Gerçeker et al. (Reference Gerçeker, Binay, Bilsin, Kahraman and Yılmaz2018); Guo et al. (Reference Guo, Deng and Yang2015); Miller et al. (Reference Miller, Rodger, Bucolo, Greer and Kimble2010).
e Excluding trials with a no treatment control arm.
Retrospective: Twenty-two trials resulted into a pooled ES of g = 0.87 (95% CI 0.54–1.21), NNT = 2.16 with very high heterogeneity, I 2 = 89% (95% CI 78–95). Effects were smaller with the Henmi-Copas, g = 0.69, 95% CI 0.36–1.01, and similar with the Quality Effects models, g = 0.89, 95% CI 0.61–1.16, with heterogeneity remaining high (I 2 = 82%).
Sensitivity analyses showed decreased ESs with the exclusion of potential outliers (n = 4), g = 0.66 (95% CI 0.46–0.85), I 2 = 53% (95% CI 8–81), of MMD trials (n = 3), g = 0.77 (95% CI 0.51–1.02), I 2 = 78% (95% CI 60–90), or of trials with a no intervention control (n = 4), g = 0.77 (95% CI 0.41–1.14), I 2 = 87% (95% 68 to 95). Effects were also considerably smaller across crossover trials (n = 10), g = 0.61 (95% CI 0.34–0.88), I 2 = 57% (95% CI 1–89). Pain was self-reported in all but two trials. Analyses restricted to burns dressing change (n = 11), g = 1.03 (95% CI 0.37–1.68), I 2 = 91% (95% 78 to 97) or in parallel designs (n = 12), g = 1.08 (95% CI 0.46–1.70), I 2 = 92% (95% CI 82–98) led to slightly higher effects. Effects were similar for children participants (n = 11), g = 0.87 (95% CI 0.17–1.57), I 2 = 94% (95% 85–98), or in trials with at least 20 randomized participants per arm (n = 14), g = 0.97 (95% CI 0.44–1.51), I 2 = 94% (95% CI 87–98). All PIs except for the analysis without outliers included 0.
Affective and cognitive components of pain (secondary outcome)
Five studies assessed the affective component of pain real-time, g = 0.94 (95% CI 0.33–1.56), NTT = 2.02, I 2 = 51% (95%, 0–94) and 14 trials retrospectively, g = 0.55 (95% CI 0.34–0.77), NNT = 3.30, I 2 = 58% (95% CI 4–86). The cognitive component was assessed only retrospectively in eight trials, g = 0.82 (95% CI 0.39–1.26), NTT = 2.28, I 2 = 75% (95% CI 24–95).
VR-based interventions v. active comparators
Two studies assessed pain intensity real-time and four studies retrospectively, g = 0.69 (95% CI −0.58–1.97), I 2 = 83% (95% CI 43–99), PI −2.86 to 4.25. The affective component was assessed in 2 studies.
Adverse effects
Twelve studies evaluated potential nausea or simulator sickness associated with VR interventions (online Supplementary Table S1). In one, 15% of the participants reported nausea, and in another 5.2% reported nausea and 8% simulator sickness. In the remaining trials, none or under 5% of participants reported nausea.
Subgroup analysis
We only conducted planned subgroup analyses for VR-based interventions v. TAU for pain intensity assessed retrospectively. The two characteristics planned were correlated (Cramér's V = −0.57), therefore analyses were only conducted with analgesic use. Differences between studies using concomitant analgesic (n = 16, g = 0.78, 95% CI 0.37–1.19) v. those not using (n = 6 g = 1.09, 95% CI 0.33–1.86) were not significant, F(1,20) = 0.86, p = 0.36.
Small study effects
These were gauged for pain intensity assessed retrospectively (22 trials) (online Supplementary Fig. S2). The funnel plot appeared asymmetrical (online Supplementary Fig. S2A), and visualization with contour enhanced funnel plot (online Supplementary Fig. S2B) suggested that most studies were significant at the conventional threshold of p < 0.05. Egger's test was significant (intercept = 3.09, 95% CI 0.50–5.67, p = 0.021).
Discussion
In a meta-analysis of twenty-seven randomized trials, VR-based distraction interventions for procedural pain demonstrated reductions in pain intensity, assessed either real-time or retrospectively, compared to treatment as usual. Though effects appeared generally large, they were associated with high heterogeneity, with all predictive intervals including zero. Effectively, this implies that the effects of 95% of future similar trials fluctuate across a wide range of effects, both favorable and not to VR-based interventions. Across several sensitivity analyses, involving both alternative statistical models (i.e. robust to publication bias, considering study quality), and restricted to the largest, clinically relevant and more homogenous categories (e.g. children participants, burn dressing change procedures), heterogeneity remained high and effect estimates largely similar. VR-based interventions were also effective for the affective and cognitive components of pain, assessed retrospectively, though the number of trials was more limited. Only four studies contrasted VR-based interventions with active comparators with a non-significant but large effect. Adverse effects were reported in a minority of participants and mostly consisted of nausea and simulator sickness.
Despite these seemingly promising effects, serious methodological and reporting issues across the entire evidence base preclude any inferences regarding clinical effectiveness. First, trial risk of bias was rated as high or raising some concerns for most of the included trials for randomization, deviations from the intended intervention and selective reporting. In most instances, ratings were motivated by the absence of essential information for the assessment of these domains. Only three trials were registered, all retrospectively, and just one had a published protocol (Brown et al., Reference Brown, Kimble, Rodger, Ware and Cuttle2014). Crossover trials in particular were missing essential, often basic, descriptive information, such as the comparative baseline characteristics of participants randomized to receive the VR-intervention first (i.e. AB sequence) or last (i.e. BA sequence), reported in none of the trials. As the VR-based intervention involved specialized equipment, it was generally impossible to blind participants and the personnel administering it. Also, owing to the outcome (pain) or the assessment timepoint (real-time), all studies relied on self-report measures or used unblinded observers (i.e. parent, nurse, researchers).
The gaps in reporting also translated in the frequent absence of data necessary for effect estimation, particularly for crossover trials, where initially only half included usable data. Attempts to contact the authors in order to recover necessary data, even though repeated, were generally unsuccessful, leaving out almost a third of eligible trials from the meta-analysis. Missing information most likely affected the precision of the effect estimates, exposing our meta-analysis to the risk of selective reporting, and hampered a more meaningful exploration of moderator effects.
Other important caveats relate to heterogeneity around effect estimates being generally high and even extremely high, or with large confidence intervals (Jackson and Bowden, Reference Jackson and Bowden2016) across most analyses, and generally not diminished in sensitivity analyses. High heterogeneity impacts the precision of the effect estimates, and raises questions about their reliability (Ioannidis et al., Reference Ioannidis, Patsopoulos and Evangelou2007). Several analyses relied on a small number of studies and most included trials had small sample sizes. We tried to counteract these limitations by choosing a statistical approach resilient to bias in meta-analysis of small or few studies (Röver et al., Reference Röver, Knapp and Friede2015; Veroniki et al., Reference Veroniki, Jackson, Viechtbauer, Bender, Bowden, Knapp, Kuss, Higgins, Langan and Salanti2016), and by conducting several sensitivity analyses. There was also evidence of small study effects, and possible publication bias, with the pooled standardized mean differences reduced by 0.20 on average when the Henmi-Copas, a statistical model robust to publication bias was employed.
Our findings diverge from and extend those of a recent meta-analysis (Chan et al., Reference Chan, Foster, Sambell and Leong2018), reporting a moderate effect of VR interventions for self-reported ratings of worst pain. We used a larger array of pain intensity outcomes and distinguished between pain reported real-time and retrospectively, as well as between self- and other-report. Though generally larger, our estimates were similar in several sensitivity analyses. Most of the included studies focused on burns, where VR-based interventions appeared particularly effective for reducing pain intensity associated with dressing change. Scheffler et al. (Reference Scheffler, Koranyi, Meissner, Strauß and Rosendahl2017) also found a large effect for VR-based distraction for burn wound care in adults, though they combined pain intensity and other pain components and did not distinguish between dressing change and physical therapy. A very limited number of trials assessed other types of procedural pain, such as dental or needle related (e.g. IV placement). About half of the trials included in the meta-analysis involved children participants, resulting into estimates and heterogeneity nearly identical to the overall ones. Sensitivity analyses excluding studies using the MMD device considerably reduced the pooled effect size. Use of concomitant analgesic was not a moderator, though the number of studies in the ‘absent’ subgroup was disproportionately small. The dosage of analgesic received was usually not reported. Only one study (Carrougher et al., Reference Carrougher, Hoffman, Nakamura, Lezotte, Soltani, Leahy, Engrav and Patterson2009) examined whether VR-based interventions reduced concomitant analgesic use, with non-significant results.
Conclusions
Interpreting these results is a glass half-full/half-empty conundrum. The setting is challenging, with large trials absent and difficult to conduct. For several indications, such as burns, particularly with children, recruiting a reasonably large number of participants to be randomized is difficult. Moreover, VR-based interventions were, until recently, difficult to scale. Not coincidentally, half of the trials we included were cross-over. Procedural pain is an unavoidable side-effect in settings such as burn care, compelling medical staff, patients and caregivers to try to alleviate it by any intervention that appears safe. Distraction intervention are generally effective (Scheffler et al., Reference Scheffler, Koranyi, Meissner, Strauß and Rosendahl2017) for adults, but less so for children and adolescents (Birnie et al., Reference Birnie, Noel, Parker, Chambers, Uman, Kisely and McGrath2014). A new technology like VR, purported to enhance the effectiveness of ‘regular’ distraction, will likely be embraced. Moreover, a cost analysis simulation estimated that using adjuvant VR therapy for pain management in hospitalized patients would reduce costs by $5.4/patient (95% CI $11–$156) compared with TAU (Delshad et al., Reference Delshad, Almario, Fuller, Luong and Spiegel2018). Hence, our meta-analysis provides reassurance VR-based distraction interventions appear safe and with some benefits in reducing procedural pain.
Conversely, our results were based on small trials, at risk of bias in their design, implementation and reporting. The overall quality of evidence for VR-based interventions is poor, barring any meaningful implications for clinical practice. The patchy and often non-transparent reporting of trials hinders progress in the field, by stymieing larger scale replication and accurate assessment of effects. If anything, our findings amount to establishing proof-of-concept. Conclusions about clinical effectiveness and any potential for real-world implementation at a wider scale should be based on larger, prospectively registered and transparently reported Phase 3 trials. These could extend to medical conditions other than burns, such as cancer treatment or post-surgical pain, focus more on clinically relevant assessment points for pain, such as real-time, and include relevant clinical outcomes like changes in analgesics dose and type.
Supplementary material
The supplementary material for this article can be found at https://doi.org/10.1017/S0033291719001855.
Data
All extracted data used for effect size calculation and the STATA code for conducting meta-analysis (with the exception of study level effect size calculations done in Comprehensive Meta-analysis) are available on the Open Science Framework (DOI 10.17605/OSF.IO/J2QCF)
Author contributions
Author contributions (with author initials): Conceptualization (IAC); Methodology (RG, LAF, IAC); Data curation (RG, LAF); Formal analysis (RG, LAF); Supervision (IAC, AD); Writing- original draft (RG, LAF); Writing- reviews & editing (IAC, AD).
Financial support
Raluca Georgescu, Liviu A. Fodor and Ioana A. Cristea were supported by a grant from the Romanian Ministery of Research and Innovation, CNCS – UEFISCDI (project number PN-III-P1-1.1-TE-2016-1054). The funder had no role in the design of the study, collection, analysis and interpretation of data, and the decision to approve publication.
Conflict of interest
All authors have completed the ICMJE uniform disclosure form at http://www.icmje.org/coi_disclosure.pdf (available upon request from the corresponding author) and have nothing to disclose.