Introduction
Depression in adolescence and young adulthood
Adolescence and young adulthood are regarded as a transitional period of cognitive, psychosocial, physical and neurobiological development (IOM & NRC, 2014). Adolescence refers to the developmental stage spanning between the ages 10 and 18 years (Dick & Ferguson, Reference Dick and Ferguson2015; Patton et al., Reference Patton, Sawyer, Santelli, Ross, Afifi, Allen and Viner2016) while late teens to early 20s (approximately 18–26 years of age) is recognized as a distinct period of development known as emerging adulthood or young adulthood (Arnett, Reference Arnett2000; IOM & NRC, 2014; Patton et al., Reference Patton, Sawyer, Santelli, Ross, Afifi, Allen and Viner2016). Behavioural and emotional disturbances experienced during childhood and adolescence have been found to increase the likelihood of mental health problems during emerging adulthood (Kim-Cohen et al., Reference Kim-Cohen, Caspi, Moffitt, Harrington, Milne and Poulton2003). A recent literature review has suggested that young people having an episode of depression during the early years are more likely to experience episodes of depression later in life (Costello & Maughan, Reference Costello and Maughan2015).
Depression is currently the second leading cause of global disease burden, only after heart diseases (Ferrari et al., Reference Ferrari, Charlson, Norman, Patten, Freedman, Murray and Whiteford2013; Jamison et al., Reference Jamison, Breman, Measham, Alleyne, Claeson, Evans and Musgrove2006). The occurrence of depression increases rapidly during adolescence and young adulthood (Hankin et al., Reference Hankin, Young, Abela, Smolen, Jenness, Gulley and Oppenheimer2015; Lewinsohn, Rohde, Seeley, Klein, & Gotlib, Reference Lewinsohn, Rohde, Seeley, Klein and Gotlib2003). Symptoms typically start emerging during adolescence (Kessler et al., Reference Kessler, Berglund, Bruce, Koch, Laska, Leaf and Wang2001) and are likely to reoccur during young adulthood (Lewinsohn et al., Reference Lewinsohn, Rohde, Seeley, Klein and Gotlib2003), suggesting that depression occurs as a continuous development with no specific age markers (IOM & NRC, 2014). The National Institute of Mental Health (NIMH, 2017) estimated nearly 13.3% of adolescents, aged 12–17 years, in the USA suffer from depression between the ages 12 and 17 years. Abela and Hankin (Reference Abela, Hankin, Abela and Hankin2008) found 20–50% of adolescents reporting symptoms of depression before 18 years of age. Tanner et al. (Reference Tanner, Reinherz, Beardslee, Fitzmaurice, Leis and Berger2007) identified that emerging adults between the ages 18 and 29 years are at a greater risk of developing psychiatric conditions. Nearly 22% of young adults experiencing mood disorders, with depression being the most prevalent mood disorder with around 8.3% of young adults suffering from depressive illnesses (Tanner et al., Reference Tanner, Reinherz, Beardslee, Fitzmaurice, Leis and Berger2007). Research has further suggested that approximately 75% of mental health conditions develop before the age of 25 years, making adolescence and young adulthood a critical period of vulnerability in which the risk of developing depression increases sharply (Kessler et al., Reference Kessler, Berglund, Demler, Jin, Merikangas and Walters2005). Considering together the epidemiological data of depression among adolescents and young adults, the occurrence and pattern of depression in these two developmental phases is similar, providing a basis to explore depression as a developmental trajectory (Chaiton et al., Reference Chaiton, Contreras, Brunet, Sabiston, O'Loughlin, Low and O'Loughlin2013; Schubert, Clark, Van, Collinson, & Baune, Reference Schubert, Clark, Van, Collinson and Baune2017). Notably, gender differences in depression also start emerging during mid to late adolescence, following the same trend in young adults, with girls experiencing depressive symptoms 2–3 times more than boys (Abela & Hankin, Reference Abela, Hankin, Abela and Hankin2008; Hyde, Mezulis, & Abramson, Reference Hyde, Mezulis and Abramson2008; Zarate, Reference Zarate, Grant and Potenza2010).
Aetiology of depression
Symptoms of depression are often ascribed to early childhood experiences such as childhood psychopathology and maltreatment including violence, different forms of childhood abuse (Yaroslavsky, Pettit, Lewinsohn, Seeley, & Roberts, Reference Yaroslavsky, Pettit, Lewinsohn, Seeley and Roberts2013; Young, Klosko, & Weishaar, Reference Young, Klosko and Weishaar2003), insecure parental attachment (Costello, Swendsen, Rose, & Dierker, Reference Costello, Swendsen, Rose and Dierker2008), low socio-economic status (Yaroslavsky et al., Reference Yaroslavsky, Pettit, Lewinsohn, Seeley and Roberts2013), lack of effective education or changes associated with educational environment (Reinecke & Simons, Reference Reinecke and Simons2005), individual's behavioural-emotional characteristics such as dependency, internalizing behaviour problems (Reinherz, Paradis, Giaconia, Stashwick, & Fitzmaurice, Reference Reinherz, Paradis, Giaconia, Stashwick and Fitzmaurice2003) and other genetic or environment-associated stressors (WHO, 2017; Wray et al., Reference Wray, Ripke, Mattheisen, Trzaskowski, Byrne, Abdellaoui and Sullivan2018). However, the incidence of life stressors alone does not account for all symptoms of depression. Cognitive vulnerabilities have been identified as another leading factor towards the development of depression (Cohen, Hankin & Young, Reference Cohen, Hankin and Young2018; Hankin & Abramson, Reference Hankin and Abramson2002).
Previous research has identified various forms of cognitive vulnerabilities as key triggers towards depression such as dysfunctional attitudes (Lee & Hankin, Reference Lee and Hankin2009; Lewinsohn et al., Reference Lewinsohn, Rohde, Seeley, Klein and Gotlib2003), rumination (Hankin, Reference Hankin2008), low self-esteem (Abela & Taylor, Reference Abela and Taylor2003; MacPhee & Andrews, Reference MacPhee and Andrews2006), cognitive errors and maladaptive attributional styles (Cole & Turner, Reference Cole and Turner1993; Hankin, Reference Hankin2008; Hankin & Abramson, Reference Hankin and Abramson2002). Recently, research has shifted its focus towards another form of cognitive vulnerability, early maladaptive schemas (EMS), as an underlying contributing factor towards the development of depression.
Early maladaptive schemas
Young (Reference Young1990, Reference Young1999) defined EMS as broad, pervasive themes or patterns that directly influence individual's emotions, feelings, memories and cognitive processes (Young et al., Reference Young, Klosko and Weishaar2003). While these schemas are believed to develop mainly during childhood and adolescence, they continue to develop throughout one's lifetime and, if left untreated, can lead to significant functional impairments (Gong & Chan, Reference Gong and Chan2018). Maladaptive schemas exist in almost all the individuals, which are usually remaining dormant and hidden unless/until activated by a distressing situation or stressor, increasing an individual's risk for developing psychological difficulties (Schmidt & Joiner, Reference Schmidt and Joiner2004). EMS are classified into 18 schemas containing different cognitive content, grouped together in five different schema domains of; (i) disconnection/rejection, (ii) impaired autonomy/performance, (iii) other-directedness, (iv) impaired limits and (v) hyper-vigilance (Young et al., Reference Young, Klosko and Weishaar2003).
EMS and depression
Indeed, the presence of maladaptive schemas is considered as a strong vulnerability factor for the development of different mental health conditions including depression (Dozois & Beck, Reference Dozois, Beck, Dobson and Dozois2008). Research evidence based on mixed clinical samples has suggested significant associations between EMS and depressive symptoms, with EMS acting as a significant predictor of depression (Glaser, Campbell, Calhoun, Bates, & Petrocelli, Reference Glaser, Campbell, Calhoun, Bates and Petrocelli2002; Renner, Lobbestael, Peeters, Arntz, & Huibers, Reference Renner, Lobbestael, Peeters, Arntz and Huibers2012; Stopa, Thorne, Waters, & Preston, Reference Stopa, Thorne, Waters and Preston2001; Welburn, Coristine, Dagg, Pontefract, & Jordan, Reference Welburn, Coristine, Dagg, Pontefract and Jordan2002). However, there has been a lack of research investigating the role of specific EMS domains that contribute towards depressive tendencies (Glaser et al., Reference Glaser, Campbell, Calhoun, Bates and Petrocelli2002; Stopa et al., Reference Stopa, Thorne, Waters and Preston2001). Further, research studies were often confounded by comorbidities, thus providing insufficient evidence to draw conclusions about the relationship between EMS and depression. Significant associations between EMS and depression have been found in other studies employing non-clinical student samples, providing evidence of predominant activated EMS in adolescents (Calvete, Orue, & González-Diez, Reference Calvete, Orue and González-Diez2013; Muris, Reference Muris2006; Yigit, Kilic, Guzey Yigit, & Celik, Reference Yigit, Kilic, Guzey Yigit and Celik2018) and young adults with elevated depressive symptoms (Camara & Calvete, Reference Camara and Calvete2012; Eberhart, Auerbach, Bigda-Peyton, & Abela, Reference Eberhart, Auerbach, Bigda-Peyton and Abela2011; Harris & Curtin, Reference Harris and Curtin2002). However, results were generated using selective schema domains that make it difficult to interpret and integrate findings for all EMS domains linked to depression.
Schema domains as predictors of depression
In a clinically depressed sample, schema domains of disconnection/rejection and impaired autonomy/performance were found to be significant predictors of depressive symptoms (Glaser et al., Reference Glaser, Campbell, Calhoun, Bates and Petrocelli2002; Renner et al., Reference Renner, Lobbestael, Peeters, Arntz and Huibers2012; Wegener, Alfter, Geiser, Liedtke, & Conrad, Reference Wegener, Alfter, Geiser, Liedtke and Conrad2013). Similar results were replicated in a comparative study of three different clinically diagnosed patients (clinically depressed, previously depressed and other clinical diagnoses; Wang, Halvorsen, Eisemann, & Waterloo, Reference Wang, Halvorsen, Eisemann and Waterloo2010). In another study conducted with clinically depressed patients, the schema domains of disconnection/rejection and impaired limits were significantly associated with maintaining symptoms of depression (Halvorsen, Wang, Eisemann, & Waterloo, Reference Halvorsen, Wang, Eisemann and Waterloo2010; Welburn et al., Reference Welburn, Coristine, Dagg, Pontefract and Jordan2002).
Overall, research evidence suggests that three schema domains, disconnection/rejection, impaired autonomy/performance and other-directedness, mainly act as potent vulnerability markers towards developing and maintaining depressive tendencies (Glaser et al., Reference Glaser, Campbell, Calhoun, Bates and Petrocelli2002; Halvorsen et al., Reference Halvorsen, Wang, Eisemann and Waterloo2010; Renner et al., Reference Renner, Lobbestael, Peeters, Arntz and Huibers2012; Wang et al., Reference Wang, Halvorsen, Eisemann and Waterloo2010; Wegener et al., Reference Wegener, Alfter, Geiser, Liedtke and Conrad2013; Welburn et al., Reference Welburn, Coristine, Dagg, Pontefract and Jordan2002). However, these studies did not take into account any other psychopathologies or include a control group. In addition, findings based on clinical individuals could not be generalized to a non-clinical youth sample.
Similar results have been explored in adolescents, with reciprocal relationships found between schema domains of disconnection/rejection, impaired autonomy/performance and other-directed schemas and depression (Calvete, Orue, & González-Diez, Reference Calvete, Orue and González-Diez2013; Lumley & Harkness, Reference Lumley and Harkness2007; Van Vlierberghe, Braet, Bosmans, Rosseel, & Bögels, Reference Van Vlierberghe, Braet, Bosmans, Rosseel and Bögels2010). The same three schema domains were found to be contributing towards depressive tendencies among young adults (Braet, Van Vlierberghe, Vandevivere, Theuwis, & Bosmans, Reference Braet, Van Vlierberghe, Vandevivere, Theuwis and Bosmans2013; Calvete, Orue, & Hankin, Reference Calvete, Orue and Hankin2015; Camara & Calvete, Reference Camara and Calvete2012; Eberhart et al., Reference Eberhart, Auerbach, Bigda-Peyton and Abela2011; Schmidt & Joiner, Reference Schmidt and Joiner2004). Again, the research studies with adolescents and young adults only included selective schema domains based on previous research findings with clinical sample making it difficult to analyse an overall effect of EMS and schema domains on depression among adolescents and young adults.
Aims of the current meta-analysis
Taken together, while the association between EMS and depression has been widely investigated in adults, the strength of this relationship among adolescents and young adults is less certain. Added to the complexity is that EMS are considered fluid during the developmental period of adolescents and young adults (Rijkeboer & De Boo, Reference Rijkeboer and De Boo2010). The overall objective of the current systematic literature review and meta-analysis was to synthesize research regarding the overall effect of EMS, different EMS domains and depressive symptoms among adolescent or young adults. Specifically, we sought to address the following research questions:
1. What is the strength of the association between EMS and depression among adolescents and young adults?
2. What is the strength of relationship between each of the schema domains and depressive symptoms among adolescents and young adults?
3. Do age and gender moderate the association between schema domains and depressive symptoms?
The protocol for the current meta-analysis was registered on PROSPERO: CRD42019135911.
Method
Literature search
The review was carried out in October 2019 following the guidelines provided by the Preferred Reporting Items for Systematic Review and Meta-analysis (PRISMA; Moher, Liberati, Tetzlaff, & Altman, Reference Moher, Liberati, Tetzlaff and Altman2009). A systematic literature search was completed using seven databases including Embase, CINAHL, Medline, ASSIA, Psych INFO, Scopus and Web of Science. The following specific search terms applying truncations (* and $) in combination with Boolean characters ‘AND’ or ‘OR’ was used to enhance the search sensitivity: ‘Early maladaptive schemas’ OR ‘Young schema’ OR ‘EMS’ AND ‘Depression’ OR ‘Depressive Disorder’ OR ‘Depressive Symptoms’. Google Scholar and reference lists of relevant articles were further scanned to include grey literature that was unavailable through the databases.
Inclusion/exclusion criteria
Searches were limited to articles published in the English language in peer-reviewed journals. Studies were included in this review if they fulfilled the following criteria: (i) reported effect sizes of the relationship between EMS and depression or contained the information necessary to analyse effect sizes; (ii) the primary outcomes were measured using standardized and validated measures of EMS and depression; (iii) participants were adolescents and young adults (with a mean age between 10 and 29 years); studies with a sample mean age below 10 years or over 30 years were excluded; (iv) studies measuring beliefs, cognitive biases or other constructs that were not explicitly associated with Young's concept of EMS were excluded; (v) case reports, book chapters, qualitative studies, dissertations, conference proceedings, theoretical papers and reviews were also excluded. The PRISMA diagram (Moher et al., Reference Moher, Liberati, Tetzlaff and Altman2009) in Fig. 1 shows the results of the systematic search and selection process conducted for the present review.
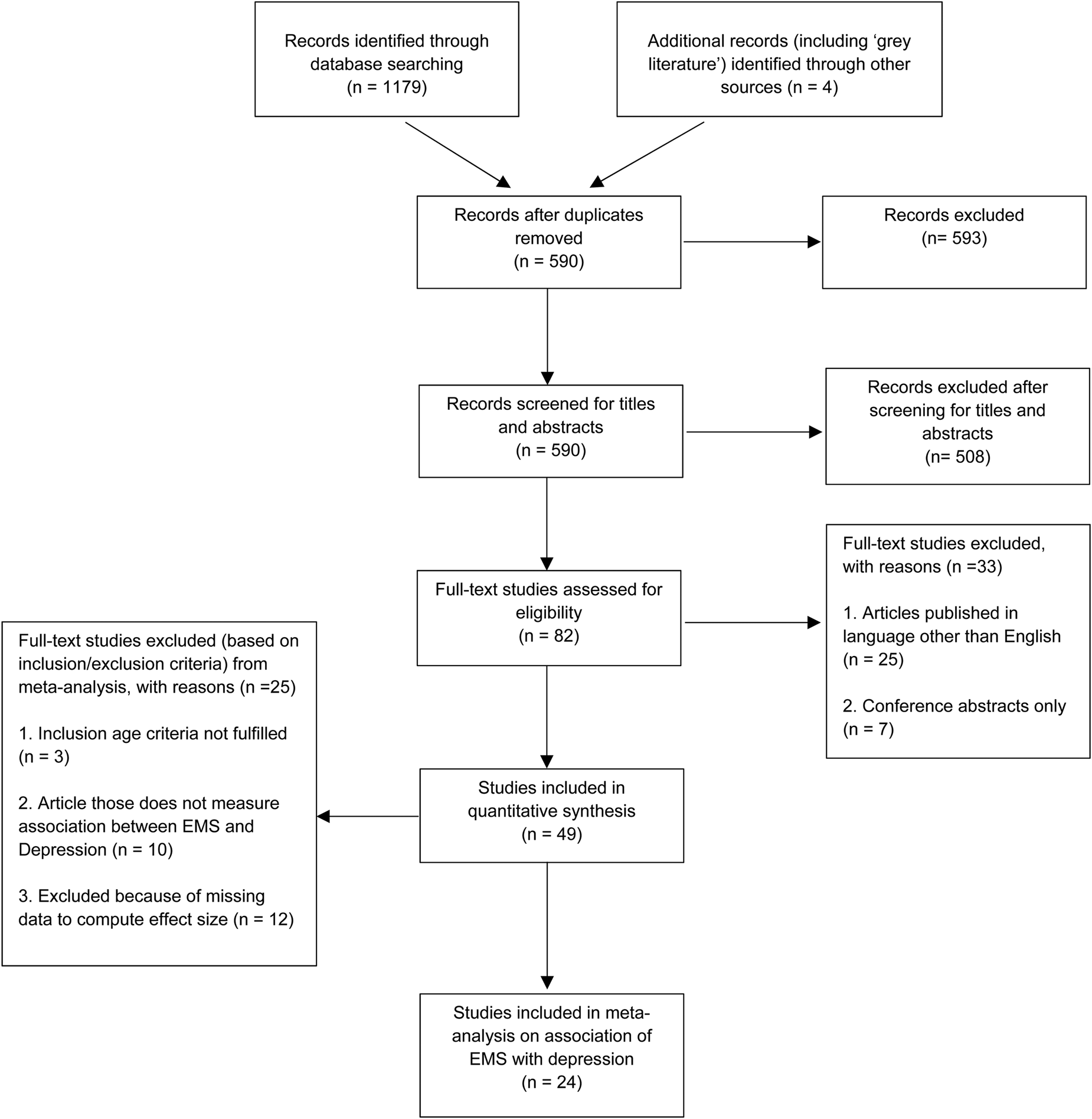
Fig. 1. Systematic search and selection process (PRISMA; Moher et al., Reference Moher, Liberati, Tetzlaff and Altman2009).
Sample of studies
The initial search resulted in 1183 studies (Embase = 194, ASSIA = 170, CINHAL = 116, Medline = 182, Psych INFO = 275, Scopus = 164, Web of Science = 78 and Grey Literature = 04). The primary reviewer screened the titles and abstracts of each article retrieved through the initial search of databases. The eligibility of each article based on title and abstract was assessed against the inclusion and exclusion criteria described above. Full texts of potentially eligible articles were checked to confirm their eligibility. A second reviewer independently carried out an additional screening of the full-text articles to assess eligibility. Few minor disagreements were resolved through discussion between the two reviewers. Following the implementation of the search scheme and inclusion/exclusion criteria, 24 studies representing 24 samples (N = 13 632) were found eligible to be included in the current meta-analysis (see Table 1). All included studies were reported from peer-reviewed articles, published between 2002 and 2018.
Table 1. Characteristics of the studies included in the meta-analysis (n = 24)

BDI-II, Beck Depression Inventory-II (Beck, Steer, & Brown, Reference Beck, Steer and Brown1996); CES-D, Centre for Epidemiologic Studies Depression scale (Radloff, Reference Radloff1977); SCL-Dep subscale, Symptom Checklist Depression sub-scale (Derogatis, Reference Derogatis and Maruish1994); ZSDS, Zung Self-Rating Depression Scale (Zung, Richards, & Short, Reference Zung, Richards and Short1965); PQY, Psychopathology Questionnaire for Youths (Hartman et al., Reference Hartman, Hox, Mellenbergh, Boyle, Offord, Racine and Sergeant2001); TDI, Teate Depression Inventory (Balsamo & Saggino, Reference Balsamo and Saggino2013); BDI, Beck Depression Inventory (Beck, Rush, Shaw, & Emery, Reference Beck, Rush, Shaw and Emery1979); DASS, Depression, Anxiety and Stress Scale (Lovibond & Lovibond, Reference Lovibond and Lovibond1995); CDI, Children's Depression Inventory (Kovacs, Reference Kovacs1992).
Data extraction
The primary reviewer used a pre-determined form to carry out data extraction from the included studies. The following information was extracted: study setting/design, participants' demographics such as age mean, s.d. and range, gender ratio, sample size, measures employed to assess EMS and depression. Table 1 provides a summary of study characteristics extracted from the 24 included studies.
Measurement of early maladaptive schemas
All studies included in the quantitative synthesis used one of the four EMS measures based on Young's schema theory (Young et al., Reference Young, Klosko and Weishaar2003). Specifically, the 90-item Young Schema Questionnaire-Short form version 3 (YSQ-S3; Young & Brown, Reference Young and Brown2005) was the most commonly used version, having been used in 12 of the included studies. The 75-item Young Schema Questionnaire-Short form (YSQ-SF; Young & Brown, Reference Young and Brown1998) was used in seven studies, while its adolescent version (YSQ-A; Van Vlierberghe et al., Reference Van Vlierberghe, Braet, Bosmans, Rosseel and Bögels2010) was used in two studies. The longest version (i.e. the 232-item Young Schema Questionnaire-Long form version 3; YSQ-L3) was used only in two studies.
All the above measures assess five different schema domains. However, the reviewed articles mostly employed three major schema domains, i.e. disconnection and rejection, impaired autonomy and other-directedness schema, which have schema content considered to be linked with symptoms of depression (Calvete, Orue, & González-Diez, Reference Calvete, Orue and González-Diez2013; Lumley & Harkness, Reference Lumley and Harkness2007; Van Vlierberghe et al., Reference Van Vlierberghe, Braet, Bosmans, Rosseel and Bögels2010; Young et al., Reference Young, Klosko and Weishaar2003).
Risk of bias assessment
To assess and appraise the quality of eligible studies, the risk of bias assessment was carried out independently by two reviewers using a bespoke quality assessment tool adapted by Marsh, Chan and MacBeth (Reference Marsh, Chan and Macbeth2018). The tool comprises of 11 items to be rated qualitatively by answering ‘Yes’, ‘No’, ‘Partially’ or ‘Cannot Tell’. Furthermore, the numerical scores were assigned to the qualitative ratings to help generate a total quality score for each study: ‘Yes’ = 2, ‘Partially’ = 1, ‘No’ = 0, ‘Cannot Tell’ = 0. No numerical value was assigned where the items did not meet the criteria for the study. The total score for each study was calculated by summing the numerical values and then expressed as percentage based on the number of items assigned a numerical rating. Inter-rater reliability (Cohen's κ) was calculated between two independent reviewers, which was found to be 0.86, indicating high level of agreement (McHugh, Reference McHugh2012) (see Table 2).
Table 2. Risk of bias (ratings assessed using the adapted AHRQ tool)

Quality ratings of included based on percentages, high quality = 80–100% category; moderate quality = 60–79% category; low quality = 50% or below.
Analytic procedure
Effect size coding
The correlation coefficient ‘r’ values were extracted as an effect size measure for the association between schema domains and depression. Where separate correlation coefficients were reported to describe the association between separate schemas with depression, Fischer z transformation was carried out to compute an average effect size estimate for each schema domain. According to Corey, Dunlap, and Burke (Reference Corey, Dunlap and Burke2010), averaging the correlation coefficient could lead to an underestimation as sampling distribution for correlation coefficients is always considered to be skewed; the recommended method was to convert the correlations to Fischer z and calculating a weighted mean using sample size for each study. After obtaining weighted means, Fischer z values were converted back to the correlation ‘r’.
Meta-analytical model
Results for meta-analysis were generated in RStudio (Version 1.2.5001) using ‘metaphor’ (Viechtbauer, Reference Viechtbauer2010), ‘robumeta’ (Fisher & Tipton, Reference Fisher and Tipton2015) and ‘MAc’ (Del Re & Hoyt, Reference Del Re and Hoyt2010) package developed to facilitate reviewers by the R Development Core Team (2015). A random-effect model was used to synthesize quantitative results considering the heterogeneous nature of the study sample. Correlation ‘r’ to Fischer z transformations were employed to compute the meta-analytic results. Fischer z's were then converted back to correlation ‘r’ to report the effect size estimates. Q-statistic was calculated to estimate the true heterogeneity of effect sizes. Higgins, Thompson, Deeks, and Altman (Reference Higgins, Thompson, Deeks and Altman2003) suggested that a statistically significant Q-statistic indicates the presence of heterogeneity, i.e. the presence of true between-studies variation. I 2 statistic was calculated to provide a percentage of the actual variance between studies presenting the real differences between effect sizes, with 25, 50 and 75% representing the estimation of low, medium and high levels of heterogeneity (Higgins et al., Reference Higgins, Thompson, Deeks and Altman2003). Although Q and I 2 statistics are considered reliable tests to ascertain heterogeneity, they do not specify the studies which are more likely to influence heterogeneity. Baujat, Mah, Pignon, and Hill (Reference Baujat, Mah, Pignon and Hill2002) have developed ‘Baujat plots’ to identify the contribution of each study in overall results of heterogeneity with studies falling in the top quadrant of the plot contributing the most.
Publication bias
The funnel plots for each study are generated with effect sizes plotted on the horizontal axis and corresponding sample size (standard error) on the vertical axis. Studies with large standard errors tend to gather around the mean effect size, while those having smaller errors are more dispersed around the plot. Funnel plots are usually considered as a subjective measure of potential publication bias.
Rank of correlation test (Begg & Mazumdar, Reference Begg and Mazumdar1994) and Egger's test (Egger, Smith, Schneider, & Minder, Reference Egger, Smith, Schneider and Minder1997) were additionally employed as an objective method of assessing publication bias. A significant rank of correlation test and Egger's test represent the presence of potential publication bias.
Results
A total sample of N = 13 632 (mean age = 19.49, s.d. = 3.07) from the eligible studies (k = 24) were included in the meta-analytic results to examine the association between EMS and depression. Separate meta-analytic results were generated to explore the association between depression and different schema domains. Table 3 summarizes the results for meta-analytic models.
Table 3. Meta-analytic results of association between early maladaptive schemas and depression

k, Number of studies; N, total number of participants; r, average uncorrected correlation; 95% CI, confidence interval, *p < 0.05, **p < 0.01, ***p < 0.001.
Effect size reporting for association between EMS and depression
The random-effect model estimate for association between overall EMS and depression was r = 0.56 (95% CI 0.49–0.63, Z = 12.88, p < 0.0001), suggesting that higher predominant EMS were significantly linked to higher levels of depressive symptoms among adolescents and young adults with a large effect size (Cohen, Reference Cohen1992). Moderator analysis was carried out with the mean age of sample showing no moderating effect of age on study variance [Q (1) = 3.78, p = 0.052]. Further, an additional moderator analysis was carried out using gender as a moderating variable to assess the effect of gender on overall association of EMS and depression. Two studies were excluded during meta-regression analysis, as they comprised of only female participants. The meta-regression conducted on n = 22 studies suggests that gender did not moderate the overall effect size association between EMS and depressive symptoms [Q (1) = 1.81, p = 0.18]. Figure 2 represents the forest plot of the overall meta-analytic model with almost all of the included studies reported moderate to large effect sizes.

Fig. 2. Forest plot of EMS and depression meta-analysis.
The results of heterogeneity showed significant heterogeneity among the sample with Q = 777.77, p < 0.0001 and I 2 = 96.84%, indicating 97% of the study variance resulted from the actual difference between studies. Besides, the Baujat plot was plotted to identify the studies that contributed to overall heterogeneity. Fig. 3 depicts that study 7, i.e. Alba et al. (2018), was the only study lying in the top quadrant contributing most to heterogeneity statistics. The rank of correlation (p = 0.33) and Egger's regression tests (p = 0.07) were non-significant suggesting that the above findings were not influenced by publication bias (see Fig. 4 for studies distribution around funnel plot).

Fig. 3. Baujat plot for total EMS scores and depression meta-analysis.

Fig. 4. Funnel plot for total EMS scores and depression meta-analysis.
Effect size reporting between disconnection/rejection schemas and depression
Based on all 24 included studies, the effect size estimate r = 0.49 (95% CI 0.43–0.55, Z = 14.22, p < 0.0001) showed a significant association between disconnection/rejection schemas and depressive symptoms, with a moderate effect size in a significantly heterogeneous set of samples (Q = 436.62, p < 0.0001). I 2 statistic indicated that 95% of the variance resulted from true between-study variance. Further, the rank of correlation (p = 0.11) and Egger's tests (p = 0.26) suggested that no evidence of publication bias. Neither age nor gender significantly moderates the association between disconnection/rejection schemas and depressive symptoms [age: Q (1) = 2.90, p = 0.09; gender: Q (1) = 0.97, p = 0.33]. Figure 5 shows the details of contribution of each study in overall meta-analytical results.
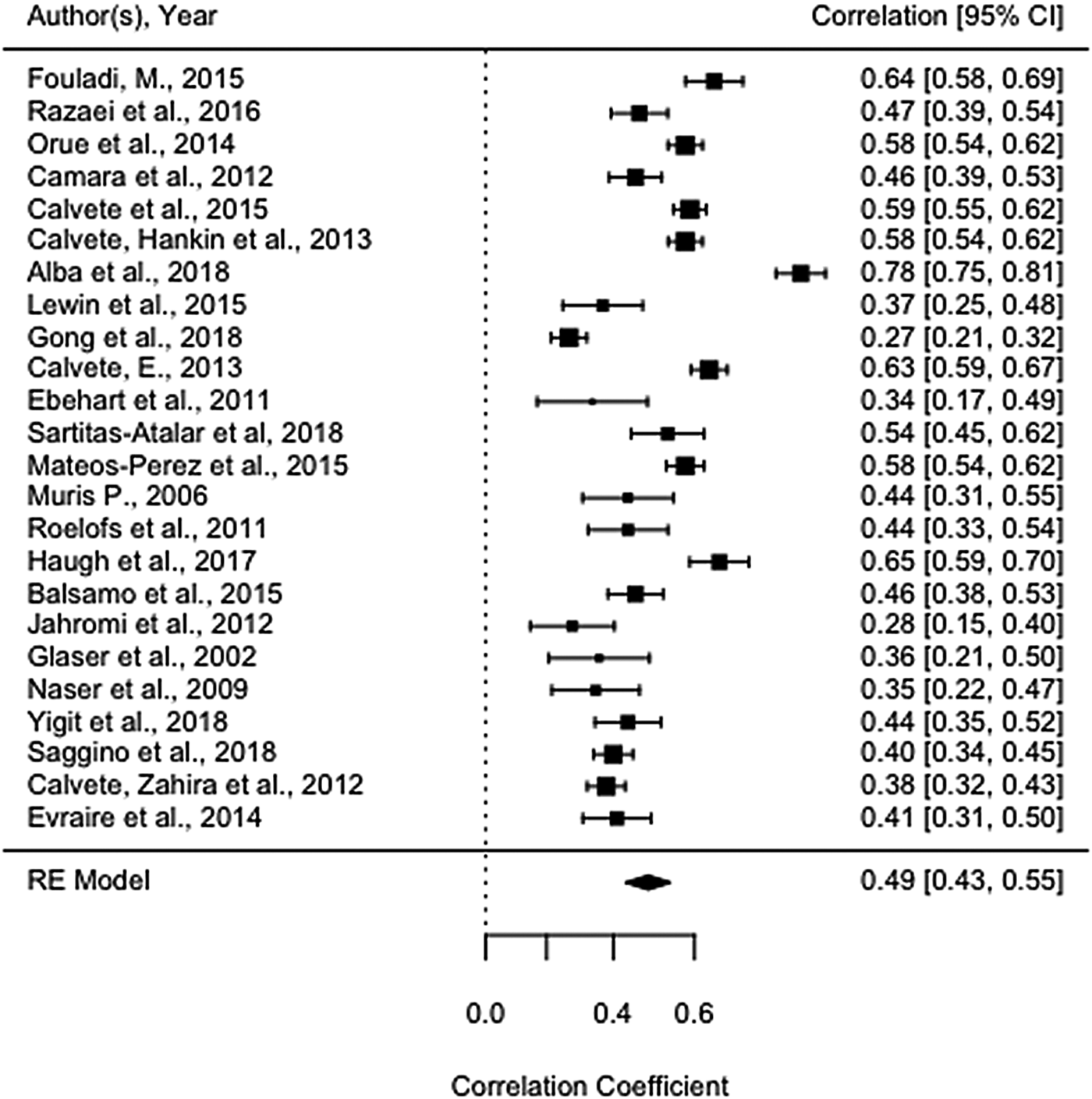
Fig. 5. Forest plot of disconnection/rejection and depression meta-analysis.
Effect size of association between impaired autonomy/performance schemas and depression
The random-effect estimate of association between impaired autonomy/performance schemas and depression was r = 0.47 (95% CI 0.42–0.52, Z = 15.26, p < 0.0001), depicting a moderate relationship between impaired autonomy/performance schemas and depressive symptoms. The overall sample estimate suggested a significant heterogeneity (Q = 284.82, p ≤ 0.0001), with 93% of effect size variance occurring due to between-study variance. No publication bias was observed using the Egger's test (p = 0.15) and rank test of correlation (p = 0.19). Furthermore, age and gender did not have a moderating effect on the meta-analytic estimates [Q (1) = 1.81, p = 0.18 and Q (1) = 0.36, p = 0.55, respectively; see Fig. 6 below for details].

Fig. 6. Forest plot of impaired autonomy/performance and depression meta-analysis.
Effect size estimate of association between other-directedness schemas and depression
As shown in Fig. 7, a medium effect size of r = 0.40 (95% CI 0.32–0.47, Z = 9.76, p < 0.0001) was estimated between other-directed schemas and depressive symptoms in a heterogeneous sample of 17 studies, reporting significant results for heterogeneity (Q = 393.05, p ≤ 0.0001). The I 2 statistics showed that 95% of study variance was attributed to high variance occurring due to the actual difference between studies rather than within-study variance. The rank correlation test (p = 0.48) and Egger's regression test (p = 0.22) suggest that the findings were not influenced by publication bias. However, the moderation analysis reveals a significant impact of mean age on the relationship between other-directed schemas and depressive symptoms [Q (1) = 5.09, p = 0.02], proposing that the association between other-directed schema and depression increases with increasing age. Furthermore, gender also had a significant impact on the associations between other-directedness schemas and depressive symptoms, such that females with higher other-directed schemas were shown to have more depressive symptoms [Q (1) = 6.25, p = 0.01].

Fig. 7. Forest plot of other-directedness and depression meta-analysis.
Effect size estimate of association between impaired limit domain and depression
A meta-analytical result of 13 studies reported a medium effect size of relationship between impaired limits domain with depressive symptoms (r = 0.36, 95% CI 0.30–0.41, Z = 11.80, p < 0.0001). The results of heterogeneity depict a significantly heterogeneous nature of included studies with Q = 64.90 (p ≤ 0.0001). The value of I 2 proposes that 79% of effect size variance is associated with the actual variance occurring between studies. The non-significant results of Egger's test (p = 0.34) and rank correlation test (p = 0.95) suggest the absence of publication bias. Neither age [Q (1) = 0.03, p = 0.87] nor gender [Q (1) = 0.06, p = 0.81] moderate the relationship between impaired limit schemas and depressive symptoms. See Fig. 8 for details.

Fig. 8. Forest plot of impaired limits and depression meta-analysis.
Effect size estimate of association between hypervigilance schemas and depression
Based on 11 studies, a significant relationship with a medium effect size (r = 0.31; 95% CI 0.25–0.38, Z = 8.87, p < 0.0001) was found between hypervigilance schemas and depressive symptoms (see Fig. 9). The studies included were estimated to have a significant heterogeneity (Q = 62.09, p ≤ 0.0001) and 83% of effect size variance accredited to actual sample variance between studies. The results were not influenced by publication bias (Egger's test p = 0.36, rank correlation test p = 0.35), or moderation effects of age [Q (1) = 0.05, p = 0.83] and gender [Q (1) = 3.72, p = 0.06].

Fig. 9. Forest plot of hyper-vigilance and depression meta-analysis.
Meta-analytical results for adolescents v. young adults
Finally, meta-analytical results were generated using random-effect model to estimate the effect size of the associations between EMS, schema domains and depression in adolescents and young adults separately to help clinicians have a better understanding of EMS role in specific developmental period. The overall effect size estimate depicts a slightly stronger association between EMS and depression in adolescents (r = 0.64) compared to young adults (r = 0.50) (see Table 4).
Table 4. Meta-analysis of relationship between domains of early maladaptive schemas and anxiety/depression (random-effect model)

n, number of studies; N, total sample size; mean effect size r, average uncorrected correlation; 95% CI, lower and upper limits of 95% confidence interval for uncorrected correlations; p value, statistical significance; Q, heterogeneity; I 2, study variance.
Quality assessment
All the included studies were either based on cross-sectional (k = 15) or longitudinal (k = 9) research design. None had an experimental design; therefore, the criteria for measuring baseline differences were not applicable. All the included studies provided adequate description about selection criteria and demographic information of the participant's sample. However, only one study provided details about power calculation, rendering it difficult to determine if the included samples were sufficiently powered. All studies employed a validated and reliable measure to assess EMS (YSQ-L, YSQ-S3, YSQ-SF, YSQ-A) and depression (BDI-I or BDI-II, CES-D, DASS, CDI; see Table 1). The included studies have mixed quality in terms of controlling for confounding variable during analysis, with 16 shown to have taken adequate measures while six provided only partial details for this. Two of the included studies did not take into account any confounding variables. Overall the quality assessment shows that all the studies fall in moderate to high quality with none of the included study falling in low-quality category. Seven of the included studies are of moderate quality having 60–79% of quality category, posing a moderate risk of bias. Seventeen studies are of high quality with ratings falling between 80% and 100% category, indicating a low risk of bias (see Table 2).
Discussion
The current meta-analysis examined the association between EMS, different domains of EMS and depression among adolescents and young adults using separate meta-analytical models for each schema domain. Findings from 24 studies revealed that EMS were positively correlated with depression with large effect sizes, indicating that adolescents and young adults with predominately active EMS are on a greater verge of experiencing depressive symptoms. These findings are consistent with the theoretical framework proposed by Young (Reference Young1990; Reference Young1999) as well as empirical studies based on adolescents and adult population (Cooper, Rose, & Turner, Reference Cooper, Rose and Turner2005; Glaser et al., Reference Glaser, Campbell, Calhoun, Bates and Petrocelli2002; Harris & Curtin, Reference Harris and Curtin2002; McGinn, Cukor, & Sanderson, Reference McGinn, Cukor and Sanderson2005; Welburn et al., Reference Welburn, Coristine, Dagg, Pontefract and Jordan2002).
Young et al. (Reference Young, Klosko and Weishaar2003) indicated the association of different maladaptive schemas with enduring psychological symptoms, with similar schemas associated with multiple psychological conditions. Therefore, it is important to identify the specific schemas or schema content associated with depressive symptoms to enhance treatment outcome by targeting specific maladaptive schemas. To enhance the understanding of specific schemas, separate meta-analyses were conducted with each schema domain to assess their associations with depressive symptoms. Our results showed that the schema domains of disconnection/rejection, impaired autonomy/performance and other-directedness have moderately stronger associations with depressive symptoms, whereas hypervigilance and impaired limits have comparatively weaker associations with depression. Consistent with Young's model (Young et al., Reference Young, Klosko and Weishaar2003), these findings suggest that adolescents and young adults with greater depressive tendencies are likely to have their thoughts or feelings revolving around these three strongly associated maladaptive schemas. According to schema theory, disconnection rejection schemas usually involve beliefs related to insufficient acceptance and security with a lack of stable relationships with significant figures. Impaired autonomy and performance schemas involve beliefs associated with impaired ability to survive and cope with stressful and catastrophic situations. Finally, other-directed schemas involve giving excessive prominence to other people at the expense of one's own need (Young et al., Reference Young, Klosko and Weishaar2003).
These findings are consistent with other theoretical frameworks that explain the role of cognitive vulnerabilities in developing and maintaining depressive symptomology. The hopelessness theory proposed that individuals with greater depressive tendencies are involved in maladaptive inferences about stressful situations, catastrophizing stressors and drawing negative conclusion about self (Abela & Sarin, Reference Abela and Sarin2002; Abramson, Alloy, & Metalsky, Reference Abramson, Alloy, Metalsky and Alloy1988). Similarly, Beck (Reference Beck1967) suggests the presence of a negative triad that includes negative interpretation about the self, world and future among depressive individuals (Abramson et al., Reference Abramson, Alloy, Hankin, Haefell, MacCoon, Gibb, Gotlib and Hammen2002). These findings also echo interpersonal theories that emphasize the role of one's negative view of relationship with others in the development of depression (Abela et al., Reference Abela, Hankin, Haigh, Adams, Vinokuroff and Trayhern2005) and empirical findings suggesting that individuals with symptoms of or risk for depression are more likely to have low self-worth, pessimistic expectations about interpersonal relations and belief that they are unworthy of healthy social relationships (Rudolph & Clark, Reference Rudolph and Clark2001).
Age did not moderate the association between EMS, different schema domains and depression, suggesting a similar role of maladaptive schemas in the development of depression among adolescents and young adults. It also provides the basis for considering adolescence and young adulthood as a continuous transitional process marked with similar development changes. There exists no previous evidence comparing the role of EMS with depression in adolescents and young adults. Therefore, future research should focus on exploring the role of EMS in adolescent and young adults using a comparative research design.
Similarly, results suggest that gender did not moderate the association between maladaptive schemas, schema domains and depressive symptoms. There is a limited evidence-base suggesting the absence of gender differences in experiencing EMS. Colman (Reference Colman2010) found no gender differences in a sample of 82 college students with predominant activated EMS. However, the current findings are inconsistent with previous evidence that found significantly stronger associations between EMS and depressive symptoms among adolescent girls (Calvete & Cardeñoso, Reference Calvete and Cardeñoso2005) and female young adults (Camara & Calvete, Reference Camara and Calvete2012; Welburn et al., Reference Welburn, Coristine, Dagg, Pontefract and Jordan2002). One possible explanation is the skewed nature of gender data in most of the studies, with two studies (Eberhart et al., Reference Eberhart, Auerbach, Bigda-Peyton and Abela2011; Fouladi, Reference Fouladi2015) based on a female-only sample. In addition, the results were based on biologically assigned sexual categories. In future, it would be interesting to take into account the gender differences based on individuals' self-identified gender orientations.
Clinical implications
Adolescence and young adulthood are critical developmental stages with heightened vulnerability for depression, and therefore offer a unique window of opportunity to foster psychological health and well-being through early mental health interventions (Xavier, Cunha, & Pinto Gouveia, Reference Xavier, Cunha and Pinto Gouveia2015). Earlier identification, interventions and promotion of mental health can substantially prevent future distress and social cost (Arango et al., Reference Arango, Díaz-Caneja, McGorry, Rapoport, Sommer, Vorstman and Carpenter2018). Findings of the current meta-analysis suggest that it would be helpful for clinicians to identify specific maladaptive schemas contributing to depression, to have a better understanding of underlying cognitive processes and in turn take a targeted, individualized approach to promoting psychological health, well-being and resilience in adolescents and young adults. The impact of depression through the life-course is cumulative. Looking for early markers could provide valuable opportunities for early intervention. Screening for the onset of EMS provides the potential for early identification and diverting from the adult trajectory towards depression. General practitioners and local medical professional may be in the best place to develop expertise in recognizing precursors during routine early childhood check-ups, vaccines and assessment of developmental milestones. In addition, our findings also encourage clinicians to focus more on the content of three significant schema domains of disconnection/rejection, impaired autonomy/performance and other-directedness in particular. Identifying specific schemas will help clinicians in devising a targeted treatment plan suited for each individual's need.
Limitations and areas for future research
The current meta-analysis has several limitations. The inclusion criteria for searches of review articles were limited only to peer-reviewed journal articles, excluding the grey literature such as unpublished theses, abstracts and conference proceedings. This ensured the presence of high-quality peer-reviewed articles but could also induce an upward bias in findings. It is encouraging to note however that no significant publication bias was found. Furthermore, a large number of studies (n = 25) were excluded because of publication in a language other than English. Time and cost constraints have made it impossible to have these papers translated, which may make it difficult to generalize our results. Finally, the included studies were based mostly on a cross-sectional research design describing only the magnitude of the associations between EMS and depression, rather than explaining causal interactions.
Future systematic reviews and meta-analysis should include grey literature and literature published in a language other than English to enhance the generalizability of findings.
Conclusion
Adolescents and young adults with significantly activated maladaptive schemas are likely to experience greater symptoms of depression. Further, the findings suggest that schemas associated with disconnection/rejection, impaired autonomy and other-directed schema content are likely to contribute more towards depressive tendencies.
Supplementary material
The supplementary material for this article can be found at https://doi.org/10.1017/S0033291721001458.
Acknowledgements
We acknowledge and thank Unaiza Iqbal for her contribution as a second reviewer for carrying out the quality assessment of studies included in this review.
Author contributions
We confirm that the manuscript has been read and approved by all named authors and that there are no other persons who satisfied the criteria for authorship but are not listed. We further confirm that the order of the authors listed in the manuscript has been approved by all of us.
Financial support
A.T. completed this work while enrolled on Ph.D. in Clinical Psychology at the University of Edinburgh, funded by the Higher Education Commission (HEC) of Pakistan. The funder had no involvement in carrying out this review.
Conflict of interest
None.