Introduction
Theories of psychopathology have emphasized the sources of common and specific variance among disorders, clustering disorders into internalizing and externalizing domains (Weiss et al., Reference Weiss, Susser and Catron1998; Lilienfeld, Reference Lilienfeld2003; Kendler and Myers, Reference Kendler and Myers2014). The internalizing domain comprises anxiety disorders, depressive disorders, post-traumatic stress disorder (PTSD) and other related conditions (Weiss et al., Reference Weiss, Susser and Catron1998; Caspi et al., Reference Caspi, Houts, Belsky, Goldman-Mellor, Harrington, Israel, Meier, Ramrakha, Shalev, Poulton and Moffitt2014; Kotov et al., Reference Kotov, Krueger, Watson, Achenbach, Althoff, Bagby and Zimmerman2017). Similarly, the externalizing domain comprises substance use disorders, antisocial personality disorder, conduct disorder, and attention-deficit/hyperactivity disorder (Weiss et al., Reference Weiss, Susser and Catron1998; Kotov et al., Reference Kotov, Krueger, Watson, Achenbach, Althoff, Bagby and Zimmerman2017). In contrast to narrowband-specific sources of variance that capture the features that are unique to particular disorders (e.g. physiological arousal in anxiety), internalizing and externalizing latent factors have been useful in capturing the shared variance among disorders in these two broad domains. These factors tend to explain much of the associations among individual disorders, as well as their associations with cognition and personality (Krueger et al., Reference Krueger, Hicks, Patrick, Carlson, Iacono and McGue2002; Whiteside and Lynam, Reference Whiteside and Lynam2003; Young et al., Reference Young, Friedman, Miyake, Willcutt, Corley, Haberstick and Hewitt2009; Hink et al., Reference Hink, Rhee, Corley, Cosgrove, Hewitt, Schulz-Heik, Lahey and Waldman2013; Tackett et al., Reference Tackett, Lahey, van Hulle, Waldman, Krueger and Rathouz2013; Caspi et al., Reference Caspi, Houts, Belsky, Goldman-Mellor, Harrington, Israel, Meier, Ramrakha, Shalev, Poulton and Moffitt2014; Gustavson et al., Reference Gustavson, du Pont, Hatoum, Rhee, Kremen, Hewitt and Friedman2017).
Internalizing and externalizing factors have historically been studied in children and adolescents (Weiss et al., Reference Weiss, Susser and Catron1998; Krueger et al., Reference Krueger, Hicks, Patrick, Carlson, Iacono and McGue2002; Lilienfeld, Reference Lilienfeld2003), especially in twin studies that inform their genetic and environmental architecture (King et al., Reference King, Iacono and McGue2004; Blonigen et al., Reference Blonigen, Hicks, Krueger, Patrick and Iacono2005; Hink et al., Reference Hink, Rhee, Corley, Cosgrove, Hewitt, Schulz-Heik, Lahey and Waldman2013; Tackett et al., Reference Tackett, Lahey, van Hulle, Waldman, Krueger and Rathouz2013; Gustavson et al., Reference Gustavson, du Pont, Hatoum, Rhee, Kremen, Hewitt and Friedman2017). Genetic influences continue to account for the commonality between disorders at a pairwise level well into adulthood both within the internalizing (Chantarujikapong et al., Reference Chantarujikapong, Scherrer, Xian, Eisen, Lyons, Goldberg, Tsuang and True2001; Koenen et al., Reference Koenen, Fu, Ertel, Lyons, Eisen, True, Goldberg and Tsuang2008) and the externalizing domains (True et al., Reference True, Heath, Scherrer, Xian, Lin, Eisen, Lyons, Goldberg and Tsuang1999a, Reference True, Xian, Scherrer, Madden, Bucholz, Heath, Eisen, Lyons, Goldberg and Tsuang1999b), and across the two domains (Xian et al., Reference Xian, Chantarujikapong, Scherrer, Eisen, Lyons, Goldberg, Tsuang and True2000; Fu et al., Reference Fu, Heath, Bucholz, Lyons, Tsuang, True and Eisen2007). However, when middle-aged or older adults are included in studies of internalizing and externalizing psychopathology, they tend to have heterogeneous age ranges that included adolescents and/or young adults (Krueger, Reference Krueger1999; Kendler et al., Reference Kendler, Prescott, Myers and Neale2003; Eaton et al., Reference Eaton, Krueger, Keyes, Skodol, Markon, Grant and Hasin2011; Kendler and Myers, Reference Kendler and Myers2014). Thus, it will be useful to demonstrate the genetic and environmental structure of internalizing and externalizing factors in middle age within a sample with a more homogenous age range.
Although genetic influences on individual disorders are relatively stable throughout the lifespan (Eley et al., Reference Eley, Lichtenstein and Moffitt2003; Bergen et al., Reference Bergen, Gardner and Kendler2007; Bornovalova et al., Reference Bornovalova, Hicks, Iacono and McGue2009; Petkus et al., Reference Petkus, Gatz, Reynolds, Kremen and Wetherell2016), studies have not yet established the stability of internalizing and externalizing psychopathology across the lifespan. Because genetic influences on both psychopathology factors account for much of the genetic variance in individual disorders (e.g. Tackett et al., Reference Tackett, Lahey, van Hulle, Waldman, Krueger and Rathouz2013; Kendler and Myers, Reference Kendler and Myers2014; Gustavson et al., Reference Gustavson, du Pont, Hatoum, Rhee, Kremen, Hewitt and Friedman2017), internalizing and externalizing factors also likely demonstrate at least moderate genetic stability over time. Furthermore, the longitudinal studies on individual disorders noted above focus on early life (ages 0–30) or late life (> age 60), so it is still somewhat unclear the extent to which genetic influences on psychopathology demonstrate stability in midlife.
In the current study, we began to address these issues by utilizing data from a large study of middle-aged male twins. Participants completed diagnostic interviews of psychopathology symptoms in early middle age (~age 41) as part of the Harvard Drug Study of Substance Abuse (HDS; Tsuang et al., Reference Tsuang, Bar, Harley and Lyons2001). These twins also completed self-reported measures of relevant psychopathology symptoms at mean age 56 and/or 62 as part of the Vietnam Era Twin Study of Aging (VETSA), including measures of anxiety symptoms, depression symptoms, PTSD symptoms, and aggression (Kremen et al., Reference Kremen, Thompson-Brenner, Leung, Grant, Franz, Eisen, Jacobson, Boake and Lyons2006, Reference Kremen, Franz and Lyons2013a, Reference Kremen, Franz and Lyons2013b). They also answered basic questions about their current tobacco and alcohol use. We tested the hypothesis that latent internalizing and externalizing psychopathology factors (at age 41) would predict similar latent factors capturing internalizing and externalizing symptoms/behaviors in late middle age, and that much of this stability would be explained by genetic influences. Such evidence would be consistent with recent findings that genetic influences on psychiatric disorders are similar to those on quantitative variation of milder versions those same disorders (e.g. anxiety disorders v. trait anxiety) in the general population (for review, see Martin et al., Reference Martin, Taylor and Lichtenstein2018), but would extend these findings by demonstrating that there is also longitudinal stability in this covariance.
Method
Subjects
Analyses were based on 1484 individual male twins [433 monozygotic (MZ) twin pairs, 308 dizygotic (DZ) twin pairs, 10 unpaired twins] from the Vietnam Era Twin Registry. All of the men underwent psychiatric diagnostic interviews in early middle age as part of the HDS (M = 41.29 years, s.d. = 2.42) and participated in at least one of the two waves of the longitudinal VETSA project at average age 56 (N = 1291, M = 55.90 years, s.d. = 2.44) or average age 62 (N = 1207, M = 61.72 years, s.d. = 2.45). Most twins completed both waves of VETSA (N = 1014).
All VETSA participants were recruited randomly from the HDS and were not selected for history of any diagnosis. The only inclusion criteria for VETSA 1 was that both twins must have been between ages 51 and 59 at the time of recruitment, and that both twins in a pair agreed to participate in the study. All twins were invited to participate in the second wave of VETSA regardless of the participation of their co-twin. Individuals all served in the United States military at some time between 1965 and 1975 and are generally representative of American men in their age group with respect to health and lifestyle characteristics (Kremen et al., Reference Kremen, Thompson-Brenner, Leung, Grant, Franz, Eisen, Jacobson, Boake and Lyons2006; Schoenborn and Heyman, Reference Schoenborn and Heyman2009). Nearly 80% did not serve in combat or in Vietnam, and lifetime PTSD rates (7.3%) were similar to that of the general population (Kilpatrick et al., Reference Kilpatrick, Resnick, Milanak, Miller, Keyes and Friedman2013; see online Supplementary Table S1 for lifetime prevalence of other disorders based on DSM-III-R criteria at mean age 41). Table 1 displays basic demographic characteristics of the sample.
Table 1. Demographic characteristics of the sample

VETSA, Vietnam Era Twin Study of Aging.
Note: Lifetime education was the number of years of school completed. Lifetime occupation was based on the Hollingshead Four-Factor index (Hollingshead, Reference Hollingshead1975), from 0 (unemployed) to 9 (major professionals). A mean of 5.47 corresponds to a clerical worker, sales worker, technician, or semiprofessional.
Measures
Internalizing and externalizing psychopathology (Age 41)
Psychopathology symptoms were assessed using structured interviews based on the Diagnostic Interview Schedule, Version III Revised (DIS-3R; Robins et al., Reference Robins, Helzer, Cottler and Goldring1988), administered by telephone. Questions from the DIS-3R assess mental disorders according to the revised third edition of the Diagnostic and Statistical Manual of Mental Disorders (DSM-III-R; American Psychiatric Association, 1987). All measures used in these analyses were based on the count of lifetime symptoms, including both dependence and abuse symptoms.Footnote †Footnote 1
Because all summed symptom counts were positively skewed, measures were binned into ordinal variables for each disorder, thereby creating polychotomous measures. This method produces unbiased estimates of censored data in twin models, and is preferred to transformations in liability-threshold models such as those examined here (Derks et al., Reference Derks, Dolan and Boomsma2004). Bins were selected prior to analyses based on three a priori considerations, similar to our previous work using a different twin sample (Gustavson et al., Reference Gustavson, du Pont, Hatoum, Rhee, Kremen, Hewitt and Friedman2017): (i) equalizing the n per bin as much as possible, (ii) maximizing the number of bins for each trait (i.e. to preserve as much variance as possible), and (iii) attempting to have at least 150–200 individuals per bin (i.e. to prevent empty bins after subdividing the sample based on twin pair and zygosity). The binning procedure for each disorder is described here and displayed in online Supplementary Table S2, but more information on the unbinned variables can be found in the supplement (online Supplementary Table S1).
Internalizing psychopathology was assessed based on symptoms of generalized anxiety disorder (GAD), major depressive disorder (MDD), and post-traumatic stress disorder (PTSD)Footnote 2. For GAD, 3 bins were created based on symptom endorsement: 0 symptoms (n = 1199), 1–6 symptoms (n = 132), and 7 or more symptoms (n = 151). For MDD, 5 bins were created: 0 symptoms (n = 507), 1 symptom (n = 331), 2 symptoms (n = 213), 3–4 symptoms (n = 218), and 5 or more symptoms (n = 213). For PTSD, 4 bins were created: 0 symptoms (n = 879), 1–3 symptoms (n = 220), 4–7 symptoms (n = 203), and 8 or more symptoms (n = 174). PTSD symptoms were based on the number of unique symptoms reported across three events.
Externalizing psychopathology was based on symptoms for tobacco use disorder, alcohol use disorder, cannabis use disorder, and antisocial personality disorder (ASPD). For tobacco use disorder, 5 bins were created: 0 symptoms (n = 513), 1–2 symptoms (n = 263), 3 symptoms (n = 234), 4 symptoms (n = 215), and 5 or more symptoms (n = 259). For alcohol use disorder, 5 bins were created: 0 symptoms (n = 477), 1 symptom (n = 228), 2 symptoms (n = 233), 3–4 symptoms (n = 318), and 5 or more symptoms (n = 224). For cannabis use disorder, 3 bins were created: 0 symptoms (n = 1166), 1 symptom (n = 131), and 2 or more symptoms (n = 182). For ASPD, 4 bins were created: 0 symptoms (n = 438), 1 symptom (n = 399), 2 symptoms (n = 293), and 3 or more symptoms (n = 351).
Internalizing symptoms (Age 56 and 62)
At mean age 56, self-reported internalizing symptoms were assessed using three scales: the 20-item Center for Epidemiologic Studies – Depression scale (CES-D; Radloff, Reference Radloff1977), the stress reaction subscale of the Multidimensional Personality Questionnaire factor-form NZ (MPQ; Tellegen, Reference Tellegen, Tuma and Maser1985, Krueger et al., Reference Krueger, Caspi, Moffitt, Silva and McGee1996), and one item related to anxiety in the Short-Form Health Survey (Have you been a very nervous person? SF-36; Ware and Sherbourne, Reference Ware and Sherbourne1992).
At mean age 62, self-reported internalizing symptoms were assessed using three scales: the CES-D, the 20-item trait form of the State-Trait Anxiety Inventory (STAI; Spielberger, Reference Spielberger1983),Footnote 3 and the 17-item self-report DSM-IV-based PTSD Checklist civilian version (PCL; Weathers et al., Reference Weathers, Litz, Herman, Huska and Keane1993). At both waves, all measures were log transformed to improve the distributional characteristics.
Externalizing symptoms/behaviors (Age 56)
At mean age 56, externalizing symptoms/behaviors were assessed with four measures: the Aggression subscale and Constraint factors of the MPQ, tobacco use, and alcohol consumption. The MPQ aggression subscale was included to capture antisocial tendencies and the reverse-scored MPQ constraint factor (including control, harm avoidance, and traditionalism subscales) was included to assess behavior disinhibition, similar to previous work (Krueger et al., Reference Krueger, Hicks, Patrick, Carlson, Iacono and McGue2002). Tobacco use was a categorical variable in which individuals were given a score of 1 if they answered yes to the question ‘Do you smoke cigarettes now?’ or answered yes to similar questions for cigars, pipe tobacco, or chewing tobacco (n = 385; see supplemental method for more information). Otherwise, they were given a score of 0 (n = 906). Alcohol use was based on the total number of alcoholic beverages reported in the past two weeks, binned into 5 bins: no drinks (n = 437), 7 or less drinks (n = 127), 8–14 drinks (n = 234), 14–28 drinks (n = 215), and >28 drinks (n = 233). We were unable to create a measure of externalizing symptoms/behaviors at age 62 because the MPQ was not assessed at this wave and there were no other comparable measures of personality (e.g. aggression, antisocial behavior). Although tobacco use and alcohol consumption data were available, the latent factor would not be identified with only two indicators.
Data analysis
Analyses were conducted using Mplus Version 7.2 (Muthén and Muthén, Reference Muthén and Muthén2010–2012). For all analyses involving polychotomized symptom count data, we fit liability-threshold models and accounted for missing data using pairwise deletion with weighted least squares, means and variance adjusted (theta parameterization). For models without ordinal variables (Fig. 3c only), we used full-information maximum likelihood. Model fit was determined using chi-square values (χ2), the root-mean-square error of approximation (RMSEA), and the comparative fit index (CFI). Models had good fit if the χ2 value was less than two times the degrees of freedom, RMSEA < 0.06, and CFI > 0.95 (Hu and Bentler, Reference Hu and Bentler1998). Significance of parameters was evaluated with χ2 difference tests using the ‘difftest’ option. We also provide 95% confidence intervals (95% CIs) using standard errors, but when significance tests based on standard errors disagree with χ2 difference tests, we only interpret the latter (see online Supplemental Data Analysis). Figures display standardized factor loadings, but the full unstandardized and standardized output of all models are displayed in the supplement (online Supplementary Tables S5–S9).
Genetically-informative analyses were based on the assumptions of the classical twin design, which decompose variance in a trait (and covariance between traits) into three sets of influences. Additive genetic influences (A) are assumed to correlate at 1.0 for MZ twins and 0.5 for DZ twins because MZ twins share 100% of their alleles identical-by-descent while DZ twins share 50% of their alleles identical-by-descent. Shared environmental influences (C), which are influences that make twins in a pair more similar, are assumed to correlate at 1.0 in both sets of twins. Non-shared environmental influences (E), which are influences that make twins dissimilar, are assumed to be uncorrelated in both sets of twins. We also assume equal means and variances across twin number and zygosity. These standard assumptions for univariate models also apply to the multivariate common pathway models described here, including when phenotypic correlations are decomposed into their genetic (r a), shared environmental (r c), and non-shared environmental components (r e). Genetic and environmental correlations were not estimated directly but were computed from statistically-equivalent Cholesky decompositions.
Results
Descriptive statistics
Descriptive statistics for the self-reported measures at age 56 and 62 are displayed in Table 2. Phenotypic correlations among all variables are displayed in the supplement (online Supplementary Table S3).
Table 2. Descriptive Statistics for Continuous Dependent Measures at Mean Ages 56 and 62

CES, Center for Epidemiologic Studies; SF-36, Short-Form Health Survey; MPQ, Multidimensional Personality Questionnaire; PTSD, Post-traumatic stress disorder.
Note: Reliability was computed with Cronbach's alpha. All questionnaire measures (except MPQ-Aggression and MPQ-Constraint) were log-transformed in all analyses to improve their distributional characteristics (range in skewness: −0.61 to 1.05, range in kurtosis: −0.72 to 0.72). The final two columns display the cross-twin correlations for monozygotic (MZ) and dizygotic (DZ) twins.
Internalizing and externalizing at age 41
The genetic and environmental model of internalizing and externalizing psychopathology symptoms at age 41 is displayed in Fig. 1 (see figure captions for model fit). The estimated phenotypic correlation between the internalizing and externalizing factors was r = 0.65.
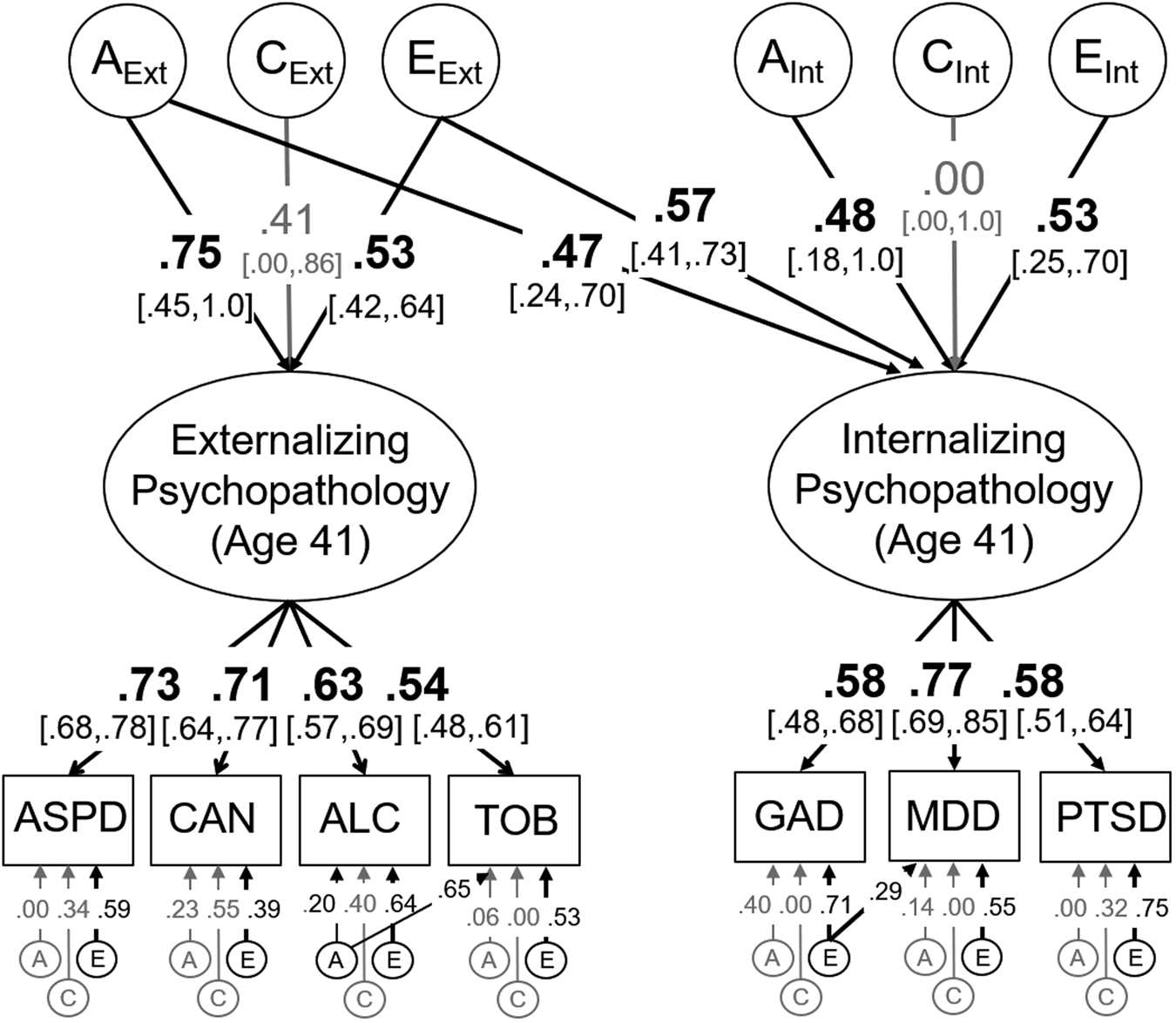
Fig. 1. Model of the genetic (A), shared environmental (C), and non-shared environmental influences (E) on internalizing and externalizing psychopathology at age 41. Individual measures (in rectangles) were binned symptom counts based on dependence and abuse criteria for that disorder. Variance explained by latent variables (ovals) can be computed by squaring these standardized factor loadings whereas genetic correlations (r g) are computed by dividing the genetic covariance between internalizing and externalizing psychopathology (0.75 × 0.47) by the square root of the product of their genetic variances [√(0.752*(0.472 + 0.482)]. Significant factor loadings and correlations are displayed in bold with black lines (p < 0.05) and 95% standard error-based confidence intervals are displayed in brackets. 95% confidence intervals for residual ACEs are displayed in the supplement (online Supplementary Table S4). This model fit the data well: χ2(234) = 258.50, p = 0.130, RMSEA = 0.017, CFI = 0.993. ASPD, antisocial personality disorder; CAN, Cannabis use disorder; ALC, Alcohol use disorder; TOB, Tobacco use disorder; GAD, Generalized anxiety disorder; MDD, Major depressive disorder; PTSD, Post-traumatic stress disorder.
Variance in the internalizing factor was relatively evenly split between genetic influences, a 2 = 0.45, and non-shared environmental influences, e 2 = 0.55, with no evidence for shared environmental influences, c 2 = 0.00. About half the variance in the externalizing psychopathology factor was also explained by genetic influences, a 2 = 0.56, which were strongly correlated with those genetic influences on internalizing psychopathology, r a = 0.70, χ2(1) = 46.76, p < 0.001, 95% CI (0.12–1.0), explaining 54% of their phenotypic correlation. Non-shared environmental influences explained an additional 28% of the variance in the externalizing psychopathology factor and were also correlated with the environmental influences on internalizing psychopathology, r e = 0.77, χ2(1) = 44.87, p < 0.001, 95% CI (0.55–0.99). Shared environmental influences explained the remaining 18% of the variance in externalizing psychopathology, but were nonsignificant, c 2 = 0.17, χ2(1) = 1.06, p = 0.303, likely due to low power to detect these influences even in large samples (Martin et al., Reference Martin, Eaves, Kearsey and Davies1978).
There was little evidence for significant genetic influences on the symptom counts for most individual disorders after accounting for those on the latent factors (range in unique a 2 = .00 to 0.16; see online Supplementary Table S4 for 95% CIs). However, significant residual genetic influences were identified for tobacco use (a 2 = 0.43) and alcohol use disorders (a 2 = 0.04), both χ2(1) > 17.13, ps < 0.001; for other disorders, all χ2(1) < 1.49, ps > 0.222. Residual non-shared environmental influences were significant for all disorders (range e 2 = 0.15 to 0.56). All residual shared environmental influences were nonsignificant, and those influences that were estimated at zero (i.e. GAD, MDD, tobacco use disorder) were fixed to zero in all subsequent models, including the nonsignificant shared environmental influences on the internalizing psychopathology factor, to aid in model convergence. We also included two residual paths in this model (and all subsequent models): a path from the non-shared environmental influences on GAD to those for MDD, χ2(1) = 10.15, p = 0.001, and a path from the genetic influences on alcohol use disorder to tobacco use disorder, χ2(1) = 17.13, p < 0.001.Footnote 4
Prediction of internalizing symptoms at ages 56 and 62 from psychopathology at age 41
Next, we examined the genetic and environmental overlap between internalizing psychopathology at age 41 and later internalizing symptoms. Figure 2a displays the associations between internalizing psychopathology at age 41 and internalizing symptoms at age 56. Figure 2b displays the associations between internalizing psychopathology at age 41 and internalizing symptoms at age 62. Figure 2c displays the associations between internalizing symptoms at age 56 and age 62. We present separate bivariate models for ease of interpretation, but the results were nearly identical in a trivariate model that simultaneously examined all three time-points (online Supplementary Fig. S1).

Fig. 2. Model of the genetic (A), shared environmental (C), and non-shared environmental (E) overlap between internalizing psychopathology at age 42 and self-reported internalizing symptoms at age 56 (a) or age 62 (b). Figure 3c displays the overlap between self-reported internalizing symptoms at age 56 and 62. Variance explained by latent variables (ovals) can be computed by squaring these standardized factor loadings. Measured variables are displayed in rectangles. Significant factor loadings are displayed in bold with black lines (p < 0.05) and 95% standard error-based confidence intervals are displayed in brackets. All models fit the data well: χ2(161) = 161.83, p = 0.467, RMSEA = 0.004, CFI = 0.999 (Fig. 2a); χ2(161) = 160.15, p = 0.504, RMSEA = 0.000 CFI = 1.00 (Fig. 2b); χ2(142) = 220.52, p < 0.001, RMSEA = 0.038, CFI = 0.982 (Fig. 2c). GAD, Generalized anxiety disorder; MDD, Major depressive disorder; PTSD, Post-traumatic stress disorder; CES-D, Center for Epidemiologic Studies – Depression; SF36, Short-Form Health Survey; MPQ, Multidimensional Personality Questionnaire; STAI, State-Trait Anxiety Inventory; PCL, PTSD Checklist.

Fig. 3. Model of the genetic (A), shared environmental (C), and non-shared environmental (E) overlap between externalizing psychopathology at age 41 and an externalizing factor at age 56 based on measures of aggression, constraint, current tobacco use, and current alcohol use. Variance explained by latent variables (ovals) can be computed by squaring these standardized factor loadings. Measured variables are displayed in rectangles. Significant factor loadings are displayed in bold with black lines (p < 0.05) and 95% standard error-based confidence intervals are displayed in brackets. This model fit the data well: χ2(285) = 316.73, p = 0.102, RMSEA = 0.017, CFI = 0.990. ASPD, antisocial personality disorder; Cannabis, Cannabis use disorder; Alcohol, Alcohol use disorder; Tobacco, tobacco use disorder; MPQ Aggr, Multidimensional Personality Questionnaire – Aggression subscale; MPQ Constr, Multidimensional Personality Questionnaire – Constraint subscale.
Genetic influences on internalizing psychopathology at age 41 (A Int) explained 22.1% of the total variance in internalizing symptoms at age 56, χ2(1) = 26.91, p < 0.001. This resulted in a genetic correlation of r a = 0.66, 95% CI (0.33–0.98) that accounted for 61% of the total phenotypic correlation (r = 0.51). Non-shared environmental influences on internalizing psychopathology at age 41 (E Int) explained an additional 6.8% of the variance in symptoms at age 56, r e = 0.43, χ2(1) = 19.56, p < 0.001, 95% CI (0.25–0.60). The remaining variance in internalizing symptoms at age 56 was explained by unique genetic influences (A56), a 2 = 0.29, χ2(1) = 1.73, p = 0.189, unique non-shared environmental influences (E56), e 2 = 0.31, χ2(1) = 24.40, p < 0.001, and unique shared environmental influences (C56), c 2 = 0.10, χ2(1) = 0.40, p = 0.527. Although the unique genetic and shared environmental influences were nonsignificant, both could not be simultaneously removed without a significant reduction in model fit, χ2(2) = 8.96 p = 0.011.
Similar results were observed at age 62 (Fig. 2b). Genetic influences on internalizing psychopathology at age 41 (AInt) explained 21.2% of variance the internalizing symptoms latent factor at age 62, resulting in a genetic correlation of r a = 0.69, χ2(1) = 30.33, p < 0.001, 95% CI (0.34–1.0) that explained 70% of their phenotypic correlation (r = 0.43). Non-shared environmental influences on internalizing psychopathology at age 41 (EInt) explained an additional 2.9% of the variance in internalizing symptoms at age 62, r e = 0.24, χ2(1) = 8.20, p = 0.004, 95% CI (0.08–0.40). The remaining variance was explained by non-shared environmental influences specific to age 62 (E62), e 2 = 0.49, χ2(1) = 35.15, p < 0.001, and nonsignificant genetic influences (A62), a 2 = 0.23, χ2(1) = 1.68, p = 0.195, and shared environmental influences specific to age 62 (C62), c 2 = .03, χ2(1) = 0.05, p = 0.830.
In both models, we examined evidence for stability of residual genetic or environmental influences on individual disorders to later self-reported symptoms (e.g. between MDD at age 41 and CES-D at age 56 in Fig. 2a). Based on these analyses, we included a path from the non-shared environmental influences on PTSD symptoms (age 41) to PTSD symptoms at age 62 (Fig. 2b), χ2(1) = 22.93, p < 0.001. All other paths were nonsignificant, χ2(1) < 1.24, p > 0.265, and were excluded from the final models. Additional analyses displayed in the supplement (online Supplementary Fig. S2) found no evidence that the genetic or environmental influences on externalizing psychopathology (at age 41) were significantly associated with internalizing symptoms at age 56 or age 62 after accounting for its covariance with the internalizing psychopathology at age 41.
As shown in Fig. 2c, despite some measurement differences (i.e. different measures of anxiety, PTSD symptoms only at age 62), the genetic influences on the internalizing symptoms factors were perfectly correlated between ages 56 and 62, r a = 1.0, χ2(1) = 16.37, p < 0.001, 95% CI (0.12–1.0), explaining 59% of the phenotypic correlation (r = 0.78). There was also a moderate non-shared environmental correlation, r e = 0.54, χ2(1) = 99.18, p < 0.001, 95% CI (0.45–0.63), but most of the non-shared environmental variance at age 62 was unique (explaining 37% of its total variance).
Prediction of externalizing symptoms/behaviors at age 56 from psychopathology at age 41
Figure 3 displays a similar model in which externalizing psychopathology at age 41 predicts externalizing symptoms/behaviors at age 56. Although factor loadings for externalizing symptoms/behaviors at age 56 were somewhat low, genetic influences on externalizing psychopathology at age 41 accounted for 72.3% of the variance in externalizing symptoms/behaviors at age 56. This resulted in a perfect genetic correlation, r a = 1.0, χ2(1) = 20.17, p < 0.001, 95% CI (0.37–1.0), that explained 98% of their phenotypic correlation (r = 0.67). There were also some unique non-shared environmental influences on the externalizing symptoms/behaviors factor at age 62, explaining 25.0% of its variance, χ2(1) = 7.14, p < 0.008.
The model displayed in Fig. 3 also included residual genetic and non-shared environmental associations between tobacco use disorder symptoms (age 41) and tobacco use at age 56, explaining 50.4% and 4.0% of the variance in tobacco use at age 56, respectively, both χ2(1) > 6.27, ps < 0.012. Similar paths were tested between alcohol use disorder (age 41) and alcohol use (age 56), but they were nonsignificant, both χ2(1) < 3.73 p > 0.053. Finally, unlike the models of the internalizing factors, additional analyses suggested that the new nonshared environmental influences on externalizing symptom/behaviors at age 56 were explained by environmental influences internalizing psychopathology at age 56, e 2 = 0.25, χ2(1) = 4.53, p = .033, 95% CI (0.10–0.49), even after controlling for externalizing psychopathology at age 41 (online Supplemental Fig. S3). Thus, most – if not all – of the variance in externalizing symptoms/behaviors at age 62 were explained by earlier psychopathology symptoms.
Discussion
We observed considerable genetic and environmental correlations between internalizing and externalizing psychopathology symptoms in these middle-aged adults not selected for psychopathology diagnoses. Both latent factors were also moderately to strongly correlated with self-reported measures of related symptoms and behaviors 15 to 20 years later. Internalizing and externalizing factors have been useful in understanding psychopathology's associations with cognition and personality (Krueger et al., Reference Krueger, Hicks, Patrick, Carlson, Iacono and McGue2002; Hink et al., Reference Hink, Rhee, Corley, Cosgrove, Hewitt, Schulz-Heik, Lahey and Waldman2013; Tackett et al., Reference Tackett, Lahey, van Hulle, Waldman, Krueger and Rathouz2013; Caspi et al., Reference Caspi, Houts, Belsky, Goldman-Mellor, Harrington, Israel, Meier, Ramrakha, Shalev, Poulton and Moffitt2014), but studies have most often focused on children and adolescents, or adult samples with heterogeneous age ranges. Our results demonstrate that these latent factors show strong stability across midlife, even though the symptom/behavior measures at age 56 and 62 differed substantially from the diagnostic interviews in earlier middle age.
Although internalizing and externalizing psychopathology factors were explained roughly equally by genetic and non-shared environmental influences, genetic influences accounted for the majority of their phenotypic stability across the 15- to 20-year interval. Previous work has focused on stability of genetic influences on individual disorders (Eley et al., Reference Eley, Lichtenstein and Moffitt2003; Bergen et al., Reference Bergen, Gardner and Kendler2007; Bornovalova et al., Reference Bornovalova, Hicks, Iacono and McGue2009; Petkus et al., Reference Petkus, Gatz, Reynolds, Kremen and Wetherell2016), but this is the first evidence, to our knowledge, that much of this genetic stability can be attributable to higher-order internalizing and externalizing psychopathology variance (at least, to the extent that the self-reported symptom/behavioral measures and symptom counts from diagnostic interviews tap similar internalizing/externalizing variance). In contrast, there was little evidence that genetic influences specific to individual disorders predicted later symptoms/behaviors (except for tobacco use disorder).
These results are relevant to ongoing genome-wide association tests (GWAS) of psychopathology. GWAS have also demonstrated high genetic overlap among psychiatric disorders (Bulik-Sullivan et al., Reference Bulik-Sullivan, Finucane, Anttila, Gusev, Day, Loh, ReproGen, Duncan, Perry, Patterson, Robinson, Daly, Price and Neale2015; Lo et al., Reference Lo, Hinds, Tung, Franz, Fan, Wang, Smeland, Schork, Holland and Kauppi2017; Duncan et al., Reference Duncan, Ratanatharathorn, Aiello, Almli, Amstadter, Ashley-Koch, Baker, Beckham, Bierut, Bisson, Bradley, Chen, Dalvie, Farrer, Galea, Garrett, Gelernter, Guffanti, Hauser, Johnson, Kessler, Kimbrel, King, Koen, Kranzler, Logue, Maihofer, Martin, Miller, Morey, Nugent, Rice, Ripke, Roberts, Saccone, Smoller, Stein, Stein, Sumner, Uddin, Ursano, Wildman, Yehuda, Zhao, Daly, Liberzon, Ressler, Nievergelt and Koenen2018). Adequately powered GWAS, however, require samples in the tens or hundreds of thousands (Visscher et al., Reference Visscher, Wray, Zhang, Sklar, McCarthy, Brown and Yang2017). Consequently, studies include individuals from across the lifespan. Although these results suggest that there is considerable genetic stability across middle age, to the extent that this stability is not perfect (e.g. for internalizing), this will hurt power to detect genes that are not currently influencing some individuals in the sample (e.g. those who are younger than the majority of the sample). Beyond gene identification, development of polygenic risk scores for internalizing and externalizing psychopathology may be useful in identifying individuals at risk for problems throughout the lifespan.
Finally, non-shared environmental influences also exhibited some stability across this 15- to 20-year span. Just as the genetic influences reflect the combined effects of many independent genetic influences, these non-shared environmental influences likely reflect a multitude of factors, each of which only accounts for a small fraction of the variance (Plomin et al., Reference Plomin, Asbury and Dunn2001; Friedman et al., Reference Friedman, Miyake, Altamirano, Corley, Young, Rhea and Hewitt2016). Nevertheless, it will be useful to identify those that contribute to this persistence of psychopathology symptoms over time, as they may be crucial in future treatment and prevention efforts.
Strengths and limitations
First, the sample comprised only men, so these findings may not generalize to women. Second, this community-based sample was not selected for psychopathology symptoms, and associations may differ in samples with higher rates of symptoms. Third, power to detect shared environmental influences was low, even in this large sample (Martin et al., Reference Martin, Eaves, Kearsey and Davies1978). However, significant shared environmental influences have been observed in some (Gjone and Stevenson, Reference Gjone and Stevenson1997; Burt et al., Reference Burt, McGue, Krueger and Iacono2005), but not all previous work (Krueger et al., Reference Krueger, Hicks, Patrick, Carlson, Iacono and McGue2002; Gustavson et al., Reference Gustavson, du Pont, Hatoum, Rhee, Kremen, Hewitt and Friedman2017).
Fourth, there were limitations to the available measures because VETSA was not designed as a study of psychopathology. For internalizing symptoms, the SF-36 anxiety measure at age 56 was based on only a single questionnaire item and PTSD symptoms were only assessed at age 62. Nevertheless, there were high factor loadings in both cases and the overall results were highly consistent at age 56 and 62 (e.g. as evidenced by the perfect genetic correlation between these internalizing symptom factors in Fig. 2c). The factor loadings on the externalizing symptoms/behaviors factor were small, and it was only possible to examine externalizing symptoms/behaviors at age 56 due to the lack of inclusion of the MPQ at the age 62 assessment. The low factor loadings may reflect the cruder dependent measures (i.e. a dichotomous variable for smoking, only one question about alcohol use in the past two weeks) and the lack of measures of illicit substance use (e.g. cannabis use). Despite these limitations, most of the variance in externalizing symptoms/behaviors at age 56 was explained by externalizing psychopathology at age 41, and the remaining nonshared environmental influences were explained by internalizing psychopathology at age 41.
Finally, and perhaps most importantly, the internalizing and externalizing factors at age 56 and 62 were based on responses to self-reported questionnaires about symptoms and substance use rather than diagnostic interviews. Although this severely limits our ability to draw strong conclusions about the stability of internalizing and externalizing psychopathology over time, we nevertheless observed strong genetic associations between diagnostic interviews and symptoms measures 15 to 20 years later. The genetic and environmental correlations may have been even larger if assessments were identical. However, the fact that we observed strong associations despite measurement differences suggests that these psychopathology factors are highly stable across midlife.
Summary and conclusions
Internalizing and externalizing psychopathology factors in early middle age are explained roughly equally by genetic and non-shared environmental influences. Both factors demonstrate substantial ability to predict self-reported internalizing and externalizing-related problems 15 to 20 years later, with substantial stability of both genetic and non-shared environmental influences. In fact, there was little evidence for new genetic influences on psychopathology symptoms. These findings highlight the persistence of psychopathology-related symptoms and behaviors across adulthood and middle-age.
Supplementary material
The supplementary material for this article can be found at https://doi.org/10.1017/S0033291719001533.
Author ORCIDs
Daniel E. Gustavson, 0000-0002-1470-4928.
Acknowledgements
The content of this manuscript is solely the responsibility of the authors and does not necessarily represent the official views of the NIA/NIH, or the VA. The U.S. Department of Veterans Affairs has provided financial support for the development and maintenance of the Vietnam Era Twin (VET) Registry. Numerous organizations have provided invaluable assistance in the conduct of the VET Registry, including: Department of Defense; National Personnel Records Center, National Archives and Records Administration; Internal Revenue Service; National Opinion Research Center; National Research Council, National Academy of Sciences; the Institute for Survey Research, Temple University. Most importantly, the authors gratefully acknowledge the continued cooperation and participation of the members of the VET Registry and their families as well as the contributions of many staff members and students.
Financial support
This research was supported by Grants R01's AG050595, AG022381, AG054002, AG059329, K08 AG047903 and P01 AG055367 funded by the National Institutes of Health/National Institute on Aging.
Conflict of interest
None.
Ethical standards
The authors assert that all procedures contributing to this work comply with the ethical standards of the relevant national and institutional committees on human experimentation and with the Helsinki Declaration of 1975, as revised in 2008.