Introduction
Attention-deficit/hyperactivity disorder (ADHD) is a childhood onset developmental disorder with profound psychosocial consequences (Barkley and Fischer, Reference Barkley and Fischer2010; Kieling et al., Reference Kieling, Kieling, Rohde, Frick, Moffitt, Nigg, Tannock and Castellanos2010) that often persist into adulthood (Faraone, Reference Faraone2007). In addition to the observed deficits in cognitive performance (McLean et al., Reference McLean, Dowson, Toone, Young, Bazanis, Robbins and Sahakian2004; Banaschewski et al., Reference Banaschewski, Jennen-Steinmetz, Brandeis, Buitelaar, Kuntsi, Poustka, Sergeant, Sonuga-Barke, Frazier-Wood and Albrecht2012; Kofler et al., Reference Kofler, Rapport, Sarver, Raiker, Orban, Friedman and Kolomeyer2013), it is commonly associated with a constellation of symptoms that include emotional lability (Skirrow et al., Reference Skirrow, McLoughlin, Kuntsi and Asherson2009), dyslexia (Germano et al., Reference Germano, Gagliano and Curatolo2010) and mental health problems such as depression, anxiety, addiction and substance use disorders (Fayyad et al., Reference Fayyad, De Graaf, Kessler, Alonso, Angermeyer, Demyttenaere, De Girolamo, Haro, Karam and Lara2007).
One common feature of ADHD symptomatology is an elevated tendency for attentional lapses and reports of uncontrolled mind-wandering, i.e. periods when attention has shifted away from the current task goals. Both inside and outside the laboratory, individuals with ADHD characterise their mind-wandering experiences as excessively frequent, spontaneous and unintentional (Franklin et al., Reference Franklin, Mrazek, Anderson, Johnston, Smallwood, Kingstone and Schooler2014; Seli et al., Reference Seli, Smallwood, Cheyne and Smilek2015), and describe their ongoing cognition as ‘thoughts that are constantly on the go, flitting from one topic to another, and multiple thoughts that appear at the same time’ (Mowlem et al., Reference Mowlem, Skirrow, Reid, Maltezos, Nijjar, Merwood, Barker, Cooper, Kuntsi and Asherson2016). Although converging evidence highlights frequent mind-wandering as a core aspect of ADHD symptomatology, the neural mechanisms that underlie this deficit remain unclear.
Contemporary accounts suggest that mind-wandering is a heterogeneous state that is not the product of a single mental process, but rather one that emerges from a component process architecture in which certain aspects of mental experience are produced by the combination of specific elements of cognition (Smallwood, Reference Smallwood2013; Smallwood and Schooler, Reference Smallwood and Schooler2015; Seli et al., Reference Seli, Carriere, Wammes, Risko, Schacter and Smilek2018). For example, during off-task thought, attention is often focused on mental content generated from internal memory stores. Consequently, individuals, who retrieve information from memory more efficiently, engage in more off-task thought (Smallwood et al., Reference Smallwood, Schooler, Turk, Cunningham, Burns and Macrae2011; Poerio et al., Reference Poerio, Sormaz, Wang, Margulies, Jefferies and Smallwood2017). One possibility, therefore, is that uncontrollable mind-wandering associated with ADHD symptomatology results from excessive tendencies to self-generate mental content from memory.
In addition to being beneficial for psychological functions that require creativity (Baird et al., Reference Baird, Smallwood, Mrazek, Kam, Franklin and Schooler2012) and planning (Medea et al., Reference Medea, Karapanagiotidis, Konishi, Ottaviani, Margulies, Bernasconi, Bernasconi, Bernhardt, Jefferies and Smallwood2016), such excessive generation of off-task thought can also have negative consequences, chiefly because it can lead to errors in task performance (Smallwood et al., Reference Smallwood, Beach, Schooler and Handy2008). Accordingly, neurotypical individuals tend to reduce off-task experiences and increase task-related thoughts when performing more attention demanding tasks – a process known as context regulation (Smallwood and Andrews-Hanna, Reference Smallwood and Andrews-Hanna2013) linked to executive control (Kane et al., Reference Kane, Brown, McVay, Silvia, Myin-Germeys and Kwapil2007; McVay and Kane, Reference McVay and Kane2009; Mrazek et al., Reference Mrazek, Smallwood, Franklin, Chin, Baird and Schooler2012; Smallwood et al., Reference Smallwood, Ruby and Singer2013b; Bernhardt et al., Reference Bernhardt, Smallwood, Tusche, Ruby, Engen, Steinbeis and Singer2014). An alternative perspective, therefore, is that alterations in patterns of ongoing thought emerge in ADHD because of problems in implementing a form of controlled cognition that is appropriate to the specific task context.
In relation to these competing views, recent advances in functional neuroimaging have provided the opportunity to evaluate changes in cognition that is linked to ADHD from a mechanistic perspective. For example, the default mode network (DMN) has been shown to reduce its activity under demanding contexts (Shulman et al., Reference Shulman, Fiez, Corbetta, Buckner, Miezin, Raichle and Petersen1997; Mazoyer et al., Reference Mazoyer, Zago, Mellet, Bricogne, Etard, Houdé, Crivello, Joliot, Petit and Tzourio-Mazoyer2001), and to increase activity during lapses in attention (Eichele et al., Reference Eichele, Debener, Calhoun, Specht, Engel, Hugdahl, von Cramon and Ullsperger2008). Individuals with ADHD, however, are reported to lack such task-evoked activity dynamics – a pattern often taken as evidence of excessive self-generation of mental contents (Liddle et al., Reference Liddle, Hollis, Batty, Groom, Totman, Liotti, Scerif and Liddle2011). In parallel, deficits in executive control (Barkley, Reference Barkley1997), and the dysregulation of associated neural systems such as the frontoparietal network (FPN) (Cortese et al., Reference Cortese, Kelly, Chabernaud, Proal, Di Martino, Milham and Castellanos2012), are both well-documented elements of ADHD.
Based on this evidence, the current study aims to compare and contrast the role of excessive generation of off-task thoughts and impaired context regulation in deficits of ongoing thought with respect to ADHD symptomatology, and to understand whether perturbation in either the connectivity of the DMN or the FPN at rest underpin these problems. For that purpose, we recruited a set of neurotypical participants who completed (i) a battery of questionnaires, including a well-established measure of ADHD, (ii) a laboratory based thought sampling method measuring ongoing cognition and (iii) a resting state functional magnetic resonance imaging (rs-fMRI) scan, which provided a measure of intrinsic neural organisation. A critical element of our design was that the thought sampling method used a behavioural paradigm that alternated between conditions that encouraged participants to restrict their thoughts to task focused information, and those that were more conducive to off-task thoughts (Teasdale et al., Reference Teasdale, Proctor, Lloyd and Baddeley1993; Smallwood et al., Reference Smallwood, Nind and O'Connor2009). This paradigm, therefore, provided the opportunity to index both context regulation (i.e. the ability to increase task-relevant cognition when a task is demanding) and self-generation (i.e. the amount of off-task thought produced throughout the task as a whole) accounts of mind-wandering, allowing us to compare these views in relation to ADHD symptomatology.
Methods
Participants
Ethical approval for this study was obtained from the Department of Psychology and York Neuroimaging Centre, University of York ethics committees. All participants gave informed consent prior to taking part in the experimental assessments. A total of 226 healthy, native English-speaker, right-handed participants were recruited subsequent to the study screening based on the following exclusion criteria: history of psychiatric or neurological illness, severe claustrophobia, anticipated pregnancy or drug use that could alter cognitive functioning. Out of this cohort, 184 participants fully completed the laboratory based thought sampling and ADHD symptomatology questionnaire and were included in the initial analysis (mean = 20.13, s.d. = 2.24, range = 18–31, 121/63 female to male ratio).
Subsequently, all of these participants were scanned with a 9 min long rs-fMRI during wakeful rest. A strict motion correction procedure (described in detail below) was utilised, which resulted in the further exclusion of nine participants, whereas three participants were removed due to problems associated with fMRI scanning. The average age for the final cohort of 172 participants suitable for the fMRI data analysis was 20.12 (s.d. = 2.28, range = 18–31) with a 113/59 female to male ratio.
Thought sampling method
The participants’ ongoing cognition was measured in a 30-min long behavioural paradigm that alternated between blocks of 0-Back and 1-Back conditions that manipulated working memory load (Fig. 1a). Non-target trials in both the 0-Back and 1-Back conditions were identical, consisting of black shapes (circles, squares or triangles) separated by a line, the colour of which signified whether the condition was 0-Back or 1-Back (mean presentation duration = 1050 ms, 200 ms jitter), counterbalanced across individuals. The non-target trials were followed by the presentation of a black fixation cross (mean presentation duration = 1530 ms, 130 ms jitter), and presented in runs of between two and eight trials with a mean of five trials after which a target trial or a multidimensional experience sampling (MDES) probe was presented. In either the 0-Back or 1-Back non-target trials, participants were not required to make a behavioural response.

Fig. 1. Thought sampling procedures and the association between individual variability in thought structures and ADHD symptomatology. (a) A thought sampling procedure was employed during an N-Back paradigm, in which the participants altered between 0-Back (i.e. easy perceptual decisions) and 1-Back (i.e. more difficult, memory based decisions) conditions (Konishi et al., Reference Konishi, McLaren, Engen and Smallwood2015). During the thought probes, participants had to rate their thoughts using a four-point Likert scale from 0 (not at all) to 1 (completely) based on a set of mind-wandering questions. (b) The participants’ ratings were then decomposed into distinct dimensions of thought using PCA and Varimax rotation in order to achieve interpretable results. (c) Individual variation on the identified thought structures were used as explanatory variables in a Pearson correlation assessing their relation to ADHD scores. Out of the four components, the difference in the participants’ detailed thoughts between the 1-Back and 0-Back versions of the N-Back task was negatively related to ADHD scores.
During the target trials, participants were required to make a response, which differed depending on the task condition. In the 0-Back condition, the target trial was a pair of coloured shapes presented on either side of a coloured line with a probe shape in the centre of the screen. Participants had to press a button to indicate whether the central shape matched the shape on the left or right-hand side of the screen. In this condition, there was no need to retain the details of the non-target trials since the response trials could be completed based on the information on the screen, releasing working memory from task relevant information (i.e. easy perceptual decisions).
In the 1-Back condition, the target trial consisted of two coloured question marks presented on either side of a coloured line with a probe shape in the centre of the screen. Participants had to indicate using a button press whether the central shape matched either the shape on the left or right side of the screen on the previous (non-target) trial. Thus, in this condition, participants had to maintain the visuo-spatial array in working memory for each trial and use this information appropriately in the target trials (i.e. more difficult, memory based decisions). This task is presented schematically in Fig. 1a.
The contents of ongoing thought during this N-Back task were measured using MDES. On each occasion that the participants were asked about their thoughts, they rated their answers to the 13 questions presented in Table 1 using a four-point Likert scale that ranged from 0 to 1. Participants always rated their level of task-focus first and then described their thoughts at the moment before the probe on a further 12 questions. MDES probes occurred on a quasi-random basis to minimise the likelihood that participants could anticipate the occurrence of a probe. At the moment of target presentation, there was 20% chance of a MDES probe instead of a target with a maximum of one probe per condition.
Table 1. MDES questions that were presented during the N-Back task. Participants rated their ongoing thoughts on a four-point Likert scale ranging from 0 to 1.

For the purpose of analyses, the ratings on the 13 MDES questions were decomposed into distinct patterns of thought that described the underlying structure of the participants’ responses. Following prior studies (Ruby et al., Reference Ruby, Smallwood, Engen and Singer2013a, Reference Ruby, Smallwood, Sackur and Singer2013b; Medea et al., Reference Medea, Karapanagiotidis, Konishi, Ottaviani, Margulies, Bernasconi, Bernasconi, Bernhardt, Jefferies and Smallwood2016; Smallwood et al., Reference Smallwood, Karapanagiotidis, Ruby, Medea, de Caso, Konishi, Wang, Hallam, Margulies and Jefferies2016; Konishi et al., Reference Konishi, Brown, Battaglini and Smallwood2017) we concatenated the responses of each participant at each probe and in each task into a single matrix and employed a principal component analysis (PCA) for factor reduction with Varimax rotation using SPSS (Version 23) (https://www.ibm.com/products/spss-statistics). We selected a total of four components based on the scree plot illustrated in online Supplementary Fig. S1.
ADHD symptomatology assessment
With the aim of determining individual variability on the ADHD symptomatology of this neurotypical cohort, we administered the widely used and validated Adult ADHD Self-Report Scale (ASRS-v1.1) (Kessler et al., Reference Kessler, Adler, Barkley, Biederman, Conners, Faraone, Greenhill, Jaeger, Secnik, Spencer, Ustun and Zaslavsky2005, Reference Kessler, Adler, Gruber, Sarawate, Spencer and Van Brunt2007). ASRS includes 18 questions that reflect the main criteria for a Diagnostic and Statistical Manual of Mental Disorders (DSM)-IV-TR based ADHD diagnosis. Previous research has indicated that six out of the 18 questions were most predictive of an ADHD diagnosis (Kessler et al., Reference Kessler, Adler, Barkley, Biederman, Conners, Faraone, Greenhill, Jaeger, Secnik, Spencer, Ustun and Zaslavsky2005, Reference Kessler, Adler, Gruber, Sarawate, Spencer and Van Brunt2007; Gray et al., Reference Gray, Woltering, Mawjee and Tannock2014), constituting the Part A of this scale. Average self-reported responses on this subscale of ASRS was thus utilised in our subsequent analyses aimed at investigating the link between ADHD symptomatology, ongoing thoughts and neural organisation at rest.
In addition, based on recent reports suggesting a close link between ADHD symptomatology, depression and dyslexia (Fayyad et al., Reference Fayyad, De Graaf, Kessler, Alonso, Angermeyer, Demyttenaere, De Girolamo, Haro, Karam and Lara2007; Skirrow et al., Reference Skirrow, McLoughlin, Kuntsi and Asherson2009; Germano et al., Reference Germano, Gagliano and Curatolo2010), we have also employed measures of these co-morbid symptoms to be removed as nuisance variables in our analyses. For depression, we used the Center for Epidemiologic Studies Depression Scale (Radloff, Reference Radloff1977); whereas for dyslexia the Dyslexia Adult Checklist was utilised (Smythe and Everatt, Reference Smythe, Everatt and Smythe2001). The correlation between these measures and ADHD scores are provided in the online Supplementary Fig. S2.
MRI data acquisition
All MRI data acquisition was carried out at the York Neuroimaging Centre, York with a 3T GE HDx Excite MRI scanner using an eight-channel phased array head coil. Following a T1-weighted structural scan with 3D fast spoiled gradient echo (TR = 7.8 s, TE = minimum full, flip angle = 20°, matrix size = 256 × 256, 176 slices, voxel size = 1.13 × 1.13 × 1 mm3), a 9-min resting state fMRI scan was carried out using single-shot 2D gradient-echo-planar imaging. The parameters for this sequence were as follows: TR = 3000 ms, TE = minimum full, flip angle = 90°, matrix size = 64 × 64, 60 slices, voxel size = 3 × 3 × 3 mm3, 180 volumes. During resting state scanning, the participants were asked to focus on a fixation cross in the middle of the screen.
MRI data preprocessing
All preprocessing steps for the MRI data were carried out using the SPM software package (Version 12.0) (http://www.fil.ion.ucl.ac.uk/spm/) based on the MATLAB platform (Version 16.a) (https://uk.mathworks.com/products/matlab.html). After removing the first three functional volumes to account for the magnetisation equilibrium, the remaining data were first corrected for motion using 6° of freedom (x, y, z translations and rotations), and adjusted for differences in slice-time. Subsequently, the high-resolution structural image was co-registered to the mean functional image via rigid-body transformation, segmented into grey/white matter and cerebrospinal fluid probability maps, and were spatially normalised to the Montreal Neurological Institute (MNI) space alongside with all functional volumes using the segmented images and a priori templates. This indirect procedure utilises the unified segmentation–normalisation framework, which combines tissue segmentation, bias correction and spatial normalisation in a single unified model (Ashburner and Friston, Reference Ashburner and Friston2005). Finally, all the functional images were smoothed using an 8 mm full width at half maximum (FWHM) Gaussian kernel.
Functional connectivity analysis
MRI data denoising procedures and the subsequent seed-based functional connectivity analyses were carried out using the Conn functional connectivity toolbox (Version 17.f) (https://www.nitrc.org/projects/conn) (Whitfield-Gabrieli and Nieto-Castanon, Reference Whitfield-Gabrieli and Nieto-Castanon2012). With the goal of ensuring that motion and other artefacts did not confound our data, we first employed an extensive motion-correction procedure and denoising steps, comparable with those reported in the literature (Ciric et al., Reference Ciric, Wolf, Power, Roalf, Baum, Ruparel, Shinohara, Elliott, Eickhoff, Davatzikos, Gur, Gur, Bassett and Satterthwaite2017). In addition to the removal of six realignment parameters and their second-order derivatives using the general linear model (Friston et al., Reference Friston, Williams, Howard, Frackowiak and Turner1996), a linear detrending term was applied as well as the CompCor method that removed five principal components of the signal from white matter and cerebrospinal fluid (Behzadi et al., Reference Behzadi, Restom, Liau and Liu2007). Moreover, the volumes affected by motion were identified and scrubbed based on the conservative settings of motion >0.5 mm and global signal change larger than z = 3. A total of nine participants, who had more than 15% of their data affected by motion was excluded from the analysis (Power et al., Reference Power, Mitra, Laumann, Snyder, Schlaggar and Petersen2014). The distribution of average and maximum framewise displacement and global blood oxygen level dependent (BOLD) signal change, as well as the percentage of invalid scans in the final cohort utilised in this study are provided in online Supplementary Fig. S3. Though recent reports suggest the ability of global signal regression to account for head motion, it is also known to introduce spurious anti-correlations, and thus was not utilised in our analysis (Saad et al., Reference Saad, Gotts, Murphy, Chen, Jo, Martin and Cox2012). Finally, a band-pass filter between 0.009 and 0.08 Hz was employed in order to focus on low frequency fluctuations (Fox et al., Reference Fox, Snyder, Vincent, Corbetta, Van Essen and Raichle2005).
Following this procedure, we performed two separate seed-based functional connectivity analyses based on two regions of interest (ROIs) that were selected from the Yeo 7-Network parcellation scheme (Yeo et al., Reference Yeo, Krienen, Sepulcre, Sabuncu, Lashkari, Hollinshead, Roffman, Smoller, Zollei, Polimeni, Fischl, Liu and Buckner2011), namely the frontoparietal and default mode networks. For each participant, average BOLD signal from the binarised seed ROIs described above were correlated with time courses from the rest of the brain with the aim of obtaining individual connectivity maps. Group-level inferences on positive and negative connectivity of the chosen seed ROIs were made based on one-sample t tests. Further linear regressions with FPN as well as DMN connectivity were performed with ADHD symptomatology as the variable of interest, while correcting for dyslexia, depression and the percentage of invalid scans based on the motion scrubbing procedure. All reported clusters were corrected for multiple comparisons using the family wise error (FWE) detection technique at the 0.05 level of significance (uncorrected at the voxel-level, 0.001 level of significance). Beta values representing connectivity of the clusters and the chosen seed ROIs that significantly explained individual variability in ADHD symptomatology, were then extracted for each participant for subsequent statistical analyses.
Statistical analysis
We performed three main analyses to test the relationships between ADHD symptomatology, patterns of ongoing thought and their potential neural mechanisms. First, using a mixed analysis of variance (ANOVA) we examined the relationship between patterns of ongoing thought in the two tasks and variation in ADHD symptomology with the aim of determining if their relationships support either the excessive self-generation, or the impaired context regulation accounts of ADHD, while correcting for depression and dyslexia. Second, we used linear regressions in seed-based functional connectivity analyses to identify how the intrinsic neural organisation varies with natural variation in ADHD symptomatology. For this, we included co-morbid depression, dyslexia scores and subject motion inside the scanner as nuisance variables. Finally, we examined whether patterns of shared variance in association between patterns of neural function and ongoing thought were linked to ADHD using connectivity values (beta weights) obtained from the seed-based analysis and component scores from thought sampling during specific task contexts. In this analysis, we repeated the mixed ANOVA from the first step of our analysis, additionally including the neural changes identified through our functional connectivity analysis as covariates. This last step allowed us to identify potential neural mechanisms that underpin ADHD related changes in patterns of ongoing thought.
Results
Our first analysis examined the relationship between ADHD and patterns of ongoing thought recorded in the laboratory session (Fig. 1a). Following a decomposition of the thought sampling data (Fig. 1b) we conducted a series of repeated measures analysis of covariance (ANCOVAs). In these models, while the dependent measure was the scores for each component of thought, the within participant factor was the task context (0-Back/1-Back) and the between participants factor was ADHD scores (correcting for depression and dyslexia). These analyses first revealed three components of thought that varied across the task conditions: ‘Detailed’ (F 1,182 = 9.24, p = 0.0027), ‘Off-Task’ (F 1,182 = 4.98, p = 0.027) and ‘Modality-Specific (Images/Words)’ (F 1,182 = 5.27, p = 0.023) thoughts. ‘Emotion +’ did not vary across the task conditions. In the 1-Back, thoughts were more detailed [M = 0.11, 95% confidence interval (CI) (−0.208 to 0.002)] than in the 0-Back condition [M = −0.07, 95% CI (0.028 to −0.17)]. Off-Task thoughts were more prominent in the 0-Back [M = 0.14, 95% CI (0.237–0.04)] than in the 1-Back condition [M = −0.15, 95% CI (−0.057 to −0.246)]. Finally, thoughts were more in the form of words in the 1-Back [M = −0.06, 95% CI (0.037 to −0.175)] than in the 0-Back condition [M = 0.07, 95% CI (0.170–0.06)].
We also identified an ADHD by N-Back task condition interaction for the ‘Detailed’ component (F 1,182 = 6.82, p = 0.0098) of the reported thoughts. This interaction indicated that greater ADHD scores were linked to a smaller difference in the level of thought details reported in the 1-Back than in the 0-Back task condition [Pearson r = −0.19, p = 0.0046] (Fig. 1c). Increasing levels of ADHD, therefore, were associated with reports of less detailed experiences in the more demanding 1-Back condition.
Our next analysis explored the association between brain functional connectivity at rest and levels of ADHD symptomology within our sample. After generating spatial maps for each individual that described the associations at the whole brain level for each of the two networks that formed the focus of our investigation (i.e. FPN and DMN) (Fig. 2a, b), we conducted two group-level regressions. In these analyses we included mean centred ADHD scores as a between participant variable of interest, while controlling for potential confounds such as depression, dyslexia and the percentage of motion-based invalid scans.

Fig. 2. Association between differential brain connectivity patterns and ADHD symptomatology. (a) Two binarised masks representing the FPN and DMN from the Yeo 7-Network parcellation scheme were used as ROIs in seed-based functional connectivity analyses. (b) Group-level statistical maps were created that represent the functional connectivity patterns of the chosen FPN and DMN seeds. (c) Whole-brain linear regression analyses revealed that both FPN connectivity to the right lingual gyrus (visual cortex) and DMN connectivity to the right pre/post central gyrus (sensorimotor) were negatively related to the ADHD scores. All results were corrected for depression, dyslexia and the percentage of invalid scans due to motion, and the reported clusters were multiple comparison corrected using FWE correction at the 0.05 significance level (0.001 uncorrected at the voxel level).
These analyses revealed two differences. Higher ADHD scores were linked to reduced correlation between the FPN and a region of right lingual gyrus (visual cortex). In addition, higher ADHD scores were associated with reduced correlation between the DMN and a region of right pre/post-central gyrus (sensorimotor cortex) (Fig. 2c). Increasing levels of ADHD within our sample, therefore, were linked to reduced correlation between transmodal association cortices (DMN and FPN) and unimodal sensorimotor cortices.
Thus far we have identified the correlates of ADHD symptomology with both patterns of ongoing thought and neural organisation. Our final analyses assessed whether these parallel relationships were statistically related. For that purpose, we examined whether the beta weights describing the patterns of neural coupling were linked to variations in the level of ‘Detailed’ thoughts reported by this cohort, either in terms of overall levels of thought, or in terms of how they were expressed in each N-Back task condition. We addressed this question by conducting a repeated measures ANCOVA in which the dependent variable was the PCA loading describing ‘Detailed’ thoughts. The within participant factor was the task condition (i.e. 0/1-Back). The beta weights derived from both functional connectivity analyses, as well as the ADHD scores, were entered as between-participant variables. We also included depression, dyslexia and composite motion scores as covariates of no interest. In these analyses we modelled the main effects for each variable, as well as the two-way interactions between the DMN and FPN beta weights with the ADHD symptoms. This revealed a main effect of the FPN connectivity with respect to overall levels of Detail [F 1,170 = 7.03, p = 0.0088] as well as an ADHD and FPN connectivity interaction [F 1,170 = 5.78, p = 0.017]. This analysis suggests that FPN connectivity with the right ventral visual cortex was linked to more detailed thoughts [Pearson r = 0.34, p = 0.0015] (Fig. 3a), and this association was present only for individuals that scored low on ADHD symptomatology, while no significant association was found for individuals that scored high on ADHD symptomatology [Pearson r = −0.031, p = 0.78] (Fig. 3b).
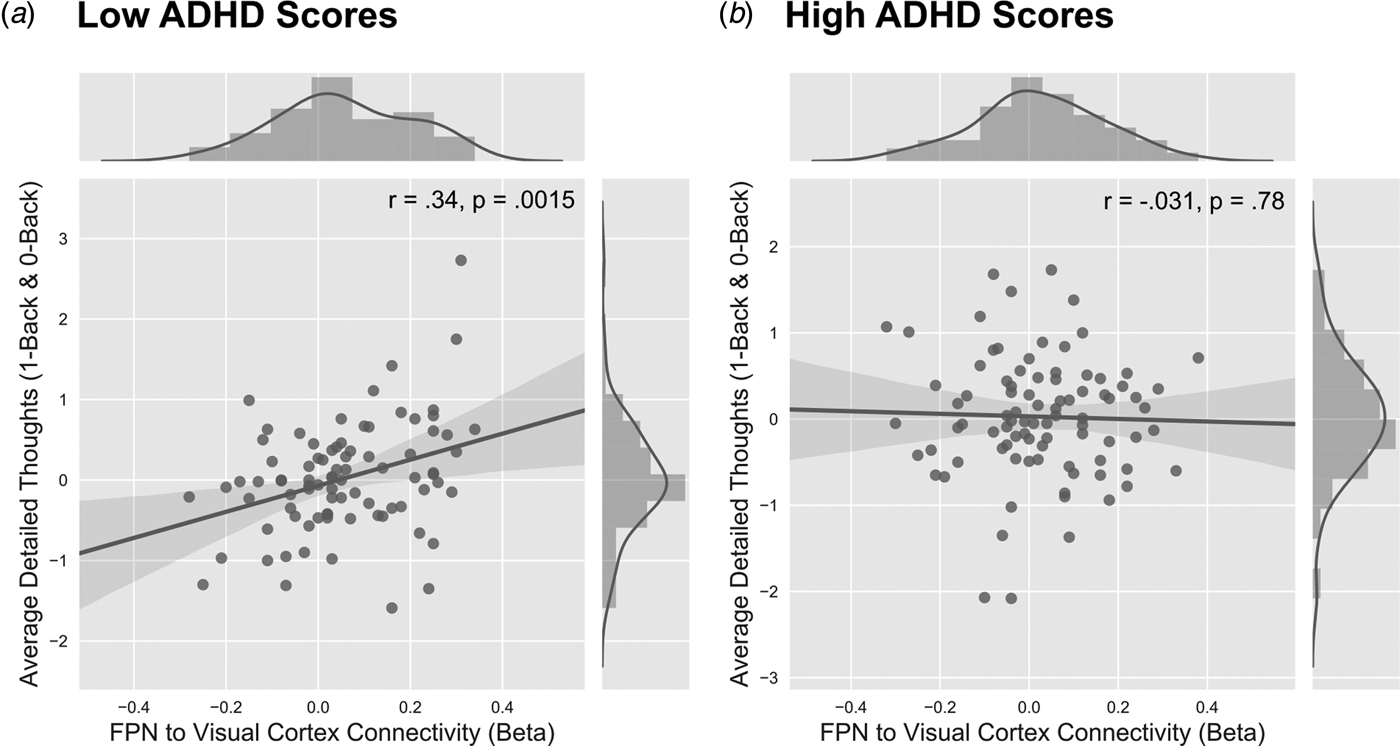
Fig. 3. The link between detailed thoughts and task context in individuals who scored low and high in ADHD scores. The participants were first divided in to low and high ADHD groups based on the median scores on the ADHD scale. (a) Participants who scored low on the ADHD scale showed a significant relationship between overall detailed thoughts in both the 0-Back and 1-Back conditions of the N-Back task. In this group, greater connectivity between the FPN with the right ventral visual cortex correlated with greater detailed thoughts reported across both conditions of the task (r = 0.34, p = 0.0015). (b) However, those who scored high on the ADHD scale did not show a significant relationship between detailed patterns of thought and FPN connectivity to the right ventral visual cortex (r = −0.031, p = 0.78).
Discussion
Our study set out to understand the relationship between individual variability in ADHD symptomology and patterns of ongoing thought in a neurotypical population, focusing on its link to the functional connectivity of two large-scale brain networks at rest – the FPN and DMN, respectively. Our behavioural analysis demonstrated that ADHD symptoms were linked to the level of detail reported in the participants’ patterns of ongoing thought during the more demanding 1-Back condition of the working memory task used in our study. In neural terms, we found that the intrinsic architecture of both the FPNs and DMNs varied with ADHD symptomology, in both cases showing reduced correlation with regions in the unimodal sensorimotor cortices. In particular, higher scores on ADHD were linked to reduced correlation between the FPN and a region of the right ventral visual cortex, while the DMN showed reduced correlation with a region of the right sensorimotor cortex. Importantly, only the connectivity of the FPN was linked to changes in the level of detail in ongoing thought for individuals with generally low ADHD symptoms. Overall, our results are consistent with the hypothesis that ADHD may be linked to deficient adjustment of cognition in line with increasing demands imposed by the environment and that this may partly arise from dysfunctions in the intrinsic organisation of the brain at rest.
Behaviourally, ADHD symptomatology was linked to reduced detail in ongoing thought when participants were actively engaged in the rehearsal of information in working memory. As maintaining a detailed visual representation of task relevant stimuli is an integral part of the 1-Back condition of our task (Owen et al., Reference Owen, McMillan, Laird and Bullmore2005), this pattern of data suggests that ADHD symptoms are linked to deficits in maintaining detailed task representations in working memory. Importantly, this association with ADHD was specific to the more difficult 1-Back task, a pattern consistent with difficulties in regulating ongoing cognition in line with the demands of a specific task context. Notably, in our data we found no evidence that problems in ADHD are associated with increased levels of off-task thinking, which is one common definition of mind-wandering (Christoff et al., Reference Christoff, Irving, Fox, Spreng and Andrews-Hanna2016). Together these observations suggest that ADHD may not simply be associated with excessively thinking about matters unrelated to the here and now, but also to problems associated with the maintenance of detailed cognitive representations of an ongoing task.
In neural terms, we found that FPN connectivity with visual cortex was reduced in participants with higher ADHD scores and this was associated with lower levels of detailed cognition. This result suggests that patterns of ongoing thought linked to ADHD are partly related to the intrinsic architecture of FPN connectivity. Such an interpretation is consistent with evidence showing that the FPN plays a general role across a variety of demanding cognitive tasks (Duncan, Reference Duncan2010; Cole et al., Reference Cole, Reynolds, Power, Repovs, Anticevic and Braver2013). We note, however, that the influence of this network on the changes of ongoing thought linked to ADHD symptoms might also depend on other variables. Behaviourally, the associations between ADHD scores and detailed thoughts were limited to the more difficult 1-Back task condition, while the interaction with the brain was related to lower levels of detail in general. It is possible that this discrepancy arises due to the influence of other variables, such as levels of motivation. In neurotypical individuals, ongoing thought tends to be more deliberately focused on the task when task demands are high and this effect is partly dependent on the individuals’ level of motivation (Seli et al., Reference Seli, Carriere, Wammes, Risko, Schacter and Smilek2018). It is possible, therefore, that the variation in levels of motivation to focus on the task in the non-demanding 0-Back condition, and, in particular in individuals that score low in ADHD symptoms, may explain why neural processes linked to ADHD were related to lower levels of detail in general, rather than in a task specific manner.
Contemporary accounts of spontaneous thought have argued that individuals with ADHD are unable to suppress internally oriented cognition that is supported by the DMN (Andrews-Hanna et al., Reference Andrews-Hanna, Smallwood and Spreng2014; Christoff et al., Reference Christoff, Irving, Fox, Spreng and Andrews-Hanna2016). Our analysis using MDES found no evidence that ADHD was linked to greater off-task thought. Moreover, while high levels of ADHD were linked to low levels of connectivity between the DMN and sensorimotor cortex, unlike the neural activity in the FPN, this connection showed no relationship with changes in detailed thought that were associated with ADHD scores. These results suggest that instead of problems in suppressing internally oriented cognition related to over activity within the DMN, experiential differences in ADHD may be, at least in part, mediated by problems in maintaining detailed task representations. As is made explicit in executive failure views of mind-wandering (McVay and Kane, Reference McVay and Kane2009), the inability to sustain attention on task relevant information could indirectly produce periods of elevated off-task thought since individuals would spend less time focused on the task in hand (Smallwood et al., Reference Smallwood, Gorgolewski, Golchert, Ruby, Engen, Baird, Vinski, Schooler and Margulies2013a).
More generally, recent studies suggest that the DMN might carry out a role that extends beyond that of internally oriented cognition (Vatansever et al., Reference Vatansever, Manktelow, Sahakian, Menon and Stamatakis2018). For example, recent work has demonstrated that the DMN can make an important contribution to externally oriented tasks, especially when behaviour is guided by representations gained from memory (Konishi et al., Reference Konishi, McLaren, Engen and Smallwood2015; Vatansever et al., Reference Vatansever, Menon, Manktelow, Sahakian and Stamatakis2015, Reference Vatansever, Manktelow, Sahakian, Menon and Stamatakis2016a, Reference Vatansever, Manktelow, Sahakian, Menon and Stamatakis2016b, Reference Vatansever, Menon and Stamatakis2017; Murphy et al., Reference Murphy, Rueschemeyer, Watson, Karapanagiotidis, Smallwood and Jefferies2017). Thus, it is possible that the absence of a relationship between the DMN and patterns of ongoing thought linked to ADHD emerges because of the task in which we assessed ongoing cognition. Plausibly, this relationship may emerge more readily in the context of a task requiring greater DMN engagement such as reading (Smallwood et al., Reference Smallwood, Gorgolewski, Golchert, Ruby, Engen, Baird, Vinski, Schooler and Margulies2013a; Regev et al., Reference Regev, Simony, Lee, Tan, Chen and Hasson2018) or during unconstrained states of rest (Castellanos et al., Reference Castellanos, Margulies, Kelly, Uddin, Ghaffari, Kirsch, Shaw, Shehzad, Di Martino, Biswal, Sonuga-Barke, Rotrosen, Adler and Milham2008).
Alternatively, it is possible that the role of the DMN in ongoing cognition is more transient and is therefore undetectable using our cross-sectional design in a neurotypical cohort. Notably, however, in a recent online experience sampling study we were able to predict patterns of off-task thought in regions of attention and sensorimotor cortex (Sormaz et al., Reference Sormaz, Murphy, Wang, Hymers, Karapanagiotidis, Poerio, Margulies, Jefferies and Smallwood2018) while connectivity between the ventral attention network with motor cortex predicted the ability to regulate the occurrence of off-task thought (Turnbull et al., Reference Turnbull, Wang, Schooler, Jefferies, Margulies and Smallwood2018). Future cognitive research, therefore, may be able to provide valuable empirical evidence on the brain basis of patterns of ongoing thought, by measuring neural function in individuals with ADHD concurrently with experience sampling. Such studies could help determine whether activity within the DMN, or other large-scale brain networks, varies with the level of ADHD symptoms during mind-wandering. Nonetheless, in the absence of new data, our study suggests that in the context of a working memory task, (i) ADHD related changes in ongoing thought are more parsimoniously explained by changes in the intrinsic architecture of the FPN, rather than the DMN, and (ii) do not reflect the inability to suppress off-task thought, but reflect problems in maintaining detailed task representations.
More generally, the results of both our functional connectivity analyses highlight changes in connectivity linked to ADHD that reflect reduced communication between regions of the transmodal cortex (DMN and FPN) with aspects of cortex linked to more specialised unimodal functions (visual and sensorimotor cortices). Current views of both ongoing thought (Smallwood et al., Reference Smallwood, Beach, Schooler and Handy2008; Kam et al., Reference Kam, Dao, Farley, Fitzpatrick, Smallwood, Schooler and Handy2011; Baird et al., Reference Baird, Smallwood, Lutz and Schooler2014; Seli, Reference Seli2016) and ADHD (Ghanizadeh, Reference Ghanizadeh2011) highlight patterns of sensorimotor decoupling as an important feature. Both of these literatures suggest that a general problem in ADHD may emerge from an exacerbation in the decoupling between transmodal and unimodal cortical regions. It is important to note, however, that the process of sensorimotor decoupling is most effectively measured when indices of neural function are assessed online during task performance (Baird et al., Reference Baird, Smallwood, Lutz and Schooler2014). Nonetheless, it is intriguing that neural patterns associated with ADHD show patterns of connectivity that are consistent with a reduction in neural communication between aspects of unimodal cortex that support task performance in a direct manner (i.e. perception and action) and those that play a more general supervisory role. Future research into deficits linking ADHD and ongoing thought, may wish to explore the coupling between regions of unimodal and transmodal cortex online during task performance, perhaps using an electrophysiological neuroimaging method that is more suited to assessing momentary changes in the dynamics of neural function (Vidaurre et al., Reference Vidaurre, Quinn, Baker, Dupret, Tejero-Cantero and Woolrich2016; Fox et al., Reference Fox, Foster, Kucyi, Daitch and Parvizi2018).
We also consider the implications of our results for the occurrence and management of ADHD symptoms in the real world. Our study provides complementary neural and subjective markers that, if replicated within a clinical population, would provide an important metric for assessing the efficacy of both psychological and pharmacological interventions for individuals with this disorder. For example, psychological interventions, such as mindfulness training (Mitchell et al., Reference Mitchell, Zylowska and Kollins2015), and drug interventions (Turner et al., Reference Turner, Blackwell, Dowson, McLean and Sahakian2005) have both shown promise in reducing ADHD symptomatology. Based on our results, studies combining experience sampling with measures of neural function may provide important insight into the specific neurocognitive changes that underlie the effectiveness of such interventions. In addition, given mounting evidence on the genetic basis of ADHD (Mick and Faraone, Reference Mick and Faraone2008; Pironti et al., Reference Pironti, Lai, Muller, Dodds, Suckling, Bullmore and Sahakian2014), population studies that examine experiential and neural differences that emerge in this cohort may provide unique insight into the link between genes, behaviour and cognition.
There are a number of limitations that should be considered when interpreting the results of this study. We examined levels of ADHD symptomatology in a group of neurotypical, healthy undergraduate students, rather than in a clinical population. While it is reasonably common to examine differences in ADHD in the normal population as a proximal measure for a clinical population (van Dongen et al., Reference van Dongen, von Rhein, O'Dwyer, Franke, Hartman, Heslenfeld, Hoekstra, Oosterlaan, Rommelse and Buitelaar2015), it is possible that some of the relationships we identified in our current study may vary in clinical populations for whom symptoms are likely to be more extreme. In addition, as outlined earlier, our study used a cross-sectional design in which differences in functional connectivity at rest was used to explain patterns of ongoing cognition measured outside of the scanner in a behavioural laboratory. While this approach provides important evidence on how neural architecture can relate to the manner in which cognition unfolds during tasks, it is possible that certain aspects of the relationships described in our study would vary if neural function was measured during task performance. Such limitations notwithstanding, our study suggests that patterns of ADHD symptomatology are linked to problems in maintaining detailed representations during a working memory task and that this pattern is partially accounted for by associated changes in the coupling between regions of cortex important in demanding tasks and those linked to visual processing.
Supplementary material
The supplementary material for this article can be found at https://doi.org/10.1017/S0033291718003598
Author ORCIDs
Deniz Vatansever 0000-0002-2494-9945
Acknowledgements
The authors extend their gratitude to Theodoros Karapanagiotidis, Mladen Sormaz, Charlotte Murphy, Hao-Ting Wang and Giulia Poerio for their invaluable contribution to the scanning and behavioural testing of participants. In addition, the authors thank Andre Gouws, Ross Devlin, Jane Hazell and the rest of the York Neuroimaging Centre staff for their support in setting up the imaging protocol and scanning. Finally, we thank all the participants for their time and effort in taking part in this study.
Financial support
This work was supported by the European Research Council (Project ID: 646927) and a grant from the John Templeton Foundation (Prospective Psychology Stage 2: A Research Competition).
Conflict of interest
None.
Ethical standards
The authors assert that all procedures contributing to this work comply with the ethical standards of the relevant national and institutional committees on human experimentation and with the Helsinki Declaration of 1975, as revised in 2008.