Introduction
Traditional medicine is used extensively worldwide with as much as two-thirds of the world's population relying on it for primary healthcare needs (Farnsworth et al., Reference Farnsworth, Akerele, Bingel, Soejarto and Guo1985; Barnes et al., Reference Barnes, Bloom and Nahin2008; World Health Organization, 2015b). Despite on-going loss of traditional knowledge (Reyes-García et al., Reference Reyes-García, Guèze, Luz, Paneque-Gálvez, Macía, Orta-Martínez, Pino and Rubio-Campillo2013), the global medicine chest of plants still includes tens of thousands of species (Schippmann et al., Reference Schippmann, Leaman and Cunningham2002; McChesney et al., Reference McChesney, Venkataraman and Henri2007). Many modern drugs are derived from plants and other natural sources (Newman and Cragg, Reference Newman and Cragg2007; Cragg and Newman, Reference Cragg and Newman2009; Cragg et al., Reference Cragg, Grothaus and Newman2009; Li and Vederas, Reference Li and Vederas2009). Yet, most traditional medicinal plants remain to be chemically or pharmacologically investigated, and many of the approximately 300,000 plant species that are not used traditionally are likely to have undiscovered medicinal value (Balandrin et al., Reference Balandrin, Kinghorn and Farnsworth1993; Fabricant and Farnsworth, Reference Fabricant and Farnsworth2001; Raskin et al., Reference Raskin, Ribnicky, Komarnytsky, Ilic, Poulev, Borisjuk, Brinker, Moreno, Ripoll and Yakoby2002; ThePlantList, 2016). Against the rapid loss of biodiversity (Brummitt and Bachman, Reference Brummitt and Bachman2010), a key challenge is to speed up the process of identifying the plants most likely to yield useful products, and hence best targeted for screening.
Unexplored biodiversity presents great opportunities but necessitates methodological developments in bioprospecting, the endeavour of finding valuable products in nature (McClatchey, Reference McClatchey2005). Bioprospecting is often carried out randomly across geographic regions and taxa. An alternative approach is ethnodirected bioprospecting, guided by traditional medicine, under the assumption that traditional uses are more likely to identify plants with medically useful compounds (Balandrin et al., Reference Balandrin, Kinghorn and Farnsworth1993; Fabricant and Farnsworth, Reference Fabricant and Farnsworth2001; Raskin et al., Reference Raskin, Ribnicky, Komarnytsky, Ilic, Poulev, Borisjuk, Brinker, Moreno, Ripoll and Yakoby2002; Gurib-Fakim, Reference Gurib-Fakim2006). Comparison of outcomes from random versus ethnodirected sampling has offered generally ethnodirected-positive results (Balick and Cox, Reference Balick and Cox1996; Slish et al., Reference Slish, Ueda, Arvigo and Balick1999; Khafagi and Dewedar, Reference Khafagi and Dewedar2000; Gyllenhaal et al., Reference Gyllenhaal, Kadushin, Southavong, Sydara, Bouamanivong, Xaiveu, Xuan, Hiep, Hung and Loc2012). Following chemosystematic hypotheses established in the 1980s (e.g. (Dahlgren, Reference Dahlgren1980; Gottlieb, Reference Gottlieb1982)), evolutionarily guided approaches have also been suggested as an additional, and complementary, way of narrowing down the search for bioactive plants. By mapping properties, such as specific bioactivities and/or traditional uses on phylogenetic trees, we can reveal evolutionary patterns that enable a targeted investigation of groups with abundant bioactivity representation. Combining an ethnodirected approach with phylogenetic analyses presents a promising methodology to highlighting lineages with desired chemical or medicinal properties (Wink, Reference Wink2003; Rønsted et al., Reference Rønsted, Symonds, Birkholm, Christensen, Meerow, Molander, Mølgaard, Petersen, Rasmussen and Van Staden2012; Saslis-Lagoudakis et al., Reference Saslis-Lagoudakis, Savolainen, Williamson, Forest, Wagstaff, Baral, Watson, Pendry and Hawkins2012).
A global need exists for new treatments for diseases related to the central nervous system (IFPMA, 2012). Numerous psychoactive plants are traditionally used for the treatment of these diseases, and many have been studied for relevant bioactivity (Benishin, Reference Benishin1992; DeFeudis, Reference DeFeudis1998; Roz and Rehavi, Reference Roz and Rehavi2003; Heinrich and Teoh, Reference Heinrich and Teoh2004; Hao et al., Reference Hao, Xing-Jun, Yong-Yao, Liang, Yang and Hong-Zhuan2005) affecting – among other areas of influence – the cholinergic and serotonergic systems. We propose that a phylogenetic investigation of psychoactive plants and their properties can inform the discovery of new neuropharmacological leads. Therefore, our objectives were: (i) to generate a comprehensive database of psychoactive plants and their properties and (ii) to investigate phylogenetic patterns of psychoactive plants and generate predictions as to which lineages are more likely to deliver new neuropharmacological leads.
Materials and methods
Data collection
The first objective of our study was to produce a comprehensive database of known psychoactive plants and their properties. Psychoactive plants are a diverse group of plants with the common trait of producing chemical compounds that affect the central nervous system of humans and induce a noticeable cognitive effect (World Health Organization, 2015a). In the present study, we defined a psychoactive plant as a species noted in the literature with documented traditional practices or clinically described cognitive effects induced after administration of the raw or processed plant material, regardless of the availability of additional chemical information. We performed a literature research examining four major encyclopaedias on psychoactive plants (Shultes, Reference Shultes1976; Ott, Reference Ott1996; Rätsch, Reference Rätsch2005; Wink and Van-Wyk, Reference Wink and Van-Wyk2008). We scrutinized literature sources and we extracted records of cognitive effects for each species, which was classified in one, two or all of three cognitive effect categories: stimulant, sedative or hallucinogenic. Reported cognitive effects in the literature can come from subjective experiences and sometimes do not directly translate to a certain neuroactivity. Therefore, to investigate patterns in neuroactivity, when chemical information was available, we recorded the activity of a species’ chemical compounds on neurotransmitter systems and receptors. Of particular relevance to this study, we recorded effects on serotonergic and cholinergic neurotransmitter systems. Cholinergic neurons rely on acetylcholine as neurotransmitter, which is found widely in both the central and peripheral nervous system. Peripherally, acetylcholine is involved in signalling of the neuromuscular junction and thus muscle activity, as well as various autonomic nervous system functions. In the brain, acetylcholine has several modulating effects; for instance influencing neuronal plasticity as well as in networks related to e.g. perception and reward. Alzheimer's and other neurodegenerative diseases seem to correlate with changes in the cholinergic systems, the so-called cholinergic hypothesis (Geula and Mesulam, Reference Geula and Mesulam1995; Francis et al., Reference Francis, Palmer, Snape and Wilcock1999; Lachowicz et al., Reference Lachowicz, Duffy, Ruperto, Kozlowski, Zhou, Clader, Billard, Binch, Crosby and Cohen-Williams2001; Lee et al., Reference Lee, Shiao, Chen and Wang2001; Olincy et al., Reference Olincy, Harris, Johnson, Pender, Kongs, Allensworth, Ellis, Zerbe, Leonard and Stevens2006; Binder et al., Reference Binder, Hirokawa and Windhorst2009; Contestabile, Reference Contestabile2011; Craig et al., Reference Craig, Hong and McDonald2011; Lendvai et al., Reference Lendvai, Kassai, Szájli and Némethy2013). Serotonin is a monoamine neurotransmitter largely found in the gastrointestinal tract as well as in the central nervous system. Serotonin is involved in various cognitive networks, and consequently most antidepressants target serotonin deficiency, for instance by functioning as reuptake inhibitors (Andersen et al., Reference Andersen, Vestergaard and Lauritzen1994). Several of the classical hallucinogenic substance groups (e.g. tryptamines, b-carbolines and ergots) are affecting serotonergic systems giving rise to the cognitive effects and their suggested uses in, for instance, depression therapies (Andersen et al., Reference Andersen, Vestergaard and Lauritzen1994; Chugani et al., Reference Chugani, Muzik, Rothermel, Behen, Chakraborty, Mangner, Da Silva and Chugani1997; Boyer and Shannon, Reference Boyer and Shannon2005; Kish et al., Reference Kish, Tong, Hornykiewicz, Rajput, Chang, Guttman and Furukawa2008; Binder et al., Reference Binder, Hirokawa and Windhorst2009; Abbas et al., Reference Abbas, Naqvi, Erum, Ahmed and Dar2013; Hieronymus et al., Reference Hieronymus, Emilsson, Nilsson and Eriksson2016).
Furthermore, within the effects on the cholinergic neurotransmitter system we differentiated agonistic and antagonistic (promoting and inhibiting) activity on the two major acetylcholine receptors, namely the nicotinic and muscarinic acetylcholine receptors. Nicotinic agonists are of particular relevance to treatments of schizophrenia (Olincy et al., Reference Olincy, Harris, Johnson, Pender, Kongs, Allensworth, Ellis, Zerbe, Leonard and Stevens2006), nicotine dependence (Foulds, Reference Foulds2006) and Alzheimer's disease (Lendvai et al., Reference Lendvai, Kassai, Szájli and Némethy2013; Lenz et al., Reference Lenz, Pritchett, Berry, Llano, Han, Berry, Sadowsky, Abi-Saab and Saltarelli2015), whereas muscarinic antagonists are of relevance to, for instance, learning and cognition (Hagan et al., Reference Hagan, Jansen and Broekkamp1987; Lachowicz et al., Reference Lachowicz, Duffy, Ruperto, Kozlowski, Zhou, Clader, Billard, Binch, Crosby and Cohen-Williams2001) and Parkinson's disease (Xiang et al., Reference Xiang, Thompson, Jones, Lindsley and Conn2012).
Following data collection, the database was curated using the Taxonomic Names Resolution Service (iPlant Collaborative) and additional consultation of online resources (ThePlantList, 2016). Synonymous species names were replaced with accepted names, in 12 instances leading to two or more synonyms being replaced by a single accepted species. Additional data management, analysis and visualization were performed in R (R Core Team, 2015) using the libraries ape, geiger, picante, plyr, caper and taxize (Paradis et al., Reference Paradis, Claude and Strimmer2004; Harmon et al., Reference Harmon, Weir, Brock, Glor and Challenger2008; Kembel et al., Reference Kembel, Cowan, Helmus, Cornwell, Morlon, Ackerly, Blomberg and Webb2010; Wickham, Reference Wickham2011; Chamberlain and Szocs, Reference Chamberlain and Szocs2013; Orme et al., Reference Orme, Freckleton, Thomas, Petzoldt, Fritz, Isaac and Pearse2013).
Phylogenetic manipulations
For the phylogenetic analyses of psychoactive plants and selected properties, we used a publicly available genus level phylogenetic tree (Hinchliff and Smith, Reference Hinchliff and Smith2014). The tree includes 13,093 genera and is the most comprehensive phylogeny of the subkingdom Embryophyta (land plants) to date. Three of the genera in our database were not included in the phylogeny and were therefore omitted in subsequent analyses. All analyses were performed at the genus level, in accordance with the genus level phylogeny.
We performed two types of phylogenetic analyses: one testing for phylogenetic clustering of psychoactive plants and their properties (D metric) and the other exploring the lineages where that clustering is present (hot nodes). To estimate the degree of phylogenetic clustering of different psychoactive properties, we used the D metric (Fritz and Purvis, Reference Fritz and Purvis2010). We chose the D metric to estimate the degree of phylogenetic clustering because it tests the observed phylogenetic pattern not only against a random phylogenetic distribution like other metrics (e.g. MPD, NTI), but also against the pattern expected under the Brownian model. Hence, it is more stringent in detecting phylogenetic clustering compared with other metrics. A D value of 1 corresponds to a random distribution and a value of 0 corresponds to a clustered distribution as expected under Brownian motion (Fritz and Purvis, Reference Fritz and Purvis2010). The computation yields a D score and two probability values of D equalling 1 (random phylogenetic distribution) and 0 (clustered phylogenetic distribution as expected under Brownian motion). The D score and probability values provide the opportunity of distinguishing three levels of phylogenetic signal strength: (i) random distribution if the phylogenetic distribution of the analysed property is not significantly different from a random distribution (P(D = 1) > 0.05), (ii) non-random distribution if the property shows a distribution significantly more clustered than random (P(D = 1) < 0.05), however still significantly less clustered than a distribution expected by Brownian motion (P(D = 0) < 0.05), and (iii) clustered distribution if the observed D value is different from random (P(D = 1) < 0.05) and furthermore statistically indistinguishable from a clustered distribution expected by Brownian motion (P(D = 0) > 0.05). All D randomizations were calculated using 1000 permutations. Computation of D values was performed using the phylo.d function of the caper library (Orme et al., Reference Orme, Freckleton, Thomas, Petzoldt, Fritz, Isaac and Pearse2013) using an R script adapted from Ernst et al. (Reference Ernst, Saslis-Lagoudakis, Grace, Nilsson, Toft Simonsen, Horn and Rønsted2016).
To identify the position of phylogenetic clustering for different properties, we highlighted the ‘hot nodes’ on the phylogeny, i.e. nodes that are significantly overrepresented in genera with a given property compared with the rest of the tree. This approach has been proposed in the past to identify lineages that are the best candidates for drug discovery screening (Saslis-Lagoudakis et al., Reference Saslis-Lagoudakis, Klitgaard, Forest, Francis, Savolainen, Williamson and Hawkins2011, Reference Saslis-Lagoudakis, Savolainen, Williamson, Forest, Wagstaff, Baral, Watson, Pendry and Hawkins2012). The rationale is that if a lineage contains significantly more genera with a given property (e.g. cholinergic activity), it is very likely that other genera in that lineage will share this property with their relatives. The ‘hot nodes’ were identified using the nodesigl command in PHYLOCOM v4.2 (Webb et al., Reference Webb, Ackerly and Kembel2008). To ensure that ‘hot nodes’ narrow down our search for new plant species with psychoactive properties, we only considered nodes that included up to 100 taxa. This was done to ensure that the clades identified by our approach would be informative in the bioprospecting context of this study, as larger clades do not substantially narrow down the search for new psychoactive plants.
We performed these two types of analyses for five traits over three hierarchical levels: (i) all psychoactive genera, (ii) two select groups of psychoactive genera, influencing serotonergic or cholinergic neurotransmitter systems, and (iii) genera with cholinergic activity, subdivided into those with agonistic nicotinic receptor activity and antagonistic muscarinic receptor activity.
Results
Description of the database
The literature included in our survey contained information about 501 psychoactive plant species, distributed in 249 genera and 93 families, corresponding to 0.14% of all plant species, 1.9% of all plant genera, and 14% of all plant families (ThePlantList, 2016) (Table 1). Out of all species, 42% were reported as stimulants, 31% with as sedatives and 76% as hallucinogens. Almost half of the species (44%) had more than one reported cognitive effect. For 78% of species, we were able to find additional information on chemical compounds affecting the nervous system and for 70% these chemical data could be linked to specific neuroactivity. A total of 226 unique compounds with described biological activities in the nervous system were reported, classified into nine chemical structural classes. Of the nine structural classes, alkaloids comprised the majority of compounds (160 compounds in 318 species), followed by terpenoids (38 compounds in 50 species). Furthermore, 46 neuronal targets and 11 affected neurotransmitter systems were reported (Table 2). Cholinergic and serotonergic activities were present in 16 and 20% of psychoactive genera, respectively. Out of 87 genera that demonstrate either of these activities, only three demonstrate both, showing limited taxonomic overlap. Further, 19 genera express nicotinic agonistic activity, and 12 genera express muscarinic antagonistic activity. The database is presented in Table S1.
Table 1. Description of the psychoactive plant database presented in this study. Percentage of all land plants in brackets

Table 2. Number of compounds and plant species in which different categories of chemistry, neuroactivity and cognitive effects are found

Phylogenetic clustering
We found moderate signal of phylogenetic clustering for psychoactive plants on the land plant (Embryophyta) phylogeny: their distribution on the tree is significantly non-random ((P(D = 1) < 0.05); Table 3), but not significantly clustered corresponding to Brownian motion (P(D = 0) < 0.05). Similarly, genera with cholinergic and serotonergic activity were non-randomly distributed on the phylogeny, but not also significantly clustered according to Brownian motion. Genera with nicotinic agonistic activity were also non-randomly distributed on the phylogeny, while genera with muscarinic antagonistic activity were significantly clustered being indistinguishable from Brownian motion ((P(D = 0) > 0.05); Table 3).
Table 3. Phylogenetic clustering of different properties related to psychoactivity on an Embryophyta phylogeny of 13,093 plant genera (Hinchliff and Smith, Reference Hinchliff and Smith2014). Degree of clustering was assessed with the D metric (Fritz and Purvis, Reference Fritz and Purvis2010)

Hot nodes
The hot nodes identified for the different traits related to psychoactivity are shown in Fig. 1 and Figs S1–S2. For psychoactive plants in general, the hot nodes identified 1,115 out of 13,093 genera (8.5%) on the Embryophyta phylogeny, including 141 of a total of 249 psychoactive genera. For plants with cholinergic activity, hot nodes highlighted 399 genera (3.1% of all genera on the phylogenetic tree), of which 39 are genera with known cholinergic activity, while for serotonergic activity, there were 536 genera in the hot nodes (4.1% of all genera on the phylogenetic tree), including all 49 genera with known serotonergic activity. Finally, for plants with nicotinic agonists, the hot nodes included 381 genera (2.9% of all genera on the phylogenetic tree), of which 18 have known nicotinic agonists, and for genera with muscarinic antagonists, the hot nodes highlighted 117 genera (0.9% of all genera on the phylogenetic tree), including all 12 genera with known muscarinic antagonists. The genera identified in the hot nodes for all five properties investigated are given in Tables S2–S6.
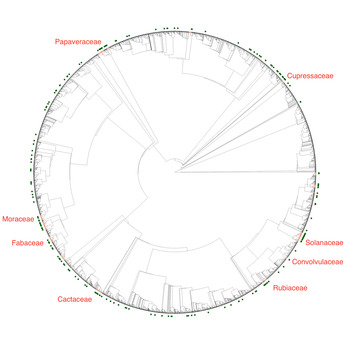
Fig. 1. Distribution of known psychoactive genera and hot nodes on the embryophyta phylogeny. There are 249 psychoactive plant genera (green dots) that are non-randomly distributed on the embryophyta phylogeny. The hot nodes (red clades) represent lineages that are overrepresented in psychoactive genera, and hence should be prioritized in bioprospecting. The phylogenetic tree was generated by Hinchliff and Smith (Reference Hinchliff and Smith2014).
Discussion
Psychoactive plants are traditionally used to treat diseases related to the nervous system. The study of psychoactive plants has guided the development of modern neuropharmacology (Chatterjee et al., Reference Chatterjee, Bhattacharya, Wonnemann, Singer and Müller1998; Robson, Reference Robson2001; Klockgether-Radke, Reference Klockgether-Radke2002; Pittler and Ernst, Reference Pittler and Ernst2003; Roz and Rehavi, Reference Roz and Rehavi2003; Abbas et al., Reference Abbas, Naqvi, Erum, Ahmed and Dar2013; Palhano-Fontes et al., Reference Palhano-Fontes, Andrade, Tofoli, Santos, Crippa, Hallak, Ribeiro and de Araujo2015) and could direct future bioprospecting efforts for neuropharmacological leads. In this study, we compiled a database of 501 psychoactive plant species with described cognitive effects, along with 226 chemical compounds with described neuroactivity at 46 specific neuronal targets (Table S1). Following an ethnodirected approach, future studies can utilize this database to investigate plants for neuropharmacological leads. Our database also provides information on the presence of plant chemical compounds related to specific activities on neurotransmitter systems and receptors, which can potentially direct future investigations in the search for novel drugs or other commercial products affecting the nervous system.
The main objective of our study was to explore the potential of phylogenetic tools for informing future bioprospecting efforts looking for neuropharmacological leads. We used a large public phylogeny of 13,093 plant genera and explored the distribution of psychoactive properties of plants in our database on the phylogeny. First, we found that psychoactive plants have a non-random distribution on the Embryophyta phylogeny (Table 3); psychoactive species are more common in some plant lineages than in others. We further investigated the phylogenetic distributions of plants affecting cholinergic and serotonergic neurotransmitter systems, and found that both these plant groups were also non-randomly distributed on the phylogeny (Table 3). Finally, we partitioned plants affecting cholinergic neurotransmitter systems into those with nicotinic agonists and muscarinic antagonists and again we found non-random and clustered phylogenetic distributions, respectively. These phylogenetic patterns are relevant to bioprospecting because they can reflect underlying phylogenetic patterns of chemistry and bioactivity (Wink, Reference Wink2003; Zhu et al., Reference Zhu, Qin, Tao, Liu, Shi, Ma, Jia, Tan, Cui and Lin2011).
Our results of phylogenetic clustering in medicinal properties are in agreement with findings from previous studies of other aspects of plant medical potential. For instance, phylogenetic investigations of medicinal properties in different plant genera have consistently found phylogenetic clustering (Lukhoba et al., Reference Lukhoba, Simmonds and Paton2006; Saslis-Lagoudakis et al., Reference Saslis-Lagoudakis, Klitgaard, Forest, Francis, Savolainen, Williamson and Hawkins2011; Grace et al., Reference Grace, Buerki, Symonds, Forest, van Wyk, Smith, Klopper, Bjorå, Neale and Demissew2015; Ernst et al., Reference Ernst, Saslis-Lagoudakis, Grace, Nilsson, Toft Simonsen, Horn and Rønsted2016). Other studies have investigated traditional medicinal uses of plants across whole floras from different regions and found similar patterns of phylogenetic clustering of medicinal properties (Forest et al., Reference Forest, Grenyer, Rouget, Davies, Cowling, Faith, Balmford, Manning, Procheş, van der Bank, Reeves, Hedderson and Savolainen2007; Saslis-Lagoudakis et al., Reference Saslis-Lagoudakis, Savolainen, Williamson, Forest, Wagstaff, Baral, Watson, Pendry and Hawkins2012). Although these investigations have looked at a wide range of medicinal properties, a smaller subset of studies has considered properties related to neuroactivity. For instance, work on the Amaryllidaceae family has shown phylogenetic signal in the distribution of acetylcholine esterase (AChE) inhibitory activity in the genus Narcissus (Rønsted et al., Reference Rønsted, Savolainen, Mølgaard and Jäger2008), as well as tribes Haemantheae (Bay-Smidt et al., Reference Bay-Smidt, Jäger, Krydsfeldt, Meerow, Stafford, Van Staden and Rønsted2011) and Galantheae (Larsen et al., Reference Larsen, Adsersen, Davis, Lledó, Jäger and Rønsted2010). More recently, Rønsted et al. (Reference Rønsted, Symonds, Birkholm, Christensen, Meerow, Molander, Mølgaard, Petersen, Rasmussen and Van Staden2012) found significantly non-random phylogenetic distributions of chemical compounds and neuroactivities related to the cholinergic and serotonergic neurotransmitter systems across the whole family Amaryllidaceae. The findings from all these studies demonstrate that phylogenetic patterns of plant uses, chemistry and bioactivity are widely present across land plants and, therefore, the phylogenetic predictive method can help us select lineages for drug lead discovery.
Building on these studies, our results indicate that psychoactive plants are also not randomly distributed across all land plant lineages. As a result, targeting lineages with an overrepresentation of psychoactive properties can be a rapid and effective way to bioprospect for novel plants containing compounds with neuroactivity. We identified ‘hot nodes’ of psychoactive properties (Webb et al., Reference Webb, Ackerly and Kembel2008; Saslis-Lagoudakis et al., Reference Saslis-Lagoudakis, Klitgaard, Forest, Francis, Savolainen, Williamson and Hawkins2011) to pinpoint specific plant lineages in which those properties are overrepresented (Fig. 1 and Figs S1–S2). The phylogenetic approach we used here has two important advantages compared with random bioprospecting. The first advantage is that, compared with a random search, it substantially narrows down the search. Depending on the property investigated, our methodology identifies from 0.9% (muscarinic antagonists) to 8.5% (general psychoactivity) of all land plant genera.
The hot nodes identified for general psychoactivity are found in 45 of approximately 640 plant families, including some well-known psychoactive families (e.g. Cactaceae, Convolvulaceae, Fabaceae, Papaveraceae, Rubiaceae and Solanaceae) and other families that are less known for their psychoactivity (e.g. Cupressaceae and Moraceae), as shown in Fig. 1. The respective families in hot nodes from other properties were even fewer. For example, the hot nodes for nicotinic agonists are found in only eight families: Apocynaceae, Asteraceae, Berberidaceae, Fabaceae, Primulaceae, Proteaceae, Ranunculaceae and Solanaceae (Table S5). Psychoactive plant substances, along with other plant medicines and poisons, are often secondary metabolites whose likely function is chemical defence against herbivores through affecting animal nervous systems. Since herbivory acts upon the whole plant tree of life, it is not surprising to find hot nodes of psychoactive plants in several lineages.
The second advantage of our approach is that, compared to ethnodirected bioprospecting, it identifies lineages not reported in our database of psychoactive plants, based on available information on uses or properties, but on phylogenetic patterns. For example, only 149 of the 1,115 genera included in the hot nodes for psychoactivity are reported in our database, which translates to approximately 87% of novel genera to investigate for psychoactivity. The equivalent novelty, i.e. genera included in hot nodes that are not in the database, is approximately 90% for serotonergic and cholinergic activity, as well as for muscarinic antagonists, and increases even further for nicotinic agonists (96%). Hence, our approach provides an in silico methodology based on existing data (reports on plant psychoactivity) to narrow down our search for bioactivity in plants, while ensuring that plants with no reports will also be considered/highlighted for further investigation. For instance, if we are looking for plants that produce muscarinic antagonists, the most prominent hot node from our analyses is a clade containing 95 genera from the nightshade family (Solanaceae) (Fig. 2). Ten genera from this family are included in the database (Atropa, Brugmansia, Cestrum, Datura, Duboisia, Hyoscyamus, Latua, Mandragora, Scopolia and Solandra). Four of these (Atropa, Hyoscyamus, Latua and Scopolia) are found in a clade of the subfamily Solanoideae (Fig. 2), highlighting this clade as a good potential source of muscarinic antagonists, particularly tropane alkaloids that these four genera produce. To explore the validity of our approach, we looked at the phytochemistry of this clade and found that other genera that are not included in the database, but included in this clade (Anisodus, Przewalskia and Physochlaina) produce tropane alkaloids (Peigen and Liyi, Reference Peigen and Liyi1982; Gorinova et al., Reference Gorinova, Atanassov, Velcheva and Bajaj1999; Wink, Reference Wink2003). On the other hand, Atropanthe, Sclerophylax, Nolana, Lycium, Phrodus, Grabowskia and Jaborosa remain to be screened for tropane alkaloids, but our results strongly suggest that such alkaloids will be found in species of these genera.

Fig. 2. An example of phylogenetic prediction of psychoactivity: the search for muscarinic antagonists. A: Distribution of genera with known muscarinic acetylcholine receptor antagonists (green dots) and hot nodes (red branches) on the embryophyte phylogeny (Hinchliff and Smith Reference Hinchliff and Smith2014). B: The most prominent hot node includes a large clade in the Solanaceae. which contains several genera producing tropane alkaloids. We argue that this clade, and particularly the subclade highlighted in green, should be prioritised in bioprospecting for muscarinic antagonists.
Our study also explores the potential of the phylogenetic approach to predict bioactivity at different levels. Here, we explored three levels: (i) psychoactivity in general, (ii) psychoactivity from cholinergic or serotonergic activity and (iii) cholinergic neuroactivity from nicotinic agonists or muscarinic antagonists. Our results show that investigations at different levels can yield different levels of specificity. While the hot nodes for all psychoactive plants identify more than 1,000 genera to be investigated, the hot nodes for cholinergic or serotonergic activity almost halve that number, and the hot nodes for neuroactivity from nicotinic agonists or muscarinic antagonists reduce the candidate genera to a couple of hundreds. Further, it is worth noticing that these investigations might be more specific when stronger phylogenetic clustering is observed. For example, genera with muscarinic antagonists were significantly clustered on the phylogeny, and the hot nodes for this trait narrow down the search to less than 1% of all genera. Therefore, when applying phylogenetic predictive approaches, it is important to investigate not only broad categories of bioactivity, but also pay special attention to more specific bioactivity categories.
Psychoactive plants are a diverse group of plants, many with long-standing traditional uses and pharmacological applications that are highly relevant to central nervous system disorders (Carlini, Reference Carlini2003). This study has shown a significantly non-random phylogenetic distribution of psychoactive plants across the phylogeny of land plants. In agreement with previous studies (Rønsted et al., Reference Rønsted, Savolainen, Mølgaard and Jäger2008; Saslis-Lagoudakis et al., Reference Saslis-Lagoudakis, Klitgaard, Forest, Francis, Savolainen, Williamson and Hawkins2011, Reference Saslis-Lagoudakis, Savolainen, Williamson, Forest, Wagstaff, Baral, Watson, Pendry and Hawkins2012; Zhu et al., Reference Zhu, Qin, Tao, Liu, Shi, Ma, Jia, Tan, Cui and Lin2011; Rønsted et al,. Reference Rønsted, Symonds, Birkholm, Christensen, Meerow, Molander, Mølgaard, Petersen, Rasmussen and Van Staden2012; Ernst et al., Reference Ernst, Saslis-Lagoudakis, Grace, Nilsson, Toft Simonsen, Horn and Rønsted2016), our findings provide support for the phylogenetic approach to bioprospecting, particularly for the selection of candidate plant lineages to screen for neuropharmacological leads. In the future, the predictive quality of the phylogenetic approach can be applied to other types of investigations. For example, phylogenetic correlations between reported cognitive effects from traditional medicine and plant chemistry can help us better understand which chemical compounds are associated with those effects. Similarly, a phylogenetic correlation between reported cognitive effects and neuroactivity can reveal links to different neurotransmitter systems. Ultimately, such investigations could lead to a better understanding appreciation of the biological and chemical underpinnings of traditional medicine.
Supplementary Material
The supplementary material for this article can be found at https://doi.org/10.1017/S1479262116000344
Acknowledgements
This work was supported by the Marie Curie Actions of the 7th European Community Framework Programme: FP7/2007-2013/, PIEF-GA-2012-328637-BiodiversityAltitude and REA grant agreement no. 606895-MedPlant. The authors thank Hugo de Boer for useful comments on earlier versions of this manuscript.