1 Introduction
Native speakers of a language have strong intuitions not only about what the existing words are in their language, but also about which novel forms are phonologically possible or impossible. It is assumed that these intuitions are guided by their phonotactic knowledge of the language. Non-word acceptability judgement studies in a variety of languages have demonstrated that speakers are able to make fine-grained judgements on various types of non-words (e.g. Frisch et al. Reference Frisch, Large and Pisoni2000, Frisch & Zawaydeh Reference Frisch and Zawaydeh2001, Frisch et al. Reference Frisch, Pierrehumbert and Broe2004, Myers & Tsay Reference Myers and Tsay2005, Kirby & Yu Reference Kirby, Yu, Trouvain and Barry2007, Albright Reference Albright2009, Daland et al. Reference Daland, Hayes, White, Garellek, Davis and Norrmann2011, Hayes & White Reference Hayes and White2013, Myers Reference Myers2015), suggesting that their phonotactic knowledge is a gradient and intricate system.
Using data from a syllable well-formedness judgement experiment, this paper explores the nature of phonotactic knowledge in Mandarin Chinese. Thirty-one native Mandarin speakers were recruited to rate the well-formedness of 200 Mandarin syllables (both attested and unattested). The unattested syllables violated various types of grammatical constraints. The results showed that phonotactic judgement in Mandarin is influenced by a number of grammatical factors, such as systematic phonotactic constraints, allophonic restrictions and syllable–tone co-occurrence patterns.
The observed phonotactic judgement was then modelled by a maximum entropy phonotactic grammar consisting of markedness constraints penalising certain combinations of feature matrices and natural classes. The weights of these markedness constraints were assigned by the UCLA Phonotactic Learner (Hayes & Wilson Reference Hayes and Wilson2008), which takes the existing syllable inventory in Mandarin weighted by morpheme frequency (Hsiao et al. Reference Hsiao, Tsai, Hsieh, Yeh and Tan2013) as the input to the training, and assigns the constraint weights by maximising the probability of the input forms. The output grammar is thus an inductive reflection of the statistical properties of the lexicon. The well-formedness predictions offered by the output grammar are overall a good reflection of speakers’ acceptability ratings obtained experimentally, but there are also a number of systematic mismatches. These mismatches indicate that phonological learning is a biased process: some phonotactic patterns are easier to learn and thus have stronger effects on non-word judgement, whereas other patterns are harder to learn and have limited effects on non-word judgement. Hence, several post hoc biases are superimposed on the grammar to compensate for the generalisations missed by the phonotactic learner (Hayes & White Reference Hayes and White2013). The biases introduced are (i) phonetic naturalness bias, (ii) allophony bias and (iii) suprasegmental bias. Adding these biases improved the performance of the phonotactic grammar. This indicates that phonotactic knowledge can largely be determined from the lexicon, but multiple biases also have effects.
The remainder of this paper is organised as follows. §2 reviews the factors that are known to influence phonological well-formedness and non-word acceptability judgements. §3 discusses the properties of Mandarin phonotactics and why Mandarin is an ideal language to address the factors mentioned in §2. §4 presents a Mandarin syllable acceptability judgement experiment and argues that phonological principles account for the most deviation in speakers’ judgements compared to lexical statistics. In §5–§6, we model the phonotactics of Mandarin in maximum entropy grammar, and consider how well the output grammar can predict speakers’ judgements. The discrepancies between the model's prediction and speakers’ judgements are handled by multiple learning biases, as discussed in §7. §8 provides further discussions of the experimental findings and their theoretical modelling. §9 concludes.
2 Factors influencing non-word acceptability
2.1 Systematic and accidental gaps
Not all non-words are judged to be equally acceptable by native speakers. A number of proposals have argued that non-words that violate universal grammatical principles are judged to be less acceptable than those that obey them (e.g. Chomsky & Halle Reference Chomsky and Halle1968, Kager & Pater Reference Kager and Pater2012). The most frequently discussed grammatical principles are sonority sequencing constraints in consonant clusters (Coleman & Pierrehumbert Reference Coleman, Pierrehumbert and Coleman1997, Moreton Reference Moreton2002, Berent et al. Reference Berent, Steriade, Lennertz and Vaknin2007) and similarity avoidance based on the Obligatory Contour Principle (Frisch & Zawaydeh Reference Frisch and Zawaydeh2001, Frisch et al. Reference Frisch, Pierrehumbert and Broe2004). Missing syllables in a language can then be roughly grouped into two types: those which violate systematic phonotactic constraints such as sonority sequencing can be labelled as systematic gaps, whereas those that do not are accidental gaps (Chomsky & Halle Reference Chomsky and Halle1965, Coetzee Reference Coetzee2006, Reference Coetzee2008). This division is supported by studies showing that systematic gaps are generally judged to be significantly worse than accidental gaps in non-word acceptability judgement tasks (Wang Reference Wang and Benjamin1998, Frisch & Zawaydeh Reference Frisch and Zawaydeh2001, Myers & Tsay Reference Myers and Tsay2005, Berent et al. Reference Berent, Steriade, Lennertz and Vaknin2007, Hayes & White Reference Hayes and White2013).
We discuss here the Obligatory Contour Principle (OCP) – the principle used to distinguish systematic gaps and accidental gaps in the current study – in greater detail. The OCP states that identical features or segments are not permitted to occur in sequence (Leben Reference Leben1973, McCarthy Reference McCarthy1986). This principle has been argued to have a production basis. For instance, Dell et al. (Reference Dell, Burger and Svec1997) argue that there is a turn-off function to deactivate each gesture in production planning, so that in sequences with similar segments the later sounds will be activated more slowly. This difficulty in production planning for nearly identical segments also induces speech errors such as sound misordering (Dell Reference Dell1984). For a review of the production difficulties associated with OCP violations, see Frisch (Reference Frisch, Hayes, Kirchner and Steriade2004) and Frisch et al. (Reference Frisch, Pierrehumbert and Broe2004). The principle may also have a perception basis (Graff Reference Graff2012). For instance, studies have shown that, when CVC syllables were presented in speech-spectrum noise, initial and final consonants sharing the same place of articulation were identified less accurately than those differing in place (Woods et al. Reference Woods, William Yund, Herron and Cruadhlaoich2010). Typologically, OCP effects in the lexicon are widely reported in many languages, for example Arabic (Frisch & Zawaydeh Reference Frisch and Zawaydeh2001), Hebrew (Berent & Shimron Reference Berent and Shimron1997), Muna (Coetzee & Pater Reference Coetzee and Pater2008) and Quechua (Gallagher Reference Gallagher2010), where homorganic consonants tend not to co-occur in the same root. The criteria for defining the systematic phonotactic constraints of a language are controversial. But, given the OCP's strong functional grounding in production and perception, as well as its widespread typological manifestation, it is reasonable to assume that it can serve as a systematic constraint to distinguish systematic gaps from accidental ones.
A different view on the basis of the acceptability differences among unattested structures suggests that the differences originate not from grammatical constraints but from lexical statistics that measure how likely it is for a sound or a sequence of sounds to occur in a certain position, or how similar a non-word is to existing lexical entries. For example, the phonotactic probability of a non-word, as measured by Vitevitch & Luce (Reference Vitevitch and Luce2004), refers to the cumulative biphone transitional probability, while the neighbourhood density of a non-word, as defined in Greenberg & Jenkins (Reference Greenberg and Jenkins1964), counts the number of words generated by substituting, deleting or adding a single phoneme. These measures are calculated directly from the lexicon. Non-words with higher lexical statistical measures will be judged as more acceptable (e.g. Coleman & Pierrehumbert Reference Coleman, Pierrehumbert and Coleman1997, Bailey & Hahn Reference Bailey and Hahn2001, Myers & Tsay Reference Myers and Tsay2005, Kirby & Yu Reference Kirby, Yu, Trouvain and Barry2007). In this view, phonotactics can be reduced to these types of statistical properties of the lexicon, and does not need to be accounted for by phonological grammar (Ohala Reference Ohala1986).
Results from judgement experiments, however, suggest that grammaticality and lexical statistics can both contribute to phonotactic knowledge. Frisch & Zawaydeh (Reference Frisch and Zawaydeh2001) examined the acceptability of Arabic novel words varying in phonotactic probability and neighbourhood density, as well as in whether they violated the OCP-Place constraint. After the two lexical measures were controlled, non-words violating the OCP were still judged as less wordlike than non-words that did not violate it. The difference in acceptability could only be accounted for by the independent effect from the grammar, in this case, the OCP-Place constraint. In Coetzee's (Reference Coetzee2008) English non-word judgement study, speakers judged OCP[labial] violation forms to be worse than OCP[dorsal] violation forms, even though lexical measures support the opposite. This counterlexical pattern is due to grammatical asymmetry between OCP[labial] and OCP[dorsal]. These experimental results indicate that grammatical principles such as the OCP and lexical statistics such as neighbourhood density play independent roles in phonotactic processing.
The grammaticality and lexical statistics approaches to phonotactic knowledge, however, are not mutually exclusive. For instance, Daland et al. (Reference Daland, Hayes, White, Garellek, Davis and Norrmann2011) show that the grammatical principle of sonority sequencing is learnable from the English lexicon, provided that the phonotactic learning algorithm can access the syllable structure and is able to generalise over features. Similarly, Frisch et al. (Reference Frisch, Pierrehumbert and Broe2004) argue that similarity avoidance is a substantive bias that shapes the lexicon in such a way that OCP-violating roots are severely underrepresented. Speakers then learn the statistical patterns of this lexicon, and internalise the OCP effect into the grammar. In this view, the OCP itself does not directly affect the learner's construction of a grammar; rather, it shapes the lexicon by preferring those items that do not contain adjacent repetitive features and dispreferring the items that do (Martin Reference Martin2007). Speakers then learn the OCP-based constraints abstracted over the existing items in the lexicon.
This review suggests that grammatical and lexical factors potentially both contribute to speakers’ acceptability judgements of non-existing structures, and that these factors may sometimes be difficult to tease apart. Shademan (Reference Shademan2007) and Coetzee (Reference Coetzee2008) take the position that speakers’ phonotactic knowledge is the result of the interaction between the grammatical and lexical factors. This is the position that we explore further in this paper. The grammatical principle used to distinguish between systematic and accidental gaps is the OCP. Non-words that violate the OCP are marked as systematic gaps, whereas accidental gaps do not violate this principle. For the lexical factors, we evaluate the effect of neighbourhood density on non-word judgement. If non-word judgement is truly a result of the interaction between these two factors, we expect that the difference between OCP-based systematic gaps and accidental gaps will make a unique contribution to speakers’ non-word judgement after neighbourhood density has been taken into account.
2.2 Allophony in phonotactics
In addition to the interaction between grammatical principles and lexical statistics reviewed above, there are additional factors that have not been systematically investigated previously in phonotactics research, but could also have effects on phonotactic judgement. Studies on phonotactics have generally focused on phonotactic restrictions held on the phonemic level, but few have looked into the phonotactic effects of allophonic distributions. For instance, in many dialects of English, plosives exclusively occupying the onset position are aspirated (e.g. [pʰik] peak), but are unaspirated in onset [sC] clusters (e.g. [spik] speak). We are interested in how native English speakers will respond to non-words violating such allophonic restrictions (e.g. *[spʰik]).
The reason that allophony is often disregarded in the discussion of phonotactics is related to perception findings showing that allophonic differences are less salient perceptually. For instance, allophones of the same phoneme are often categorised as the same by speakers, due to perceptual similarity (e.g. Jaeger Reference Jaeger1980). This insensitivity effect is even stronger when the allophones occur in non-lexical conditions (Whalen et al. Reference Whalen, Best and Irwin1997). In auditory discrimination tasks, speakers make more mistakes with respect to allophonic differences than phonemic differences (Pegg & Werker Reference Pegg and Werker1997, Peperkamp et al. Reference Peperkamp, Pettinato, Dupoux, Beachley, Brown and Conlin2003), suggesting that they are less sensitive to the former. Therefore, allophonic details are often abstracted away from in phonotactic models, in order to keep the grammar concise (Hayes & Wilson Reference Hayes and Wilson2008).
However, these results do not indicate that allophones are irrelevant to perception. Some allophonic differences can be reliably heard. For example, Peperkamp et al.'s (Reference Peperkamp, Pettinato, Dupoux, Beachley, Brown and Conlin2003) AX discrimination experiment on the French allophonic pair [χ] ~ [ʁ] (voicing assimilation to the following consonant determines the choice of the allophone) and phonemically contrastive /m/ ~ /n/ showed that when the consonant appeared in coda position without any following segment, [χ] ~ [ʁ] was acoustically distinct enough that native speakers’ discrimination rate for this pair was no worse than that for the phonemic /m/ ~ /n/ contrast. Mitterer et al. (Reference Mitterer, Scharenborg and McQueen2013) and Mitterer et al. (Reference Mitterer, Reinisch and McQueen2018) argue that perceptual learning and selective adaptation, which shift listeners’ perceptual boundary between two sounds based on exposure to variants of these sounds, operate on the allophonic level; their evidence came from experimental results showing that the boundary shift between /r/ and /l/ in Dutch only occurred when the positionally appropriate allophones of these liquids were used in the exposure phase. These experiments indicate that allophonic variations are noticed by speakers, and play a role in word recognition.
To return to the question of phonotactic well-formedness: the studies reviewed above have established that at least some allophonic differences can be reliably heard, and guide word recognition. However, experiments that directly probe speakers’ phonotactic knowledge generally do not consider allophones (Coleman & Pierrehumbert Reference Coleman, Pierrehumbert and Coleman1997, Vitevitch & Luce Reference Vitevitch and Luce1999, Bailey & Hahn Reference Bailey and Hahn2001, Albright & Hayes Reference Albright and Hayes2003, Berent et al. Reference Berent, Steriade, Lennertz and Vaknin2007, Hayes & Wilson Reference Hayes and Wilson2008, Daland et al. Reference Daland, Hayes, White, Garellek, Davis and Norrmann2011, Hayes & White Reference Hayes and White2013). Two previous Chinese non-word judgement studies, one on Mandarin (Myers & Tsay Reference Myers and Tsay2005) and the other on Cantonese (Kirby & Yu Reference Kirby, Yu, Trouvain and Barry2007), also did not include any stimuli violating allophonic generalisations. The current study aims to fill this gap by examining how non-words violating allophonic rules are evaluated in acceptability judgement tasks. Using allophonic differences that can be heard and recognised from a pre-test, the study investigates whether Mandarin listeners ignore allophonic gaps, treating these stimuli like existing lexical items, or treat them as other types of phonotactic gaps. And if they are treated as lexical gaps, in terms of phonotactic acceptability, will they behave more like accidental gaps, systematic gaps or neither? Since a surface-based analysis that involves allophonic distinctions must refer to more complex phonotactic generalisations than are required in traditional underlying analysis, allophonic gaps are more likely to be accidentally missing from the lexicon, due to the higher complexity of the phonotactic generalisations involved (Wilson & Gallagher Reference Wilson and Gallagher2018). However, allophonic gaps could also be systematic: the Mandarin vowel allophony patterns to be discussed later are triggered by simple and phonetically grounded phonotactic constraints, such as backness harmony within a rhyme (Duanmu Reference Duanmu2007), similarly to non-allophonic systematic phonotactic generalisations.
Finally, given the experimental findings that listeners tend to be less attuned to allophonic differences than phonemic differences (Peperkamp et al. Reference Peperkamp, Pettinato, Dupoux, Beachley, Brown and Conlin2003, Boomershine et al. Reference Boomershine, Hall, Hume, Johnson, Avery, Elan Dresher and Rice2008), it is possible that allophonic differences are part of speakers’ perceptual grammar. This perceptual knowledge may influence the non-word judgements of allophonic gaps by reducing their deviance from real words, such that even when allophonic gaps can be correctly perceived, they will not be penalised as much as phonemic gaps. If so, we expect that the acceptability of allophonic gaps will be higher than that of both systematic and accidental gaps.
2.3 Suprasegmental information in phonotactics
Most work on phonotactics has focused on segmental phonotactics, and we know very little about how suprasegmental properties, such as lexical tones, contribute to acceptability judgements. Phonotactics may operate beyond just the segmental level. Co-occurrence patterns on segmental and suprasegmental levels are also likely to be noticed by speakers and form a part of their phonotactic grammar. For instance, in some tone languages, each syllable bears a lexical tone that distinguishes meanings, but not all lexical tones can combine with every syllable. As an example, Mandarin has four lexical tones, but the syllable [man] can only bear Tone 2, Tone 3 or Tone 4, not Tone 1. [man1] would therefore represent a tonal gap, due to a segmental–suprasegmental co-occurrence restriction in Mandarin.
These tonal gaps (missing syllable–tone combinations) behave differently from other segmental gaps, as previous non-word judgement studies have revealed that the acceptability of tonal gaps is significantly lower than real words, but also significantly higher than segmental gaps (Wang Reference Wang and Benjamin1998, Myers Reference Myers2002, Kirby & Yu Reference Kirby, Yu, Trouvain and Barry2007). Furthermore, Do & Lai (Reference Do and Yau Lai2020) attempted to incorporate tonal differences among syllables into the modelling of non-word judgements. They report that the co-occurrence probability between tones and segments had minimal effects on well-formedness ratings in Cantonese. Therefore, it seems that there is a bias against these suprasegmental restrictions, which leads to tonal gaps having greater acceptability than segmental gaps.
One possible explanation for this bias is the complexity induced by referring to both segmental and suprasegmental tiers. A noticeable property of suprasegmental phonotactic restrictions is that they are cross-tier constraints. Compared to segmental phonotactic constraints, co-occurrence restrictions among tones and segments need to refer to both the segmental and the suprasegmental tiers, rendering them formally more complex. Results from artificial grammar learning experiments have suggested that patterns with higher formal complexity are harder for speakers to acquire in experimental conditions (Moreton Reference Moreton2008, Moreton & Pater Reference Moreton and Pater2012a). Another possibility is that, similarly to allophonic gaps, perceptual factors may contribute to the phonological well-formedness of tonal gaps. Results from psycholinguistic experiments suggest that the processing of suprasegmental information, such as lexical tones, is disadvantaged in comparison to segmental information (Cutler & Chen Reference Cutler and Chen1997, Sereno & Lee Reference Sereno and Lee2015, Wiener & Turnbull Reference Wiener and Turnbull2016). For example, Cutler & Chen's Cantonese lexical decision study found that accuracy was lower for tonal gaps than for segmental gaps. It is likely that mismatches in tones are perceived less saliently than segmental mismatches in speakers’ perception grammar. We therefore hypothesise that the violation of the suprasegmental restrictions will be less severe compared to pure segmental ones, either because high-complexity patterns are harder to internalise, or because the perceptual disadvantage of tonal features makes the speakers penalise suprasegmental violations less.
2.4 Summary
The literature review above indicates that phonotactic well-formedness judgement is likely a result of the interaction between lexical statistics and various grammatical factors, such as phonetically systematic phonotactic constraints, allophonic restrictions and syllable–tone co-occurrence constraints. This forms the basis of our hypotheses on Mandarin non-word judgements. Furthermore, by modelling the speakers’ judgement data using both biased and unbiased grammars, we will show that, aside from lexical statistics and grammatical principles, multiple learning biases also affect speakers’ phonotactic knowledge, as the addition of these biases improves the well-formedness predictions of the phonotactic grammar based on grammatical principles and inductive learning of the lexicon.
3 An overview of Mandarin phonotactics
Mandarin Chinese has a number of phonological properties that make it a good test case for investigating the effects of the abovementioned factors on speakers’ gradient phonotactic judgement.
First, Mandarin displays a clearer boundary between systematic gaps and accidental gaps than languages like English. In a Mandarin syllable, consonants, glides and vowels are organised in a CGVX structure, where C = onset, G = glide, V = vowel and X = ending sound (Duanmu Reference Duanmu1990, Reference Duanmu2007) (see the inventory in (1)). For example, in the word [tʰjen] ‘sky’, [tʰ] is the onset, [j] the glide, [e] the vowel and [n] the ending sound.
-
(1)
Numerous attempts have been made to identify the systematic constraints of Mandarin phonotactics (Lin Reference Lin1989, Yip Reference Yip1989, Wiese Reference Wiese, Jialing and Smith1997, Duanmu Reference Duanmu2007). This study adopts the four constraints in (2), adapted from Yi & Duanmu (Reference Yi and Duanmu2015).
-
(2)
Sounds bearing the vocalic [+high] feature are the three glides plus the three high vowels [j w ɥ i u y]. The natural class [coronal] includes [ʦ̢ ʦ̢ʰ ʂ ʐ ʨ ʨʰ ɕ j ɥ i y], and [labial] includes [p pʰ m f w ɥ u y o]. The dental sounds [t tʰ n l ʦ ʦʰ s] are assumed by Lahiri & Reetz (Reference Lahiri and Reetz2010) to be universally underspecified for place of articulation, and are thus able to combine with the coronal glides [j ɥ], as in [tʰjen]. All the constraints in (2) are examples of the Obligatory Contour Principle; we therefore consider them to be viable criteria for the identification of systematic gaps in Mandarin.
Existing syllables in Mandarin generally do not violate these constraints. However, there are two exceptions. First, the labial consonants [p pʰ m f] can be followed by the glide [w] if the nucleus vowel is [o]. Therefore, syllables like [pwo] ‘glass’ occur, even though they violate (2d). Second, although Yi & Duanmu (Reference Yi and Duanmu2015) transcribe the word ‘to embrace’ as [yŋ], with a well-formed VX sequence, a more accurate transcription of this sequence is the GVX form [ɥuŋ], which violates (2a), as both the glide [ɥ] and the nucleus vowel [u] are [+high].
The second reason for Mandarin being an appropriate object of study for our purposes is that it has a rich set of vowel allophones, and hence provides many opportunities to investigate the contribution of allophonic restrictions to the phonotactic grammar. There are multiple analyses of the Mandarin segment inventory, both in terms of surface phones and underlying phonemes. According to Cheng (Reference Cheng1973), Mandarin has ten surface vowels, [i y u e ə ɤ o ɛ a ɑ], and two syllabic consonants, [ɹ̩] and [ɻ̩]. Lin (Reference Lin1989) notes that [e o] are lowered to [ɛ ɔ] in open syllables; and therefore adds one more vowel [ɔ] to the surface inventory.Footnote 1 To ensure that participants can reliably hear all of the allophonic differences in the well-formedness judgement task, this study will not consider the tenseness differences among the surface vowels. Instead, we include eight surface forms, [i y u e ə o a ɑ], generated from five underlying vowel phonemes, /i y u ə a/ (Duanmu Reference Duanmu2007). Moreover, the syllabic consonants [ɹ̩] and [ɻ̩] only occur after the dental and retroflex sibilants respectively, and are often analysed as a voiced prolongation of their preceding sibilants (Duanmu Reference Duanmu2007, Lin Reference Lin2007). Due to their extremely limited distributions and close connections with preceding consonants, this study will not consider [ɹ̩] and [ɻ̩] following other onset consonants (e.g. [pɹ̩], [kɻ̩]), in order to avoid creating illegal forms for the judgement task. The Mandarin vowel allophony investigated in this study is given in (3) (# represents a syllable boundary).
-
(3)
The third reason for studying Mandarin is that it has four lexical tones that distinguish meanings, namely high (T1), rising (T2), low (T3) and falling (T4). However, as noted above, these four tones occasionally do not occur with certain syllables. These missing syllable–tone combinations are known as tonal gaps. Many tonal gaps are the result of historical sound changes, and can be easily filled in loanwords, neologisms and onomatopoeic words (Duanmu Reference Duanmu, van Oostendorp, Ewen, Hume and Rice2011). Tonal gaps are not evenly distributed across the four lexical tones. Most tonal gaps are rising tone gaps, followed by high, low and falling. Jin & Lu (Reference Jin and Lu2018) report that the frequency of distribution of the four types of tonal gaps influences the gradient acceptability of toned syllables.
This summary of the basic patterns of Mandarin phonotactics indicates that missing syllables in Mandarin fall into four types: systematic gaps, accidental gaps, allophonic gaps and tonal gaps. The following section reports a Mandarin syllable acceptability judgement experiment based on these four types of missing syllables. The experiment serves two purposes. First, it tests the independent roles of these categories in Mandarin phonotactics. Second, and more importantly, it provides acceptability data from native speakers that are needed to evaluate the performance of theoretical models of phonotactics.
4 The Mandarin syllable acceptability judgement experiment
4.1 Methods
Thirty-one native Mandarin speakers (mean age = 24.53, SD = 6.68), born and raised in Northern China, were recruited for the current experiment. None of the participants reported any speech or hearing problems.
We used the phonotactic properties described above to design the stimuli for the syllable acceptability judgement experiment. An exhaustive list of all theoretically possible Mandarin syllables (both existing and missing) was constructed from the factorial combination of all possible surface sounds in the Mandarin CGVX syllable structure. In this structure, only the vowel is obligatory, so the C, G and X slots can be empty. Tonal distinctions were not considered, and all syllables used in the study carried high tone. The factorial combination of all sounds plus empty slots gave rise to (21 + 1) × (3 + 1) × 8 × (4 + 1) = 3520 possible syllables, of which 384 were existing (Chen & Li Reference Chen and Li1994) and 3136 were missing.Footnote 2 Syllables containing the syllabic consonants [ɹ̩] and [ɻ̩] were not included.
Perceptual illusion and misperception are likely to occur when speakers hear stimuli containing sequences that are phonotactically illegal in their native language, where illegal sequences tend to be assimilated to sequences that are legal (Massaro & Cohen Reference Massaro and Cohen1983, Hallé et al. Reference Hallé, Segui, Frauenfelder and Meunier1998). For example, Japanese listeners tend to perceive an additional vowel between the consonants in VC1C2V sequences when the C1C2 sequence is impossible in Japanese, e.g. [ebzo] heard as [ebuzo] (Dupoux et al. Reference Dupoux, Kakehi, Hirose, Pallier and Mehler1999, Dupoux et al. Reference Dupoux, Parlato, Frota, Hirose and Peperkamp2011). Similarly, English listeners have also been reported to hear an illusory schwa in illicit onset consonant clusters, for example [bnif] heard as [bənif] (Pitt Reference Pitt1998, Berent et al. Reference Berent, Steriade, Lennertz and Vaknin2007). Speakers’ native phonological systems may prevent them from accurately perceiving a phonotactically illegal sequence.
To ensure that non-word stimuli were perceived as intended, not as legal forms or some other perceptually similar forms, we first ruled out syllables that may lead to perceptual illusion, based on the criteria in (4).
-
(4)
Criteria (a)–(c) are motivated on typological grounds: these distinctions are not known to exist cross-linguistically. For (a) and (b), Steriade (Reference Steriade1994) states that although the high vowels [i u y] can freely occur in different contexts, their glide counterparts, [j w ɥ], are often subject to distributional restrictions. For instance, in French, [ɥ] only occurs before [i], not before other high vowels. Moreover, these distinctions are difficult to hear even for linguistically trained native speakers, and these transcriptions are often used interchangeably by Chinese linguists, sometimes even by the same scholar. For instance, for (c), Duanmu (Reference Duanmu1994) uses [ʨ] and [ʨw], whereas Duanmu (Reference Duanmu2007) has [ʨj] and [ʨɥ]. The motivation for (d) comes from the finding that the pronunciation of the high back vowel before the velar nasal is more open and lax (Chao Reference Chao1968), which makes it easily confusable with [o]. For (e), studies from loanword phonology show that the allophonically impossible [aŋ] was consistently perceived as [an] by native speakers, and [ɑn] as [ɑŋ] (Hsieh et al. Reference Hsieh, Kenstowicz, Mou, Calabrese and Leo Wetzels2009). These criteria identified 1273 syllables as indistinguishable from some other syllables. The remaining list therefore contains 1863 missing syllables and 384 existing syllables. According to Chen & Li (Reference Chen and Li1994), among the 384 existing syllables, 63 of them happen not to take the high tone; these will be referred to as tonal gaps. The remaining 321 syllables are real words.
The missing syllables were further divided into 434 allophonic gaps, which are gaps that only violate the allophonic rules of Mandarin, 1041 systematic gaps, which are gaps that violate one or more of the four major phonotactic constraints of Mandarin (Yi & Duanmu Reference Yi and Duanmu2015), and 388 other segmental phonotactic gaps, the gaps that remain unexplained by the four constraints. We refer to these as accidental gaps.
Table I summarises the different types of syllables discussed so far. For example, [wei] ‘micro’ is a real word. [ʐan1] is a tonal gap, because [ʐan] cannot bear a high tone, but it can occur with other tones, for example [ʐan3] ‘to dye’, with a low tone. [ʂuŋ] is missing, and does not violate any of the constraints listed in (2); therefore, it is an accidental gap. [mui] is a systematic gap, because it violates constraint (2a), *[+high][+high]. [njeu] is an allophonic gap, because it has the wrong mid vowel allophone: [o] instead of [e]. [ljoi] is a gap violating both Mandarin phonotactics (2b) and an allophonic rule (the mid vowel should be front [e] before the offglide [i], instead of back [o]). According to the definitions above, it is considered to be a systematic gap, not an allophonic gap.
Table I Syllable categorisation.

The types in bold are the five stimulus groups in this study. Forty syllables were randomly selected as the test stimuli for each type, making a total of 200 stimulus syllables. The word-to-non-word ratio is 1∶4. On the one hand, this avoids having too many existing syllables, which would run the risk of turning the experiment into a lexical decision task and polarising the rating results; on the other hand, it allows us to collect sufficient data on real words to allow a comparison with other word types. The complete list of all test stimuli is given in Table IV in the online Appendix.Footnote 3
An AX discrimination pre-test was carried out, to ensure that the allophonic differences that remained in the stimulus list could be perceived by the participants. Stimuli for the pre-test consisted of pairs of syllables, where forms violating an allophonic generalisation were paired with corresponding words without the allophonic violation. For example, the study assumed three allophones for the mid vowel, so there were three sets of environments to host them: (i) w _ #, or _ u; (ii) {j, ɥ} _ #, or _ i; (iii) _ {n, ŋ, #}. For each environment set, say after [j ɥ] or before [i], we could insert three allophones to yield one allophonically possible syllable (e.g. [tei]) and two impossible ones (e.g. [toi] and [təi]). The three items yield six different pairings: AA, BB, CC, AB, AC and BC. This process was repeated for the other five environment sets (another two sets for the mid vowel and three for the low vowel). Two different onsets were used for each rhyme pair. Altogether participants heard 6 × 6 × 2 = 72 pairs (see Table III in the Appendix for the full list).Footnote 4 Three syllables, [tei], [pən] and [tən], occurred as stimuli in both the pre-test and the main judgement task. All syllables in the pre-test were normalised for pitch, intensity and duration. For each trial, a pair of stimuli with an intervening 500 ms pause was played, then a fixation cross appeared at the centre of the screen, together with a text instruction asking whether the participant thought the two sounds were the same or different. Participants then responded using the keyboard; the S key for ‘yes’, and the L key for ‘no’. After the response was received, the screen turned blank for another 500 ms, then the next trial began. Participants were instructed to make a judgement as quickly and accurately as possible. Ten practice pairs (without any feedback) were provided before the pre-test to familiarise participants with the task. Participants’ yes/no responses were recorded. Overall, the accuracy rate for the pre-test was 91.3%. We therefore concluded that the allophonic differences used in the main test stimuli could be reliably heard by the participants.Footnote 5
The stimulus syllables were recorded with a high tone by a phonetically trained male native Mandarin speaker in an anechoic chamber. All stimuli were normalised for peak intensity using Praat (Boersma & Weenink Reference Boersma and Weenink2017). Pitch and duration were not normalised, in order to preserve the naturalness of the stimuli. The stimuli had a mean duration of 555 ms (SD = 75).
The main task was an auditory syllable well-formedness judgement task for the 200 test stimuli described in the previous section. The test was carried out on a Lenovo laptop, using Paradigm software.Footnote 6 Participants listened to the stimuli using earphones connected to the laptop and were asked to rate the test stimuli as Mandarin syllables on a Likert scale from 1 (bad) to 7 (good). No written forms were given, because allophonic gaps cannot be represented orthographically. In each trial, the stimulus was played, and then seven buttons with number tags (1–7) appeared on the screen, together with a text instruction asking the participants to click on one of the buttons to rate the acceptability of the syllable they just heard. The task was self-paced, without any time limit. After the participant responded, there was a 500 ms pause preceding the onset of the next trial; the screen was left blank during the pause. Five practice trials, one for each syllable type, were provided prior to the 200 main stimuli, which were presented in a randomised order. Again, these practice trials were intended for familiarisation, and no feedback was provided. Participants’ rating responses were recorded.
4.2 Data analysis
One participant's data deviated from all the others: he gave a score of 1 (the lowest rating score) for 196 out of all 200 test items (98%), including most of the real words. His data were excluded from analysis. To reduce the impact of the varying uses of the rating scale by subjects and to achieve better normalisation (Cowart Reference Cowart1997), the raw rating scores were transformed to z-scores based on all the data points of each participant. This facilitates the convergence of the computationally intensive mixed-effects models (Bates et al. Reference Bates, Mächler, Bolker and Walker2015).
Neighbourhood density was used as a covariate to represent the lexical statistics effects on non-word judgement in the current study. It is defined as the number of words generated by substituting, deleting or adding a single phoneme together with their summed frequency (Greenberg & Jenkins Reference Greenberg and Jenkins1964). Even though the stimulus construction process ignored tonal distinctions, they were taken into consideration when searching for lexical neighbours, as previous work has shown that including tonal neighbours in neighbourhood density counts improves the correlation between neighbourhood density and reaction time in lexical decision tasks (Yao & Sharma Reference Yao and Sharma2017). For example, the form [ku1] would have [ku3] and [ku4] as its neighbours. The neighbourhood density was also weighted by each neighbour's homophone density in Chen & Li (Reference Chen and Li1994).Footnote 7 For example, the non-word stimulus [pyŋ1] has two neighbours, [pəŋ1] and [pɑŋ1]. According to the list, [pəŋ1] has two homophones and [pɑŋ1] has three. Therefore, the final neighbourhood density for [pyŋ1] is 2 + 3 = 5. The neighbourhood density calculations were carried out using surface forms rather than underlying representations, so that [pan1] and [pɑŋ1] were not counted as neighbours, even though underlyingly they are (/pan1/ ~ /paŋ1/). Other possible lexical measures (e.g. biphone transitional probability) were not included, because they are often highly correlated with neighbourhood density (Vitevitch & Luce Reference Vitevitch and Luce1999). Additionally, unlike transitional probabilities, which can only capture local restrictions, neighbourhood density can to some degree reflect long-distance co-occurrence restrictions. For instance, if [+labial] V [+labial] is unattested in the lexicon, then replacing the V will necessarily lead to a non-word. Therefore, the sequence is more likely to have lower neighbourhood density, as only the manipulation of the onset and coda consonants can lead to lexical neighbours.
The z-scores of the rating judgements calculated on the basis of each participant's mean rating were then fitted with a mixed-effects linear regression model, with stimulus types (Type) and homophone-weighted neighbourhood density (ND) and their interaction as fixed effects; the random effects were the slopes for each participant for the five stimulus types (Type) and the intercepts for items. The random intercepts for participants were not included, as the mean of each participant's ratings was zero after by-participant z-score transformation. Although the duration of the test items was not normalised, its potential effect on the rating results can be captured by the item random intercepts. For the categorical variable Type, Real word was set as the baseline for comparison. All analyses were conducted using the lme4 package (Bates et al. Reference Bates, Mächler, Bolker and Walker2015) in R, and p-values were obtained using the lmerTest package (Kuznetsova et al. Reference Kuznetsova, Brockhoff and Christensen2017).
4.3 Results
In searching for the optimal fixed-effects structure, we conducted a series of linear mixed-effects analyses. Fixed-effects factors were added one by one, and likelihood ratio tests were performed to see if they significantly improved the model. This process determined that the best model for the ratings is the full model that includes Type, ND and their interaction. The model's parameter estimates are shown in Table II.
Table II The best model for ratings.

The effect of Type stands out even with ND in the model. Figure 1 illustrates that the acceptability of real words is the highest, followed by tonal gaps, allophonic gaps, accidental gaps and systematic gaps. Post hoc multiple comparisons with Holm's p-value adjustments suggested that, except for the difference between tonal and allophonic gaps (χ 2 = 3.7513, p = 0.0528), the ratings of all other pairs among the five stimulus types were significantly different from each other (χ 2 ≥ 6.8884, p ≤ 0.0174). Neighbourhood density had a positive parameter estimate for real words, but the effect was not significant. It had a greater positive effect on ratings for the other stimulus types than for real words, as indicated by the positive parameter estimates for the interactions, but this difference was only significant for the accidental gaps.
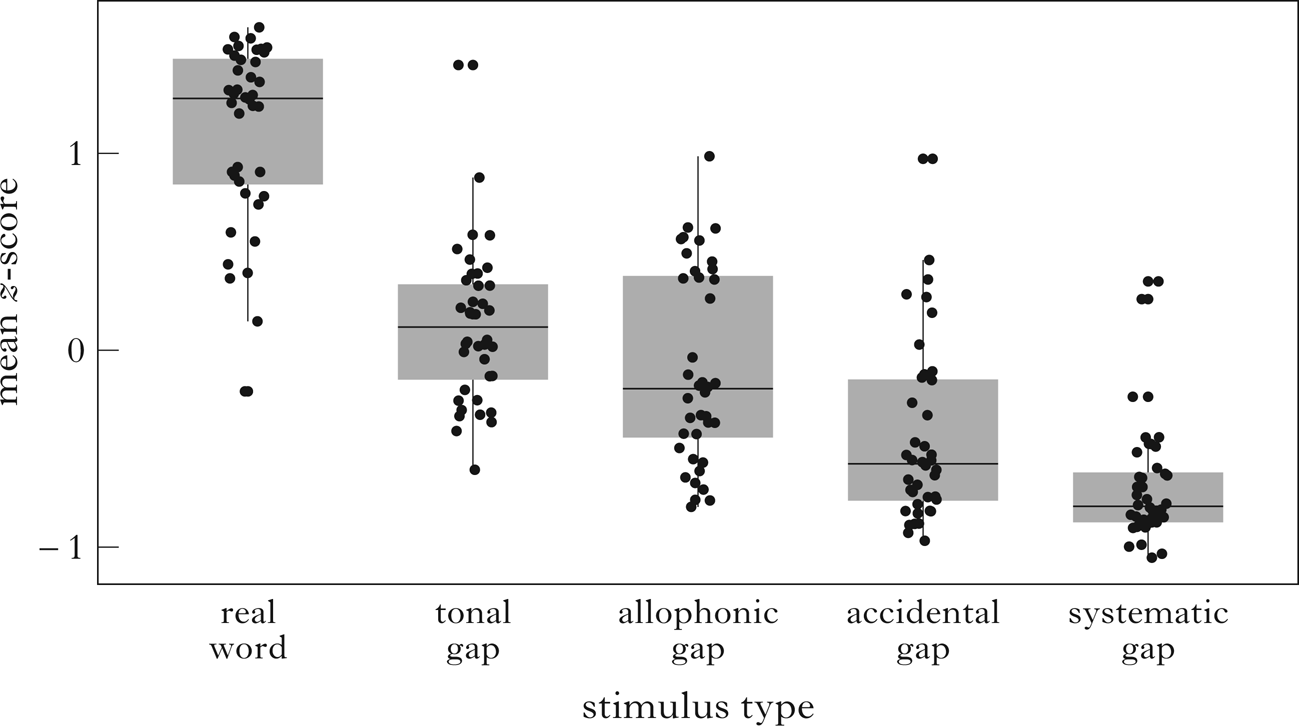
Figure 1 Mean z-scores of well-formedness ratings by stimulus types. Each dot represents the mean z-score transformed acceptability rating of one test stimulus. Boxes indicate the range between the first and third quartiles. Whiskers delimit the minimum and maximum data points, excluding any outliers.
4.4 Discussion
The results of the experiment showed that Mandarin speakers’ non-word judgement was mainly modulated by grammatical factors (the five stimulus types); lexical statistics in the form of neighbourhood density alone could not explain all of the variance. Allophonic gaps behaved neither like real words nor like systematic gaps, but more similarly to tonal gaps. This indicates that the phonotactic grammar is not blind to allophonic variations, yet at the same time speakers are not as sensitive to allophonic restrictions as to systematic phoneme-level phonotactic violations, even when the allophonic violations can be reliably heard. Given that the pre-test showed that the allophonic differences investigated in the non-word judgement task were easily perceivable by native Mandarin speakers, the higher acceptability of allophonic gaps is less likely to be due to misperception. But we cannot entirely rule out the possibility that Mandarin speakers’ perception of these allophonic differences was not as good as their perception of phonemic differences, as we did not directly test for phonemic differences in our AX discrimination pre-test.
Neighbourhood density, in general, had a positive effect on acceptability ratings, replicating previous findings in Bailey & Hahn (Reference Bailey and Hahn2001) and Kirby & Yu (Reference Kirby, Yu, Trouvain and Barry2007). Myers & Tsay's (Reference Myers and Tsay2005) finding that higher neighbourhood density resulted in lower ratings in the judgements of Mandarin non-words were not replicated. Instead, our results suggest that the effect of neighbourhood density was positive in both real words and non-words, and that the effect was stronger for non-words, even though the stronger trend was only significant for the accidental gaps. Our results demonstrate that various grammatical factors, including phonetically systematic phonotactic constraints, allophonic restrictions and onset–tone co-occurrence constraints, can influence phonotactic acceptability. In the next four sections, we use computational strategies to mimic the process of phonotactic learning, and compare the modelling results with the acceptability judgement data we collected. In so doing, we investigate whether the statistical properties of the lexicon and the grammatical constraints abstracted from them can predict speakers’ non-word judgements, or whether additional learning biases are needed for accurate prediction.
5 Building a model for speakers’ phonotactic knowledge
Numerous phonotactic models have been proposed to explain speakers’ gradient phonotactic knowledge. Almost all phonotactic models operate on n-grams as the basic descriptive structure; the elements of the n-grams can be segments (Jurafsky & Martin Reference Jurafsky and Martin2009, Vitevitch & Luce Reference Vitevitch and Luce2004), phonological features (Albright Reference Albright2009) or a combination of the two (Futrell et al. Reference Futrell, Albright, Graff and O'Donnell2017). In addition, some models also make use of syllabic and prosodic structures to make phonotactic generalisations (Coleman & Pierrehumbert Reference Coleman, Pierrehumbert and Coleman1997, Phillips & Pearl Reference Phillips and Pearl2015).
Some of these phonotactic models posit that the well-formedness of non-words is evaluated by directly comparing how similar a non-word is to other existing lexical entries. For example, as mentioned in §2.1, lexical measures such as phonotactic probability and neighbourhood density directly compare all of the lexical entries in the lexicon without referring to other linguistic structures. In contrast, other models may resort to linguistic concepts such as feature and syllable structure to measure the well-formedness of non-words. Rather than treating each segment as a distinct unique type, the featural bigram model deploys phonological features, so that each segment may be characterised by the natural classes to which it belongs; co-occurrence probabilities of natural classes are then calculated in lieu of segmental bigram probabilities (Albright Reference Albright2009). Hayes & Wilson's (Reference Hayes and Wilson2008) UCLA Phonotactic Learner also operates on feature co-occurrences: the learner attempts to identify a set of feature-based markedness constraints and their constraint weights that maximise the probability of the input forms.
Using the Sonority Sequencing Principle in consonant clusters as the testing ground, Daland et al. (Reference Daland, Hayes, White, Garellek, Davis and Norrmann2011) evaluate the performance of some phonotactic models, including the classical bigram model (Jurafsky & Martin Reference Jurafsky and Martin2009), the featural bigram model (Albright Reference Albright2009), the syllabic parser (Coleman & Pierrehumbert Reference Coleman, Pierrehumbert and Coleman1997), phonotactic probability (Vitevitch & Luce Reference Vitevitch and Luce2004), the generalised neighbourhood model (Bailey & Hahn Reference Bailey and Hahn2001) and the UCLA Phonotactic Learner (Hayes & Wilson Reference Hayes and Wilson2008). They generated CCVCVC non-words with attested or unattested onset clusters (violating the Sonority Sequencing Principle), and asked native English speakers to rate these non-words. They then fed the English lexicon to these phonotactic models, trained the models to make predictions on the test stimuli and measured the correlation between the predicted results and the real acceptability rating data from the participants. The results suggested that, although all models made good predictions with respect to the phonological well-formedness of these non-words, the model that showed the strongest correlation was the UCLA Phonotactic Learner. In addition, this learner was also shown to have successfully modelled a variety of other phonotactic phenomena, including OCP effects and vowel harmony (Hayes & Wilson Reference Hayes and Wilson2008, Colavin et al. Reference Colavin, Levy and Rose2010, Gallagher Reference Gallagher2013, Wilson & Gallagher Reference Wilson and Gallagher2018).
Hayes & White (Reference Hayes and White2013) trained the learner to acquire a phonotactic grammar of English consonant clusters. The resulting grammar was a combination of phonetically natural and unnatural constraints. Both types of constraints were assigned equivalent weights by the learner, because they were true generalisations of the English lexicon. However, when the acceptability ratings of non-words violating natural constraints and non-words violating unnatural constraints were compared, only the former received lower ratings than the control items that violated no other constraints, whereas non-words violating unnatural constraints showed little to no effect. This is reminiscent of ‘the surfeit of the stimulus’ effect reported in Becker et al. (Reference Becker, Ketrez and Nevins2011), in which some lexical trends in Turkish phonotactics could be productively extended to wug words, while some other equally salient trends could not. Many other wug test productivity studies have demonstrated that speakers’ phonotactic knowledge is not a simple reflection of the statistical patterns in the lexicon (Hayes & Londe Reference Hayes and Londe2006, Zuraw Reference Zuraw2007, Moreton Reference Moreton2008, Hayes et al. Reference Hayes, Zuraw, Siptár and Londe2009, Becker et al. Reference Becker, Ketrez and Nevins2011). In addition, evidence from artificial grammar learning experiments demonstrates that speakers are biased learners of language patterns; some patterns are easy to learn, whereas others are more difficult (Moreton & Pater Reference Moreton and Pater2012a, Reference Moreton and Paterb). If we view the phonotactic models as simulations of the speakers’ learning of the phonotactic patterns in the lexicon, the discrepancy between model prediction and acceptability judgements can be understood as the biases that speakers have during this learning, biases that lead them to behave differently from what is predicted from lexical statistical patterns alone.
A successful phonotactic model should therefore not only capture the statistical properties of the lexicon, but also account for the effects of these linguistic biases. Hayes & White (Reference Hayes and White2013) examine the phonetic naturalness bias in phonotactic judgement. It is worth investigating whether the same naturalness bias exists in Mandarin phonotactic knowledge as well, since the distinction between the systematic and accidental gaps in our experiment was based on a phonetically grounded principle: whether the gap violates the OCP. We address the issue of whether grammatical principles like the OCP are directly accessible from the lexicon during phonotactic learning. If the OCP effect is a salient characteristic of the Mandarin lexicon, speakers may directly incorporate this constraint into their phonotactic knowledge by being exposed to the lexicon without the help from a naturalness bias to identify systematic gaps as exceptionally ill-formed. But if there is a mismatch between the prediction made by the inductive generalisations of the lexicon and speakers’ non-word judgement, this would suggest that an additional bias is required to accurately capture how the speakers have internalised the strength of OCP constraints projected from the lexicon.
Our experimental results also suggest that allophonic restrictions can affect speakers’ acceptability judgements. Few previous studies have attempted to model allophonic relations in the lexicon; however, we are interested in whether current phonotactic models are able to account for the judgement variance caused by allophony, especially for languages with rich allophony, like Mandarin. Transcribing the lexicon using surface representations allows the model to access phonotactic patterns on the allophonic level, so that allophonic relations can be successfully modelled via inductive learning of the lexicon. Furthermore, since perceptual distinctiveness among allophones is less salient than phonemic contrasts (Jaeger Reference Jaeger1980, Pegg & Werker Reference Pegg and Werker1997, Peperkamp et al. Reference Peperkamp, Pettinato, Dupoux, Beachley, Brown and Conlin2003, Boomershine et al. Reference Boomershine, Hall, Hume, Johnson, Avery, Elan Dresher and Rice2008), it is possible that the inductive learning process will overestimate the effect of allophonic violations, because the learning algorithm has no access to the allophonic relations among the segments and will treat allophonic and phonemic gaps equally. If the allophonic gaps are judged by speakers to be more well-formed than predicted by the phonotactic model, this would suggest that an allophony bias exists in the phonotactic knowledge to downplay the violations of allophonic constraints.
We are also interested in how the learning of co-occurrence constraints between tones and segments can be modelled. Our results showed that tonal gaps were generally rated as closer to real words than other types of non-words. This agrees with the results from earlier studies showing that these types of restrictions do not have as strong an impact on acceptability (e.g. Do & Lai Reference Do and Yau Lai2020). These suggest that there is a bias against the learning of this type of co-occurrence constraints, and that the basis for the bias could be structural complexity (Pycha et al. Reference Pycha, Nowak, Shin and Shosted2003, Moreton Reference Moreton2008) or perception (Cutler & Chen Reference Cutler and Chen1997, Sereno & Lee Reference Sereno and Lee2015, Wiener & Turnbull Reference Wiener and Turnbull2016), as discussed in §2.3.
6 Building a Mandarin phonotactic grammar
6.1 The UCLA Phonotactic Learner
The UCLA Phonotactic Learner (Hayes & Wilson Reference Hayes and Wilson2008) was used to build a phonotactic grammar for Mandarin. The learner starts with a feature matrix that defines the segments of a language and a procedure to create constraints consisting of feature combinations to penalise illegal or infrequent segment sequences. The output of the learner is a grammar of weighted constraints, whose weights are assigned based on the principle of maximum entropy (Goldwater & Johnson Reference Goldwater, Johnson, Spenader, Eriksson and Dahl2003). The phonotactic constraints of the learned grammar are determined by searching through possible markedness constraints that ban certain features or feature combinations using a set of search heuristics encoded in the learner, and the weights of the constraints are determined by the maximum entropy principle. The learning stops when the number of constraints in the grammar reaches a user-set maximum. For more details about maximum entropy grammar and the learning procedure used in the learner, see Hayes & Wilson (Reference Hayes and Wilson2008).
We can then use the output grammar to evaluate the phonological well-formedness of any sound sequence x, which will return a penalty score h(x), defined as the sum of the product of the weight w of each constraint C in the grammar that the sequence violates and the number of times the sequence violates that constraint, as in (5a).
-
(5)
The MaxEnt value of the penalty score h(x) is defined as e raised to the negative power of the penalty score h(x), as in (5b). In maximum entropy grammar, the MaxEnt value of a form is proportional to its probability of occurrence.
6.2 Procedures
Phonotactic learning starts with an input lexicon. The input data for the phonotactic learning were derived from Tsai (Reference Tsai2000), which contains 1238 syllables with tonal distinctions. It iterated through the 111,417 words included in Hsiao et al. (Reference Hsiao, Tsai, Hsieh, Yeh and Tan2013), and counted the frequency of each syllable, defined as the number of characters that share the syllable's pronunciation). The input lexicon comprised 1238 syllables weighted by their character type frequency, which roughly corresponds to morpheme type frequency, as cases where two morphemes share the same character and the same pronunciation (e.g. 花 [xwa1], which can mean either ‘flower’ or ‘to spend’) are rare.
This input lexicon was transcribed according to the inventory in (1). The syllabic consonants [ɹ̩] and [ɻ̩] were coded as [i], because these three phones are in complementary distribution. Lexical tones were encoded with upper-case letters (H for T1, R for T2, L for T3, F for T4) at the beginning of each syllable, in order to capture any onset–tone interactions. The feature set defining the sounds in the input lexicon is provided in Table IV in the Appendix. The feature specification follows Hayes (Reference Hayes2009), with four additional features, [High], [Rising], [Low] and [Falling], representing the four lexical tones.
We first trained the learner to learn 1000 constraints, in order to obtain a list of natural classes the learner could derive from the data. Then, based on these natural classes and the Mandarin phonotactic properties discussed in §3, we constructed a handwritten grammar with 40 constraints: six systematic constraints representing the four systematic phonotactic constraints in (2), 16 other segmental accidental constraints, 17 allophonic constraints representing the allophonic rules in (3) and one onset–tone co-occurrence constraint penalising syllables with a sonorant onset with high tone. The weights of this set of handwritten constraints were then trained by the learner using the ‘Reweight the constraints of an existing grammar’ function. The output of the learner is a weighted 40-constraint phonotactic grammar based on feature co-occurrence restrictions.
There are two reasons for using handwritten constraints. First, the handwritten constraints allow us to better control the effect of each constraint, so what the role that each constraint plays in the phonotactic grammar is clear. We found that a majority of machine-learned constraints serve complex functions and cannot be clearly categorised according to what type of gaps (stimulus types) they rule out. For example, the machine learner learned a constraint *[―back][―lo, ―fr], banning sequences of [ʨ ʨʰ ɕ j ɥ i y e ɤ a] + [w u ə o]. Part of this constraint does the work of the systematic constraint *[+high][+high], because it penalises combinations such as [ju], [ɥu] and [iu]. But it also carries the function of an allophonic constraint, since allophonically impossible forms like [jə], [jo], [ɥə] and [ɥo] are also penalised by this constraint. This will prove to be problematic when we assess the role of each type of phonotactic generalisation in the speakers’ non-word judgement and make specific proposals on how the learned grammar can be improved. Second, given the same number of constraints, the machine-learned grammar performed worse than the handwritten grammar. When we trained the learner to produce a 40-constraint grammar (matching the number of constraints in the handwritten grammar) and used this machine-learned grammar to assign penalty scores for the 200 stimulus syllables in the experiment, correlation tests between the MaxEnt values of the penalty scores and the well-formedness judgement data showed that the handwritten grammar outperformed the machine-learned grammar by a wide margin (r = 0.735 vs. r = 0.585).
The number of systematic constraints in the handwritten grammar is more than four because the constraint Articulator Dissimilation cannot be generalised as a single constraint using the natural classes compatible with the learner. We therefore divided this constraint into three sub-constraints: *[―approx, +lab][+rd, ―syll] accounts for the labial co-occurrence effect in CG sequences, *[―ant][+approx, +fr, ―syll] accounts for the coronal co-occurrence effect and *[―son, +hi, ―cor][+approx, +hi, +fr] accounts for the dorsal co-occurrence effect. For the same reason, each allophonic rule in (3) is often realised by multiple constraints in the handwritten grammar as well. For instance, rule (3a) stipulates that the mid vowel becomes [o] either after [w] in open syllable or before [u]. In terms of constraints, this means that the other two allophones of the mid vowel, [ə] and [e], cannot occur in these environments. Therefore, two allophonic constraints are proposed to capture the role of this allophonic rule: *[―fr, ―syll][―hi, ―lo, ―back][+#], and *[―hi, ―lo, ―back][+hi, ―fr, +syll]. They ban sequences like [wə#], [we#], [əu] and [eu], which are all allophonically impossible. For a list of these constraints, see Table V in the Appendix.
We can use this output phonotactic grammar to evaluate any form and generate a penalty score (see (5a)). The correlation between the penalty scores assigned by the grammar and speakers’ well-formedness judgements can then serve as a measure of the performance of the model. The z-score transformed well-formedness ratings for thirty participants are averaged for each syllable. The penalty scores of each syllable are transformed to their MaxEnt values, because the distribution of the penalty scores is extremely right-skewed. In addition, the MaxEnt values are proportional to probability, which has direct theoretical implications (Hayes & Wilson Reference Hayes and Wilson2008). Pearson's correlation coefficient between the speakers’ judgements and the MaxEnt values of the model penalty scores can then be calculated, where r = 0.735.Footnote 8 This correlation is weaker than the English onset cluster case study reported in Hayes & Wilson (Reference Hayes and Wilson2008) (r = 0.946), presumably because we are here trying to model the entire phonotactic properties of a language. Figure 2 illustrates the prediction of this handwritten grammar. Notice that the higher the MaxEnt value for a form, the more grammatical it is. This grammar in general replicates the participants’ gradient acceptability among stimulus types reported in Fig. 1, except that it predicts that allophonic gaps are less acceptable than accidental gaps.
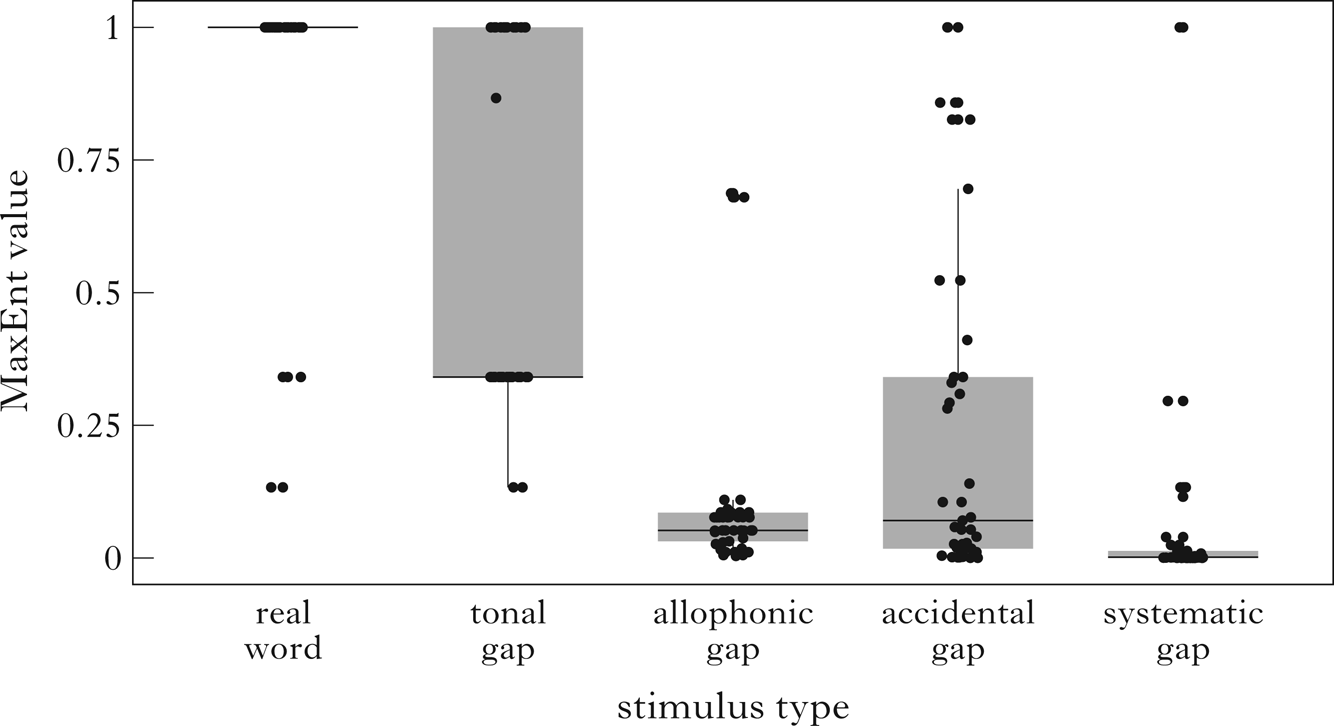
Figure 2 MaxEnt values of penalty scores predicted by the original handwritten grammar grouped by stimulus type. Each dot represents the MaxEnt value of one test stimulus.
Compared to traditional lexical statistics measures such as phonotactic probability and neighbourhood density, whose computations are based on atomic segmental representations, the UCLA Phonotactic Learner is equipped with various linguistic properties, such as phonological features and autosegmental tiers. However, the learning algorithm behind it is designed to maximise the probability of all the forms in the input lexicon. Therefore, the outcome of the phonotactic learner is still by and large based on the statistical properties of the lexicon (Hayes & Wilson Reference Hayes and Wilson2008, Daland et al. Reference Daland, Hayes, White, Garellek, Davis and Norrmann2011, Hayes & White Reference Hayes and White2013). Furthermore, the constraints of the phonotactic grammar we built are all handwritten, which means that grammatical principles of phonological theory are incorporated into the grammar, as well as the lexical statistics. Even so, as indicated by the correlation coefficient between the model-predicted well-formedness and speakers’ well-formedness judgements, the prediction of this enriched phonotactic grammar does not fully match the speakers’ judgement data. For example, the most salient mismatch is that the well-formedness of allophonic gaps predicted by the learned grammar is much lower compared to the speakers’ judgements. These mismatches suggest that grammatical principles with weights directly deduced from the lexicon are not sufficient to explain all of the variation in phonotactic judgements. Additional learning biases may also contribute to speakers’ phonotactic knowledge. The next section introduces these learning biases, and examines whether the biased grammar offers more accurate well-formedness predictions, as measured by the correlation between model prediction and speakers’ rating data.
7 Implementing biases
In this paper, we have adopted Hayes & White's (Reference Hayes and White2013) post hoc method to introduce learning biases into the MaxEnt phonotactic grammar by adjusting the weights of individual constraints in the output grammar after the weights have been trained.
Based on the phonotactic properties of the Mandarin lexicon and our experimental results, we introduced three types of biases by adjusting the weights of the according types of constraints in the handwritten grammar. First, all systematic constraints in the handwritten grammar are based on the OCP, and these constraints account for a significant number of missing syllables in Mandarin (Yi & Duanmu Reference Yi and Duanmu2015); the OCP effect is thus a salient feature of Mandarin lexicon. We are interested in whether the weights of OCP constraints are accurately assessed during the inductive learning of the lexicon, or whether the phonotactic learner still underlearns their effects. The adjustment of the weights of systematic constraints represents a naturalness bias (Hayes & White Reference Hayes and White2013). Second, the richness of allophony in Mandarin allowed us to examine the acceptability of allophonic gaps. The closer perceptual distance among allophonic differences compared to phonemic differences (Peperkamp et al. Reference Peperkamp, Pettinato, Dupoux, Beachley, Brown and Conlin2003, Boomershine et al. Reference Boomershine, Hall, Hume, Johnson, Avery, Elan Dresher and Rice2008) predicts that allophonic gaps should be rated as more acceptable than other segmental gaps, and this is indeed confirmed by our experimental results. However, the well-formedness predictions of the unbiased grammar with respect to allophonic gaps were much lower than the speakers’ judgements, indicating that the effects of the allophonic constraints are overlearned by the phonotactic learner. The adjustment on the weights of allophonic constraints is considered as a bias on allophony. Third, the onset–tone interaction constraint crosses the segmental and the suprasegmental tiers. We also tried to adjust the weight of this constraint to see if there was any bias on suprasegmental features in phonotactics.
We implemented the biases by upgrading or downgrading the constraint weights in the handwritten grammar produced by the phonotactic learner (Table III in the Appendix). We multiplied the weights of the three types of constraints by a factor ranging from 0 to 2. A value between 0 and 1 downgrades the constraints in the grammar, and a value between 1 and 2 upgrades the effects of the constraints. We then used the biased grammar to assign penalty scores to all the test stimuli, and checked the correlation between the MaxEnt values of the penalty scores and the participants' ratings to see if the biased grammar generated better predictions than the original handwritten grammar. All factors between 0 to 2 were simulated, with an increment of 0.1; the factors for each type of constraints varied orthogonally. The best-fit factor set that maximised the correlation was 0.6 for systematic constraints, 0.3 for allophonic constraints and 0.7 for the tonal constraint; the correlation coefficient increased from r = 0.735 to r = 0.760. Figure 3 shows that, after the biases were introduced, the predicted well-formedness of the allophonic gaps increased significantly and was more acceptable than the accidental gaps (cf. Fig. 2). The best-fit factors for the three constraint types being modified were all below 1, meaning that the effects of systematic constraints, allophonic constraints and tonal constraints were all overlearned by the phonotactic learner.Footnote 9
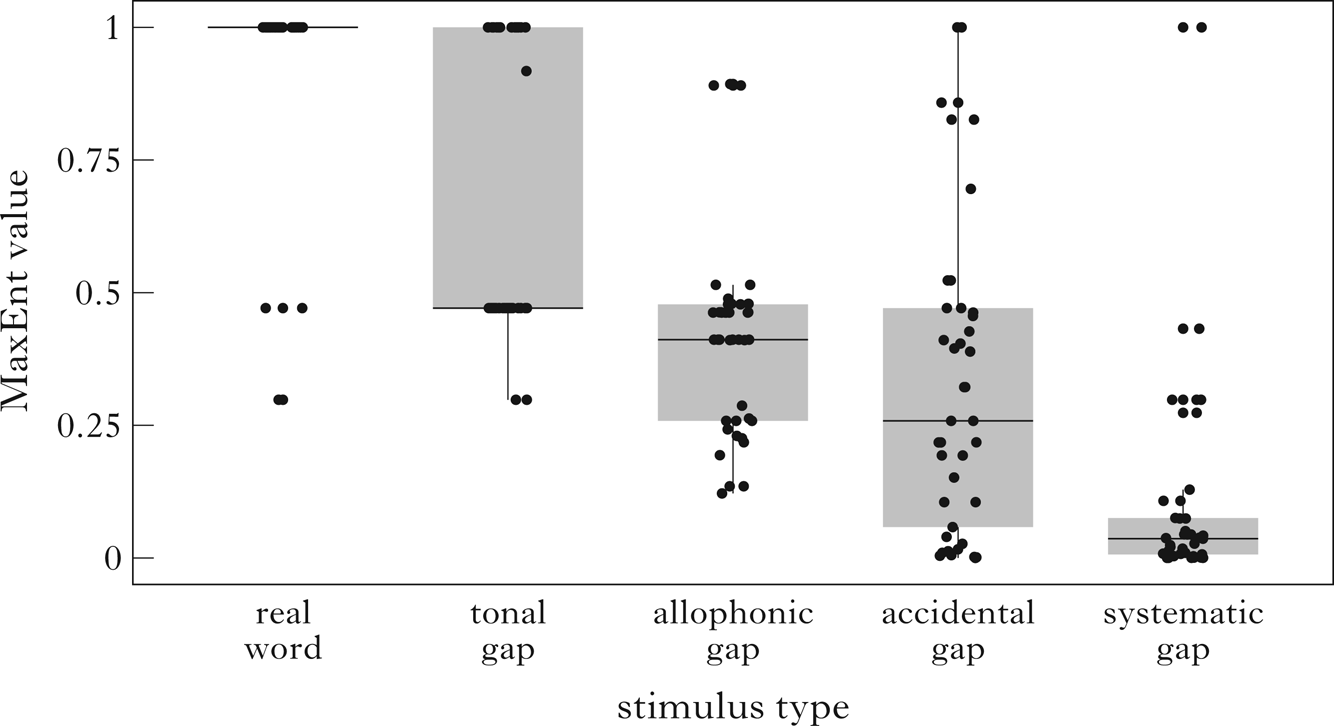
Figure 3 MaxEnt values of penalty scores predicted by the biased handwritten grammar grouped by stimulus type. Each dot represents the MaxEnt value of one test stimulus.
8 Discussion
Our modelling procedure showed that, by introducing three learning biases to the learning model – the phonetic naturalness bias, the allophony bias and the suprasegmental bias – the model's predictions of speakers’ behaviour improved. This suggests that these biases are part of speakers’ phonotactic knowledge.
The phonotactic grammar that we added biases to was based on 40 handwritten phonotactic constraints. Although we tried to be as inclusive as possible in our construction of the constraints, it is still possible that the mismatch between the grammar prediction and speakers’ well-formedness judgements is due to violations of constraints not included in the handwritten constraints. To safeguard against this possibility, we compared the handwritten grammar with a machine-learned grammar with 40 constraints determined by the phonotactic learner in their evaluation of the 200 test stimuli. The predicted MaxEnt values of the latter grammar mostly resembled those of the handwritten grammar: it also predicted that the allophonic gaps were less well-formed than accidental gaps, which was inconsistent with the speakers’ judgements, as shown in Fig. 4.Footnote 10

Figure 4 MaxEnt values of penalty scores predicted by a machine-learned 40-constraint grammar grouped by stimulus type. Each dot represents the MaxEnt value of one test stimulus.
Figure 4 also shows that the predicted well-formedness of tonal gaps is very close to that of real words, meaning that the machine-learned grammar did not penalise tonal gaps as much as the handwritten grammar. However, when we allowed the phonotactic learner to learn more constraints (100 constraints), the well-formedness predictions between tonal gaps and real words widened (Fig. 5). On the one hand, this indicates that the handwritten grammar, with a clear tonal gap constraint in place, may have overestimated its effect, casting doubt on the necessity of the suprasegmental bias. On the other hand, it also shows that, although tonal gap constraints may not be as effective as other phonotactic constraints, they can nonetheless emerge from the lexicon.

Figure 5 MaxEnt values of penalty scores predicted by a machine-learned 100-constraint grammar grouped by stimulus type. Each dot represents the MaxEnt value of one test stimulus.
Relatedly, our judgement experiment only selected a subset of all theoretically possible Mandarin syllables as test stimuli. To safeguard against the possibility that our results are due to the specific stimuli included in our study, we calculated the MaxEnt scores for the entire set of possible syllables, based on both the original handwritten grammar and the biased grammar. The distributions of the scores of all possible syllables on each stimulus type were very similar to the patterns found for the 200 test stimuli illustrated by Figs 2 and 3. For example, the original grammar predicted that the well-formedness of allophonic gaps would be lower than accidental gaps, and would be reversed in the biased grammar. This pattern also held true when we extended the predictions to all possible syllables, as in Fig. 6.Footnote 11

Figure 6 MaxEnt values of penalty scores of all theoretically possible syllables predicted by the original handwritten grammar and the biased handwritten grammar, grouped by stimulus type. Each dot represents the MaxEnt value of one syllable.
Furthermore, we conducted a cross-validation procedure which took ten random 100-stimuli subsets of the well-formedness rating data to see whether they still correlated with the model-predicted well-formedness and whether the biases still increased the correlation between the two. For the ten random subsets, we performed correlation tests between speakers’ judgements and the MaxEnt values of penalty scores predicted by (a) the original handwritten grammar and (b) the biased grammar. The correlation coefficients for the handwritten grammar varied from 0.708 to 0.753 (mean = 0.727), and the coefficient range for the biased grammar was from 0.728 to 0.790 (mean = 0.757). The correlation coefficient gains of these ten random subsets before and after the biases ranged from 0.011 to 0.049 (mean = 0.030). From these results we can infer that the well-formedness rating data we collected are homogeneous and reliable, as random subsets displayed similar correlation patterns and benefited from the biases.
Hayes & White's (Reference Hayes and White2013) post hoc modelling suggested that degrading the weights of unnatural constraints would improve the overall model prediction on non-word judgement. Our study adopted a different approach, adjusting the weights of systematic (phonetically natural) constraints instead of accidental (not phonetically motivated) constraints. Contrary to Hayes & White's findings, the best-fit factor for systematic constraints was 0.6, which suggests that the phonetically natural constraints were also downgraded. If the naturalness bias did exist, we would expect these systematic constraints to be upgraded, since they should be more easily exploited by speakers and play a significant role in phonotactic judgement. A closer examination of the correlation results revealed that the variation of the correlation coefficients induced by the manipulation of the weights of systematic constraints was minimal. For example, changing the factor of systematic constraints from 1 to 0.6 only increased the correlation by around 0.002. This is partly due to the MaxEnt transformation, which reduces the variation size of extreme values (gaps that violate systematic gaps are often associated with high penalty scores). Another factor that leads to the excessive penalisation of systematic gaps by the unbiased grammar is multiple constraint violation. Hayes & White (Reference Hayes and White2013) created their stimuli in such a way that each non-word only violated one natural or unnatural constraint. However, ten of the 40 systematic gaps in our list violate more than one systematic constraint. These gaps will receive exceptionally high penalty scores, due to the ‘ganging up’ effect of the constraints they violate. But speakers’ non-word judgement may not work in this cumulative fashion. In fact, if we remove all stimuli with multiple constraint violations and apply the same routine for determining the best-fit factors, the biasing factor for systematic constraints changes to 2.5. The direction for the naturalness bias is then consistent with previous findings: the effects of phonetically natural constraints were underlearned by the phonotactic learner, and the weights of the systematic constraints should be upgraded to better match the experiment results.
Indeed, how naturalness biases phonological learning remains debatable. Some studies have reported that phonetically natural patterns are more likely to be applied to wug forms (Becker et al. Reference Becker, Ketrez and Nevins2011) and hence to have a stronger effect in non-word judgements (Hayes & White Reference Hayes and White2013). Results from artificial grammar learning experiments are inconclusive. Some studies suggest that phonetically natural rules are not necessarily easier to learn compared to unnatural patterns when the complexity of the patterns is controlled (Pycha et al. Reference Pycha, Nowak, Shin and Shosted2003, Moreton & Pater Reference Moreton and Pater2012b). However, more recent artificial grammar learning experiments report that natural patterns do have some advantage (Finley Reference Finley2012, Myers & Padgett Reference Myers and Padgett2014, Martin & Peperkamp Reference Martin and Peperkamp2020). Given that the direction of the naturalness bias here depends on whether we include stimulus items with multiple constraint violations, we acknowledge the complexity of the issue, and hope that future research will provide more clarity on the nature of this learning bias.
The best-fit factor for the allophonic gaps was 0.3, suggesting that the effects of the allophonic constraints in the handwritten grammar were substantially overestimated by the phonotactic learner. The unbiased grammar predicted that allophonic gaps were less well-formed than segmental accidental gaps, but speakers’ acceptability rating data disagreed. This discrepancy between the model's prediction and the behavioural results demonstrates that there is a bias against allophonic violations in phonotactic judgements. The phonotactic learner considered only the co-occurrence patterns among the segments in the lexicon, and thus could not distinguish allophonic gaps from phonemic gaps. However, it is likely that the allophonic relations are part of Mandarin speakers’ phonological knowledge, so that the perceptual distance between attested words and allophonic gaps is closer than that between attested words and phonemic gaps (cf. Hayes & White's Reference Hayes and White2015 saltation bias, and other naturalness biases in perceived phonological distance). As a result, allophonic gaps are not penalised as much as segmental gaps in non-word judgements.
As recognised earlier, another potential source for this bias is perceptual difficulty. It is possible that, when hearing allophonic gaps in the judgement task (e.g. [wen]), speakers failed to accurately perceive the form, and corrected it to its corresponding allophonically appropriate real word [wən]. If so, speakers’ rating for the allophonic gap [wen] actually reflects the well-formedness of the real word [wən]. This possibility, however, is less likely, due to the AX discrimination pre-test results, which showed that Mandarin speakers were by and large able to accurately perceive the differences between allophonic gaps and their allophonically appropriate counterparts.
How can a learner tell allophonic gaps from other segmental gaps in the lexicon? Notice that the forms ruled out by allophonic constraints are in complementary distribution. Peperkamp et al. (Reference Peperkamp, Le Calvez, Nadal and Dupoux2006) developed a statistical algorithm to determine allophonic relations among the sounds in a lexicon. The algorithm evaluates the context similarity of two sounds. If two sounds occur in many similar contexts, they are less likely to be allophones. The simulation results suggested that the algorithm was able to detect real allophonic distributions in French. In our case, for example, for the sounds [a] and [ɑ], speakers identified that they occur in non-overlapping environments, and established the allophonic relation between them. Constraints which regulate the distribution of [a] and [ɑ] are subsequently marked as allophonic constraints, and the forms that violate any of these constraints are categorised as allophonic gaps.
The effect of the tonal constraint was also downgraded in the biased grammar, though the evidence for this downgrading effect is somewhat weaker. This is interpreted as a bias against phonotactic constraints that refer to both segmental and suprasegmental levels. One possible source of this bias is complexity. Since these restrictions are cross-tier in nature, they are formally more complex than segmental constraints (Moreton Reference Moreton2008, Moreton & Pater Reference Moreton and Pater2012a). If a phonotactic constraint is harder to learn during the inductive learning of the lexicon due to its high complexity, the acceptability of the forms that violate this constraint will be higher than expected. This bias can also be attributed to perception. Since psycholinguistic evidence has suggested that tones are perceived as conceptually different from segment-level features and have a perceptual disadvantage (Cutler & Chen Reference Cutler and Chen1997, Sereno & Lee Reference Sereno and Lee2015, Wiener & Turnbull Reference Wiener and Turnbull2016), the distance between real words and tonal gaps in speakers’ perceptual grammar is not as large as that between real words and segmental gaps. Under either scenario, an unbiased learning model is expected to overestimate the ill-formedness of tonal gaps.
9 Conclusion
This study has investigated the nature of the phonotactic knowledge in Mandarin Chinese. Our experimental results showed that native speakers’ phonotactic judgements were influenced not only by lexical statistics, but also by multiple grammatical principles, such as the OCP, allophonic restrictions and segmental–suprasegmental co-occurrence constraints. We then modelled such phonotactic knowledge by using a handwritten phonotactic grammar whose constraint weights were assigned by inductive learning of the lexicon, based on the maximum entropy principle (Hayes & Wilson Reference Hayes and Wilson2008). An unbiased grammar made good predictions with respect to the speakers’ well-formedness judgements, but incorporating learning biases by adjusting the weights of three types of constraints in the grammar – systematic, allophonic and suprasegmental – further improved the predictions of the model. This indicates that the speakers’ phonotactic knowledge is not merely a reflection of the statistical properties of the lexicon, even if the statistical properties are based on grammatical constraints (Shademan Reference Shademan2007, Coetzee Reference Coetzee2008, Kager & Pater Reference Kager and Pater2012, de Lacy & Kingston Reference de Lacy and Kingston2013, Hayes & White Reference Hayes and White2013); a complete model of phonotactics needs to consider other extralexical factors, such as the specific natures of the grammatical principles, and allow the extralexical factors to guide the learning of these principles.