1. Introduction
To what extent are there collocational preferences in the verb–object combinations of a large corpus of Norwegian, how important are recurrent combinations in usage, and what can the collocational preferences of individual verbs tell us about verbal complementation in general? The aim of the present article is to shed some light on these questions, focusing on dependency-based verb–object combinations in the NoWaC corpus of web-documents in Norwegian Bokmål (Guevara Reference Guevara, Kilgarriff and Lin2010). The corpus contains approximately 700 million tokens, and the availability of corpora of this size allows for new empirical studies of word co-occurrences in Norwegian.
Verbal complementation has typically been treated either from the traditional lexical perspective under labels such as argument structure (Grimshaw Reference Grimshaw1990, Bresnan Reference Bresnan2001, Levin & Rappaport Hovav Reference Levin and Rappaport Hovav2005), subcategorisation and selection (e.g. Chomsky Reference Chomsky1965), and valency (e.g. Ágel Reference Ágel2000) or, more recently, also from a constructional perspective in terms of argument structure constructions (e.g. Goldberg Reference Goldberg1995, Reference Goldberg2006; Perek Reference Perek2015). Typically, these approaches consider complementation at a rather abstract level, although the constructional approach tends to be more interested in the whole spectrum of abstractness, from specific exemplars to highly abstract generalisations. In the present investigation the aim is to complement such studies by focussing on the more concrete level of collocational preferences: We focus here on complementation in terms of specific verb–object combinations and on the collocational preferences found in such combinations. This focus is compatible both with a lexical and a constructional model of complementation; the focus is on verbs and at the same time on verbs in the transitive construction.
A crucial point is that the difference between specific word combinations in the verb–object relation on the one hand and valency on the other, is a difference in the level of abstractness at which co-occurrences are handled, and this is true regardless of whether one adheres to a lexical or to a constructional model of complementation. As argued by Herbst (Reference Herbst, Schmid and Handl2010:226), ‘[c]ollocation and valency represent different facets of the same phenomenon’.
In clauses (or constructions) containing verbs and direct objects in the form of NPs, speakers are always exposed to combinations of specific verbs and nouns, and exposure to such combinations are necessarily the basis for speakers representations of complementation of this kind. It follows from this that in order to account for complementation, we need to model these exposures. Since verb–NP–object combinations are always realised by concrete verbs and nouns, the association strength between them in a large corpus can be measured, as is normally done in studies of collocations.
A useful distinction that has emerged in corpus linguistics (Sinclair Reference Sinclair1991) is the one between the open-choice principle and the idiom principle. Whereas a notion like argument structure is clearly based on the open-choice principle, speakers seem in many cases to have clear intuitional preferences even between fully grammatical, semantically transparent and equally understandable alternatives. Recently, Goldberg (Reference Goldberg2019) discusses an example like Explain me this in English, which is felt by native speakers to be much less conventional than the alternative Explain this to me. Such preferences call for rather specific representations and are best accounted for in terms of what Sinclair (Reference Sinclair1991) calls the idiom principle, which does not imply that preferred alternatives are semantically non-transparent. Rather, the idiom principle implies that speakers have access to rather specific representations in their production and comprehension of language, and that multiword units can constitute single choices. The idiom principle is highly compatible with exemplar categorisation and representation, and these notions are further disussed in Section 2, where it is argued that they can be fruitfully applied to account also for collocational preferences in verb–object combinations.
In Section 3, the computational work that was carried out in order to build a database of corpus-based verb–object combinations is presented. As already mentioned, the combinations were extracted from the NoWaC corpus of web-documents (Guevara Reference Guevara, Kilgarriff and Lin2010), and the corpus has been lemmatised, annotated with part-of-speech tags (i.e. POS-tagged) and parsed. Based on computations in R, the overall importance of recurring verb–object combinations and collocational preferences in such combinations are discussed in Section 4, and the database is also the foundation for studies of the collocational preferences of some individual verbs and verb pairs, presented in Section 5. It is suggested that collocation measures allow us to model the mutual strength of the verb–object exemplars in the complementation clusters associated with individual verbs. Finally, in Section 6, a brief discussion is followed by some concluding remarks.
2. Open-choice vs. idiom principle and exemplar representations
The distinction between the open-choice principle and the idiom principle is due to Sinclair (Reference Sinclair1991, Reference Sinclair2004) and his work based on large electronic corpora. We will now take a closer look at these notions and relate them to verb–object combinations viewed in terms of abstract argument structure and to such combinations viewed in terms of concrete combinations of verb and noun.
The notion of argument structure is based on the open-choice principle. The notion has been adopted from mathematical logic, where arguments are the variables in formulae such as P(x,y), where the predicate P takes two arguments, x and y. In principle, the argument variables can be filled by any entity, and a grammatical model of this kind is based on open choice in the filling of argument slots: ‘Any tree structure shows it clearly: the nodes on the tree are the choice points. Virtually all grammars are constructed on the open-choice principle’ (Sinclair Reference Sinclair1991:109–110). Certainly, this is not as true today as it was 30 years ago, especially with the rise of corpus linguistics and usage-based models (Langacker Reference Langacker1987, Reference Langacker2000; Barlow & Kemmer Reference Barlow and Kemmer2000), where representations of the language system are seen as directly influenced by usage, and with the rise of exemplar-based models of linguistic categorisation (Bybee Reference Bybee2010, Reference Bybee, Hoffmann and Trousdale2013), it is more broadly acknowledged that the idiom principle plays a very important role in language.
Collocations represent par excellence examples of the idiom principle at work: ‘On some occasions, words appear to be chosen in pairs or groups and these are not necessarily adjacent’ (Sinclair Reference Sinclair1991:115). Empirical approaches to complementation suggest that speakers have access to various types of complementation knowledge. First of all, they know in which syntactic patterns predicators conventionally occur. Secondly, they know that there may be clear preferences also for the exact lexical fillers of the complement slots that are available in a pattern (Herbst Reference Herbst, Herbst and Götz-Votteler2007, Haugen Reference Haugen2013, Goldberg Reference Goldberg2019). This is the crucial point that a database of verb–object combinations allows us to investigate further.
The classical approach to restricting open choice within syntactic categories, found both in early versions of generative grammar (e.g. Chomsky Reference Chomsky1965) and in german valency grammars (e.g. Helbig Reference Helbig1992) is to posit selectional restrictions, which are typically coarse-grained semantic features, like ± ANIMATE, ± HUMAN, ± ABSTRACT, etc. In some cases, such general semantic features are useful. Consider for example the strongest collocates of the verb spise ‘eat’ in Table 1. We will return to the details of this table in Section 4. For now, the important point is that the object nouns are ranked according to the strength of their collocational association with the verb (log-likelihood (Dunning Reference Dunning1993), given in the rightmost column in Table 1 and other tables): the greater the number in the rightmost column, the stronger the collocational association. The objects of spise can be subsumed under more general semantic features like FOOD and MEAL.
Table 1. Strongest object collocates of spise ‘eat’.
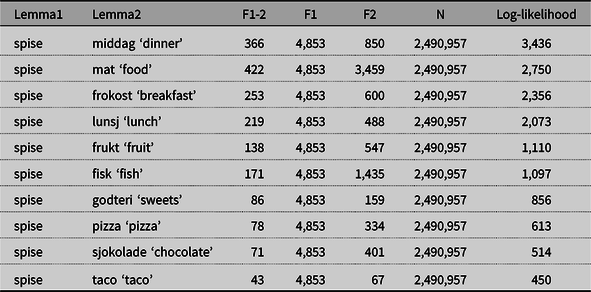
F1-2 = frequency of verb + noun, F1 = frequency of verb, F2 = frequency of noun, N = verb–object tokens
From work on valency dictionaries, however, it is well known that for most verbs it is notoriously difficult to capture specific restrictions on complements through positing general semantic categories. Herbst (Reference Herbst, Herbst and Götz-Votteler2007) discusses the treatment of semantic valency in two large valency dictionaries – for English (Herbst et al. Reference Herbst, Heath, Roe and Götz2004) and for German (Schumacher et al. Reference Schumacher, Kubczak, Schmidt and de Ruiter2004) – and states that:
What is interesting about the lexicographical treatment of the non-formal side of the characterization of complements in VDE [Valency Dictionary of English] or VALBU [Valenzwörterbuch deutscher Verben] is that both dictionaries make use of general categories such as someone or derjenige (which can be seen as equivalent to Helbig’s [Reference Helbig1992] semantic feature + HUM) but nevertheless find it necessary to give relatively specific lists of lexical items such as door, window etc. or Kommision, Bürgerinitiative. Very often this is because no suitable label can be found as in the case of the note for the verb set in VDE
A person I can set someone III something such as a deadline, a target, a task, a test, an examination, etc II . (Herbst Reference Herbst, Herbst and Götz-Votteler2007:26; bold for emphasis in the original)
A plausible way of accounting for this kind of specific preferences in complementation (the Roman numerals in the quote above refer to the different complements of the verb) is to allow for exemplar representations. As Bybee (Reference Bybee, Hoffmann and Trousdale2013:52) points out, exemplar models are models where it is proposed that the memory of experiences with language is similar to the memory of experiences in general, which means that each experienced token has an impact on the overall mental representation of the phenomenon in question. In constructional terms, the difference between an exemplar-based model of complementation and abstract selectional features can be described as follows:
A schematic slot in a construction might consist of a list of all the items that have occured in that slot (as predicted by an exemplar model), or it might be considered a set of abstract semantic features that constrains the slot, as usually proposed. It could, of course, be both. However, the importance of the specific exemplars that have occurred in the construction can be seen … in cases where a single abstract feature does not characterize a class or explain its extension. (Bybee Reference Bybee, Hoffmann and Trousdale2013:57)
When we study the specific lexical fillers of the object slot of the verb and the strength of their collocational association, we study the complementation of the verb in terms of an exemplar cluster. The main idea behind such a model is that:
[R]ather than making reference to a general semantic feature when using a construction, the speaker may very well reference a particular lexical item that has already been used in the construction and stored in memory. (Bybee Reference Bybee, Hoffmann and Trousdale2013:58)
As we see in Table 1, a verb in our verb–object combinations is associated with a list of exemplars which is ordered according to the strength of association between verb and object (measured as log-likelihood). Arguably, this approach yields not only a precise description of collocational preferences in such combinations; it can also account for the probabilistic nature of complementation choices and the different strengths of association between a verb and its habitual complements. Based on studies of individual verbs and verb pairs, I will argue that the corpus-based strength of collocational association in many cases coincide with clear intuitional preferences between equally grammatical and equally understandable alternatives.
The open-choice principle and exemplar-based models can be said to represent the extreme ends on a continuum of schematicity from the most schematic to the most specific, respectively. In between there are various possibilities for intermediate degrees of specificity, which are readily accounted for in constructional approaches to complementation. As Goldberg (Reference Goldberg2006:46) points out, an exemplar-based model does not remove the need for abstraction; not all information available from instantiations is stored, and ‘exemplar models fail to explain how exactly items cohere as a category’ (Goldberg Reference Goldberg2006:47). There are certainly cases where more schematic generalisations can be done, and where more schematic semantic categories seem plausible, see the discussion of the verb spise ‘eat’ in Table 1 above. The point that will be made here, however, is that specific collocational preferences are also part of the picture.
The study of collocational preferences in verb–object combinations also allows us to explore more fully idiomatic combinations in the sense of combinations lacking semantic transparency. Consider for example the Norwegian verb brenne ‘burn’. Fully transparent combinations with this verb are examples like the following:
-
(1)
There are, however, also strongly associated combinations where verb + noun yield more idiomatic meanings. One example is the object bro ‘bridge’, which occurs in the idiomatic expression brenne alle broer ‘lit.: burn all bridges, break all contact’ as in (2a) below. Other strong object collocates of brenne are the nouns straffe ‘penalty’, sjanse ‘chance’ and straffespark ‘penalty kick’, see the examples in (2b, c).
-
(2)
The use of this verb as in (2b, c) seems to be restricted to ‘missing possibilities for scoring a point in ball games’. Expressing other types of failure is not conventional; one cannot say, for example, *Han brente 2,40 ‘He burned 2.40’ about an athlete in high jump. Such idiomatic and semi-idiomatic uses of a verb are also a part of speakers’ knowledge about these verbs, and a clear advantage of an exemplar-based model is that it can account equally well for transparent as well as non-transparent, contextually more skewed combinations; in exemplar models, contextual information is hypothesised to be part of the representations kept in memory (Bybee Reference Bybee2010, Goldberg Reference Goldberg2019).
The database of verb–object combinations is presented in the following section, where we first take a look at collocations in general.
3. Extraction of verb–object combinations and collocational preferences
3.1 On collocations, methodology and tools
‘Collocations of a given word are statements of the habitual or customary places of that word’ (Firth Reference Firth1957:181) is often cited as the founding definition of the notion of collocation.
To be extreme, any two linguistic entities that co-occur within the boundaries of a linguistically significant domain (e.g. a phrase, sentence, paragraph, text or discourse) might be treated as a potential collocation.
In computational approaches to collocations, the key notions are frequency of occurrence and association. Given any two linguistic entities that co-occur within the boundaries of a linguistically significant domain, those that occur with a higher than chance frequency are said to be lexically associated or attracted. Therefore, if one can reliably measure and quantify the extent of lexical association between words, it becomes possible to sort the candidate pairs by the strength of their mutual attraction. A number of statistical methods to measure lexical association have been devised and perfected over the years; a full overview of such methods is well beyond the aims of the present article, but the reader is referred to Evert (Reference Evert2005, Reference Evert, Luüdeling and Kytö2008) for in-depth treatment.
In order to be reliable, the measurements of association in word combinations must be based on representative and solid frequency counts, such as those obtained from large electronic corpora. The NoWaC corpus of Norwegian Bokmål (Guevara Reference Guevara, Kilgarriff and Lin2010) contains approximately 700 million tokens from web-documents. The representativity of a corpus is always a difficult question that needs to be discussed, and of course it would be interesting to do comparisons across different corpora in the future. Guevara (Reference Guevara, Kilgarriff and Lin2010) estimates NoWaC to contain between 3–15% of the indexed online Bokmål at the time of extraction, and there is reason to believe that the corpus, containing texts from the general *.no domain, is a reasonably representative web-corpus. In its current version, the corpus (v. 1.1) is annotated with POS tags from the Oslo–Bergen tagger (latest releaseversion, Johannessen et al. Reference Johannessen, Hagen, Lynum, Nøklestad and Andersen2012).
The classical approach to the extraction of collocations is what Evert (Reference Evert2005) calls distance-based co-occurrences, where collocation candidates are typically extracted by exploiting a combination of POS-tags and a contextual window of up to four or five words (as suggested by Sinclair Reference Sinclair1991). In recent years, however, relation-based co-occurrences have become a more common basis for extraction (Uhrig, Evert & Proisl Reference Uhrig, Evert, Proisl, Cantos-Gómez and Almela-Sánchez2018). In such approaches, the co-occurring words are typically in a syntactic relation, which in our case will be a verb–object dependency relation, which is the type of syntactic relation used in the available treebank, see below.
Relational co-occurrences share important features with collostructional analysis, more specifically with covarying collexeme analysis (Stefanowitsch & Gries Reference Stefanowitsch and Gries2005). The main difference is that whereas the relational co-occurrence analysis computes the associations between words in a syntactic (in our case dependency) relation, the covarying collexeme analysis computes the associations between words in a more abstract and independently established construction. As discussed in the introduction, however, the collocations as studied in the present investigation are also collocations within the transitive construction.
Since the first Norwegian dependency treebank, NDT v. 1.0 (containing about 300,000 tokens, about 20,000 sentences), was made available (see Solberg Reference Solberg, Oepen, Hagen and Johannessen2013), it was possible to train parsing models for Norwegian with the Maltparser software (Nivre, Hall & Nilsson Reference Nivre, Hall, Nilsson, Calzolari, Choukri, Gangemi, Maegaard, Mariani, Odijk and Tapias2006). The parsing model that was used in the final experiment was trained on 75% of the sentences in the treebank. Estimation of the parser’s parameters was carried out on 10% of the sentences, while the system’s performance was validated on the remaining 15%. The sentences in the treebank had been randomly shuffled prior to the experiments. The best resulting models used the ‘stacklazy’ algorithm from Maltparser, and obtained a labeled attachment score (LAS) of 0.864, a value that is very much in line with the state of the art. LAS is a standard evaluation metric in dependency parsing, and the score shows the percentage of words that are assigned the correct syntactic head as well as the correct dependency label. The score indicates the high quality of the data in the NDT treebank. The parsed version of NoWaC was computed on the cluster facilities owned by the University of Oslo in just over 10 hours and using an allowance of 24Gb RAM. The corpus contains approximately 33.5 million sentences (dependency trees).
3.2 Extracting combinations, computing lexical associations, and cleaning of the data
With the annotated, lemmatised and parsed corpus in place, for each finite verb (i.e. each sentence head) in each main sentence in the corpus the object dependents bearing the POS tag ‘common noun’ were extracted. Altogether approximately 2.5 million verb–direct object combination tokens (0.53 million types) were obtained. The candidate lists were then processed with the UCS toolkit v. 0.6 in order to compute the measure of association for each combination (more on this below). In addition to the dependency relation observed for each candidate pair, the information used for the computation comprises the conjoined frequency of the pair and the independent frequencies of each lemma, both within the verb–object dependency relation (i.e. the basis for all calculations is the 2.5 million extracted verb–object tokens).
Given the Zipfian distribution of words in a linguistic corpus, most candidate pairs in the data had very low frequencies and would be useless for any statistical measure of significance. A frequency filter was thus introduced in order target the combinations where the strongest associations between verb and object are most likely to be found: Only the verb–object pairs that co-occur at least 10 times in the corpus were kept. This filter yielded a final figure of 26,425 verb–object combinations (types) out of 1.6 million tokens, which means that as much as 64% of the 2.5 million tokens originally obtained are still represented in the material, despite the 10-occurrences threshold. We will return to this interesting finding in Section 4 below.
For measurement of the statistical associations between the collocations, log-likelihood values (Dunning Reference Dunning1993) were computed. Uhrig et al. (Reference Uhrig, Evert, Proisl, Cantos-Gómez and Almela-Sánchez2018) evaluate different association measures for collocation extraction across a range of syntactic relations, using the Oxford Collocations Dictionary for Learners of English, 2nd edition (OCD2 2009) as the gold standard of comparison. Log-likelihood comes out on top overall, and as the best performing measure when it comes to the verb–object relation, see Uhrig et al. (Reference Uhrig, Evert, Proisl, Cantos-Gómez and Almela-Sánchez2018:125).
As far as I know, there are no previous studies of this kind for Norwegian, and unfortunately, no collocations dictionary is available for Norwegian, which means that there is no standard against which the extracted verb–object combinations could be evaluated automatically. Therefore, a manual cleaning of the 26,425 verb–object combinations has been carrried out, to evaluate and secure the quality of the data. The cleaning resulted in a revised dataset, containing 20,964 verb–object combinations. In addition, the studies of individual verbs and verb pairs to be presented in Section 5 below, in themselves represent some in depth evaluations of the data.
The cleaning of the data was carried out in two steps. First, the 360 combinations lemmatised as the verb le ‘laugh’ are actually occurrences with the imperative form of the verb lese ‘read’; hence, the verbs of these combinations were changed to lese ‘read’. One of these combinations, originally le sak, now lemmatised as lese sak ‘read case’ is an extreme outlier, probably because of the high frequency of this combinations in media texts and in hyperlinks. In the original data, this combination has a log-likelihood value of 692,126, compared to a log-likelihood value of 168,278 for the second highest combination. In the computations to follow, this extreme value has been neutralised, and the combination lese sak ‘read case’ has been given a log-likelihood value of 6,000, in line with the second strongest combination with lese, lese anmeldelse ‘read review’ (log-likelihood 5,921). Another outlier is the combination skrive venn ‘write friend’ (log-likelihood 168,278), which seems to be due to the fact that skrive ‘write’ in the phrase skriv ut ‘print’ frequently occurs adjacent to the frequent phrase tips en venn ‘tell a friend’. Since venn ‘friend’ is intuitively not a possible NP object of skrive ‘write’ at all, this combination was deleted.
The extreme log-likelihood values of the two outliers above also means that other combinations with sak ‘case’ and venn ‘friend’ as objects get artificially low log-likelihood scores. Negative log-likelihood values for combinations with these objects have therefore been set to 0. The first computations on the material were carried out after the preliminary cleaning described above.
In a second step, a more thorough cleaning was carried out, where the number of verb–object types was reduced from 26,424 to 20,964 combinations. The cleaned data set thereby contains 20,964 verb–object combinations where the objects are all intuitively possible NP objects of the 955 verbs. The cleaning resulted in a reduction of the number of verbs from 1,183 to 955, and was carried out as follows:
-
185 verbs that intuitively do not take NP objects were deleted (see Table A1 in the appendix; examples of combinations with such verbs are: falle oljepris ‘fall oil price’, flykte hals ‘flee neck’, kjefte kort ‘yell card’).
-
An additional 45 verbs were deleted (see Table A1 in the appendix), where an NP object is intuitively possible, but where the collocation candidates extracted are seemingly not complements of this kind (examples of combinations with these verbs are beslutte styre ‘decide board’, mette fett ‘fill fat’, sminke hjelp ‘make up help’).
-
All non-noun object candidate extractions were deleted.
-
Additional combinations where the extracted object candidate is not an intuitively possible NP object of the respective verb, were deleted.
4. Some frequencies and overall collocational preferences in the corpus
The large data set on which the present investigation is based, allows us to say something about the importance of recurring verb–object combinations and how collocational strength is distributed among the combinations. As already mentioned in Section 3, the point of departure of the investigation was ca 2.5 million verb–object tokens, out of which there were ca 0.53 million types. As the focus was the strongest collocation candidates among these combinations, a threshold of occurring at least 10 times in the corpus was set. This filtering reduced the number of types to 26,425, i.e. to ca 5% of the original 0.53 million types. Interestingly, however, the 26,425 types passing the threshold of at least 10 occurrences, account for as many as 1.6 million of the verb–object tokens, i.e. 64% of the tokens, see Figure 1. In other words, the 5% verb–object types where the strongest collocational associations are expected to be found account for as much as 64% of the tokens, which means that recurring verb–object combinations strongly dominate usage as represented in the corpus. Hence, collocational preferences seem, overall, to play a very important role in verb–object combinations.

Figure 1. Verb–object-combinations: types and tokens (based on the numbers in the filtered, raw material).
Since the original 0.53 million types have not been manually cleaned, we do not have exact relative figures for the cleaned data. However, if we take the reduction in tokens (by 215,794 tokens) from the filtered, raw material to the both filtered and cleaned material as a point of departure for the calculation, the tokens in the cleaned material (1,388,921) account for 61% of the total number of tokens, which is very much in line with the calculation above.
Let us now take a closer look at the overall frequencies of the verb–object combinations occurring at least 10 times in the corpus. The computations presented below have been carried out on the cleaned data set, using the R environment for statistical computing. When we ignore the extreme outlier lese sak ‘read case’ which has a frequency of 113,548, see 3.2 above, the frequencies of the combinations are distributed as shown in Table 2. We see that ¾ of the verb–object combinations have a frequency between 10–37, and that the variation is great in the 4th quartile, with the maximum frequency as high as 14,144. One thousand eight hundred and seventy-eight (1,873) combinations (8.9%) have a frequency of 100 or higher, 308 (1.5%) a frequency of 500 or higher, and 139 (0.7%) a frequency of 1,000 or higher.
Table 2. Statistical summary of verb–object frequencies.

More interesting for the measurement of collocational strength, however, is the log-likelihood values of the verb–object combinations. These values are distributed as shown in Table 3. The maximum value belongs to the combination forbeholde rett ‘reserve right’, where the verb forbeholde occurs almost exclusively with this object. There is a large gap from this combination to the second strongest, legge vekt ‘put weight’, which has a log-likelihood of 57,034.
Table 3. Statistical summary of the log-likelihood values of the verb–object combinations.

An overview of the 20 strongest combinations in terms of log-likelihood is given in Table 4. The high association strength of some of these combinations, such as se bilde ‘see picture’, se video ‘see video’, sende appell ‘send appeal’, skrive kommentar ‘write comment’, and skrive mening ‘write meaning’ might be due to the nature of the corpus, whereas other combinations seem to be more neutral in terms of text type. Combinations like forbeholde rett ‘reserve right’, legge vekt ‘put weight’, ta kontakt ‘contact’, stille krav ‘demand’, ta tid ‘take time’, sette pris ‘appreciate’, legge merke ‘notice’, finne sted ‘take place’, trenge hjelp ‘need help’, gi beskjed ‘tell’ and ta utgangspunkt ‘take as starting point’ are intuitively very strongly associated combinations independently of context and text type. As mentioned in Section 3.1, it would certainly be interesting to do the study also based on other corpora in the future.
Table 4. Verb–object combinations with highest log-likelihood values.
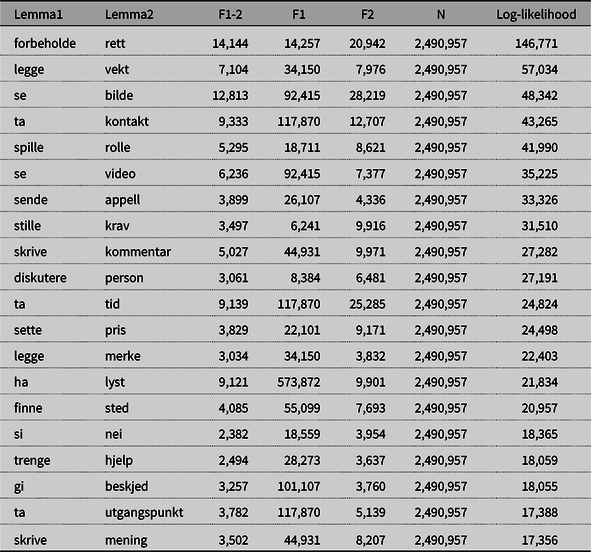
F1-2 = frequency of verb + noun, F1 = frequency of verb, F2 = frequency of noun, N = verb–object tokens
In addition to the overall collocational strengths of the combinations, it is interesting to take a look at how the collocational strength is distributed among the verbs. There is no established measurement for the overall collocational strength of the verbs in a corpus, and a range of different computations are of course possible. Below, the overall collocational strength of the verbs is measured in terms of the mean log-likelihood value of their verb–object combinations. If we exclude two verbs with extreme outliers, forbeholde ‘reserve’ (mean log-likelihood 146,771, see also above) and sensurere ‘censor’ (mean log-likelihood 7,931),Footnote 1 we end up with the statistics in Table 5.
Table 5. Statistical summary of the mean log-likelihood values of the verbs.

The mean log-likelihood values are distributed among the verbs as shown in Figure 2. An interval of 50 has been used up to 400, thereafter an interval of 100 up to 1,000, and finally 1,000–4,000. We see that a mean value from 50 up to 300 is the most common, but also that the variation in mean collocational strength is relatively large, from a minimum of four up to almost 4,000 (excluding the two outliers discussed above).

Figure 2. Mean log-likelihood values, distributed over verbs.
A list of the verbs with the highest mean log-likelihood values is given in Table 6. When we throw a glance at the number of NP object types connected to these verbs, it is tempting to hypothesise a correlation between a high log-likelihood mean and a low number of objects. A Pearsons r calcuation of number of objects and log-likelihood mean for the material as a whole, however, comes out with as weak a correlation as –0.0067, which basically means an absence of correlation between the two variables. Hence, a verb occurring with few objects in the material is not more likely to have a high mean log-likelihood value than a verb occurring with a large range of objects.
Table 6. Verbs with the highest mean log-likelihood values in their verb–object combinations.

4.1 Preliminary discussion
What do the results presented above tell us about verb–object combinations, primarily, and about the complementation of verbs in general? First, recurrent verb–object combinations, i.e. combinations passing the 10-occurrences threshold dominate usage; as noted above, the 5% of the types passing this threshold account for as many as 64% of the verb–object tokens. Secondly, the overall collocational strengths of the verbs, measured as the mean log-likelihood value of their verb–object combinations, is unequally distributed among the verbs. Hence, overall collocational strength is subject to great lexical variation.
Large-scale empirical studies of valency, both for verbs (Helbig & Schenkel Reference Helbig and Schenkel1973, Boas Reference Boas2003, Herbst et al. Reference Herbst, Heath, Roe and Götz2004, Schumacher et al. Reference Schumacher, Kubczak, Schmidt and de Ruiter2004, Faulhaber Reference Faulhaber2011) and for polyvalent adjectives (Herbst Reference Herbst1983, Sommerfeldt & Schreiber Reference Sommerfeldt and Schreiber1983, Daugaard Reference Daugaard2002, Haugen Reference Haugen2015), show that it is common for these valency carriers to occur in several different valency patterns. At the same time, these large-scale corpus investigations also suggest that the range of patterns in which individual valency carriers occur, is highly restricted and to a large extent idiosyncratic, also at the specificity level of grammatical categories such as NPs, PPs, clauses and infinitive constructions, which is normally the main focus in valency studies. Here, it has been demonstrated not only (i) that recurrent conventional combinations seem to dominate usage also at the level of concrete verb–object combinations but also (ii) that there is great variation in the overall collocational strength of the verb–object combinations of individual verbs. Valency and collocational preferences of the kind studied here indeed provide problems par excellance for any strict division between lexicon and grammar, as complementation is (i) found to a large extent to be lexically determined and (ii) to a large extent to be based on recurring concrete combinations.
In the following section some more detailled studies of individual verbs and closely related verb pairs will be presented.
5. Some individual verbs and verb pairs
We will now zoom in on some individual verbs and verb pairs. First, we will discuss the relationship between the open-choice principle and the idiom principle (Section 5.1), and then we will take a closer look at how collocational preferences can distinguish between semantically similar verbs (Section 5.2). As mentioned above, the more detailled studies presented in this section also provide some deeper evaluations of the extracted material. The verbs in the database are presented in tables with their NP objects sorted in descending order based on log-likelihood value, and the numbers and calculations are based on all the verb–object examplars extracted from the corpus. Hence, the tables display clusters of verb–object combinations for the individual verbs, and the mutual collocational strengths of the combinations. As we shall see, there is reason to argue that collocational preferences in concrete verb–object combinations need to be accounted for in the modelling of verbal complementation.
5.1 Open-choice vs. idiom principle
We will first consider the relationship between the open-choice and the idiom principle. An example of a verb whose objects seem to be almost entirely open choice is mangle ‘lack’, allthough there are considerable differences in association strength (measured in log-likelihood) between the collocates. The 30 strongest object collocates of this verb are provided in Table 7. The three collumns in the middle of the table give frequency of the combination (F1-2), frequency of the verb (F1), and frequency of the noun (F2), respectively. The basis for all calculations is the 2.49 million extracted tokens (N) in the verb–object dependency relation.
Table 7. The strongest object collocates of mangle ‘lack’.

F1-2 = frequency of verb + noun, F1 = frequency of verb, F2 = frequency of noun, N = verb–object tokens
It is indeed difficult to come up with a noun that would not be possible in the object slot of this verb. However, also with mangle ‘lack’ there are idiomatic, less transparent combinations. An interesting example is mangle sidestykke ‘be unparalleled’:
-
(3)
It is interesting to note, given the data in Table 7, that about 32% of the examples of sidestykke in the database co-occur with mangle. It is not conventional, for example, to say that something *har sidestykke ‘has a parallel’. There is, however, one other verb with which sidestykke is even more strongly associated, namely savne ‘miss’ (log-likelihood: 221), which accounts for the 68% remaining combinations with this noun in the material. Hence, the noun sidestykke shows a very strong tendency to co-occur with these two verbs. We also note that the idiomatic (in the sense of non-transparent) combination mangle sidestykke is not at all the strongest collocational preference of mangle (measured in log-likelihood). The strongest combination, mangle kunnskap ‘lack knowledge’ is both much more frequent (206 versus 10) and has a much higher log-likelihood value (817 versus 75), which means that log-likelihood does not target semantic non-transparent combinations specifically. Rather, the findings seem to be more in accordance with the broader understanding of idiomaticity in Sinclair’s (Reference Sinclair1991) idiom principle, see the discussion in Section 2 above. Nevertheless, examplar clusters such as those in Table 7 seem to be a good point of departure to identify also non-transparent combinations.
Overall, the objects of the verb mangle ‘lack’ seem to be almost entirely open choice, however, and this verb has a relatively low mean log-likelihood value of 51, based on 103 object types. When we now turn to the verb kaste ‘throw’, we observe a higher incidence of idiomatic combinations among the strongest collocates, and this verb has a mean log-likelihood value of 315, based on 31 object types. We remember from Section 2 that the mean log-likelihood value of all verbs is 219 (see Table 5 above). As is evident from Table 8, the strongest collocates of kaste are included in idiomatic expressions like kaste et blikk på noe ‘glance at something’, kaste lys over noe ‘shed light on something’, and kaste skygge over noe ‘cast a shadow over something’, whereas the perhaps most prototypical use of kaste, as in kaste stein ‘throw stone’, occurs with a slightly lower value of association (log-likelihood).
Table 8. The strongest object collocates of kaste ‘throw’.
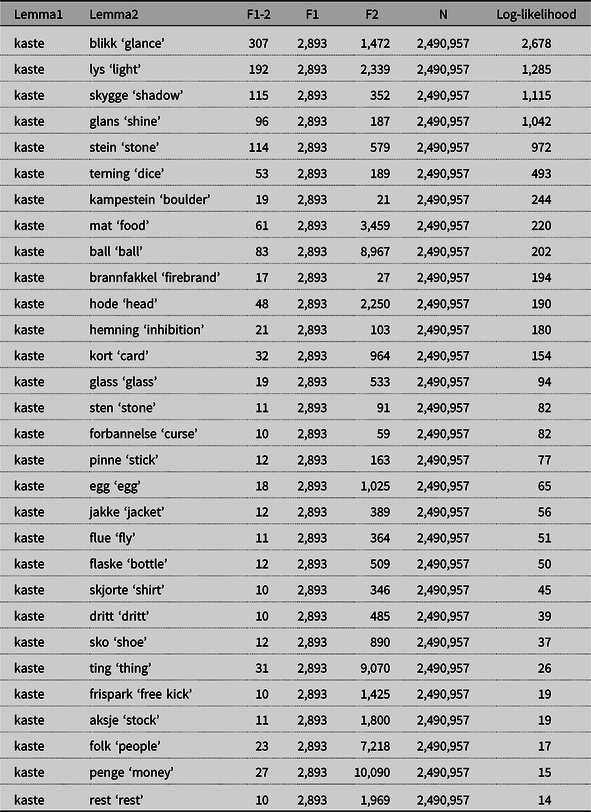
F1-2 = frequency of verb + noun, F1 = frequency of verb, F2 = frequency of noun, N = verb–object tokens
Consider, however, also a combination like kaste jakka ‘take off the jacket’, which has an idiomatic flavour, but is not frequent enough (12) to get a very high log-likelihood score (56). Again, less transparent combinations are not necessarily the ones that are most strongly associated, although the strongest collocates of kaste are indeed idiomatic. Here is an example with kaste jakka:
-
(4)
To kaste jakka normally does not mean that you necessarily throw it at all, but rather that the jacket is simply taken off, and such meanings of specific verb–object combinations are hard to pin down if complementation is handled in terms of general syntactic and/or semantic categories. As Sinclair (Reference Sinclair1991:71) argues, ‘Intuitively, we feel that some instances of a word are quite independently chosen, while in other cases we feel that the word combines with others to deliver a single multi-word unit of meaning’. As we have already seen, however, and as we shall see below, collocational preferences are also found among combinations that are otherwise fully transparent, equally grammatical and equally understandable.
Finally in this section we will discuss a verb whose object slot seems to be very restricted, namely avlegge ‘submit’. Even though the database is restricted to collocates that occur at least 10 times in NoWaC, and there are more objects attested with this verb than the ones represented among the strongest collocates, the data do suggest that the object slot is subject to very specific restrictions, which exemplar representations let us account for in a straightforward manner. For example, one can avlegge sin stemme ‘vote’, but one cannot *avlegge sin mening ‘give one’s opinion’. Furthermore one can avlegge rapport ‘report’, but one cannot avlegge informasjon ‘inform’ or avlegge en tekst ‘submit a text’, for example. Hence, there are very specific restrictions on the nouns that can occur as objects with this verb, and the best description we can give might just be a list of the most significant exemplars, as provided in Table 9.
Table 9. The strongest object collocates of avlegge ‘submit’.

F1-2 = frequency of verb + noun, F1 = frequency of verb, F2 = frequency of noun, N = verb–object tokens
Even though avlegge ‘submit’ is more restricted in its range of objects (seven object types) than was the case with kaste ‘throw’ (31 object types), its strongest verb–object combinations are much less frequent than the strongest combinations of kaste ‘throw’, and the mean log-likelihood value of avlegge is also somewhat lower at 228, which is still considerably higher than the open-choice verb mangle ‘lack’, which we saw above has a mean log-likelihood value of 51. For these verbs, then, open-choice correlates with a low mean log-likelihood value, but it is not the case, as we also saw for the material as a whole in Section 4, that high mean log-likelihood necessarily correlates with a low number of object types. As we just saw, avlegge has a lower mean log-likelihood value than kaste. Consider also a case like spille ‘play’, which has a mean log-likelihood as high as 601, and which still occurs with 131 object types. This is due to very strongly associated combinations such as spille rolle ‘play role’ (log-likelihood 41,990), spille kamp ‘play match’ (log-likelihood 9,228), spille fotball ‘play football’ (log-likelihood 4,710), spille hovedrolle ‘play leading part’ (log-likelihood 2,971), and spille musikk ‘play music’ (log-likelihood 1,411) in the top part of the cluster, which result in a high mean log-likelihood value, despite the fact that the weakest combinations with this verb in fact have negative log-likelihood scores. Examples are combinations like spille menneske ‘play human being’ (log-likelihood –20) and spille person ‘play person’ (log-likelihood –22). In conclusion, it indeed seems that the degree of open choice versus idiomaticity has to be assessed based on the verb–object clusters of individual verbs, which underscores the large lexical variation discussed for the material as a whole in Section 4.
The limited studies of individual verbs and verb pairs presented in this section suggest that specifying complementation in terms of general syntactic and semantic categories does not suffice to account for verbal complementation as attested in usage. Collocational preferences seem to be of great importance, also for syntactic combinations that are normally accounted for at the grammatical pole of the lexicon-grammar. As Sinclair (Reference Sinclair1991) argues, the idiom principle might be more important in language than the open-choice principle. We will see this more clearly when we consider closely related alternatives with semantically similar verbs in the following section.
5.2 Differences between semantically similar verbs
Verb–object collocations are very useful in distinguishing among semantically similar verbs. Consider for example the verbs in Table 10, bytte and skifte, both meaning ‘change’, which are intuitively very close in meaning.
Table 10. The strongest object collocates of the verbs bytte and skifte, both meaning ‘change’.
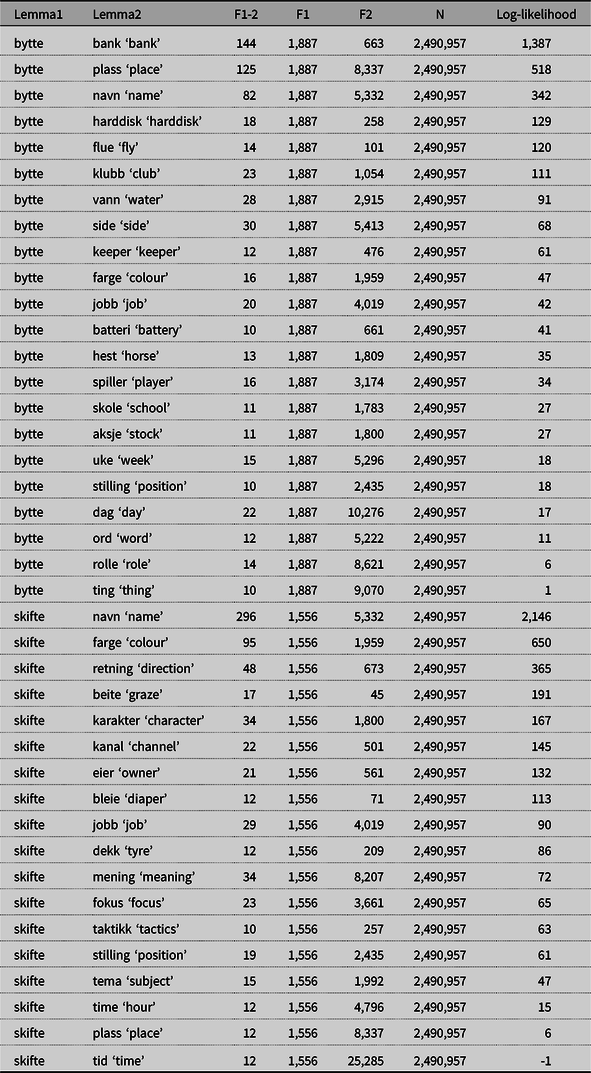
F1-2 = frequency of verb + noun, F1 = frequency of verb, F2 = frequency of noun, N = verb–object tokens
The verb bytte occurs with 22 objects passing the 10-occurrences threshold, whereas skifte occurs with 18 such objects. Out of these, four nouns are common, whereas the rest differ between the two verbs. This already provides a good basis for distinguishing between them.
Let us start with some of the collocates that differ among the two verbs. Consider first the combination skifte retning ‘change direction’, which is much more conventional with skifte than with bytte:
-
(5)
The same is true for the combination skifte mening ‘change opinion’:
-
(6)
Preferences such as those in (5) and (6) above involve alternatives that are fully grammatical, semantically transparent, and fully understandable. Still, the (a)-alternatives are clearly preferred in both cases, and preferences such as these need to be accounted for. As Goldberg (Reference Goldberg2019) argues, people treat language as a normative enterprise. As members of a speech community, we normally strive to talk the way others do, and we are able to judge utterances as more or less in accordance with conventional usage in the speech community.
Psychologically, Goldberg (Reference Goldberg2019 and elsewhere) argues that we can distinguish between closely related constructions based on what she calls statistical preemption, which seems to fit nicely with preferences between alternatives such as those in (5) and (6). Statistical preemption is hypothesised to happen when two different constructions compete to convey the same meaning in the same context. In such cases, the more entrenched alternative will be felt to be the right, more conventional way of expressing the meaning in point, even though both alternatives are equally grammatical and understandable. For Goldberg, statistical preemption is used to explain the partial productivity of verbs in different ranges of argument structure constructions, and it might be an interesting mehanism to account also for alternatives among closely related examplar clusters within the same argument structure construction, of the kind we have studied in this section. Hence, the reason we prefer skifte mening ‘change opinion’ (log-likelihood 72) instead of bytte mening (not attested in the material) might be due to statistical preemption.
In the cases we have discussed, intuitional preferences seem to coincide with association measures, which suggests that collocation analyses might be an interesting point of departure for exploring the mutual strength of exemplar representations, also in terms of mental representations. Combinations with strong collocational associations tend also to be intuitively more conventional than the same objects with the alternative verb.
As already mentioned, there are also some strong object collocates that are common among the two verbs. As we see in Table 10, the nouns plass ‘place’, navn ‘name’, jobb ‘job’, and stilling ‘position’ all turn up among the strongest collocates of both verbs. For the combination bytte plass ‘change place’ (log-likelihood 518) the association is much stronger than is the case for skifte plass ‘change place’ (log-likelihood 6), and there is also an intuitive difference in preference among these alternatives.
For the remaining common objects, however, navn ‘name’, jobb ‘job’, and stilling ‘position’, they seem to a much higher extent to be equally conventional alternatives, and more specific contexts are needed to distinguish between them. Starting with the object navn, we see that this object is both more frequent with skifte than with bytte (296 versus 82 occurrences) and has a much stronger log-likelihood (2,146 versus 382). On the other hand, navn is a highly ranked object of both verbs (1 versus 3) and, intuitively, both alternatives are at least close to equally conventional according to the native speaker intuition of the author, perhaps with a slight preference for skifte navn. A slight preference is also felt for skifte stilling (19 occurrences, log-likelihood 61) over bytte stilling (10 occurrences, log-likelihood 18), whereas bytte jobb (20 occurrenes, log-likelihood 42) and skifte jobb (29 occurrences, log-likelihood 90) seem intuitively very hard to separate in many contexts. A context where these alternatives are not interchangable, however, is if I ask Skal vi bytte jobb? ‘Do you want to change jobs?’, which can mean a mutual exchange of jobs, whereas this is not possible with Skal vi skifte jobb? ‘Do you want to change jobs?’, which can only mean that we both change to different jobs that none of us have at the moment of speaking. Even though close to equal alternatives exist, however, we can certainly distinguish between bytte and skifte on the basis of their strongest object collocations.
Finally, also with the verbs in this section we find strongly idiomatic, non-transparent combinations; the collocate beite ‘graze’ of skifte is a case in point:
-
(7)
Let us now consider another verb pair, namely anvende and benytte, both meaning ‘make use of’, see Table 11. These are also semantically very similar verbs, but the database suggests that the latter collocates strongly with objects such as anledning ‘occasion’ and sjanse ‘chanse’, which are not associated with the former.
Table 11. The strongest object collocates of anvende and benytte, both meaning ‘make use of’.

F1-2 = frequency of verb + noun, F1 = frequency of verb, F2 = frequency of noun, N = verb–object tokens
Some examples are provided in (8):
-
(8)
Native speakers of Norwegian are very unlikely to produce utterances such as those in (8b, d), which demonstrates that they probably have access to exemplar-level complementation preferences for these verbs.
Summing up, we have seen in our zooming in on a few individual verbs and verb pairs, that although the variation among verbs is considerable, the idiom principle seems to play an important role in verbal complementation. Due to the great variation, however, it seems clear that the assessment of the relationship between the open-choice and the idiom principle needs to be carried out on the basis of detailed studies of individual verbs. We have also seen that studying the exemplar clusters of semantically similar verbs allows us to specify the differences between them in a way that would be difficult on the basis of abstract semantic categories. The comparison of closely related verb pairs also highlights the fact that clear collocational preferences are found between alternatives that are otherwise fully grammatical, semantically transparent and equally understandable.
6. Concluding remarks
In this article, a database of verb–object combinations semi-automatically extracted from a large electronic corpus has been presented. A database of this kind could be of interest not only to theoretically oriented linguists, but also to lexicographers and language teachers. While we have concentrated on extraction of verbal complements, similar extractions can be used to retrieve additional datasets for other important linguistic patterns, such as adjective–noun combinations. A great part of the merit is due to a number of freely available tools for NLP and to freely available resources for Norwegian: NoWaC, the NDT treebank, the Oslo–Bergen tagger, Maltparser and the UCS toolkit.
The database has been used to explore a bottom–up approach to verbal complementation in terms of specific verb–object combinations. Following Herbst (Reference Herbst, Schmid and Handl2010), it has beed argued that valency and collocations refer to closely related phenomenon in language, differing mainly in their level of abstractness. As large-scale valency studies have shown, verbs normally appear in a range of different valency patterns; at the same time, however, such patterns are to a large extent idiosyncratically restricted, which means that verbal complementation seems to a high degree to be lexically determined. The overall importance also of collocational preferences in verb–object combinations is clearly demonstrated by the fact that the 26,425 verb–object types passing the 10-occurrences threshold (in the filtered, raw material), which amounts to 5% of the verb–object types, account for as much as 64% of the 2.5 million tokens we started out with. It has also been demonstrated that the collocational strength of individual verbs, measured in terms of the mean log-likelihood values of their verb–object combinations, is subject to great variation.
In addition to the overall importance of recurring combinations, we have explored the verb–object combinations of some individual verbs and some verb pairs. Clearly, collocational preferences in usage, as represented in the corpus, play an important role in verb–object combinations. In the cases we have explored in depth, it also seems safe to assume that speakers do distinguish between and prefer certain conventional verb–object combinations to other equally grammatical, equally transparent and equally understandable alternatives, and that speakers have access to complementation information at the level of exemplars. Hence, rich memory of specific verb–object combinations seem to be needed in a plausible account of complementation. Needless to say, however, it remains to be explored to what extent measured collocational associations are in accordance with speaker intuitions for the material as a whole.
The database of verb–object combinations constitutes a solid empirical basis for accurate descriptions of the differences between semantically similar transitive verbs, and measures of collocational strength allow us to model the mutual strength between the exemplars in the clusters. In my view, exemplar clusters of the strongest noun collocates of verbs can yield more accurate descriptions of verb–object combinations than approaches operating with general syntactic and/or semantic features only, and I would argue that the role of collocational preferences in grammatical relations should be explored further also for a language like Norwegian.
Acknowledgements
The initial computational part of this work was carried out by Emiliano Guevara. I would like to thank him for crucial help with the data and for his valuable comments and contributions to previous versions of this paper. The computations for the data extraction was performed on the Abel Cluster, owned by the University of Oslo and the Norwegian metacenter for High Performance Computing (NOTUR), and operated by the Research Computing Services group at USIT, the University of Oslo IT department. We would like to thank them for their professional support and assistance. I would also like to thank the reviewers of NJL for valuable comments.
Appendix. The verbs removed in the cleaning of the data
Table A1. List of intransitive and transitive verbs removed in the cleaned data.
